- 1Department of Biostatistics, The University of Texas MD Anderson Cancer Center, Houston, TX, United States
- 2Department of Clinical Cancer Prevention, The University of Texas MD Anderson Cancer Center, Houston, TX, United States
- 3Department of Breast Medical Oncology, The University of Texas MD Anderson Cancer Center, Houston, TX, United States
- 4Breast Cancer Research Program, Baylor College of Medicine, Houston, TX, United States
- 5Department of Abdominal Imaging, The University of Texas MD Anderson Cancer Center, Houston, TX, United States
- 6Department of Breast Imaging, The University of Texas MD Anderson Cancer Center, Houston, TX, United States
There is a need to identify biomarkers predictive of response to neoadjuvant chemotherapy (NACT) in triple-negative breast cancer (TNBC). We previously obtained evidence that a polyamine signature in the blood is associated with TNBC development and progression. In this study, we evaluated whether plasma polyamines and other metabolites may identify TNBC patients who are less likely to respond to NACT. Pre-treatment plasma levels of acetylated polyamines were elevated in TNBC patients that had moderate to extensive tumor burden (RCB-II/III) following NACT compared to those that achieved a complete pathological response (pCR/RCB-0) or had minimal residual disease (RCB-I). We further applied artificial intelligence to comprehensive metabolic profiles to identify additional metabolites associated with treatment response. Using a deep learning model (DLM), a metabolite panel consisting of two polyamines as well as nine additional metabolites was developed for improved prediction of RCB-II/III. The DLM has potential clinical value for identifying TNBC patients who are unlikely to respond to NACT and who may benefit from other treatment modalities.
Introduction
Triple-negative breast cancer (TNBC) accounts for ~15–20% of breast cancers and represents a heterogeneous subtype characterized by high pathological grade, strong invasiveness, local recurrence, high metastasis rate, and poor prognosis (Foulkes et al., 2010). TNBCs are defined based on the lack expression of estrogen receptor (ER), progesterone receptor (PR), and human epidermal growth factor receptor type 2 (HER2) and are thus not amenable to endocrine therapy or therapies targeted to the HER2 receptor type (Foulkes et al., 2010). Chemotherapy remains the mainstay of systemic treatment, typically consisting of anthracycline and taxane-based chemotherapy regimens (Foulkes et al., 2010; Bianchini et al., 2022). Platinum-based neoadjuvant chemotherapy has been shown to increase pathological complete response (pCR) rates compared to platinum-free neoadjuvant chemotherapy. However, platinum-based treatment is associated with higher rates of toxicity and treatment discontinuation, and the optimal integration of platinum-based agents remains controversial (Poggio et al., 2018). The addition of immunotherapy has shown promise with recent Phase III clinical trials demonstrating that the addition of the anti-PD-L1 inhibitor atezolizumab or the anti-PD1 inhibitor pembrolizumab with chemotherapy improved pCR compared to chemotherapy alone in patients with TNBC (Schmid et al., 2018, 2020).
In the curative neoadjuvant setting, a pCR after neoadjuvant chemotherapy (NACT) in TNBC is associated with improved long-term survival yielding estimated 10-year relapse survival rates of 86% (Symmans et al., 2017). However, up to 60% of patients will have residual disease after receiving standard NACT and are at an elevated risk of poor outcome, with reported 10-year estimated relapse survival rates of 81, 55, and 23% for TNBC patients with a residual cancer burden (RCB) index of I, II, and III, respectively (Huober et al., 2010; Symmans et al., 2017; Schmid et al., 2020). Currently, there is a paucity of biomarkers that can reliably identify TNBC patients that will have poor response to NACT.
Polyamines, including putrescine, spermidine, and spermine, are polycationic alkylamines that are essential for eukaryotic cell growth. Dysregulation of polyamine metabolism is frequent in cancer and polyamines have been reported to play central roles in neoplastic transformation and tumor progression (Park and Igarashi, 2013; Casero et al., 2018; Chia et al., 2022). We previously obtained evidence that increased plasma levels of the acetylated polyamine diacetylspermine (DAS) in TNBC was prognostic for poor progression-free survival and overall survival. Specifically, we found that elevated levels of plasma DAS to be prognostic for worse 5-year metastasis free survival and poor 5-year overall survival in newly-diagnosed treatment naïve TNBC patients (Fahrmann et al., 2020).
Here, we tested the utility of plasma polyamines for identifying subjects who will be insensitive to NACT as part of a comprehensive plasma metabolomics profiling. We further applied artificial intelligence to plasma metabolic profiles and, using a deep-learning model (DLM), established a metabolite biomarker panel consisting of two polyamines as well as nine additional metabolites for prediction of response to NACT.
Materials and Methods
Specimen Sets
Patients with stage I–III TNBC enrolled in the prospective, Institutional Review Board (IRB)-approved, clinical study, “A Robust TNBC Evaluation framework to Improve Survival” (ARTEMIS, NCT02276443), were included in this study. Briefly, the ARTEMIS trial included treatment-naïve patients with localized TNBC (stage I-III) that underwent a pre-treatment ultrasound with biopsy following by 4 cycles of Adriamycin-cyclophosphamide (AC) chemotherapy. The outcome of the molecular characterization from the pre-treatment biopsy in combination with response assessment (clinical exam/diagnostic imaging, after 4 cycles of AC) were used to identify chemotherapy-insensitive disease and to inform the second phase of neoadjuvant therapy. Patients deemed to have chemo-sensitive disease after 4 cycles of AC (≥70% volumetric reduction by ultrasound after 4 cycles of AC) were recommended to undergo standard paclitaxel-based chemotherapy as the second phase of their NACT consisting of 4 cycles or weekly for 12 doses. Patients with TNBC predicted to be chemo-insensitive (≤ 70% volumetric reduction by ultrasound after 4 cycles of AC) were offered therapy on clinical trials using targeted therapy in combination with chemotherapy based on the specific molecular characteristics of their tumor as the second phase of their therapy with dose regimens varying depending on therapy. Response to neoadjuvant therapy was determined using the residual cancer burden (RCB) index (Symmans et al., 2007). The specimen set consisted of pre-treatment EDTA plasma from 88 patients who received standard-of-care NACT; 62 of the 88 patients had a second plasma sample available after four cycles of AC. Detailed patient and tumor characteristics are provided in Table 1.
EDTA plasma from cancer-free women (n = 167) were obtained from the MD Anderson Cancer Center (MDACC) Longitudinal High-Risk Cohort initiated September 1st, 2011, for the prospective follow-up of cancer-free high-risk women seen in the MDACC Cancer Prevention Center (IRB protocol LAB07-0086).
Immunohistochemistry
Immunohistochemical (IHC) staining for Ki-67 was performed on unstained 4-μm-thick tissue sections that had been prepared from a representative paraffin block of tumor in each case. IHC staining for Ki-67 was performed using the polymeric biotin-free horseradish peroxidase method on the Leica Microsystems Bond III autostainer (Leica Microsystems, Buffalo Grove, IL, USA). The slides were incubated at 60°C for 25 min. Following heat-induced epitope retrieval with Tris-EDTA buffer for 20 min at 100°C, slides were incubated with mouse monoclonal antibody to Ki-67 (clone MIB-1, Dako; 1:100). The Refine Polymer Detection kit was used to detect bound antibody, with 3,3-diaminobenzidine serving as the chromogen (Leica Microsystems). For Ki-67, the percentage of any nuclear staining of any intensity in the tumor cells was recorded.
Metabolomic Analysis
Sample Preparation
Plasma metabolites were extracted from pre-aliquoted biospecimens (15 μL) with 45 μL of LCMS grade methanol (ThermoFisher) in a 96-well microplate (Eppendorf). Plates were heat sealed, vortexed for 5 min at 750 rpm, and centrifuged at 2,000 × g for 10 mins at room temperature. The supernatant (30 μL) was transferred to a 96-well plate, leaving behind the precipitated protein. The supernatant was further diluted with 60 μL of 100 mm ammonium formate, pH3 (Fisher Scientific). For Hydrophilic Interaction Liquid Chromatography (HILIC) positive ion analysis, 15 μL of the supernatant and ammonium formate mix were diluted with 195 μL of 1:3:8:144 water (GenPure ultrapure water system, Thermofisher): LCMS grade methanol (ThermoFisher): 100 mm ammonium formate, pH3 (Fisher Scientific): LCMS grade acetonitrile (ThermoFisher). For C18 analysis, 15 μL of the supernatant and ammonium formate mix were diluted with 90 μL water (GenPure ultrapure water system, ThermoFisher) for positive ion mode. Each sample solution was transferred to 384-well microplate (Eppendorf) for LCMS analysis.
Untargeted Analysis of Primary Metabolites and Biogenic Amines
Untargeted metabolomics analysis was conducted on Waters Acquity™ UPLC system with 2D column regeneration configuration (I-class and H-class) coupled to a Xevo G2-XS quadrupole time-of-flight (qTOF) mass spectrometer as previously described (Fahrmann et al., 2019, 2020, 2021a,b). Chromatographic separation was performed using HILIC (Acquity™ UPLC BEH amide, 100 Å, 1.7 μm 2.1 × 100 mm, Waters Corporation, Milford, U.S.A) and C18 (Acquity™ UPLC HSS T3, 100 Å, 1.8 μm, 2.1 × 100 mm, Water Corporation, Milford, U.S.A) columns at 45°C.
Quaternary solvent system mobile phases were (A) 0.1% formic acid in water, (B) 0.1% formic acid in acetonitrile and (D) 100 mm ammonium formate, pH 3. Samples were separated on the HILIC using the following gradient profile at 0.4 mL/min flow rate: (95% B, 5% D) linear change to (70% A, 25% B and 5% D) over 5 min; 100% A for 1 min; and 100% A for 1 min. For C18 separation, the chromatography gradient was as follows at 0.4 mL/min flow rate: 100% A with a linear change to (5% A, 95% B) over 5 min; (95% B, 5% D) for 1 min; and 1 min at (95% B, 5% D).
A binary pump was used for column regeneration and equilibration. The solvent system mobile phases were (A1) 100 mm ammonium formate, pH 3, (A2) 0.1% formic in 2-propanol and (B1) 0.1% formic acid in acetonitrile. The HILIC column was stripped using 90% A2 for 5 min at 0.25 mL/min flow rate, followed by a 2 min equilibration using 100% B1 at 0.3 mL/min flow rate. Reverse phase C18 column regeneration was performed using 95% A1, 5% B1 for 2 min followed by column equilibration using 5% A1, 95% B1 for 5 min at 0.4 mL/min flow rate.
Mass Spectrometry Data Acquisition
Mass spectrometry data was acquired using ‘sensitivity' mode in positive and negative electrospray ionization mode within 50–800 Da range. For the electrospray acquisition, the capillary voltage was set at 1.5 kV (positive), sample cone voltage 30 V, source temperature at 120°C, cone gas flow 50 L/h and desolvation gas flow rate of 800 L/h with scan time of 0.5 sec in continuum mode. Leucine Enkephalin; 556.2771 Da (positive) was used for lockspray correction and scans were performed at 0.5 sec. The injection volume for each sample was 6 μL. The acquisition was carried out with instrument auto gain control to optimize instrument sensitivity over the samples acquisition time.
Data were processed using Progenesis QI (Non-linear, Waters). Peak picking and retention time alignment of LC-MS and MSe data were performed using Progenesis QI software (Non-linear, Waters). Data processing and peak annotations were performed using an in-house automated pipeline as previously described (Fahrmann et al., 2019, 2020, 2021a; Vykoukal et al., 2020). Annotations were determined by matching accurate mass and retention times using customized libraries created from authentic standards and by matching experimental tandem mass spectrometry data against the NIST MSMS, LipidBlast or HMDB v3 theoretical fragmentations. To correct for injection order drift, each feature was normalized using data from repeat injections of quality control samples collected every 10 injections throughout the run sequence. Measurement data were smoothed by Locally Weighted Scatterplot Smoothing (LOESS) signal correction (QC-RLSC) as previously described. Values are reported as ratios relative to the median of historical quality control reference samples run with every analytical batch for the given analyte (Fahrmann et al., 2019, 2020, 2021a; Vykoukal et al., 2020).
Statistical Analysis
A deep learning algorithm employing all quantified metabolites with tuned hyperparameters using the grid search approach (Candel et al., 2016) was run 20 times, and the relevance importance score for each metabolite was calculated using the Gedeon method (Gedeon, 1997). Metabolites were prioritized based on consistently exhibiting a relative importance score >0.5. Ten models, including deep learning, random forest, ensemble learning and gradient boosting method algorithms, incorporating eleven metabolites were assessed for distinguishing responder/partial responders (RCB-0/I) from non-responders (RCB-II/III). The predictability, reliability, and stability of the models in the training set was evaluated using a 5-fold cross validation as well as through introducing perturbations (e.g., random sample selection with replacement) to the dataset.
Model discrimination was assessed based on receiver operating characteristic curve (ROC), as well as sensitivity and specificity estimates. The 95% confidence intervals (CI) for AUCs were estimated using the Delong method (DeLong et al., 1988). All modeling was performed using the H2O package and R statistical program (Candel et al., 2016).
Results
Plasma Polyamine Levels in Triple-Negative Breast Cancer
Using mass spectrometry, we first assessed polyamines levels in plasmas from 88 newly diagnosed treatment-naïve TNBC cases and 167 cancer-free women enrolled in the MDACC Longitudinal High-Risk Cohort (Table 1). A total of four polyamines, acetylspermidine (AcSpmd), diacetylspermidine (DiAcSpmd), diacetylspermine (DAS), and N-(3-acetamidopropyl)pyrrolidin-2-one (N3AP) were detected and quantified. Of these, AcSpmd and DAS were statistically significantly elevated (Wilcoxon rank sum test 2-sided p < 0.01) in case plasmas compared to controls (Figure 1). DAS exhibited the highest discrimination performance for distinguishing all cases from controls with an AUC of 0.72 (95% C.I.: 0.64–0.79) (Figure 1).
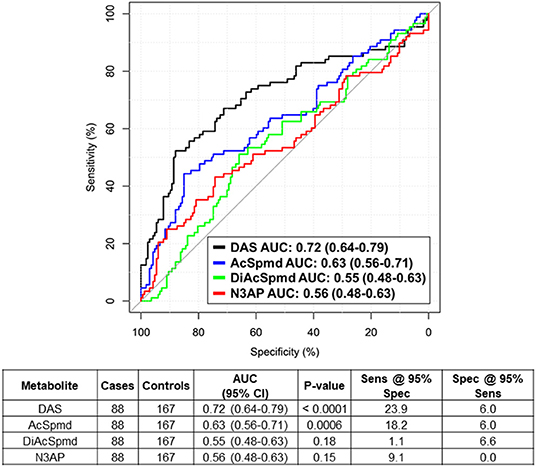
Figure 1. Predictive performance of individual polyamines for distinguishing treatment-naïve TNBC cases from healthy controls. Table beneath shows AUC (95% CI), Wilcoxon rank sum test 2-sided P-values as well as sensitivity and specificity estimates at 95% specificity/sensitivity thresholds of individual polyamines for distinguishing TNBC cases from healthy controls.
Association of Polyamines With RCB Status
All 88 TNBC patients were treated with AC in the neoadjuvant setting. A subset of 62 (70.5%) had a complete pathological response (pCR/RCB-0) or minimal residual disease (RCB-I) following NACT, whereas 26 (29.5%) had a moderate to extensive tumor burden (RCB-II and III) (Table 1). Pathological response tended to be associated with tumor stage and % tumoral Ki-67 staining positivity, albeit not statistically significant (Supplementary Figure 1).
Elevated pre-treatment plasma levels of AcSpmd, N3AP, DiAcSpmd and DAS were associated with higher odds of RCB-II/III following NACT [adjusted ORs of 1.24 (95% CI: 0.76–2.04), 1.33 (95% CI: 0.79–2.46), 1.15 (95% CI: 0.71–1.85) and 1.26 (95% CI: 0.71–1.91) per standard deviation increase, respectively] (Table 2).
Applying Artificial Intelligence to Metabolic Profiles to Develop a Combination Rule for Prediction of RCB-II/III
Complementary to the four polyamines, untargeted metabolomics analyses of these plasmas yielded an additional 82 uniquely annotated metabolite features (Supplementary Table 1). To prioritize metabolites associated with response to NACT for model building, relative importance scores were calculated using the Gedeon method (Gedeon, 1997) and metabolites were selected that constantly showed a relative important score of > 0.5 (see Methods). This approach resulted in 11 cancer-related metabolites, consisting of two polyamines, two lipids, three amino acids, a purine catabolite, and two indole-derivatives (Supplementary Table 2). Spearman correlation analyses indicated low to moderate associations between these metabolites (Supplementary Figure 2).
We next sought to develop a machine learning algorithm that incorporated the eleven metabolites for predicting RCB-II/III. For model building, we tested 10 different machine learning algorithms (Table 3). Of these, a deep learning model (DLM) with 3 hidden layers and 20 nodes in each layer achieved the highest predictive performance with an AUC of 0.97 (95% CI: 0.93–1.00) with 85% sensitivity at 95% specificity for identifying RCB-II/III (Figure 2). Notably, the DLM yielded an AUC of 0.76 (95% CI: 0.65–0.87) with 48% sensitivity at 95% specificity for distinguishing TNBC cases with residual disease (RCB-I/II/III) from those that achieved a pCR (Supplementary Table 3). To assess model reproducibility and stability, we introduced perturbation into the dataset (e.g., random selection with replacement) and re-evaluated model performance, the results of which showed that the DLM was robust (Supplementary Table 4).
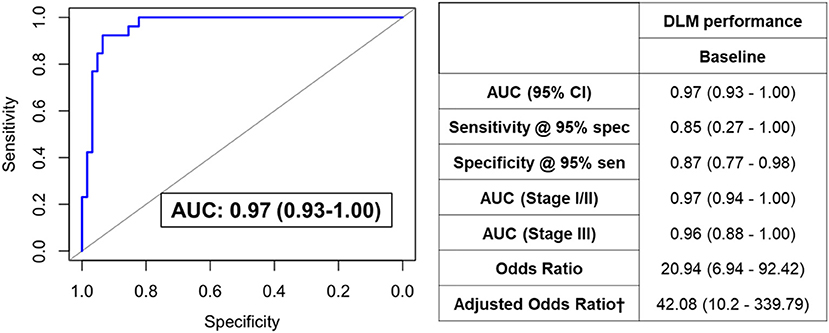
Figure 2. ROC curve for the DLM for distinguishing TNBC patients that went on to have RCB-II/III following NACT from those that had RCB-0/I. Table provides tabulated performance estimates of the DLM.†Age and stage were included as covariables in adjusted odd ratios.
We additionally assessed the predictive performance of the DLM model using plasma samples collected during NACT from a subset of TNBC patients (n = 62). The DLM model showed an AUC of 0.74 (95% CI: 0.62–0.87) with 21% sensitivity at 95% specificity for RCB-II/III (Figure 3).
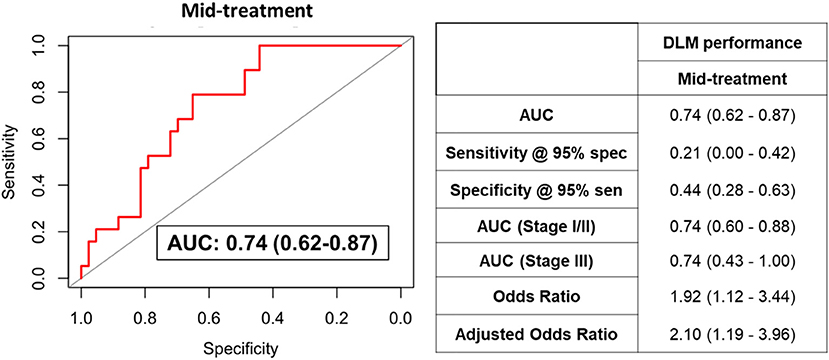
Figure 3. ROC curve for the DLM for distinguishing TNBC patients that went on to have RCB-II/III following NACT from those that had RCB-0/I using plasmas collected after four cycles of AC. Table provides tabulated performance estimates of the DLM.†Age and stage were included as covariables in adjusted odd ratios.
Discussion
The heterogeneity of TNBC results in a spectrum of responses to NACT with pCR being achieved in only a subset of patients (Sikov et al., 2015; Gamucci et al., 2018). Several methods have been used to measure and predict residual disease during course of treatment, including ultrasound, MRI scans, histopathology; however, none have yet achieved adequate performance to predict response to NACT (Croshaw et al., 2011; Shin et al., 2011; Ono et al., 2012; De Los Santos et al., 2013; Leon-Ferre et al., 2018). Here, we applied artificial intelligence to metabolomic profiles of TNBC patient plasmas obtained prior to NACT and, using a DLM, establish a blood-based polyamine-centric metabolite panel that is predictive of non-response to NACT. The metabolite panel may be implemented in the clinical setting to stratify TNBC patients who are at high-risk of being non-responsive to NACT and who may benefit from alternate treatment modalities. Conversely, TNBC patients who are likely to be responsive to NACT may potentially benefit from dose de-escalation, thereby permitting management of treatment-associated toxicity.
The metabolite panel consisted of several cancer-relevant metabolites including the acetylated polyamines DAS and AcSpmd, which were found to be elevated in TNBC patients who were less likely to respond to NACT. Elevated levels of acetylated polyamines in various biofluids including urine, plasma, and serum, have been shown to report on cancer status (Park and Igarashi, 2013; Wikoff et al., 2015; Fahrmann et al., 2019, 2020, 2021b). Targeting of cancer cell polyamine metabolism via small molecule inhibitors has been proposed for anti-cancer therapy for cancer, including TNBC (Casero et al., 2018; Geck et al., 2020; Capellen et al., 2021). Acetylation of polyamines is mediated by spermidine/spermine N1-acetyltransferase 1 (SAT1) (Pegg, 2013; Fahrmann et al., 2020). Our prior investigations demonstrated that oncogenic MYC regulates transcription of polyamine metabolizing enzymes ornithine decarboxylase (ODC1), spermidine synthase (SRM), and spermine synthase (SMS) in TNBC, and that elevated intracellular polyamine levels induce expression of SAT1 (Pegg, 2013) resulting in elevated cancer cell biosynthesis and secretion of acetylated polyamines (Fahrmann et al., 2020). We further reported that plasma polyamines, particularly DAS, are associated with TNBC development and progression (Fahrmann et al., 2020). Given our prior findings, we posit that the elevation in polyamines may underly an aggressive subtype of TNBC (Fahrmann et al., 2020) that is less likely to respond to NACT.
Elevated serum levels of urate, a purine catabolite, are also reported to be prognostic for TNBC recurrence and poor overall survival (Ackermann and Tardito, 2019; Gong et al., 2021). Lysophosphatidylethanolamines and lauroylcarnitine are associated with cancer metabolic plasticity and fatty acid oxidation (Melone et al., 2018). We have previously reported that JAK/STAT3-mediated fatty acid oxidation promotes chemoresistance in TNBC (Chakraborty et al., 2016; Wang et al., 2018). Methylhistidine has been shown to be elevated in serum of TNBC patients who had an cPR following NACT (He et al., 2021). TNBC cells are reported to exhibit a glutamine-dependent phenotype; promoting survival advantage as well as chemo-resistance (Kung et al., 2011; Lampa et al., 2017).
Remarkably, among the metabolites in the metabolite panel were two microbiome-related metabolites, indoleacrylic acid (IAA) and indole-acetylaldehyde (IAALD). IAA and IAALD are produced through the catabolism of tryptophan by the gut microbes (Vujkovic-Cvijin et al., 2013). Increasing evidence implicates that alterations in the microbiome influence resistance to anticancer treatment, including conventional chemotherapy, immunotherapy, radiotherapy, and surgery (Pryor et al., 2020; Garajová et al., 2021; Pandey and Umar, 2021). The relationship between changes in the microbiome and response to NACT warrants further investigation.
On balance, limitations to our study include limited sample availability and lack of external validation. To assess for potential overfitting, we tested the model by introducing perturbation (e.g., random selection and replacement) to the dataset and re-evaluated performance, the results of which demonstrated that our model was robust. We performed further validation using available samples and found that the metabolite panel provided good classifier performance for distinguishing individuals with RCB-II/III following four cycles of NACT from those with RCB-0/I, thus providing an independent validation. We note that attenuation of model performance after four cycles of NACT could be attributable to elevations in plasma metabolites consistent with chemotherapy-induced cancer cell death and turnover. Additionally, the relative cost-effectiveness of using the metabolite panel for risk-based prediction of non-responsiveness to NACT needs to be considered compared to other clinical predictors (Croshaw et al., 2011; Shin et al., 2011; Ono et al., 2012; De Los Santos et al., 2013; Leon-Ferre et al., 2018).
In conclusion, using a deep learning model, we developed a blood-based metabolite panel and that offers potential utility for identifying TNBC patients who are at high-risk of being non-responsive to NACT and who may benefit from more personalized treatment modalities.
Data Availability Statement
The data presented in the study is available at the NIH Common Fund's National Metabolomics Data Repository (NMDR) website, the Metabolomics Workbench, where it has been assigned Study ID ST002235. The data can be accessed directly via its Project DOI: http://dx.doi.org/10.21228/M8HX4C.
Ethics Statement
The studies involving human participants were reviewed and approved by patients with stage I–III TNBC enrolled in the prospective, Institutional Review Board (IRB)-approved, clinical study, A Robust TNBC Evaluation framework to Improve Survival (ARTEMIS, NCT02276443), were included in this study. Cancer-free women (n = 167) were obtained from the MD Anderson Cancer Center (MDACC) Longitudinal High-Risk Cohort initiated September 1st, 2011, for the prospective follow-up of cancer-free high-risk women seen in the MDACC Cancer Prevention Center (IRB protocol LAB07-0086). The patients/participants provided their written informed consent to participate in this study.
Author Contributions
EI, SH, and JF: conceptualization. EI, RW, EM, JD, JLo, KD, and JF: methodology. EI and JF: validation, visualization, writing-original draft preparation, and formal analysis. ER, BL, JL, DT, VV, SD, GR, BAd, BAr, RC, JW, and AB: resources. RW, EM, JD, JW, and JF: data curation. RW, JV, EM, RS, JD, SM, ER, BL, JLi, DT, VV, SD, GR, BAd, RC, JW, AB, BAr, JLo, KD, and SH: writing-review and editing. SH and JF: supervision. JLo, KD, and SH: funding acquisition.
Funding
The work presented was supported by Cancer Prevention & Research Institute of Texas (CPRIT) (RP180505) (SH), the generous philanthropic contributions to the University of Texas MD Anderson Cancer Center Moon Shots Program™, the Little Green Book Foundation, and the Still Water Foundation. KD was partially supported by a Cancer Center Support Grant NCI Grant P30 CA016672, NIH grants UL1TR003167, 5R01GM122775. JLo was partially supported by the National Cancer Institute and the National Center for Advancing Translational Sciences of the NIH (P30CA016672 and CCTS UL1TR003167).
Conflict of Interest
Author SM declares honoraria from Novartis, Pfizer and research funding from Oncothyreon (Inst), Pfizer (Inst), Novartis (Inst), Genentech (Inst), Takeda (Inst), Bayer (Inst), EMD Serono (Inst), Genentech (Inst); travel, accommodations, expenses: Novartis, Pfizer. Author JLi declares consulting or advisory role: Pfizer, AstraZeneca, Medivation/Pfizer; speakers' bureau from Physician Education Resource, UpToDate, Med Learning, Group, Medscape; research funding from Novartis (Inst), Bristol-Myers Squibb (Inst), Genentech (Inst), Pfizer (Inst), EMD Serono (Inst), Jounce Therapeutics (Inst), GlaxoSmithKline (Inst), Medivation/Pfizer (Inst); patents, royalties, other intellectual property: UpToDate; travel, accommodations, expenses: Physician Education Resource, Med Learning Group, Medscape. Author DT declares consulting or advisory role: AstraZeneca, Genomic Health, Gilead Sciences Inc, GlaxoSmithKline, Novartis Pharma, OncoPep, Pfizer; research funding: Pfizer, Novartis Pharma. Author VV declares honoraria: Genentec; consulting or advisory role: Genentech; travel, accommodations, expenses: Genentech. Author SD declares honoraria: Novartis; consulting or advisory role: Tempus, Taiho Pharmaceutical, Pfizer; research funding: EMD Serono, Guardant Health; travel, accommodations; expenses: Phillips Gilmore Oncology Communications. Author BAd declares consulting or advisory role: Bright Pink, AbbVie; research funding: AbbVie (Inst), PharmaMar (Inst), AstraZeneca (Inst), InVitae (Inst); travel, accommodations, expenses: AstraZeneca.
The remaining authors declare that the research was conducted in the absence of any commercial or financial relationships that could be construed as a potential conflict of interest.
Publisher's Note
All claims expressed in this article are solely those of the authors and do not necessarily represent those of their affiliated organizations, or those of the publisher, the editors and the reviewers. Any product that may be evaluated in this article, or claim that may be made by its manufacturer, is not guaranteed or endorsed by the publisher.
Supplementary Material
The Supplementary Material for this article can be found online at: https://www.frontiersin.org/articles/10.3389/frai.2022.876100/full#supplementary-material
References
Ackermann, T., and Tardito, S. (2019). Cell culture medium formulation and its implications in cancer metabolism. Trends Cancer. 5, 329–332. doi: 10.1016/j.trecan.2019.05.004
Bianchini, G., De Angelis, C., Licata, L., and Gianni, L. (2022). Treatment landscape of triple-negative breast cancer - expanded options, evolving needs. Nat. Rev. Clin. Oncol. 19, 91–113. doi: 10.1038/s41571-021-00565-2
Candel, A., Parmar, V., LeDell, E., and Arora, A. (2016). Deep learning with H2O. H2O ai Inc. 2016, 1–21. Available online at: https://docs.h2o.ai/h2o/latest-stable/h2o-docs/booklets/DeepLearningBooklet.pdf
Capellen, C. C., Ortega-Rodas, J., Morwitzer, M. J., Tofilau, H. M. N., Dunworth, M., Casero, R. A., et al. (2021). Hyperglycemic conditions proliferate triple negative breast cancer cells: role of ornithine decarboxylase. Breast Cancer Res. Treat. 190, 255–264. doi: 10.1007/s10549-021-06388-0
Casero, R. A., Murray Stewart, T., and Pegg, A. E. (2018). Polyamine metabolism and cancer: treatments, challenges and opportunities. Nat. Rev. Cancer. 18, 681–695. doi: 10.1038/s41568-018-0050-3
Chakraborty, S., Ghosh, S., Banerjee, B., Santra, A., Adhikary, A., Misra, A. K., et al. (2016). Phemindole, a synthetic di-indole derivative maneuvers the store operated calcium entry (SOCE) to induce potent anti-carcinogenic activity in human triple negative breast cancer cells. Front. Pharmacol. 7, 114. doi: 10.3389/fphar.2016.00114
Chia, T. Y., Zolp, A., and Miska, J. (2022). Polyamine immunometabolism: central regulators of inflammation, cancer and autoimmunity. Cells. 11, 5. doi: 10.3390/cells11050896
Croshaw, R., Shapiro-Wright, H., Svensson, E., Erb, K., and Julian, T. (2011). Accuracy of clinical examination, digital mammogram, ultrasound, and MRI in determining postneoadjuvant pathologic tumor response in operable breast cancer patients. Annal. Surgic. Oncol. 18, 3160–3163. doi: 10.1245/s10434-011-1919-5
De Los Santos, J. F., Cantor, A., Amos, K. D., Forero, A., Golshan, M., Horton, J. K., et al. (2013). Magnetic resonance imaging as a predictor of pathologic response in patients treated with neoadjuvant systemic treatment for operable breast cancer: translational breast cancer research consortium trial 017. Cancer. 119, 1776–1783. doi: 10.1002/cncr.27995
DeLong, E. R., DeLong, D. M., and Clarke-Pearson, D. L. (1988). Comparing the areas under two or more correlated receiver operating characteristic curves: a nonparametric approach. Biometrics. 44, 837–845. doi: 10.2307/2531595
Fahrmann, J. F., Bantis, L. E., Capello, M., Scelo, G., Dennison, J. B., Patel, N., et al. (2019). A Plasma-derived protein-metabolite multiplexed panel for early-stage pancreatic cancer. J. Natl. Cancer Inst. 111, 372–379. doi: 10.1093/jnci/djy126
Fahrmann, J. F., Irajizad, E., Kobayashi, M., Vykoukal, J., Dennison, J. B., Murage, E., et al. (2021a). A MYC-driven plasma polyamine signature for early detection of ovarian cancer. Cancers. 13, 4. doi: 10.3390/cancers13040913
Fahrmann, J. F., Vykoukal, J., Fleury, A., Tripathi, S., Dennison, J. B., Murage, E., et al. (2020). Association between plasma diacetylspermine and tumor spermine synthase with outcome in triple-negative breast cancer. J. Natl. Cancer Inst. 112, 607–616. doi: 10.1093/jnci/djz182
Fahrmann, J. F., Wasylishen, A. R., Pieterman, C. R. C., Irajizad, E., Vykoukal, J., Murage, E., et al. (2021b). A blood-based polyamine signature associated with men1 duodenopancreatic neuroendocrine tumor progression. J. Clin. Endocrinol. Metab. 106, e4969–e4980.doi: 10.1142/s0129065797000227
Foulkes, W. D., Smith, I. E., and Reis-Filho, J. S. (2010). Triple-negative breast cancer. New Engl. J. Med. 363, 1938–1948. doi: 10.1056/NEJMra1001389
Gamucci, T., Pizzuti, L., Sperduti, I., Mentuccia, L., Vaccaro, A., Moscetti, L., et al. (2018). Neoadjuvant chemotherapy in triple-negative breast cancer: A multicentric retrospective observational study in real-life setting. J. Cell. Physiol. 233, 2313–2323. doi: 10.1002/jcp.26103
Garajová, I, Balsano, R., Wang, H., Leonardi, F., Giovannetti, E., Deng, D., et al. (2021). The role of the microbiome in drug resistance in gastrointestinal cancers. Exper. Rev. Anticancer Therapy. 21, 165–176. doi: 10.1080/14737140.2021.1844007
Geck, R. C., Foley, J. R., Murray Stewart, T., Asara, J. M., Casero, R. A. Jr., and Toker, A. (2020). Inhibition of the polyamine synthesis enzyme ornithine decarboxylase sensitizes triple-negative breast cancer cells to cytotoxic chemotherapy. J. Biol. Chem. 295, 6263–6277. doi: 10.1074/jbc.RA119.012376
Gedeon, T. D. (1997). Data mining of inputs: analysing magnitude and functional measures. Int. J. Neural Syst. 8, 209–218. doi: 10.1142/S0129065797000227
Gong, Y., Ji, P., Yang, Y.-S., Xie, S., Yu, T.-J., Xiao, Y., et al. (2021). Metabolic-pathway-based subtyping of triple-negative breast cancer reveals potential therapeutic targets. Cell Metabol. 33, 51–64. doi: 10.1016/j.cmet.2020.10.012
He, X., Gu, J., Zou, D., Yang, H., Zhang, Y., Ding, Y., et al. (2021). NMR-based metabolomics analysis predicts response to neoadjuvant chemotherapy for triple-negative breast cancer. Front. Mol. Biosci. 8, 21. doi: 10.3389/fmolb.2021.708052
Huober, J., von Minckwitz, G., Denkert, C., Tesch, H., Weiss, E., Zahm, D. M., et al. (2010). Effect of neoadjuvant anthracycline–taxane-based chemotherapy in different biological breast cancer phenotypes: overall results from the GeparTrio study. Breast Cancer Res. Treat. 124, 133–140. doi: 10.1007/s10549-010-1103-9
Kung, H. N., Marks, J. R., and Chi, J. T. (2011). Glutamine synthetase is a genetic determinant of cell type-specific glutamine independence in breast epithelia. PLoS Genet. 7, e1002229. doi: 10.1371/journal.pgen.1002229
Lampa, M., Arlt, H., He, T., Ospina, B., Reeves, J., Zhang, B., et al. (2017). Glutaminase is essential for the growth of triple-negative breast cancer cells with a deregulated glutamine metabolism pathway and its suppression synergizes with mTOR inhibition. PLoS One. 12, e0185092. doi: 10.1371/journal.pone.0185092
Leon-Ferre, R. A., Polley, M.-Y., Liu, H., Gilbert, J. A., Cafourek, V., Hillman, D. W., et al. (2018). Impact of histopathology, tumor-infiltrating lymphocytes, and adjuvant chemotherapy on prognosis of triple-negative breast cancer. Breast Cancer Res. Treat. 167, 89–99. doi: 10.1007/s10549-017-4499-7
Melone, M. A. B., Valentino, A., Margarucci, S., Galderisi, U., Giordano, A., and Peluso, G. (2018). The carnitine system and cancer metabolic plasticity. Cell Death Dis. 9, 1–12. doi: 10.1038/s41419-018-0313-7
Ono, M., Tsuda, H., Shimizu, C., Yamamoto, S., Shibata, T., Yamamoto, H., et al. (2012). Tumor-infiltrating lymphocytes are correlated with response to neoadjuvant chemotherapy in triple-negative breast cancer. Breast Cancer Res. Treat. 132, 793–805. doi: 10.1007/s10549-011-1554-7
Pandey, K., and Umar, S. (2021). Microbiome in drug resistance to colon cancer. Curr. Opin. Physiol. 23, 100472. doi: 10.1016/j.cophys.2021.100472
Park, M. H., and Igarashi, K. (2013). Polyamines and their metabolites as diagnostic markers of human diseases. Biomol. Ther. (Seoul). 21, 1–9. doi: 10.4062/biomolther.2012.097
Pegg, A. E. (2013). Toxicity of polyamines and their metabolic products. Chem. Res. Toxicol. 26, 1782–1800. doi: 10.1021/tx400316s
Poggio, F., Bruzzone, M., Ceppi, M., Pond,é, N. F, La Valle, G., Del Mastro, L., et al. (2018). Platinum-based neoadjuvant chemotherapy in triple-negative breast cancer: a systematic review and meta-analysis. Annal. Oncol. 29, 1497–1508. doi: 10.1093/annonc/mdy127
Pryor, R., Martinez-Martinez, D., Quintaneiro, L., and Cabreiro, F. (2020). The role of the microbiome in drug response. Ann. Rev. Pharmacol. Toxicol. 60, 417–435. doi: 10.1146/annurev-pharmtox-010919-023612
Schmid, P., Adams, S., Rugo, H. S., Schneeweiss, A., Barrios, C. H., Iwata, H., et al. (2018). Atezolizumab and nab-paclitaxel in advanced triple-negative breast cancer. New Engl. J. Med. 379, 2108–2121. doi: 10.1056/NEJMoa1809615
Schmid, P., Salgado, R., Park, Y. H., Muñoz-Couselo, E., Kim, S. B., Sohn, J., et al. (2020). Pembrolizumab plus chemotherapy as neoadjuvant treatment of high-risk, early-stage triple-negative breast cancer: results from the phase 1b open-label, multicohort KEYNOTE-173 study. Annal. Oncol. 31, 569–581. doi: 10.1016/j.annonc.2020.01.072
Shin, H., Kim, H., Ahn, J., Kim, S., Jung, K., Gong, G., et al. (2011). Comparison of mammography, sonography, MRI and clinical examination in patients with locally advanced or inflammatory breast cancer who underwent neoadjuvant chemotherapy. Br. J. Radiol. 84, 612–620. doi: 10.1259/bjr/74430952
Sikov, W. M., Berry, D. A., Perou, C. M., Singh, B., Cirrincione, C. T., Tolaney, S. M., et al. (2015). Impact of the addition of carboplatin and/or bevacizumab to neoadjuvant once-per-week paclitaxel followed by dose-dense doxorubicin and cyclophosphamide on pathologic complete response rates in stage II to III triple-negative breast cancer: CALGB 40603 (Alliance). J. clinic. oncol. 33, 13. doi: 10.1200/JCO.2014.57.0572
Symmans, W. F., Peintinger, F., Hatzis, C., Rajan, R., Kuerer, H., Valero, V., et al. (2007). Measurement of residual breast cancer burden to predict survival after neoadjuvant chemotherapy. J. Clinic. Oncol. 25, 4414–4422. doi: 10.1200/JCO.2007.10.6823
Symmans, W. F., Wei, C., Gould, R., Yu, X., Zhang, Y., Liu, M., et al. (2017). Long-term prognostic risk after neoadjuvant chemotherapy associated with residual cancer burden and breast cancer subtype. J. Clinic. Oncol. 35, 1049–1060. doi: 10.1200/JCO.2015.63.1010
Vujkovic-Cvijin, I., Dunham, R. M., Iwai, S., Maher, M. C., Albright, R. G., Broadhurst, M. J., et al. (2013). Dysbiosis of the gut microbiota is associated with HIV disease progression and tryptophan catabolism. Sci. Translat. Med. 5, 1931. doi: 10.1126/scitranslmed.3006438
Vykoukal, J., Fahrmann, J. F., Gregg, J. R., Tang, Z., Basourakos, S., Irajizad, E., et al. (2020). Caveolin-1-mediated sphingolipid oncometabolism underlies a metabolic vulnerability of prostate cancer. Nat Commun. 11, 4279. doi: 10.1038/s41467-020-17645-z
Wang, T., Fahrmann, J. F., Lee, H., Li, Y. J., Tripathi, S. C., Yue, C., et al. (2018). JAK/STAT3-Regulated fatty acid β-oxidation is critical for breast cancer stem cell self-renewal and chemoresistance. Cell Metab. 27, 136–150. doi: 10.1016/j.cmet.2017.11.001
Wikoff, W. R., Hanash, S., DeFelice, B., Miyamoto, S., Barnett, M., Zhao, Y., et al. (2015). Diacetylspermine is a novel prediagnostic serum biomarker for non-small-cell lung cancer and has additive performance with pro-surfactant protein B. J. Clin. Oncol. 33, 3880–3886. doi: 10.1200/JCO.2015.61.7779
Keywords: triple-negative breast cancer, biomarkers, artificial intelligence, deep-learning model, neoadjuvant chemotherapy, prediction
Citation: Irajizad E, Wu R, Vykoukal J, Murage E, Spencer R, Dennison JB, Moulder S, Ravenberg E, Lim B, Litton J, Tripathym D, Valero V, Damodaran S, Rauch GM, Adrada B, Candelaria R, White JB, Brewster A, Arun B, Long JP, Do KA, Hanash S and Fahrmann JF (2022) Application of Artificial Intelligence to Plasma Metabolomics Profiles to Predict Response to Neoadjuvant Chemotherapy in Triple-Negative Breast Cancer. Front. Artif. Intell. 5:876100. doi: 10.3389/frai.2022.876100
Received: 15 February 2022; Accepted: 03 May 2022;
Published: 11 August 2022.
Edited by:
Jaume Reventos, Institut d'Investigacio Biomedica de Bellvitge (IDIBELL), SpainReviewed by:
Miguel Abal, Health Research Institute of Santiago de Compostela (IDIS), SpainDavid Sarrio, Centro de Investigación Biomédica en Red del Cáncer (CIBERONC), Spain
Copyright © 2022 Irajizad, Wu, Vykoukal, Murage, Spencer, Dennison, Moulder, Ravenberg, Lim, Litton, Tripathym, Valero, Damodaran, Rauch, Adrada, Candelaria, White, Brewster, Arun, Long, Do, Hanash and Fahrmann. This is an open-access article distributed under the terms of the Creative Commons Attribution License (CC BY). The use, distribution or reproduction in other forums is permitted, provided the original author(s) and the copyright owner(s) are credited and that the original publication in this journal is cited, in accordance with accepted academic practice. No use, distribution or reproduction is permitted which does not comply with these terms.
*Correspondence: Sam Hanash, c2hhbmFzaCYjeDAwMDQwO21kYW5kZXJzb24ub3Jn; Johannes F. Fahrmann, amZmYWhybWFubiYjeDAwMDQwO21kYW5kZXJzb24ub3Jn