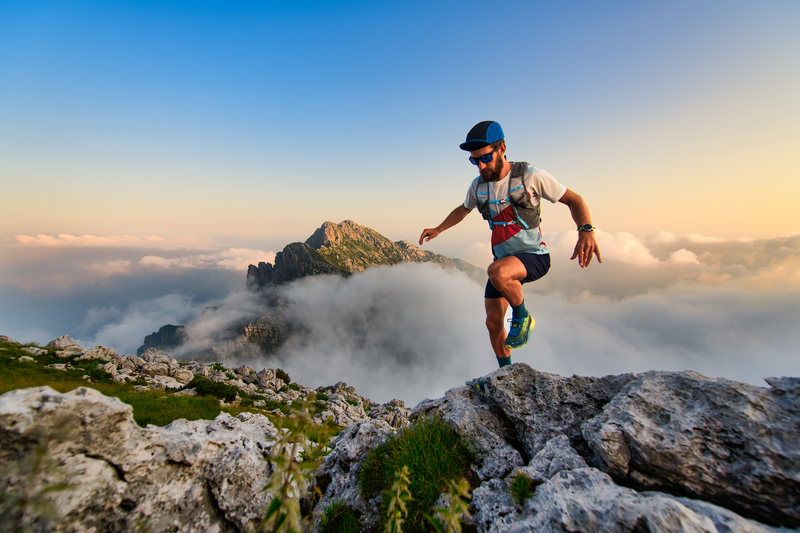
95% of researchers rate our articles as excellent or good
Learn more about the work of our research integrity team to safeguard the quality of each article we publish.
Find out more
EDITORIAL article
Front. Artif. Intell. , 13 June 2023
Sec. Machine Learning and Artificial Intelligence
Volume 6 - 2023 | https://doi.org/10.3389/frai.2023.1213419
This article is part of the Research Topic Deep Learning with Limited Labeled Data for Vision, Audio, and Text View all 4 articles
Editorial on the Research Topic
Deep learning with limited labeled data for vision, audio, and text
Deep learning has made significant strides in computer vision, audio, and natural language processing over the past decade. However, the success of deep learning models is often dependent on the availability of labeled data. Labeled data, where each data point is accompanied by a corresponding label, is crucial for training supervised deep learning models. Unfortunately, in many real-world applications, acquiring labeled data can be expensive, time-consuming, and sometimes even impossible. Hence, it is imperative that methods be developed to enable deep learning work effectively where limited labeled data is available. In this issue, we present works that tackle the problem of learning with limited data having real-world impact in various fields such as sports and medicine. The articles talk about methods that approach the problem from different perspectives and offer innovative solutions to develop state-of-the-art models.
In Jersey number detection using synthetic data in a low-data regime (Bhargavi et al.) generate synthetic datasets to address the problem of data scarcity when training models to identify players in American football. They used pre-trained models to identify potential image areas with jersey numbers and ended up with a limited size dataset with high class-imbalance after human annotations. To counter these issues, the authors created a synthetic dataset leveraging various image augmentation techniques and pre-existing image datasets. Using these methods, the authors showed a significant improvement in model performance when applied in the wild.
In “Active learning for data efficient semantic segmentation of canine bones in radiographs”, Moreira da Silva et al. address the challenge of efficiently selecting datapoints for annotation that would maximize the model's performance and reduce the redundancy in human annotations. Leveraging a model trained with a small, annotated training set, the authors selected datapoints from a larger unlabeled set to be given for human annotation in a step-wise approach where the selection criteria aimed to favor samples that are either very diverse from the existing training set or for which the trained model is not very confident on its predictions. The authors showed that smartly selecting samples for annotation can lead to high performing models trained using only a fraction of the data, with reduced time complexity.
Finally, Smith and Conovaloff explore the paradigm of one-shot learning where the assumption is that there is only labeled sample per class available for training. The authors rely on semi-supervised learning techniques to learn from unlabeled data-points. Specifically, they employ the techniques of pseudo-labeling and self-training and pick confidently labeled samples from unlabeled dataset to be added to their labeled training set. Unlike active learning there was no human effort involved at all. Finally, the authors show that selecting an iconic datapoint as the prototype training example for one shot training can allay the need of labeling a large number of samples for training deep neural networks.
We thank the authors, reviewers, and editors for all their efforts in making this issue a reality. We hope our readers enjoy the articles and get inspired to explore this field of deep learning.
All authors listed have made a substantial, direct, and intellectual contribution to the work and approved it for publication.
The authors declare that the research was conducted in the absence of any commercial or financial relationships that could be construed as a potential conflict of interest.
All claims expressed in this article are solely those of the authors and do not necessarily represent those of their affiliated organizations, or those of the publisher, the editors and the reviewers. Any product that may be evaluated in this article, or claim that may be made by its manufacturer, is not guaranteed or endorsed by the publisher.
Keywords: deep learning, few shot learning, semi-supervised, neural network, active learning, semantic segmentation
Citation: Orescanin M, Smith LN, Sahu S, Goyal P and Chhetri SR (2023) Editorial: Deep learning with limited labeled data for vision, audio, and text. Front. Artif. Intell. 6:1213419. doi: 10.3389/frai.2023.1213419
Received: 27 April 2023; Accepted: 02 May 2023;
Published: 13 June 2023.
Edited and reviewed by: Georgios Leontidis, University of Aberdeen, United Kingdom
Copyright © 2023 Orescanin, Smith, Sahu, Goyal and Chhetri. This is an open-access article distributed under the terms of the Creative Commons Attribution License (CC BY). The use, distribution or reproduction in other forums is permitted, provided the original author(s) and the copyright owner(s) are credited and that the original publication in this journal is cited, in accordance with accepted academic practice. No use, distribution or reproduction is permitted which does not comply with these terms.
*Correspondence: Marko Orescanin, bWFya28ub3Jlc2NhbmluQG5wcy5lZHU=
Disclaimer: All claims expressed in this article are solely those of the authors and do not necessarily represent those of their affiliated organizations, or those of the publisher, the editors and the reviewers. Any product that may be evaluated in this article or claim that may be made by its manufacturer is not guaranteed or endorsed by the publisher.
Research integrity at Frontiers
Learn more about the work of our research integrity team to safeguard the quality of each article we publish.