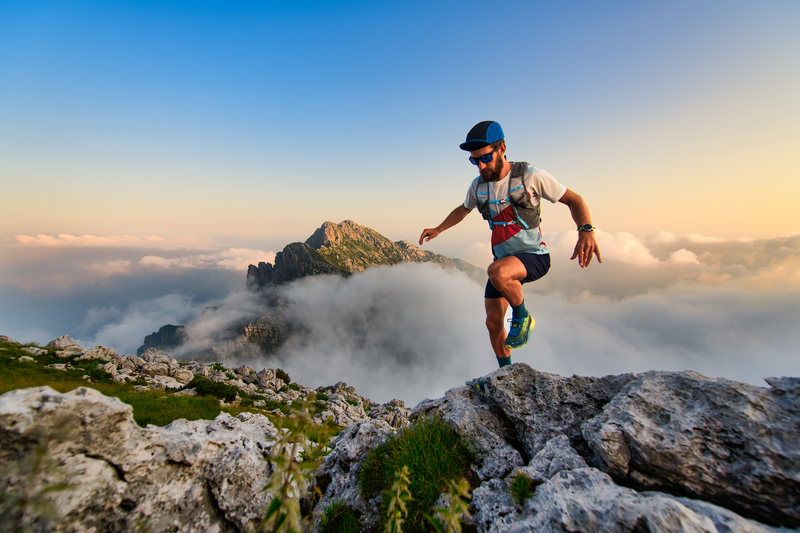
94% of researchers rate our articles as excellent or good
Learn more about the work of our research integrity team to safeguard the quality of each article we publish.
Find out more
CONCEPTUAL ANALYSIS article
Front. Artif. Intell. , 22 June 2023
Sec. AI in Business
Volume 6 - 2023 | https://doi.org/10.3389/frai.2023.1093712
This article is part of the Research Topic Artificial Intelligence (AI) Ethics in Business View all 9 articles
In this paper, we argue that we cannot expect that AI systems—even given more data or better computational resources—will be more ethical than the humans who develop, deploy and use them. As such, we advocate that it is necessary to retain the responsibility for ethical decision-making in human hands. In reality, however, human decision-makers currently do not have the ethical maturity to meaningfully take on this responsibility. So, what to do? We develop the argument that to broaden and strengthen the ethical upskilling of our organizations and leaders, AI has a crucial role to play. Specifically, because AI is a mirror that reflects our biases and moral flaws back to us, decision-makers should look carefully into this mirror—taking advantage of the opportunities brought about by its scale, interpretability, and counterfactual modeling—to gain a deep understanding of the psychological underpinnings of our (un)ethical behaviors, and in turn, learn to consistently make ethical decisions. In discussing this proposal, we introduce a new collaborative paradigm between humans and AI that can help ethically upskill our organizations and leaders and thereby prepare them to responsibly navigate the impending digital future.
As Artificial Intelligence1 (AI) systems are increasingly viewed as imperative for managing costs, increasing efficiencies, and raising productivity, organizations are increasingly adopting AI in a variety of decision-making contexts (Cockburn et al., 2018; Trunk et al., 2020; Dordevic, 2022). Organizational deployments of AI are especially noticeable in areas where they can enhance employees” ability to analyze and solve problems (e.g., Huang et al., 2019; Metcalf et al., 2019; Gregory et al., 2021). For example, AI is being adopted to facilitate human resource activities (Gee, 2017; Hoffman et al., 2018): such as recruitment and selection (Woods et al., 2020), structuring work schedules, making decisions for work teams, providing advice to those in authority positions (De Cremer, 2020; Parent-Rocheleau and Parker, 2022) and providing services to customers (Yam et al., 2021). Given recent advances in AI techniques—as notably seen in the tremendous performance improvements of large language models (LLMs) like GPT-3 and GPT-4—it is expected that the breadth and depth of organizational applications of AI will only continue to rise in the near future (cf. Hovy and Spruit, 2016; Felten et al., 2023). In turn, critical and morally sensitive decision-making tasks will increasingly be delegated to these intelligent technologies (Feuerriegel et al., 2022).
As we know, with great power also comes great responsibility. As organizations grow increasingly dependent on AI, a larger number of people will become vulnerable to AI-led decisions, and hence the more salient and urgent are the concerns about whether or not these AI systems will be ethical (De Cremer and Kasparov, 2021). That is, with increased dependence on AI, critical questions will arise about whether AI will harm the interests of the organization, be inclusive and respectful in treating its employees, and adhere to normative rules and generate fair decisions and outcomes (Lee et al., 2017; Kellogg et al., 2020).2 Given this situation, it is then also no surprise that calls for “ethical AI” and “responsible AI” have been gaining prominence in recent years (Anderson et al., 2018).
The emerging field of AI ethics is expansive in its scope—spanning a range of multidisciplinary perspectives on how to ensure that AI systems accord with norms of accountability, fairness, legality, transparency, and more. One aspect in this field that has received considerable attention—and which is the focus of the present paper—concerns the ethical consequences for the human parties that are on the receiving end of an artificial agent's recommendations. Research and policy-making efforts in this domain have been largely concerned with developing methods for ensuring that AI systems act fairly, identifying which party is accountable if anything goes wrong, and employing these systems in ways that their decisions are transparent and explainable to humans. Discussions of these key “principles” for ethical AI—i.e., fairness, accountability, transparency, etc.—are usually accompanied by various procedures and rules for ensuring compliance with these principles. However, as we will argue, these various principles and procedures will necessarily prove insufficient if our ultimate goal is to delegate ethical decision-making to AI systems. Ethical decision-making requires more than simply adhering to pre-defined rules and procedures: it also requires moral agency, robust intentions to act in moral ways even in unprecedented circumstances, and the ability to take responsibility for one's actions. As we will discuss, these requirements are why ethical decision-making ought to remain in human hands.
Even so, organizations seem increasingly willing to leave AI systems in charge of making morally-sensitive decisions. Our paper cautions against this tendency, and argues for the immutable role of humans in ethical decision-making. At the same time, this does not mean that AI has no role to play in helping organizations make more ethical decisions. Recognizing that human decision-making is itself fraught with biases and moral flaws, we propose a new collaborative paradigm in which AI systems can serve as an information tool for ethically upskilling human decision-makers, helping us decide and act morally in a more consistent manner. In so doing, we discuss how AI can—and cannot—help organizations become more ethical.
The rest of this paper proceeds as follows. In Section 2, we discuss the reasons why, despite a recent explosion of interest in issues of ethical AI, AI systems will remain fundamentally incapable of performing ethical decision-making in organizations. We then explore reasons why contemporary organizations seem to be ignoring this reality by adopting overinflated expectations about the moral properties of AI, and explicate the dangers of doing so. In turn, we argue that AI is best viewed as a “mirror”: one that reflects back to us the biases, discriminatory patterns and moral flaws that are deeply entrenched in our human cognition and social institutions. Subsequently, in Section 3, we elaborate on some ways in which human decision-making is itself routinely biased and flawed, and discuss the urgent need for “ethical upskilling”. In turn, we detail how AI systems—because of how they are trained to capture and emulate human behavior at scale—can play an invaluable role in these upskilling efforts. Finally, Section 4 concludes by discussing some implications of our view for organizations and society at large.
Extant discussions on AI ethics have primarily progressed through the development and adoption of “principles”, “ethics codes”, “guidelines”, and “best-practice recommendations”, by policymakers, organizations and civil society groups alike. Government reports such as the European Union's “Ethical Guidelines for Trustworthy AI” (European Commission, 2019), and the Government of Singapore's “Model AI Framework” (PDPC, 2020), and industry reports on “Responsible AI” from Google (2022) and Microsoft (2022), are representative examples of this approach. Indeed, as Jobin et al. (2019) and Fjeld et al. (2020) demonstrate, there has been a recent explosion of documents outlining principles for AI ethics, and growing convergence in key principles—fairness, transparency, accountability, etc.—that are deemed to be fundamental for “ethical AI”.
These principles, however, have been subject to criticism in recent times. Hagendorff (2020), for instance, characterizes principles as “essentially weak”, since they are by design unenforceable by law, and hence cannot be meaningfully used to hold companies that deploy harmful AI systems to account. Related complaints about “ethics-washing”—i.e., using the language of principles in ambiguous and superficial ways to cover up wrongdoings, and “ethics-bashing”—i.e., reducing complex moral principles into simplistic “check-lists” and procedural rules—are increasingly commonplace (Bietti, 2020; Hao, 2020), and reflect growing skepticism about whether principle-led approaches are sufficient for ensuring that AI systems are deployed toward ethical ends.
In our view, however, the main problem with principle-led approaches cuts much deeper. Principles and guidelines are, in essence, fundamentally concerned with assessing whether decision-making outcomes can be seen as ethical or not. They focus mainly on the “what” of ethical decision-making, rather than the “how” or “why”: narrowing down our assessments of whether an AI system is “ethical” or not primarily in terms of whether their outcomes align with pre-defined rules and protocols. This reflects a “law of ethics” approach (Anscombe, 1958; Rességuier and Rodrigues, 2020): whereby our previous assessments of which situations are and aren't ethical are codified in terms of fixed rules that are meant to guide future actions.3 Contemporary approaches to “embed” ethics in AI systems—by incorporating existing ethical rules and principles as design constraints—are representative of this tendency (cf. Wallach and Allen, 2009; Conitzer et al., 2017; McLennan et al., 2020). However, crucially, to be an ethical decision-maker in an organization, it is not sufficient merely to simply optimize for good over bad outcomes.4
When we expect that AI systems will be ethical decision-makers in organizations, we are ultimately expecting that these systems would be able to reason, think and make judgment calls about the most morally appropriate course of action, across a wide range of managerial contexts (Mittelstadt, 2019; De Cremer, 2022). Crucially, given that organizations operate in a volatile, uncertain, complex, and ambiguous world, delegating ethical decision-making to AI systems means that we would need them to evaluate and respond to moral situations that they have not encountered previously, to determine the most appropriate, respectful, and moral decision at any given moment. To meet such expectations, AI systems would need to have a sense of moral agency, robust intentions to act in moral ways even in uncertain situations, and the ability to take responsibility for their actions (De Cremer and Moore, 2020; Hagendorff, 2020).
In our view, moral decision-making is an ability that requires decision-makers to understand what their moral responsibilities are, to extend care and awareness to others and their concerns, and to assess via an internal moral compass what is the most morally-appropriate course of action to take in unprecedented and constantly-evolving situations. Recent scholarly work has noted that ethics is a constantly renewed ability to “see the new” (Laugier and Chalier, 2013; Rességuier and Rodrigues, 2020), and in turn, to make culturally-sensitive and situationally-aware choices about which moral actions to take in any given context (De Cremer, 2022). This line of reasoning, in turn, implies that ethics is not an ability that intelligent machines could reasonably possess—clearly, it is fundamentally a human ability. Even though AI systems can be superior in executing certain well-defined tasks, by replicating what humans would do in similar situations, they are in essence clueless about the meaning and function of their task in its broader context. Moreover, crucially, their knowledge can often fail to generalize in new morally-significant situations (Mitchell, 2019). This entails that intelligent technologies are fundamentally limited when it comes to executing certain intelligent tasks that require quintessentially human traits—such as ethical judgments, symbolic reasoning, managing social situations, and creative ideation (Brynjolfsson and McAfee, 2014).
Even though AI has no moral agency, why does it seem then that organizations seem so predisposed to attribute agentic properties to AI? In our experience, many organizational leaders seem increasingly persuaded by a common narrative propagated by “Big Tech” companies—that technology may well be the real (and only) solution to our social and ethical problems. This view reflects a mindset of “technosolutionism” (Morozov, 2013): a unilateral preference for technically-engineered solutions to complex social problems. Technology companies today seem to be increasingly popularizing this mindset. For example, the tech giant Google has been promoting “ethics-as-a-service”: a technology-first effort to help other organizations “navigate the tricky ethics of AI” (Simonite, 2020). In so doing, Google is inducing a mindset among business leaders that if intelligent technologies reveal biased decision-making outcomes, the solution is simply to fix the technology. As a result, business leaders increasingly think that ethics can now simply be delegated to intelligent machines, and therefore that they do not need to invest in becoming better and more responsible leaders themselves (De Cremer and Kasparov, 2021). In other words, thanks to AI, today's business leaders, with implicit endorsement from tech companies, are learning to think that it is easier to “fix” the biases of technologies than it is to retrain unethical humans to do the right thing (De Cremer and Narayanan, 2023).
It is crucial to critically appraise the growing influence of these technosolutionist narratives in the business world, particularly because they give rise to two important psychological phenomena that are likely to reinforce misplaced beliefs about the moral agency of AI, and in turn, make ethical decision-making in organizations much harder to achieve. These two phenomena are: (a) magical thinking about the humanlike properties of AI, and (b) increased confusion about who should take responsibility for AI-augmented decisions.
(a) Magical thinking: Technosolutionist narratives lead people to acquire overinflated beliefs about what AI systems can and cannot do (cf. Vicsek, 2020; Bareis and Katzenbach, 2021). In turn, this results in people reading agency, intentionality and humanlike intelligence into even the most mechanistic and programmed actions performed by AI systems. When we anthropomorphize AI systems in this way, we attribute to them magical—and humanlike—powers, and in so doing, come to believe that they can be inherently good or bad—like any other moral agent. As magical thinking about the powers of AI becomes increasingly commonplace, it seems increasingly socially acceptable to think that intelligent technologies could be held morally responsible for their actions and decisions.
(b) Confusion about responsibility-attribution: As people come to (mis)attribute humanlike and agential properties to AI, the notion that technological systems could be held morally responsible for the decisions made based on its output now starts to seem plausible. This can lead to deep confusion in real-world decision-making contexts, as those who work with AI systems to make decisions are no longer able to think clearly about who (i.e., workers or the AI system) should be held responsible for enacting various work-role responsibilities (see e.g., Hanson and Bar-Cohen, 2009; Brynjolfsson et al., 2018). As a result, AI systems may make employees feel more uncertain about the expectations associated with their work roles (e.g., Rizzo et al., 1970), and in turn, come to disengage from the moral ramifications of their work.
In our view, these beliefs emerging out of prevalent technosolutionist narratives are profoundly detached from reality. To understand why this is the case, however, it is necessary to take a closer look at how contemporary AI systems are developed, and what they are actually capable of.
A full discussion of the technicalities of the machine learning (ML) techniques methods5 that underlie contemporary AI systems falls outside the scope of this paper. However, to better understand why the trends we discussed above (i.e., of attributing moral agency and responsibility to AI) are so deeply misplaced, it is important to have at least a broad understanding of what exactly contemporary AI systems are (and aren't) capable of. Our overall view is perhaps best summed up by Kate Crawford's famous slogan: “Most AI is neither artificial, nor intelligent” (Crawford, 2021).
In broad terms, ML models are “trained” by letting them analyze large and highly-dimensioned datasets to identify generalizable patterns—typically either in a “supervised” manner (i.e., with human-labeled examples of correct and incorrect inferences that the model learns to extrapolate patterns from), or in an “unsupervised” manner (i.e., where the model is given some sort of reward/cost function to optimize during its training). Even though engineers do not explicitly code formal inference rules or decision-making logics into their models, they still need to make numerous consequential decisions—to define the problem that needs solving, set the background conditions under which the model will be trained, define the reward/cost function that needs to be optimized, etc.—all of which entails considerable tinkering and experimentation (Agrawal et al., 2018). Moreover, as noted earlier, the value of contemporary AI systems is based firmly on the existence of “big data” (Brynjolfsson and McAfee, 2012; Varian, 2014). However, in most cases, the large datasets used for training and testing AI models are also indelibly shaped by human choices—particularly those of the “ghost workers” who label, clean, and verify data to make them usable for AI developers (Gray and Suri, 2019). Because of these significant human decisions that shape AI systems at every step of the way—from data-labeling, to training, to testing, to deployment—it seems like a misnomer to label these systems as truly “artificial”.
Even so, at first glance, these systems at least appear to be intelligent, in certain important ways. ML models can spot patterns in large and highly-dimensioned datasets that no human could reasonably parse, and base their predictions on complex patterns that would have been otherwise invisible to humans (cf. Burrell, 2016; Joque, 2022). Indeed, if humans were asked to parse comparably large amounts of complex data, our approach would inevitably resort to heuristic-based thinking, which would likely yield less useful patterns and insights compared to ML models (Korteling et al., 2018). Moreover, with more data and more powerful computational resources, AI systems can improve their performance over time, and learn to make even more accurate judgments about the situations that are represented in their training data. For these reasons, AI systems can sometimes be seen as viable replacements for human intelligence and intuition in certain use-contexts, because their self-learning capabilities can reliably mimic human behavior and actions by using accumulated data from behavioral observations and other such data sources (Russell and Norvig, 2016).
However, this “intelligence” has deep-seated limitations. ML techniques may reveal numerous explicit and implicit patterns in the datasets they are trained on—even patterns that humans would have been unable to see—but crucially, they do not reveal anything new that was not previously contained within this data (cf. Bender et al., 2021). This is an important limitation especially when it comes to expecting ethical decision-making from AI. As we have argued above, ethical decision-making requires the ability to deal with new situations—assessing what is morally appropriate, respectful, and socially acceptable in any given situation cannot be simply generalized from previous similar situations. Every ethical decision is a new decision—and even if trends from previous decisions might contain some clues about how to respond in a new decision-making context, they cannot fully determine what is morally appropriate in a new context.
Crucially, even the most sophisticated ML techniques cannot infer meaning from learning—as they lack the rich conceptual and emotional knowledge humans have about objects and experiences, it fails to draw analogies, and they are limited in understanding frames of references to engage in subtle contextual, symbolic and cultural interpretations of human language (Nath and Sahu, 2020; Mitchell, 2021; Ricci et al., 2021; Toews, 2021). As such, it is unreasonable to attribute intentionality, in any meaningful sense of the term, to AI. Indeed, intentionality requires that the agent has mental states and awareness that motivates them to set out to accomplish a goal to satisfy one's concerns, motives, and desires, which, as we explained earlier, are abilities AI does not have and therefore cannot be regarded as an agent acting with intent (De Cremer and Kasparov, 2022).
As we have argued, ethical decision-making is fundamentally dependent on having the right intentions, extending relational care to understand what potential outcomes might mean to those who are affected by these decisions, and taking responsibility for these decision outcomes. The fact that AI cannot be an ethical decision-maker is not due to its present-day technological limitations—i.e., it is not the case that some more data, or even some future technological breakthrough, will suddenly give AI systems the ability to make ethical decisions. Intentionality, care and responsibility are deeply human attributes, and as such, even the most sophisticated AI algorithms cannot truly “decide” on their own accord to do good or bad. Even when the outputs of certain AI systems result in good or bad outcomes, any assessment of the goodness or badness of these outcomes must ultimately come down to the intentions and preferences of the people and/or organizations that employ these systems.
If AI systems do not have moral agency and intentionality, how should we make sense of the fact that their outputs are often connected to important morally-significant outcomes? In our view, rather than thinking of AI as an ethical decision-maker in its own regard, it is more accurate to think of it as a mirror: one that reflects back to us the biases, discriminatory patterns and moral flaws that are deeply entrenched in our human cognition and social institutions. It is therefore inaccurate to assume that technologically sophisticated machines would be more ethical than us in the same way that, say, an ML-based chess engine could learn to find better chess moves than us. Rather, when AI systems are trained on large amounts of data about human behavior, they simply learn to capture more accurately the ways in which humans make decisions—biases and all. As such, large AI models can sometimes capture and reflect deep truths about how humans make moral decisions—even truths that might have otherwise remained opaque to us. Complaining about the biases and moral harms of AI, therefore, is like complaining about our image in the mirror!
Human biases and moral flaws can come to be embedded in AI systems throughout different stages of their conceptualization, development, deployment, and management.6 As our understanding of this fact—and more generally, of the biases of both humans and AI systems—has matured in recent times, there has been an explosion of technical research into carefully designing AI systems in ways that mitigate these biases and moral harms (cf. Silberg and Manyika, 2019; Guizzardi et al., 2020; Richardson and Gilbert, 2021). Despite this, however, the fact remains that AI systems cannot transcend the ethicality of their creators or the social context in which they were built. Technical solutions may allow us to reactively correct some of the biases that come to be embedded in AI models and datasets during their development, but as long as humans remain in charge of deploying and managing these AI systems, there remains a real possibility that the same biases could creep back in. Moreover, complaints about biased and unfair AI decisions have been steadily on the rise in recent times (cf. Silberg and Manyika, 2019; Ntoutsi et al., 2020)—suggesting that much work on these issues remains to be done.
What we are calling for, therefore, is a fundamental and much-needed shift in mindset. We should abandon our misplaced expectations that AI systems can be autonomous ethical decision-makers, and rather, we should remind ourselves of the importance and necessity of the moral compass of humans to guide us in making ethical decisions when intelligent machines are around. Crucially, if we would like to make decisions that are morally progressive compared to the ones we have made in the past, we cannot leave decision-making simply to AI. Recognizing the irreplaceable role of humans in ethical decision-making, in turn, points us to two urgent priorities. First, we need to invest more resources, time, and training in the art of “ethical upskilling”—to ensure that people use AI systems in responsible and socially-beneficial ways. Second, if AI is indeed a mirror that reflects our biases and moral flaws, we ought to take a long and careful look into this mirror: to uncover the discriminatory, unjust, and harmful patterns that are being reflected back to us, and in turn, to learn to become more and better ethical decision-makers.
If the responsibility for ethical decision-making in general—and specifically to use powerful AI systems to achieve socially-beneficial ends—must be retained in human hands, the frequent and systematic biases, moral flaws and cognitive errors of humans become a matter of great concern. Of course, humans have been in charge of organizational decision-making long before the advent of AI, but our track record has been less than stellar: our organizations have long been beset by ethical scandals, crises of corporate governance, and irresponsible leadership (Adler, 2002; Knights and O'Leary, 2005). Indeed, as behavioral ethicists have shown, organizational actors face systematic cognitive limitations when it comes to choosing the right thing to do—this “bounded ethicality” can create a gap between our actual and intended behavior (cf. Tenbrunsel and Smith-Crowe, 2008; De Cremer et al., 2011; Chugh and Kern, 2016). Importantly, these biases and moral errors are often implicit and unconscious: their influence on our behavior can remain hidden and difficult to predict, and can sometimes be in direct opposition to our espoused beliefs and values (cf. De Cremer and Moore, 2020). As such, even the most well-intentioned person can sometimes decide to do bad things.
If our argument thus far is sound, it stands to reason that our unethical tendencies will likely be made worse—not better—with the advent of AI. If we continue to assume that AI systems will make ethical decisions on our behalf, and as a result, fail to step up and take responsibility for ensuring that AI-augmented decisions serve socially-beneficial goals, there is no reason to think that AI systems will magically figure out how to make ethical decisions by themselves. As De Cremer and Kasparov (2021) argue, for example, when people rely on technologies to make decisions, processes of “moral disengagement” can lead to them feeling less responsible for (im)moral decision outcomes, as they can perceive machines—rather than themselves—as being in charge of driving decision-making. Moreover, over time, if AI systems were left entirely in charge of ethical decision-making, there is a real danger of moral deskilling in humans: i.e., the prospect of becoming unduly reliant on technological assistance to the point where we might be unable to act morally on our own (cf. Vallor, 2015; Schwarz, 2019).7 In such cases, unethical decision-making in organizations is likely to be more frequent and severe. Moreover, bounded ethicality is known to place stricter constraints on ethical behavior in such situations of uncertainty and equivocality (cf. Sonenshein, 2007), and as such, people are likely to behave more unethically when dealing with AI—a relatively nascent technology whose long-term consequences on society are uncertain and difficult to evaluate. For these reasons, we argue, humans currently lack the ethical maturity to ensure that AI will be used for good.
Cultivating this much-needed ethical maturity, therefore, must be a key priority when we seek to prepare our organizations and business leaders for the impending digital future. We need to become better skilled at understanding our own good and bad behavior, and apply those insights to interventions and training sessions on how to use intelligent technologies in more responsible ways. Such awareness training of the psychological underpinnings of (un)ethical behavior can teach us when humans are most likely to show unethical behavior, and in turn, translate these learnings to influence the design and employment of intelligent technologies toward ethical ends. This is what we are calling “ethical upskilling”.
The pedagogical and epistemological challenges of ethical upskilling cannot be overstated: how can we efficiently learn about, and teach organizations and business leaders to appreciate, the psychological underpinnings of their (un)ethical behavior? Crucially, given the fact that our biases and moral errors are typically a result of unconscious processes, merely pointing out our moral errors is often not enough: ethical training must raise awareness of how these errors come about, and provide pathways for students to manage their biases, adjust their behavior, and measure their progress (cf. Sparks and Pan, 2010; Emerson, 2017; Gino and Coffman, 2021). In our view, one way in which we can push ethical upskilling initiatives forward, and make them more relevant and insightful for decision-makers, is by harnessing the affordances of intelligent technologies. Even if AI systems cannot be ethical decision-makers in their own respect, they might at least play a role in teaching us how to be more ethical.
As we have argued above, AI systems capture, expose, and make prominent the biases and discriminatory patterns contained within the datasets they are trained on—and hence mirror our moral flaws back to us. This makes AI an excellent teaching tool to help humans identify our own ethical blindspots—as well as the ethical blindspots related to organizational culture, leadership, and our social institutions at large—and potentially pave the way for identifying strategies to overcome these blindspots. In other words, if decision-makers working with AI systems learn to take a critical look at the outputs of these systems, and think broadly and deeply about what these outputs mean in the broader organizational, social and political context, they would be in a good position to think carefully about what made similar previous decisions morally problematic, and how to avoid these in the future.8
For example, suppose an organization is using an AI system to aid in recruitment decisions, and has trained this system on historical data of its previous hiring decisions. This system, in turn, would become a reliable repository of the biases and harms perpetuated by the organization's previous hiring decisions—documenting, for example, previous instances of discrimination on gender, racial or religious grounds—and in turn, help decision-makers think more critically about how they might make more ethical decisions.9 Interacting with AI systems in this way entails treating their outputs not simply as recommendations of how to act in the future, but rather as ways of learning about how to avoid making mistakes from the past.10
In our view, three key aspects of contemporary AI make it especially useful as a tool for ethical upskilling:
(a) Scale: Because AI systems are typically trained on large and highly-dimensioned datasets, they have the potential to reflect discriminatory patterns and biases that would have otherwise remained invisible to human eyes (cf. Burrell, 2016; O'Neil, 2016). And, because AI systems are increasingly being deployed in far-reaching and consequential decision-making contexts, the risks of their decisions being biased and unethical are formidable, since this could potentially have an adverse effect on large groups of people. The massive scale of AI—both in its development and deployment—makes it so that they are increasingly not only reflecting our biases and flaws back to us, but amplifying them—making them easier to understand and harder to ignore. As such, the threat of powerful AI systems reproducing unethical human behavior at scale powerfully underscores the urgent need for humans in engage in ethical upskilling, and can in turn motivate us to take the risks of unethical AI seriously.
(b) Counterfactual modeling: ML models can be used to produce detailed and specific counterfactual scenarios—to see how small changes in inputs (say, a change in a given decision-subjects race, or a small increase in their income, etc.) can lead to different decision outcomes, and in turn, to different ethical consequences (cf. Chou et al., 2022). In our view, the learning opportunities offered by such counterfactual modeling techniques are profound. Counterfactuals could enable decision-makers to simulate the downstream effects of different possible decisions before committing to a particular choice, and in turn, gain a deep appreciation of the disparate impact of their decisions on different demographic groups (cf. Dai et al., 2022; Shang et al., 2022). Counterfactual explanations have also been used as an interpretability technique, to help users gain an intuitive appreciation for which factors are most important to an AI system when it makes its recommendations: which can similarly offer useful insights into the process of making ethical decisions (cf. Byrne, 2019; Keane and Smyth, 2020). Moreover, interacting with and thinking through counterfactual models may also inspire decision-makers to counterfactual thinking in their own decision-making process—which once again places them in good stead to carefully consider all relevant moral ramifications when making decisions (cf. Gollwitzer et al., 1990).
(c) Interpretability: If one were to ask a human who made a morally-significant decision how they came to this decision, their answers would likely be unsatisfactory. Human cognitive processes are, for the most part, opaque black-boxes—it is usually impossible to identify the full range of factors (both conscious and unconscious; internal and external) that lead us to decide and act in certain ways. As such, there is only so much that one could learn when analyzing another human's moral errors. When it comes to AI, however, there has been a recent explosion in research to make AI systems “interpretable”: to obtain high-fidelity, precise and easy-to-understand knowledge about the inner decision-making logic that these systems use to come to their decisions (cf. Mueller et al., 2019). Although there remain some critical shortcomings with making an AI system interpretable, decision-makers can sometimes have access to information about the key variables and logic that undergirds the system's decision-making process.
An AI model trained on data about a company's previous hiring decisions might, when made interpretable, reveal that these hiring decisions were unreasonably biased against certain racial or gender minorities. Or, an AI system used for performance management might, when made interpretable, reveal that certain groups of precarious low-paid workers are unreasonably surveilled more and pushed to work longer and harder than their better-paid white-collar colleagues. Or, a credit-scoring AI system that was explicitly designed to not consider racial categories when issuing credit scores might, when made interpretable, reveal that some other seemingly innocuous variable (e.g., postal codes) was being used as a proxy to racially profile credit-score recipients.11 In such ways, through a careful analysis of the factors that lead AI systems to make biased and unethical decisions, and critical reflection on why these factors might be systematically connected to unethicality, decision-makers can come to a more nuanced understanding of what is needed to avoid making unethical decisions.
However, for the moment, the usefulness of interpretability for ethical upskilling remains limited. First, as AI systems are becoming larger and more complex, it is becoming increasingly difficult to ensure that they remain meaningfully interpretable to humans (cf. Arrieta et al., 2020; Long, 2020). Second, even given rapid advances in interpretability techniques, there remains much work to be done in making sure that these techniques faithfully reveal the inner logics of AI systems, rather than simply providing misleading and over-simplified explanations (Ribeiro et al., 2016; DiMarco, 2021). Finally, the interpretation of an AI system's explanations is fundamentally subjective. It is unclear whether people will be able to draw meaningful and unambiguous ethical lessons by scrutinizing the content of an AI system's explanations (Ananny and Crawford, 2018; Rudin, 2019).
These limitations notwithstanding, in our view, it is still useful to keep the affordances of interpretable AI in mind as we think about how AI can serve as a tool in ethical upskilling. Interpretability techniques have seen rapid advances in their sophistication and effectiveness—and there are already a few cases where interpretability techniques can reveal morally useful knowledge about decision-making processes that might have otherwise remained opaque. While much work remains to be done to ensure that interpretable AI meaningfully serves ethical upskilling, it is important to note that interpretability should not be viewed as some sort of magic repository for moral knowledge, but rather as providing a starting point for critical conversations on how to consistently make ethical decisions.
As such, when we use AI as a tool for ethical upskilling, we appropriately acknowledge the powers and capabilities of these powerful technologies in helping us make more ethical decisions, while avoiding the mistake of thinking that AI systems can make such decisions by themselves. We should not throw the proverbial baby out with the bathwater: even if AI systems cannot make ethical decisions by themselves, they can still play a crucial role in helping us become more consistent ethical decision-makers.
We have argued that it is deeply unreasonable to expect that AI systems—even given more data or better computational resources—will be more ethical than the humans who develop, deploy and use them. This entails that the responsibility for ethical decision-making must remain in human hands. However, as we have discussed, it does not seem that human decision-makers currently have the ethical maturity to meaningfully take on this responsibility. There is an urgent need, therefore, to broaden and strengthen the ways in which we ethically upskill our organizations and leaders, and AI has a crucial role to play in such efforts. Specifically, because AI is a mirror that reflects our biases and moral flaws back to us, decision-makers should look carefully into this mirror—taking advantage of the opportunities brought about by scale, interpretability, and counterfactual modeling—to gain a deep understanding of the psychological underpinnings of our (un)ethical behaviors, and in turn, learn to consistently make ethical decisions. This is, in our view, a key strategy for improving current attempts to ethically upskill our organizations and leaders for the impending digital future.
Our suggested approach represents a new kind of collaborative paradigm between humans and machines when it comes to ethical decisions: where AI plays a supportive role in helping human decision-makers make more ethical and responsible decisions (cf. Table 1). In contemporary social and policy circles, there has been much discussion about the need for a “human-in-the-loop” for algorithmic decision-making: where the role of the human in decision-making is, at best, confined to scrutinizing, and in turn approving or rejecting, their AI system's recommended decision (see e.g., Zanzotto, 2019). In our view, however, a more accurate and responsible approach would be to have an “AI-in-the-loop” for human decision-making: where the role of AI is strictly confined to providing information about biases and moral flaws in potential outcomes, to help humans make better and more informed decisions.
Table 1. Key conceptual differences between the currently-dominant technosolutionist paradigm, and our proposed collaborative alternative.
Adopting this AI-in-the-loop approach has key implications for managers and organizational decision-makers. Crucially, managers must unlearn an increasingly common tendency to pass human responsibility and accountability over to algorithms: indeed, the phrase “the algorithm did it”, should be entirely purged from our vocabulary. Moreover, managers should be trained to be more aware of, and to be able to deal with, complex ethical business dilemmas—especially those pertaining to the use of intelligent technologies in organizations. Finally, managers need to be trained to recognize the human biases and flaws that underlie the decisions outputted by AI systems—and in turn, learn to recognize blindspots in their own thinking, as well as their organizational processes. As we have argued, we can learn to tap into the affordances of intelligent technologies to make these processes of ethical upskilling more instructive and valuable to decision-makers.
However, much more work remains to be done to implement this collaborative paradigm in organizational practice. While we hope to have provided a big-picture overview of what this paradigm might entail, we recognize that organizations may need further guidance on how exactly to incorporate AI into ethical upskilling initiatives. Even when we accept that AI is simply a mirror of human biases and moral flaws, we still need to learn how to carefully interpret and derive meaningful lessons from the image in this mirror! As such, implementing this collaborative paradigm is truly an interdisciplinary endeavor: we need technical research targeted at making AI systems better suited for ethical upskilling (especially in the domains of interpretability and counterfactual modeling), pedagogical research on how to educate business leaders to think critically and carefully about the moral implications of AI in decision-making, and organizational research on how exactly to include AI in organizational decision-making workflows (cf. Mittelstadt et al., 2016; Shrestha et al., 2019; De Cremer and Narayanan, 2023). We hope that the account sketched in this paper might provide guidance and impetus for future scholars to further help organizations pursue AI-augmented ethical upskilling initiatives.
In conclusion, as AI systems become increasingly ubiquitous in our organizations and societies, human decision-makers have an immutable and crucial role to play in ensuring that AI-augmented decisions are socially-beneficial and just. As we have argued, it is ultimately the moral compass of humans that we are relying on to guide the employment of these powerful technologies toward the social good. Without such a carefully-calibrated moral compass, both machines and humans would be at a loss.
DDC came up with the idea. DDC and DN wrote the paper together. Both authors contributed to the article and approved the submitted version.
This paper was supported by research funding from the Centre on AI Technology for Humankind, NUS Business School, National University of Singapore.
The authors declare that the research was conducted in the absence of any commercial or financial relationships that could be construed as a potential conflict of interest.
All claims expressed in this article are solely those of the authors and do not necessarily represent those of their affiliated organizations, or those of the publisher, the editors and the reviewers. Any product that may be evaluated in this article, or claim that may be made by its manufacturer, is not guaranteed or endorsed by the publisher.
1. ^In this paper, we define AI broadly as computational systems that learn from large datasets to engage in pattern-recognition and problem-solving, which in turn promote the efficiency of organizations (Von Krogh, 2018): by making them more organized (Lwowski et al., 2017) and better able to achieve their objectives (Glikson and Woolley, 2020).
2. ^Importantly, these questions and concerns long predate the advent of AI: even when organizational decision-making has been exclusively led by human decision-makers (as has been the case thus far), concerns about unfair biases when treating certain groups of employees over others, about systematic errors of judgment that lead to bad strategic decisions that harm the organization, and about unduly prioritising profit even to the detriment of society at large, to name a few, have been well-explored in business scholarship thus far (cf. Hammond et al., 1998; Campbell et al., 2009; Denning, 2019). These issues have perhaps taken a new form and urgency given the recent rise of AI, but their underlying concerns remain largely the same.
3. ^Although moral philosophers sometimes distinguish between “ethics” and “morals” – typically using the former to discuss the appropriateness of actions, and the latter to discuss overarching principles and rules – in this essay we treat the two terms as largely interchangeable, unless explicitly specified otherwise.
4. ^Here, it is important to note that we are not saying that embedded ethics approaches are unhelpful or otherwise unwarranted. Designing AI systems to better align with principles of fairness, accountability, trustworthiness, and other such normative principles has an important role to play in ensuring that AI-augmented decisions are ethical. Our argument is simply that these approaches will prove insufficient if the goal is to delegate organizational decision-making to AI. Put differently, AI systems can produce more or less ethical outcomes depending on how well they are designed, but they cannot be ethical decision-makers in their own regard.
That said, we acknowledge that the status of AI as a moral agent in general has been up for debate. Various scholars have argued for AI systems to be more actively incorporated in ethical decision-making (cf. Moor, 2006; Behdadi and Munthe, 2020). However, as a recent review on this topic by Cervantes et al. (2020) notes, “there is a long way to go (from a technological perspective) before this type of artificial agent can replace human judgment in difficult, surprising or ambiguous moral situations” (p. 501). Although it falls outside the scope of this paper to substantively engage in proposals for artificial moral agency – especially outside the context of organizational decision-making – we would encourage interested readers to explore this topic further.
5. ^The term “machine learning” is somewhat of a broad category - including both simpler techniques like regression and clustering, but also more powerful and complex neural networks. The subcategory of “deep learning” (including neural networks, generative models, etc.) are generally more architecturally complex – and a more technically-oriented discussion would do well to distinguish clearly between these various types of ML/DL models. For the purposes of this paper, however, we use the term “ML” as a catch-all, since our discussion does not hinge on any specific differences between types of ML/DL models.
6. ^This is not to suggest that AI systems are only biased when humans intentionally develop, deploy, and/or manage these systems in biased and morally harmful ways. Our claim simply is that extant biases and moral flaws in AI are necessarily reflective of the biases and flaws of the humans and social forces that shape them. In other words, if an AI system is found to be biased, these biases may reliably be traced to biases and moral flaws in our human cognition and social institutions. The intentions (moral or otherwise) of those who develop, deploy, and manage these systems are, for the most part, irrelevant to our argument.
7. ^Vallor (2015) and Schwarz (2019) discussions of moral deskilling are theoretically-grounded warnings of a somewhat speculative nature. However, more generally, the risks of deskilling in light of the advent of AI have been well documented and theorized for decades. The use of autonomous self-driving vehicles can adversely impact the competencies of human drivers (Bertrandias et al., 2021); the use of clinical decision-support systems can adversely impact the skills and experience of healthcare decision-makers (Mebrahtu et al., 2021); and the use of AI-generated artwork can adversely impact human creativity and artistic practices (Roose, 2022); to name a few. As such, insofar as we care about retaining our human ability to act morally even when faced with new situations, it is important to understand and counter the dangers of moral deskilling due to AI. Our thanks to an anonymous reviewer for making this important point.
8. ^Interestingly, Kliegr et al. (2021) make a similar connection but in an opposite direction – arguing that our existing knowledge about the nature of human cognitive biases can prove instrumental in devising novel ways for debiasing AI systems. In other words, knowledge about human biases might be similarly important for” “ethically upskilling” AI systems. Our thanks to an anonymous reviewer for pointing out this connection.
9. ^This is not merely a thought experiment: there have been several high-profile cases of AI-based hiring algorithms reproducing gender and racial biases in organizations, and as a result, there have been growing policy and scholarly efforts to urgently address these hiring biases (cf. Dastin, 2018; Raghavan et al., 2020).
10. ^One interesting connection in this regard is to Clark and Chalmers' (1998) idea of an “extended mind'. Their proposal of active externalism – one where “the human organism is linked with an external entity [here, AI] in a two-way interaction, creating a coupled system that can be seen as a cognitive system in its own right” (p. 8) – can be seen as describing a human-AI collaborative paradigm that might plausibly be supported by the same arguments that we have made here (i.e., even if AI systems cannot be ethical decision-makers in their own regard, they can still play a role in improving human decision-making). There are, of course, important differences in terms of the phenomenology of human-AI interaction between their proposal (AI as part of the “extended mind' of a human decision-maker) and ours (AI as an information tool that reveals morally useful knowledge for human decision-makers to incorporate). That said, we acknowledge that one may plausibly extend the arguments in our paper to arrive at Clark and Chalmers' position, and we invite interested readers to pursue these connections further. Our thanks to an anonymous reviewer for bringing this to our attention.
11. ^Although for the sake of narrative simplicity and readability, we have chosen to illustrate our point with examples of a somewhat hypothetical nature, it should be noted that there are a growing number of real-world examples of people learning morally useful things by scrutinizing AI systems. Amazon, notably, learned more about its historical record of hiring bias when an AI system trained on its previous employment records was unduly biased against women candidates (Dastin, 2018). Prince and Schwarcz (2020) offer a comprehensive review of the ways in which proxies mask discrimination in AI decision-making, and how these might be uncovered through more closely scrutinizing AI systems. In general, the interpretability literature is replete with examples of humans learning to improve their decision-making by examining the inner decision logic of AI systems – as well as the many shortcomings of interpretability that are yet to be fully addressed. Interested readers may refer to the comprehensive literature reviews of Doshi-Velez and Kim (2017) and Mueller et al. (2019), and/or to the theoretical expositions on this topic offered by Burrell (2016), Weller (2017), and (Narayanan, 2023) to name a few. Interested readers may also refer to Floridi et al. (2018) for a broader overview of the role of interpretability in ethical AI, and the applicability of ethical AI across a variety of domains including healthcare, environmental sustainability, and more.
Adler, P. S. (2002). Corporate scandals: It's time for reflection in business schools. Acad. Manage. Perspect 16, 148–149. doi: 10.5465/ame.2002.8540425
Agrawal, A., Gans, J., and Goldfarb, A. (2018). Prediction Machines: The Simple Economics of Artificial Intelligence. Cambridge, MA: Harvard Business Press.
Ananny, M., and Crawford, K. (2018). Seeing without knowing: Limitations of the transparency ideal and its application to algorithmic accountability. New Media Soc. 20, 979–989. doi: 10.1177/1461444816676645
Anderson, J., Rainie, L., and Luchsinger, A. (2018). Artificial intelligence and the future of humans. Available online at: www.pewresearch.org (accessed May 05, 2023).
Anscombe, G. E. M. (1958). Modern moral philosophy. Philosophy 33, 1–19. doi: 10.1017/S0031819100037943
Arrieta, A. B., Díaz-Rodríguez, N., Del Ser, J., Bennetot, A., Tabik, S., Barbado, A., et al. (2020). Explainable artificial intelligence (XAI): Concepts, taxonomies, opportunities and challenges toward responsible AI. Inf. Fusion 58, 82–115. doi: 10.1016/j.inffus.2019.12.012
Bareis, J., and Katzenbach, C. (2021). Talking AI into being: the narratives and imaginaries of national ai strategies and their performative politics. Sci. Technol. Human Values 47, 7. doi: 10.1177/01622439211030007
Behdadi, D., and Munthe, C. (2020). A normative approach to artificial moral agency. Minds Mach. 30, 195–218. doi: 10.1007/s11023-020-09525-8
Bender, E. M., Gebru, T., McMillan-Major, A., and Shmitchell, S. (2021). “On the dangers of stochastic parrots: Can language models be too big?” in Proceedings of the 2021 ACM Conference on Fairness, Accountability, and Transparency 610–623. doi: 10.1145/3442188.3445922
Bertrandias, L., Lowe, B., Sadik-rozsnyai, O., and Carricano, M. (2021). Delegating decision-making to autonomous products: A value model emphasizing the role of well-being. Technol. Forecast Soc. Change 169, 120846. doi: 10.1016/j.techfore.2021.120846
Bietti, E. (2020). “From ethics washing to ethics bashing: A view on tech ethics from within moral philosophy,” in Proceedings of the 2020 Conference on Fairness, Accountability, and Transparency 210–219. doi: 10.1145/3351095.3372860
Brynjolfsson, E., and McAfee, A. (2012). Winning the race with ever-smarter machines. MIT Sloan Manag. Rev. 53, 53.
Brynjolfsson, E., and McAfee, A. (2014). The second machine age: Work, progress, and prosperity in a time of brilliant technologies. WW Norton and Company.
Brynjolfsson, E., Rock, D., and Syverson, C. (2018). “Artificial intelligence and the modern productivity paradox: A clash of expectations and statistics,” inThe Economics of Artificial Intelligence: An agenda 23–57. University of Chicago Press. doi: 10.7208/chicago/9780226613475.003.0001
Burrell, J. (2016). How the machine “thinks': Understanding opacity in machine learning algorithms. Big Data and Society 3, 2053951715622512. doi: 10.1177/2053951715622512
Byrne, R. M. (2019). “Counterfactuals in Explainable Artificial Intelligence (XAI): Evidence from Human Reasoning,” in Proceedings of the Twenty-Eighth International Joint Conference on Artificial Intelligence Survey track 6276–6282. doi: 10.24963/ijcai.2019/876
Campbell, A., Whitehead, J., and Finkelstein, S. (2009). Why Good Leaders Make Bad Decisions. Harvard Business Review. Available online at: https://hbr.org/2009/02/why-good-leaders-make-bad-decisions (accessed May 05, 2023).
Cervantes, J. A., López, S., Rodríguez, L. F., Cervantes, S., Cervantes, F., and Ramos, F. (2020). Artificial moral agents: A survey of the current status. Sci. Eng. Ethics 26, 501–532. doi: 10.1007/s11948-019-00151-x
Chou, Y.-L., Moreira, C., Bruza, P., Ouyang, C., and Jorge, J. (2022). Counterfactuals and causability in explainable artificial intelligence: Theory, algorithms, and applications. Inf. Fusion 81, 59–83. doi: 10.1016/j.inffus.2021.11.003
Chugh, D., and Kern, M. C. (2016). A dynamic and cyclical model of bounded ethicality. Res. Organiz. Behav. 36, 85–100. doi: 10.1016/j.riob.2016.07.002
Clark, A., and Chalmers, D. (1998). The extended mind. Analysis 58, 7–19. doi: 10.1093/analys/58.1.7
Cockburn, I. M., Henderson, R., and Stern, S. (2018). “The impact of artificial intelligence on innovation: An exploratory analysis,” in The economics of artificial intelligence: An agenda (University of Chicago Press) 115–146. doi: 10.7208/chicago/9780226613475.003.0004
Conitzer, V., Sinnott-Armstrong, W., Borg, J. S., Deng, Y., and Kramer, M. (2017). Moral decision-making frameworks for artificial intelligence,” inProceedings of the Thirty-First AAAI Conference on Artificial Intelligence (AAAI Press) 4831–4835. doi: 10.1609/aaai.v31i1.11140
Crawford, K. (2021). The atlas of AI: Power, politics, and the planetary costs of artificial intelligence. Yale University Press. doi: 10.12987/9780300252392
Dai, X., Keane, M. T., Shalloo, L., Ruelle, E., and Byrne, R. M. J. (2022). “Counterfactual Explanations for Prediction and Diagnosis in XAI,” in Proceedings of the 2022 AAAI/ACM Conference on AI, Ethics, and Society 215–226. doi: 10.1145/3514094.3534144
Dastin, J. (2018). Amazon Scraps Secret AI Recruiting Tool That Showed Bias Against Women. Reuters. Retrieved from: https://www.reuters.com/article/us-amazon-com-jobs-automation-insight-idUSKCN1MK08G (accessed May 05, 2023).
De Cremer, D. (2020). Leadership by algorithm: Who leads and who follows in the AI era? Harriman House Limited.
De Cremer, D. (2022). Machines are not moral role models. Nat. Human Behav. 6, 609. doi: 10.1038/s41562-022-01290-1
De Cremer, D., and Kasparov, G. (2021). AI should augment human intelligence, not replace it. Harvard Business Review.
De Cremer, D., and Kasparov, G. (2022). The ethics of technology innovation: A double-edged sword? AI Ethics 2, 533–537. doi: 10.1007/s43681-021-00103-x
De Cremer, D., and Moore, C. (2020). Toward a better understanding of behavioral ethics in the workplace. Ann. Rev. Organiz. Psychol. Organiz. Behav. 7, 369–393. doi: 10.1146/annurev-orgpsych-012218-015151
De Cremer, D., and Narayanan, D. (2023). On educating ethics in the AI era: why business schools need to move beyond digital upskilling, towards ethical upskilling. AI Ethics. doi: 10.1007/s43681-023-00306-4
De Cremer, D., Van Dick, R., Tenbrunsel, A., Pillutla, M., and Murnighan, J. K. (2011). Understanding ethical behavior and decision making in management: A behavioral business ethics approach. Br. J. Manage. 22, S1–S4. doi: 10.1111/j.1467-8551.2010.00733.x
Denning, S. (2019). Why Maximizing Shareholder Value Is Finally Dying. Forbes. Available online at: https://www.forbes.com/sites/stevedenning/2019/08/19/why-maximizing-shareholder-value-is-finally-dying/ (accessed May 05, 2023).
DiMarco, M. (2021). Wishful intelligibility, black boxes, and epidemiological explanation. Philos. Sci. 88, 824–834. doi: 10.1086/715222
Dordevic, M. (2022). Council Post: How Artificial Intelligence Can Improve Organizational Decision Making. Forbes. Available online at: https://www.forbes.com/sites/forbestechcouncil/2022/08/23/how-artificial-intelligence-can-improve-organizational-decision-making/ (accessed May 05, 2023).
Doshi-Velez, F., and Kim, B. (2017). Towards a rigorous science of interpretable machine learning. ArXiv Preprint ArXiv:1702.08608.
Emerson, J. (2017). Don't give up on unconscious bias training—Make it better. Harvard Bus. Rev. 28, 4.
European Commission (2019). Policy and Investment Recommendations for Trustworthy AI. High Level Expert Group on Artificial Intelligence, European Commission.
Felten, E., Raj, M., and Seamans, R. (2023). How will Language Modelers like ChatGPT Affect Occupations and Industries? arXiv Preprint arXiv:2303.01157. doi: 10.2139/ssrn.4375268
Feuerriegel, S., Shrestha, Y. R., von Krogh, G., and Zhang, C. (2022). Bringing artificial intelligence to business management. Nat. Mach. Intell. 4, 611–613. doi: 10.1038/s42256-022-00512-5
Fjeld, J., Achten, N., Hilligoss, H., Nagy, A., and Srikumar, M. (2020). Principled artificial intelligence: Mapping consensus in ethical and rights-based approaches to principles for AI. Berkman Klein Center Research Publication 2020–1. doi: 10.2139/ssrn.3518482
Floridi, L., Cowls, J., Beltrametti, M., Chatila, R., Chazerand, P., Dignum, V., et al. (2018). AI4People—An Ethical Framework for a Good AI Society: Opportunities, Risks, Principles, and Recommendations. Minds Mach. 28, 689–707. doi: 10.1007/s11023-018-9482-5
Gee, K. (2017). In unilever's radical hiring experiment, resumes are out, algorithms are in. Wall Street J. 26, 07. Available online at: https://www.wsj.com/articles/in-unilevers-radical-hiring-experiment-resumes-are-out-algorithms-are-in-1498478400 (accessed May 05, 2023).
Gino, F., and Coffman, K. (2021). Unconscious bias training that works. Harvard Bus. Rev. 99, 114–123. Available online at: https://hbr.org/2021/09/unconscious-bias-training-that-works (accessed May 05, 2023).
Glikson, E., and Woolley, A. W. (2020). Human trust in artificial intelligence: Review of empirical research. Acad. Manag. Ann. 14, 627–660. doi: 10.5465/annals.2018.0057
Gollwitzer, P. M., Heckhausen, H., and Steller, B. (1990). Deliberative and implemental mind-sets: Cognitive tuning toward congruous thoughts and information. J. Person. Soc. Psychol. 59, 1119. doi: 10.1037/0022-3514.59.6.1119
Google. (2022). Responsible AI practices. Google AI. Available online at: https://ai.google/responsibilities/responsible-ai-practices/ (accessed May 05, 2023).
Gray, M. L., and Suri, S. (2019). Ghost work: How to stop Silicon Valley from building a new global underclass. Eamon Dolan Books.
Gregory, R. W., Henfridsson, O., Kaganer, E., and Kyriakou, H. (2021). The role of artificial intelligence and data network effects for creating user value. Acad. Manag. Rev. 46, 534–551. doi: 10.5465/amr.2019.0178
Guizzardi, R., Amaral, G., Guizzardi, G., and Mylopoulos, J. (2020). “Ethical requirements for AI systems,” inCanadian Conference on Artificial Intelligence (Cham: Springer) 251–256. doi: 10.1007/978-3-030-47358-7_24
Hagendorff, T. (2020). The ethics of AI ethics: An evaluation of guidelines. Minds Mach. 30, 99–120. doi: 10.1007/s11023-020-09517-8
Hammond, J., Keeney, R., and Raiffa, H. (1998). The Hidden Traps in Decision Making. Harvard Business Review. Available online at: https://hbr.org/1998/09/the-hidden-traps-in-decision-making-2 (accessed May 05, 2023).
Hanson, D., and Bar-Cohen, Y. (2009). The Coming Robot Revolution: Expectations and Fears About Emerging Intelligent, Humanlike Machines. New York: Springer. doi: 10.1007/978-0-387-85349-9
Hao K. (2020),” in2020, let's stop AI ethics-washing and actually do something. MIT Technology Review. Available online at: https://www.technologyreview.com/2019/12/27/57/ai-ethics-washing-time-to-act/.
Hoffman, M., Kahn, L. B., and Li, D. (2018). Discretion in hiring. Quarterly J. Econ. 133, 765–800. doi: 10.1093/qje/qjx042
Hovy, D., and Spruit, S. L. (2016). The social impact of natural language processing,” in Proceedings of the 54th Annual Meeting of the Association for Computational Linguistics 591–598. doi: 10.18653/v1/P16-2096
Huang, M.-H., Rust, R., and Maksimovic, V. (2019). The feeling economy: Managing in the next generation of artificial intelligence (AI). California Manage. Rev. 61, 43–65. doi: 10.1177/0008125619863436
Jobin, A., Ienca, M., and Vayena, E. (2019). The global landscape of AI ethics guidelines. Nat. Mach. Intell. 1, 389–399. doi: 10.1038/s42256-019-0088-2
Joque, J. (2022). Revolutionary Mathematics: Artificial Intelligence, Statistics and the Logic of Capitalism. Verso Books.
Keane, M. T., and Smyth, B. (2020). Good Counterfactuals and Where to Find Them: A Case-Based Technique for Generating Counterfactuals for Explainable AI (XAI),” in Case-Based Reasoning Research and Development, I., Watson, and R., Weber (Cham: Springer International Publishing) 163–178. doi: 10.1007/978-3-030-58342-2_11
Kellogg, K. C., Valentine, M. A., and Christin, A. (2020). Algorithms at work: The new contested terrain of control. Acad. Manage. Ann. 14, 366–410. doi: 10.5465/annals.2018.0174
Kliegr, T., Bahník, Š., and Fürnkranz, J. (2021). A review of possible effects of cognitive biases on interpretation of rule-based machine learning models. Artif. Intell. 295, 103458. doi: 10.1016/j.artint.2021.103458
Knights, D., and O'Leary, M. (2005). Reflecting on corporate scandals: The failure of ethical leadership. Business Ethics 14, 359–366. doi: 10.1111/j.1467-8608.2005.00417.x
Korteling, J. E., Brouwer, A. M., and Toet, A. (2018). A neural network framework for cognitive bias. Front. Psychol. 9, 1561. doi: 10.3389/fpsyg.2018.01561
Laugier, S., and Chalier, J. (2013). The will to see: Ethics and moral perception of sense. Graduate Faculty Philos. J. 34, 263–281. doi: 10.5840/gfpj201334219
Lee, M. K., Kim, J. T., and Lizarondo, L. (2017). “A human-centered approach to algorithmic services: Considerations for fair and motivating smart community service management that allocates donations to non-profit organizations,” in Proceedings of the 2017 CHI Conference on Human Factors in Computing Systems 3365–3376. doi: 10.1145/3025453.3025884
Long, B. (2020). The Ethics of Deep Learning AI and the Epistemic Opacity Dilemma. Blog of the APA. Available online at: https://blog.apaonline.org/2020/08/13/the-ethics-of-deep-learning-ai-and-the-epistemic-opacity-dilemma/ (accessed May 05, 2023).
Lwowski, J., Benavidez, P., Prevost, J. J., and Jamshidi, M. (2017). Task allocation using parallelized clustering and auctioning algorithms for heterogeneous robotic swarms operating on a cloud network,” in Autonomy and Artificial Intelligence: A Threat or Savior? (New York: Springer) 47–69. doi: 10.1007/978-3-319-59719-5_3
McLennan, S., Fiske, A., Celi, L. A., Müller, R., Harder, J., Ritt, K., et al. (2020). An embedded ethics approach for AI development. Nat. Mach. Intell. 2, 488–490. doi: 10.1038/s42256-020-0214-1
Mebrahtu, T. F., Bloor, K., Ledward, A., Keenan, A.-M., Andre, D., Randell, R., et al. (2021). Effects of computerised clinical decision support systems (CDSS) on nursing and allied health professional performance and patient outcomes. Cochr Datab System Rev. 2021, CD014699. doi: 10.1002/14651858.CD014699
Metcalf, L., Askay, D. A., and Rosenberg, L. B. (2019). Keeping humans in the loop: Pooling knowledge through artificial swarm intelligence to improve business decision making. California Manag. Rev. 61, 84–109. doi: 10.1177/0008125619862256
Microsoft. (2022). Responsible AI principles from Microsoft.Microsoft AI. Available online at: https://www.microsoft.com/en-us/ai/responsible-ai?activetab=pivot1%3aprimaryr6 (accessed May 05, 2023).
Mitchell, M. (2021). Abstraction and analogy-making in artificial intelligence. Ann. New York Acad. Sci. 1505, 79–101. doi: 10.1111/nyas.14619
Mittelstadt, B. (2019). Principles alone cannot guarantee ethical AI. Nat. Mach. Intell. 1, 501–507. doi: 10.1038/s42256-019-0114-4
Mittelstadt, B. D., Allo, P., Taddeo, M., Wachter, S., and Floridi, L. (2016). The ethics of algorithms: Mapping the debate. Big Data Soc. 3, 2053951716679679. doi: 10.1177/2053951716679679
Moor, J. H. (2006). The nature, importance, and difficulty of machine ethics. IEEE Intell. Syst. 21, 18–21. doi: 10.1109/MIS.2006.80
Morozov, E. (2013). To save everything, click here: The folly of technological solutionism. Public Affairs.
Mueller, S. T., Hoffman, R. R., Clancey, W., Emrey, A., and Klein, G. (2019). Explanation in Human-AI Systems: A Literature Meta-Review, Synopsis of Key Ideas and Publications, and Bibliography for Explainable AI. Available online at: http://arxiv.org/abs/1902.01876v1
Narayanan, D. (2023). “Welfarist Moral Grounding for Transparent AI,” in 2023 ACM Conference on Fairness, Accountability, and Transparency. doi: 10.1145/3593013.3593977
Nath, R., and Sahu, V. (2020). The problem of machine ethics in artificial intelligence. AI Soc. 35, 103–111. doi: 10.1007/s00146-017-0768-6
Ntoutsi, E., Fafalios, P., Gadiraju, U., Iosifidis, V., Nejdl, W., Vidal, M., et al. (2020). Bias in data-driven artificial intelligence systems—An introductory survey. Wiley Interdisc. Rev. 10, e1356. doi: 10.1002/widm.1356
O'Neil, C. (2016). Weapons of math destruction: How big data increases inequality and threatens democracy. Crown.
Parent-Rocheleau, X., and Parker, S. K. (2022). Algorithms as work designers: How algorithmic management influences the design of jobs. Human Resour. Manag. Rev. 32, 100838. doi: 10.1016/j.hrmr.2021.100838
PDPC (2020). Model AI Governance Framework: Second Edition. Infocomm Media Development Authority. Available online at: https://www.pdpc.gov.sg/-/media/files/pdpc/pdf-files/resource-for-organisation/ai/sgmodelaigovframework2.ashx (accessed May 05, 2023).
Prince, A., and Schwarcz, D. (2020). Proxy Discrimination in the Age of Artificial Intelligence and Big Data. Iowa Law Review, 1257. Available online at: https://ilr.law.uiowa.edu/print/volume-105-issue-3/proxy-discrimination-in-the-age-of-artificial-intelligence-and-big-data/ (accessed May 05, 2023).
Raghavan, M., Barocas, S., Kleinberg, J., and Levy, K. (2020). “Mitigating bias in algorithmic hiring: Evaluating claims and practices,” in Proceedings of the 2020 Conference on Fairness, Accountability, and Transparency 469–481. doi: 10.1145/3351095.3372828
Rességuier, A., and Rodrigues, R. (2020). AI ethics should not remain toothless! A call to bring back the teeth of ethics. Big Data Soc. 7, 2053951720942541. doi: 10.1177/2053951720942541
Ribeiro, M. T., Singh, S., and Guestrin, C. (2016). Why should I trust you? Explaining the predictions of any classifier,” in Proceedings of the 22nd ACM SIGKDD International Conference on Knowledge Discovery and Data Mining 1135–1144. doi: 10.1145/2939672.2939778
Ricci, M., Cadene, R., and Serre, T. (2021). Same-different conceptualization: A machine vision perspective. Curr. Opin. Behav. Sci. 37, 47–55. doi: 10.1016/j.cobeha.2020.08.008
Richardson, B., and Gilbert, J. E. (2021). A Framework for Fairness: A Systematic Review of Existing Fair AI Solutions. ArXiv Preprint ArXiv:2112.05700.
Rizzo, J. R., House, R. J., and Lirtzman, S. I. (1970). Role conflict and ambiguity in complex organizations. Admin. Sci. Quart. 150–163. doi: 10.2307/2391486
Roose, K. (2022). A.I.-Generated Art Is Already Transforming Creative Work. The New York Times. Available online at: https://www.nytimes.com/2022/10/21/technology/ai-generated-art-jobs-dall-e-2.html (accessed May 05, 2023).
Rudin, C. (2019). Stop explaining black-box machine learning models for high-stakes decisions and use interpretable models instead. Nat. Mach. Intell. 1, 206–215. doi: 10.1038/s42256-019-0048-x
Russell, S. J., and Norvig, P. (2016). Artificial Intelligence: A Modern Approach. London: Pearson Education Limited.
Schwarz, E. (2019). Günther Anders in Silicon Valley: Artificial intelligence and moral atrophy. Thesis Eleven 153, 94–112. doi: 10.1177/0725513619863854
Shang, R., Feng, K. J. K., and Shah, C. (2022). “Why Am I Not Seeing It? Understanding Users' Needs for Counterfactual Explanations in Everyday Recommendations,” in 2022 ACM Conference on Fairness, Accountability, and Transparency 1330–1340. doi: 10.1145/3531146.3533189
Shrestha, Y. R., Ben-Menahem, S. M., and Von Krogh, G. (2019). Organizational decision-making structures in the age of artificial intelligence. California Manag. Rev. 61, 66–83. doi: 10.1177/0008125619862257
Silberg, J., and Manyika, J. (2019). Notes from the AI frontier: Tackling bias in AI (and in humans). McKinsey Global Institute 1–6.
Simonite, T. (2020). Google offers to help others with the tricky ethics of AI. Wired. Available online at: https://www.wired.com/story/google-help-others-tricky-ethics-ai/ (accessed May 05, 2023).
Sonenshein, S. (2007). The role of construction, intuition, and justification in responding to ethical issues at work: The sensemaking-intuition model. Acad. Manag. Rev. 32, 1022–1040. doi: 10.5465/amr.2007.26585677
Sparks, J. R., and Pan, Y. (2010). Ethical judgments in business ethics research: Definition, and research agenda. J. Bus. Ethics 91, 405–418. doi: 10.1007/s10551-009-0092-2
Tenbrunsel, A. E., and Smith-Crowe, K. (2008). Ethical decision making: where we've been and where we're going. Acad. Manag. Ann. 2, 545–607. doi: 10.5465/19416520802211677
Toews, R. (2021). What artificial intelligence still can't do. Available online at: https://www.forbes.com/sites/robtoews/2021/06/01/what-artificial-intelligence-still-cant-do/?sh=7d7c32b466f6 (accessed May 05, 2023).
Trunk, A., Birkel, H., and Hartmann, E. (2020). On the current state of combining human and artificial intelligence for strategic organizational decision making. Business Res. 13, 875–919. doi: 10.1007/s40685-020-00133-x
Vallor, S. (2015). Moral deskilling and upskilling in a new machine age: Reflections on the ambiguous future of character. Philos. Technol. 28, 107–124. doi: 10.1007/s13347-014-0156-9
Varian, H. R. (2014). Big data: New tricks for econometrics. J. Econ. Perspect. 28, 3–28. doi: 10.1257/jep.28.2.3
Vicsek, L. (2020). Artificial intelligence and the future of work—lessons from the sociology of expectations. Int. J. Sociol. Soc. Policy. 41, 842–861. doi: 10.1108/IJSSP-05-2020-0174
Von Krogh, G. (2018). Artificial intelligence in organizations: New opportunities for phenomenon-based theorizing. Academy Management Discoveries. doi: 10.5465/amd.2018.0084
Wallach, W., and Allen, C. (2009). Moral Machines: Teaching Robots Right from Wrong. Oxford University Press. doi: 10.1093/acprof:oso/9780195374049.001.0001
Weller, A. (2017). Transparency: Motivations and Challenges. Lecture Notes in Computer Science (Including Subseries Lecture Notes in Artificial Intelligence and Lecture Notes in Bioinformatics) 11700 LNCS, 23–40. doi: 10.1007/978-3-030-28954-6_2
Woods, S. A., Ahmed, S., Nikolaou, I., Costa, A. C., and Anderson, N. R. (2020). Personnel selection in the digital age: A review of validity and applicant reactions, and future research challenges. Eur. J. Work Organiz. Psychol. 29, 64–77. doi: 10.1080/1359432X.2019.1681401
Yam, K. C., Bigman, Y. E., Tang, P. M., Ilies, R., De Cremer, D., Soh, H., et al. (2021). Robots at work: people prefer—and forgive—service robots with perceived feelings. J. Appl. Psychol. 106, 1557. doi: 10.1037/apl0000834
Keywords: AI, ethical upskilling, moral agency, responsibility, ethical decision-making
Citation: De Cremer D and Narayanan D (2023) How AI tools can—and cannot—help organizations become more ethical. Front. Artif. Intell. 6:1093712. doi: 10.3389/frai.2023.1093712
Received: 09 November 2022; Accepted: 02 June 2023;
Published: 22 June 2023.
Edited by:
Alejo Sison, University of Navarra, SpainReviewed by:
Julian Jonker, University of Pennsylvania, United StatesCopyright © 2023 De Cremer and Narayanan. This is an open-access article distributed under the terms of the Creative Commons Attribution License (CC BY). The use, distribution or reproduction in other forums is permitted, provided the original author(s) and the copyright owner(s) are credited and that the original publication in this journal is cited, in accordance with accepted academic practice. No use, distribution or reproduction is permitted which does not comply with these terms.
*Correspondence: David De Cremer, Yml6ZGRjQG51cy5lZHUuc2c=
Disclaimer: All claims expressed in this article are solely those of the authors and do not necessarily represent those of their affiliated organizations, or those of the publisher, the editors and the reviewers. Any product that may be evaluated in this article or claim that may be made by its manufacturer is not guaranteed or endorsed by the publisher.
Research integrity at Frontiers
Learn more about the work of our research integrity team to safeguard the quality of each article we publish.