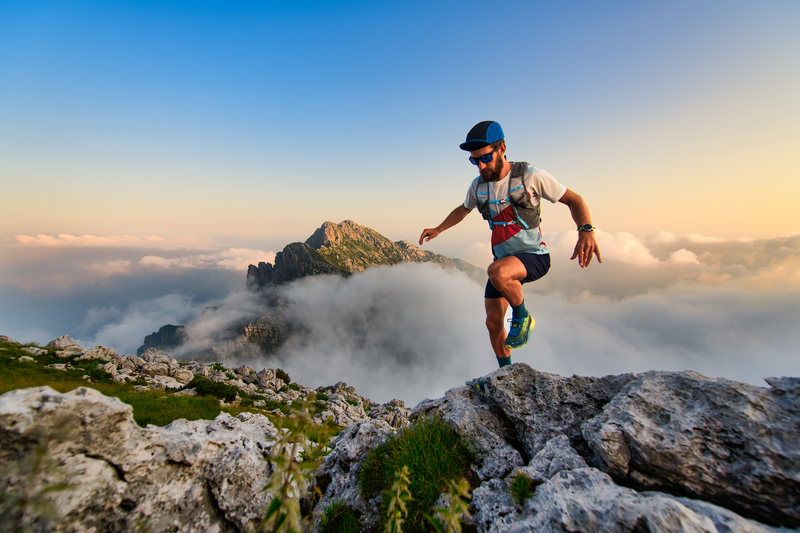
94% of researchers rate our articles as excellent or good
Learn more about the work of our research integrity team to safeguard the quality of each article we publish.
Find out more
ORIGINAL RESEARCH article
Front. Artif. Intell. , 29 September 2022
Sec. Medicine and Public Health
Volume 5 - 2022 | https://doi.org/10.3389/frai.2022.1011524
Artificial intelligence has been prevalent recently as its use in the medical field is noticed to be increased. However, middle east countries like Syria are deficient in multiple AI implementation methods in the field of medicine. So, holding these AI implementation methods in the medical field is necessary, which may be incredibly beneficial for making diagnosis more accessible and help in the treatment. This paper intends to determine AI's knowledge, attitude, and practice among doctors and medical students in Syria. A questionnaire conducted an online cross-sectional study on the google form website consisting of demographic data, knowledge, and perception of AI. There were 1,494 responses from both doctors and medical students. We included Syrian medical students and doctors who are currently residing in Syria. Of the 1,494 participants, 255 (16.9%) are doctors, while the other 1,252 (83.1%) are undergraduate medical students. About 1,055 (70%) participants have previous knowledge about AI. However, only 357 (23.7%) participants know about its application in the medical field. Most have shown positive attitudes toward its necessity in the medical field; 689 (45.7%) individuals strongly agree, and 628 (41.7%) agree. The undergraduate students had 3.327 times more adequate knowledge of AI than students in the first year. In contrast, the undergraduate 6th-year students had 2.868 times the attitude toward AI higher than students in the first year. The residents and assistant professors had 2.371 and 4.422 times the practice of AI higher than students, respectively. Although most physicians and medical students do not sufficiently understand AI and its significance in the medical field, they have favorable views regarding using AI in the medical field. Syrian medical authorities and international organizations should suggest including artificial intelligence in the medical field, particularly when training residents and fellowship physicians.
Artificial intelligence is a software system that attempts to simulate human intellect by using data sources to make independent decisions or assist humans in making decisions (He et al., 2019; Hashimoto et al., 2020). It is a general term that includes machine learning, representation learning, deep learning, and natural language processing. Artificial intelligence is a field of computer science that can analyze large amounts of data. However, it is not only related to computer science but extends into many areas such as medicine, philosophy, psychology, linguistics, and statistics (He et al., 2019). In medicine, it has contributed to treating several illnesses and decreased many mistakes in diagnosis and follow-up (Patel et al., 2009; Wang et al., 2019). It has increased recently, expanding from research to application in various sectors (Alami et al., 2020). High-income countries have provided substantial financial support for AI research, especially in the medical field. In low-income countries like Syria, there are no serious plans to implement AI, furthermore a lack of research about it (WHO, 2017). According to WHO, by 2035, there will be a shortage of nearly 12.9 million health care workers worldwide (WHO, 2017). In addition, in the last 60 years, artificial intelligence (AI) has seen significant development. However, machine learning use in low-resource countries has remained comparatively slight (Wahl et al., 2018; Triantafyllidis and Tsanas, 2019). Machine learning has been used in many medical sectors, including Diabetes, cancer, cardiology, mental health, radiology, and others (Thrall et al., 2018). Radiology provides a more direct pathway for AI because it offers digitally coded images that can be easily converted into computer language (Niazi et al., 2019). Several studies have suggested the critical role of AI in pathology (Souza Filho et al., 2020). It can provide image-based diagnosis options and increase the pathologist's understanding of microscopic slides by inserting electronic slides and computer-aided diagnostic procedures. Machine learning and deep learning have been used in cardiology to identify patients at risk of rapid coronary plaque progression, anticipate the chance of a heart attack, and determine prognosis in pulmonary hypertension patients (Bonderman, 2017; Wozniacka et al., 2021). Artificial intelligence is becoming more relevant than dermatologists, especially in diagnosing skin lesions from clinical and dermoscopic images (Niel and Bastard, 2019). AI techniques are developing in ophthalmology, particularly in diabetic retinopathy, age-related macular degeneration, and retinopathy of prematurity (Hashimoto et al., 2020). It was applied in nephrology to raise medical management, hemodialysis treatments, and transplant patient follow-up (Hessler and Baringhaus, 2018). It has a wide application in drug design, including de novo chemical compound and peptide design, as well as synthesis planning (Chan and Zary, 2019). The healthcare sector seems to be well-suited for transformation by AI. By transcribing notes, inputting and arranging patient data onto portals (such as EPIC), and diagnosing patients, AI systems might possibly spare up time for busy doctors and serve as a second opinion for them. In addition, patients may benefit from the availability of alternatives to prescribed medications and follow-up treatment from artificially intelligent systems. Aside from the world's main metropolitan centers, AI has the potential to diagnose patients remotely, enabling the expansion of medical services into rural regions. There is still much work to be done, but the future of AI in healthcare is bright and hopeful. AI may help lessen some of the drawbacks of traditional methods of diagnosis and treatment, including the risk of errors because of burnout in the medical field and other psychological impacts, the need to examine many patients quickly, the occasional inaccuracy of the diagnosis, and patients' anxiety when confronted by a clinical doctor. However, there are also unbelievable preconceptions of what AI is capable of and how the future of the healthcare sector will pan out, such as the assumption that AI will eventually replace doctors and that programming skills are required to use AI effectively. It was necessary to introduce AI in medical education because it can provide specific feedback to support learning and better understand AI algorithms (Rabaan et al., 2022). There were significant obstacles to integrating AI in the healthcare sector after the Syrian conflict, including the disintegration of healthcare services, the devastation of hospitals, the migration of healthcare staff, and the fall in medical education and training. This study aims to identify the level of doctors' and medical students' know carried perceptions of AI and its implications in Syria and evaluate their understanding of various AI practices.
During the period 7 April to 21 May 2022, we distributed a web-based survey to medical students and physicians in Syria using social media apps (WhatsApp, Facebook, and Messenger) and email. This research was a cross-sectional study. Based on prior research conducted in Pakistan, a questionnaire was translated from the Pakistani language to Arabic, developed, investigated, and confirmed to be accurate for Syrian students and doctors (Ahmed et al., 2022). Responses were anonymous without any identifying data, and only the principal investigator had access to the data. A convenience sampling technique was used to pick the sample population. We conducted an experimental survey on 30 participants before distributing the questionnaire to test the usability and technical performance of the online survey. After that, the questionnaire was tested on 50 people as part of a pilot study to confirm its validity and reliability. The tool's internal consistency of the used sub-scales was shown by Cronbach's alpha values, ranging from 0.7 to 0.8 (Knowledge = 0.795, practice = 0.702, and Attitude = 0.663). Participants had the option to go back and modify their replies. The duplicate entries were cleaned up and only completed responses were considered. Inclusion criteria were responders being medical students and doctors conducting the study. Exclusion criteria were non-medical responders and an incomplete survey. Calculator.net's sample size estimator, accessible at “https://www.calculator.net/sample-size-calculator.html”, was used to determine the sample size. The population of Syria is expected to reach over 18 million people in 2019, according to statistics from the UN. Based on that, we performed a statistical power analysis to calculate the sample size. The suggested sample size was 385 with a design effect of 50%, a margin of error of 0.05, and a confidence level of 95%. Participants were encouraged to complete the online survey on the Google form, which had 1,538 total answers. The total sample size was 1,484 after 24 people declined to complete the questionnaire.
The questionnaire consists of age, gender, qualification level, rank, and university year for the undergraduate participants.
This sub-scale has seven questions about the general knowledge of AI, including knowledge of artificial intelligence machine learning, AI in the medical field, AI in radiology, AI in pathology, and AI during the training for post-graduate doctors (for the statistical analysis, yes = 1, no = 0 & Good knowledge is upper than 3 points).
This sub-scale has ten questions about the attitude toward AI, including the necessity of AT in the medical field, training, assessment, diagnosis, radiology, pathology, and its importance during the COVID19 (for the statistical analysis, Don't know, disagree or strongly disagree = 0, agree or strongly agree = 0 & Good attitude is upper than 5 points).
This sub-scale has seven questions about the practice of AI, including if the doctor has inserted the AI in the medical field and the intention of conducting this technique during the training (for the statistical analysis, yes = 1, No, never applied = 0 & Good practice is upper than 2 points).
All participants could withdraw from the cross-sectional research at any time, and participation was completely voluntary. The participant could not be identified since the study did not provide names or emails. Each participant's identity was therefore wholly protected during the investigation. The University of Aleppo's ethics committee granted its permission and gave the research the go-ahead, and the Helsinki Declaration carried out the study.
The data was analyzed using SPSS version 25.0. The frequencies of different variables were shown using frequency tables. The internal consistency of the scale was determined using Cronbach's α coefficient. The Chi-square test was used to examine the statistical correlation between the categorical variables. A p-value of less than 0.05 indicated that the association was significant. Mann-Whitney and Kruskal-Wallis tests were used depending on the data normality. In addition, univariate logistic regression was carried out to predict the outcome measurements of artificial intelligence, such as the knowledge, attitude, and practice from the baseline characteristics of the study population. The unadjusted odds ratios and their respective 95% confidence intervals were used in the regression.
As shown in the baseline characteristics of the study population in Table 1, there are 255 (16.9%) who are a graduate or postgraduate master's or Ph.D. doctors, while medical students resemble about 1,252 (83.1%) of the total participants, most of them aged between 21 and 30 years, 1,452 (97.2%). Most participants were male, Gender 779 (52%), and most undergraduate participants were from the sixth year of medical school 476 (31.6).
As shown in Table 2, the mean score of Knowledge of AI was 1.82 ± 1.83. Regarding Knowledge of AI, individuals were questioned about the basic concept of AI, its subtypes, i.e., machine learning (ML) and deep learning (DL), and its applications. It was observed that 1,055 (70%) had a basic concept of AI, but only 523 (34.7%) had Knowledge about ML and DL, and only 357 (23.7%) had Knowledge about its applications. In contrast, 452 (30%) individuals had no knowledge about the basic concept of AI, 984 (65.3%) had no knowledge about ML and DL, and 1,150 (65.3%) were unaware of any application of AI in the medical field. Only 320 (21.2%) individuals were aware of the application of AI in radiology, and only 261 (17.3%) knew about AI application in pathology.
There were significant differences in the knowledge score as a continuous dependent variable about Gender (P < 0.001) and qualification level (P < 0.001), in which the females and the graduate doctors have higher knowledge than others, but not for the age groups (P = 0.297), as shown in Table 3.
Table 4 demonstrates how Knowledge of AI can differ depending on different baseline variables. Qualification level, undergraduate year, graduate current status, and postgraduate rank significantly differs in the proportion of good Knowledge.
Table 4. Knowledge of AI based on gender, age, qualification level, professional, current status and rank of the doctors.
The proportion of good Knowledge was 183 (12.1%) compared to 65 (4.4%) among the undergraduate and graduate participants. Undergraduate fifth-year medical students were the most who had an excellent knowledge 56 (3.7%). Compared to the residents and other categories, graduate student participants had a higher proportion of good knowledge 188 (12.5%), and the same for postgraduate students compared to professors and different types 188 (12.5%).
The prediction of adequate Knowledge of AI among the study sample depending on the demographic variables is given in Table 5. It was observed that the level of undergraduate medical students' 4th and 5th years was the only significant factor affecting their Knowledge of AI. The undergraduate students had 3.327 times more adequate knowledge of AI than students in the first year.
Table 5. Binary logistic regression between baseline characteristics of the study population and the knowledge of artificial intelligence*.
The mean score of attitudes toward AI was 6.03 ± 2.16. Regarding the attitude toward AI in the health sector, individuals were questioned about the necessity of AI in the medical field, 689 (45.7%) individuals strongly agreed, and 628 (41.7%) agreed. Regarding the opinion that AI aids practitioners in early diagnosis and assessment of disease severity, 558 (37%) strongly agree, and 690 (45.8%) agree. The idea that AI can replace the physician in the future, 127 (8.4%) strongly agree, and 97 (13.1%) agree. Individuals believe that AI is essential in radiology and pathology, as 445 (29.5) and 396 (26.3%) strongly agree. Out of all, 661 (43.9%) agree that introducing AI is essential in the current COVID 19 pandemic. While 684 (45.4%) disagree that AI would be a burden for practitioners regardless of the pandemic. Regarding the budget allocated for AI to be used in the current COVID 19 pandemics, most individuals agree 648 (43%). Most individuals disagree that AI would increase the percentage of errors in diagnosis 496 (32.9%), as shown in Table 6.
There were significant differences in the attitude score as a continuous dependent variable about Gender (P < 0.001), qualification level (P = 0.003), and age groups (P < 0.001), which the males, the undergraduate, and 21–30 years old individuals have a higher attitude score than others, as shown in Table 3.
Table 7 demonstrates how attitudes toward AI can differ depending on baseline variables. Age groups, Gender, and undergraduate year significantly differ in the proportion of positive attitudes. Undergraduate sixth-year medical students were the most who had a positive attitude 398 (26.4%). More males, 564 (37.4%) had a positive attitude than 463 (30.7%) females. Individuals aged 21–30 had the highest proportion of positive attitudes compared to 1,007 (66.8%) in other age categories.
Table 7. Attitude toward AI based on gender, age, qualification level, professional, current status and rank of the doctor.
The prediction of attitude toward AI among the study sample depending on the demographic variables is given in Table 8. It was observed that the level of undergraduate medical student's 6th year was the only significant factor affecting the attitude toward AI, with P-values of less than 0.05. The undergraduate 6th-year students had 2.868 times the attitude toward AI higher than students in the first year. But all other variables were insignificant.
Table 8. Binary logistic regression between baseline characteristics of the study population and the attitude toward artificial intelligence**.
The mean score of the practice of AI was 1.90 ± 1.06. Regarding practice of AI, 1,346 (89.3%) had never applied AI in any task of their work, while only 161 (10.6%) had ever practically applied AI. Of 161 who used AI, 95 (60%) declared it easy to apply AI. As expected, most individuals agree that the physician role is essential in the application and evaluation of AI in the medical field, 699 (46.4%). Concerning the practice in the future, 1,184 (78.6%) individuals were ready to apply AI in the future, and 274 (18.2) individuals did not have any opinion on whether or not they would work with AI in the future, As shown in Table 9.
There were significant differences in the practice score as a continuous dependent variable regarding qualification level (P < 0.001) and the age groups (P = 0.004), in which the graduate doctors and 41–50-year-old have higher practice score more than others, as shown in Table 3.
Table 10 demonstrates how the practice of AI can differ depending on different baseline variables. Age groups, qualification level, undergraduate year, graduate current status, and postgraduate rank significantly differs in the proportion of good practice except for gender. Individuals aged 21–30 had the highest proportion of good practice compared to other age categories, 207 (13.7%). The undergraduate (9.8%) had a higher proportion of good practice than the postgraduate (5.1%). The 6th-year medical student had the highest proportion of good practice (3%). Graduates considered a student had a higher proportion of good practice than residents and other graduate categories, 152 (10.1). Postgraduate rank regarded as a student had a higher proportion of good practice than a professor and different postgraduate rank categories, 152 (10.1).
Table 10. Practice of AI based on gender, age, qualification level, professional, current status and rank of the doctors.
The prediction of the current practice of AI among the study sample depending on the demographic variables is given in Table 11. It was observed that only the postgraduate rank, specifically the resident and assistant professors, were significant factors affecting the practice of AI with p-values < 0.05. The residents and assistant professors had 2.371 and 4.422 times the training of AI higher than students, respectively.
Table 11. Binary logistic regression between baseline characteristics of the study population and the Practice of artificial intelligence***.
The term “artificial intelligence” (AI) refers to the subfield of computer science that focuses on developing computer programs that can carry out activities that would normally need human intellect (Wahl et al., 2018; He et al., 2019). Due to the fast development of Artificial intelligence in healthcare, it has overcome many problems that arise while delivering medical services (Amisha Malik et al., 2019). Study participants' KAP (Knowledge, Attitude, and Practice) was the focus of this research. In our study, we had 1,494 participants (undergraduate, graduate, and postgraduate medical and dental participants), of which 52% were males. Our findings show that 70% have basic knowledge of AI, 23.7% know AI applications in Medicine, and 34.7% have sufficient knowledge about ML and DL, clearly showing insufficient medical integration knowledge of AI among Syrian participants. Although a thorough knowledge of artificial intelligence is not well observed in the study population, 87.4% of participants recommend its use and find it essential in Medicine. Also, findings report that 68.1% of the Study population showed an important acknowledgment of AI use in the COVID-19 pandemic situation; this percentage is relatively similar and consistent with other studies conducted in Pakistan (74.4%) and the UK (78%), where students acknowledged the essential role of AI in the field of Medicine. Foremother 73.3% of the study population deeply acknowledged the use of AI in radiology which is also committed to the study conducted in Pakistan, where (56.7%) of students and (74%) of doctors considered AI very important in radiology (Sit et al., 2020; Ahmed et al., 2022).
For additional comparing our findings with the results of other studies conducted in many countries and different medical specializations we observed the following: there are connections with a Singaporean study where 67.2% of respondents were keen to get involved in an AI/ML research project; also, in our study, 78.6% of individuals were ready to apply AI in the future. A National Survey Study in Columbia showed that only a minority (29.3%) of respondents agreed AI would replace radiologists in the foreseeable future, and this is consistent with our results, where just (8.4%) strongly agreed, and 97 (13.1%) agreed that AI could replace the physician in the future. In contrast to a Turkish poll, which revealed that just 48.40% of participants had a basic understanding of AI technology, our survey found that (70%) of respondents did. Additionally, there were no links to a German online poll that revealed 52% of respondents were aware of the current debate concerning AI in radiology, but in our research, just 21.2% of participants were aware of this topic. Regarding knowledge of AI and its branches, a cross-sectional study in Ireland showed that 43.4% of participants had not heard of the term ‘machine learning; on the other hand, 65.3% did not know about machine learning in our survey.
In addition, it is worrying that in our study, 21.5% of the study population believe that AI might replace physicians in the future, in contrast to the results found in the Pakistan study, where most participant medical students consider AI as a beneficial healthcare worker aid rather than a potential alternative to physicians, this percentage found in our study is probably caused by misunderstood of AI implications and limitations. Concerning AI incorporation in the medical school curriculum, most participants highly acknowledged the inclusion of the AI curriculum in medical graduate and undergraduate studies, which is also clearly consonant with results found in UK and Pakistan conducted studies (Oliveira et al., 2021; Ahmed et al., 2022).
Overall, results show a relatively moderate basic knowledge of the Syrian participants about artificial intelligence and acceptance of its curriculum inclusion in medical school. Also, Syrian participants showed satisfactory perception and attitude toward AI use in radiology and its importance in diagnosis and healthcare practice. Subtypes, deep AI and its practical implications knowledge seem to be insufficient; this is justified by a traditional curriculum based medical studies with lack or even absence of AI education, its non-integration in medical studies leading to doctors AI disinterest; also, students who did receive AI training are likely more understanding and aware of its limitations that preclude replacement of radiologists, it is also essential to note the significant impact of the Syrian crisis on the educational system and self-learning.
As new science and proper strategic method, AI requires relatively extensive training for physicians, well-established rules and practice ethics, payment restrictions for public or private organizations, and constant upgrades over time, which explains its limited usage globally and current absence in underdeveloped countries such as Syria (Aburas et al., 2018; He et al., 2019; Paranjape et al., 2019). Therefore, we recommend offering training programs, seminars, and webinars about AI, ML, and DL for Syrian health care workers. Also relevant in this study were clinical consequences, knowledge and career ambitions in radiology, and the perspective of AI as a complete replacement for doctors in the future. The findings led us to identify significant deficiencies that need to be addressed, and this necessitates the incorporation, expansion, and enhancement of artificial intelligence (AI) training in medical schools to get rid of erroneous and misleading attitudes and encourage Syrian medical professionals to work in this sector; a basic progression may be achieved by including training on electronic health records (EHRs) into the medical curriculum; in addition, AI training could be provided via continuing medical education (CME) programs. Finally, it would seem that the most difficult aspect of implementing AI in healthcare domains is not determining whether or not the technologies will be competent enough to be beneficial; rather, the most difficult aspect is assuring that the technologies will be used in everyday clinical practice (Davenport and Kalakota, 2019; He et al., 2019).
Despite its affordability and usefulness, a cross-sectional research design cannot establish causality at this time. Additionally, by using global sampling and reaching a response rate of 99 %, which is greater than the typical response rate for organizational research surveys, the generalization of this study was improved. Because surveys were anonymous, there was no way to get in touch with respondents after completing the forms to check for unusual responses. Several steps were taken to increase the study's dependability with these limitations. To increase the internal validity of study results, use a validated instrument in addition to controlling for potential confounders in the final model and sample from various research locations. A priori sample size calculations are also performed to ensure the study is robust. Furthermore, the age range of 21 to 30 attracted most participants, leaving the other age groups with little information.
It is of the utmost importance that immediate steps be taken to enhance the existing condition of the medical professionals and undergraduate medical students in Syria to raise their level of knowledge and influence their attitudes toward using artificial intelligence in medicine. A significant proportion of the respondents said they do not use artificial intelligence in their professional lives. Other than that, many people have a favorable attitude toward its utilization in the medical profession owing to its advantages in enhancing the diagnostic process and how patients are treated. Training medical professionals in Syria to utilize artificial intelligence in their work should be obligatory, and training should occur either online or in person. The Syrian government and international organizations should work together to assist the country's medical professionals.
1- Rama mazen Reslan: Faculty of medicine, Tishreen University, Latakia, Syria.
2- Nagham Ali Al Hammadi: Faculty of Medicine, Tishreen University, Latakia, Syria.
3- Ahmed Zaher Radwan Radwan: Faculty of medicine, Aleppo University, Aleppo, Syria.
4- Temaa Alkilani: faculty of medicine, Damascus university, Damascus, Syria.
5- Enas Farhan Azzam: Faculty of Medicine, Al-Furat University, Der alzoor, Syria.
6- Fouad Hasib Makhoul: Faculty of Medicine, Al Baath University, Homos, Syria.
7- Ghosoun khazma: Faculty of medicine, University of Kalamoon, Rif dimashq, Syria.
The original contributions presented in the study are included in the article/Supplementary material, further inquiries can be directed to the corresponding author.
The studies involving human participants were reviewed and approved by all participants could withdraw from the cross-sectional research at any time, and participation was completely voluntary. The participant could not be identified since the study did not provide names or emails. Each participant's identity was therefore wholly protected during the investigation. The University of Aleppo's Ethics Committee granted its permission and gave the research the go-ahead, and the Helsinki Declaration carried out the study. The patients/participants provided their written informed consent to participate in this study. Written informed consent was obtained from the individual(s) for the publication of any potentially identifiable images or data included in this article.
SSw: conceptualization, data curation, methodology, formal analysis, writing-original draft, and review and editing. HA, NE, MN, MR, AN, BS, MA, EB, LE, SA, EA, ZO, AAls, and SSh: writing—review and final editing. All authors read and approved the final manuscript.
The authors declare that the research was conducted in the absence of any commercial or financial relationships that could be construed as a potential conflict of interest.
All claims expressed in this article are solely those of the authors and do not necessarily represent those of their affiliated organizations, or those of the publisher, the editors and the reviewers. Any product that may be evaluated in this article, or claim that may be made by its manufacturer, is not guaranteed or endorsed by the publisher.
The Supplementary Material for this article can be found online at: https://www.frontiersin.org/articles/10.3389/frai.2022.1011524/full#supplementary-material
Aburas, R., Najeeb, A., Baagel, L., and Mackey, T. K. (2018). The Syrian conflict: a case study of the challenges and acute need for medical humanitarian operations for women and children internally displaced persons. BMC Med. 16, 65. doi: 10.1186/s12916-018-1041-7
Ahmed, Z., Bhinder, K. K., Tariq, A., Tahir, M. J., Mehmood, Q., Tabassum, M. S., et al. (2022). Knowledge, attitude, and practice of artificial intelligence among doctors and medical students in Pakistan: a cross-sectional online survey. Ann. Med. Surg. (Lond). 76, 103493. doi: 10.1016/j.amsu.2022.103493
Alami, H., Rivard, L., Lehoux, P., Hoffmann, S. J., Caddeddu, S. B. M., Savoldelli, M., et al. (2020). Artificial intelligence in health care: laying the Foundation for Responsible, sustainable, and inclusive innovation in low- and middle-income countries. Global Health 16, 52. doi: 10.1186/s12992-020-00584-1
Amisha Malik, P., Pathania, M., and Rathaur, V. K. (2019) Overview of Artificial Intelligence in Medicine. J. Family Med. Prim. Care, 8, 2328–2331. doi: 10.4103/jfmpc.jfmpc_440_19
Bonderman, D. (2017). Artificial intelligence in cardiology. Wien Klin Wochenschr. 129, 866–868. doi: 10.1007/s00508-017-1275-y
Chan, K. S., and Zary, N. (2019). Applications and challenges of implementing artificial intelligence in medical education: integrative review. JMIR Med. Educ. 5, e13930. doi: 10.2196/13930
Davenport, T., and Kalakota, R. (2019). The potential for artificial intelligence in healthcare. Future Healthc. J. 6, 94–98. doi: 10.7861/futurehosp.6-2-94
Hashimoto, D. A., Witkowski, E., Gao, L., Meireles, O., and Rosman, G. (2020). Artificial intelligence in anesthesiology: current techniques, clinical applications, and limitations. Anesthesiology 132, 379–394. doi: 10.1097/ALN.0000000000002960
He, J., Baxter, S. L., Xu, J., Xu, J., Zhou, X., and Zhang, X. (2019). The practical implementation of artificial intelligence technologies in medicine. Nat. Med. 25, 30–36. doi: 10.1038/s41591-018-0307-0
Hessler, G., and Baringhaus, K. H. (2018). Artificial intelligence in drug design. Molecules 23, 2520 doi: 10.3390/molecules23102520
Niazi, M. K. K., Parwani, A. V., and Gurcan, M. N. (2019). Digital pathology and artificial intelligence. Lancet Oncol 20, e253–e261. doi: 10.1016/S1470-2045(19)30154-8
Niel, O., and Bastard, P. (2019). Artificial intelligence in nephrology: core concepts, clinical applications, and perspectives. Am. J. Kidney Dis. 74, 803–810. doi: 10.1053/j.ajkd.2019.05.020
Oliveira, G., Teixeira, J. G., Torres, A., and Morais, C. (2021) An exploratory study on the emergency remote education experience of higher education students teachers during the COVID-19 pandemic. Br. J. Educ. Technol. 52, 1357–1376. doi: 10.1111/bjet.13112
Paranjape, K., et al. (2019) Introducing artificial intelligence training in medical education. JMIR Med. Educ. 5, e16048. doi: 10.2196/16048.
Patel, V. L., Shortliffe, E. H., Stefanelli, M., Szolovits, P., Berthold, M. R., Bellazzi, R., et al. (2009). The coming of age of artificial intelligence in medicine. Artif. Intell. Med. 46, 5–17. doi: 10.1016/j.artmed.2008.07.017
Rabaan, A. A., Alhumaid, S., Mutair, A. A., Garout, M., Abulhameyel, M., Halwani, M. A., et al. (2022). Application of artificial intelligence in combating high antimicrobial resistance rates. Antibiotics (Basel) 11, 784. doi: 10.3390/antibiotics11060784
Sit, C., Srinivasan, R., Amlani, A., Muthuswamy, K., Azam, A., Monzon, L., et al. (2020), Attitudes and perceptions of UK medical students towards artificial intelligence radiology: a multicentre survey. Insights Imaging 11, 14. doi: 10.1186/s13244-019-0830-7.
Souza Filho, E. M., Fernanadas, F. A., Pereira, N. C. A., Mesquita, C. T., and Gismoundi, R. A. (2020). Ethics, artificial intelligence and cardiology. Arq. Bras. Cardiol. 115, 579–583. doi: 10.36660/abc.20200143
Thrall, J. H., Li, X., Li, Q., Cruz, C., Do, S., Dreyer, K., et al. (2018). Artificial intelligence and machine learning in radiology: opportunities, challenges, pitfalls, and criteria for success. J. Am. Coll. Radiol. 15(3 Pt B), 504–508. doi: 10.1016/j.jacr.2017.12.026
Triantafyllidis, A. K., and Tsanas, A. (2019). Applications of machine learning in real-life digital health interventions: review of the literature. J. Med. Internet Res. 21, e12286. doi: 10.2196/12286
Wahl, B., Cossy-Gantner, A., Germann, S., and Schwalbe, N. R. (2018). Artificial intelligence (AI) and global health: how can AI contribute to health in resource-poor settings? BMJ Glob. Health 3, e000798. doi: 10.1136/bmjgh-2018-000798
Wang, R., Pei, W., Jin, L., Li, Y., Geng, Y., Gao, C., et al. (2019). Artificial intelligence in reproductive medicine. Reproduction 158, r139–r154. doi: 10.1530/REP-18-0523
WHO (2017). Big Data and Artificial Intelligence for Achieving Universal Health Coverage: An International Consultation on Ethics. World health Organization.
Keywords: knowledge, attitude, practice, artificial intelligence, medical student, doctor, Syria
Citation: Swed S, Alibrahim H, Elkalagi NKH, Nasif MN, Rais MA, Nashwan AJ, Aljabali A, Elsayed M, Sawaf B, Albuni MK, Battikh E, Elsharif LAM, Ahmed SMA, Ahmed EMS, Othman ZA, Alsaleh A and Shoib S (2022) Knowledge, attitude, and practice of artificial intelligence among doctors and medical students in Syria: A cross-sectional online survey. Front. Artif. Intell. 5:1011524. doi: 10.3389/frai.2022.1011524
Received: 04 August 2022; Accepted: 12 September 2022;
Published: 29 September 2022.
Edited by:
Kathiravan Srinivasan, Vellore Institute of Technology, Vellore, IndiaReviewed by:
Balakrishnan S, Sri Krishna College of Engineering & Technology, IndiaCopyright © 2022 Swed, Alibrahim, Elkalagi, Nasif, Rais, Nashwan, Aljabali, Elsayed, Sawaf, Albuni, Battikh, Elsharif, Ahmed, Ahmed, Othman, Alsaleh and Shoib. This is an open-access article distributed under the terms of the Creative Commons Attribution License (CC BY). The use, distribution or reproduction in other forums is permitted, provided the original author(s) and the copyright owner(s) are credited and that the original publication in this journal is cited, in accordance with accepted academic practice. No use, distribution or reproduction is permitted which does not comply with these terms.
*Correspondence: Abdulqadir J. Nashwan, YW5hc2h3YW5AaGFtYWQucWE=
Disclaimer: All claims expressed in this article are solely those of the authors and do not necessarily represent those of their affiliated organizations, or those of the publisher, the editors and the reviewers. Any product that may be evaluated in this article or claim that may be made by its manufacturer is not guaranteed or endorsed by the publisher.
Research integrity at Frontiers
Learn more about the work of our research integrity team to safeguard the quality of each article we publish.