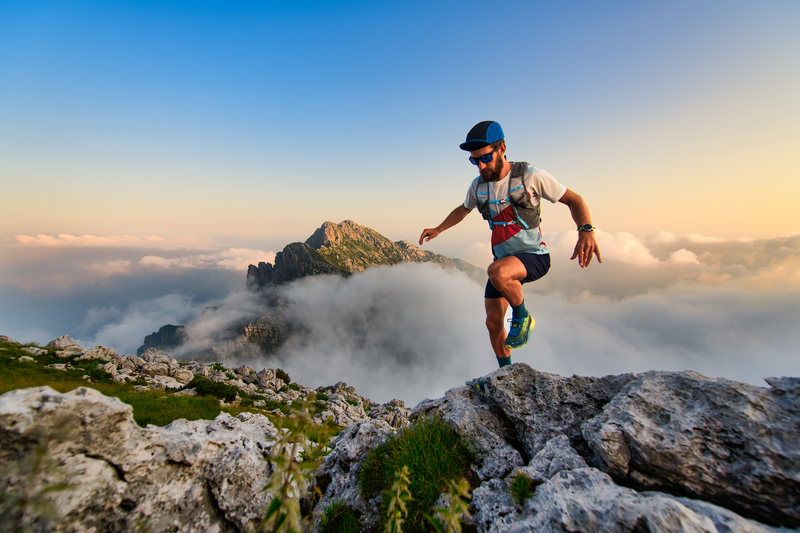
94% of researchers rate our articles as excellent or good
Learn more about the work of our research integrity team to safeguard the quality of each article we publish.
Find out more
SPECIALTY GRAND CHALLENGE article
Front. Arachn. Sci. , 20 June 2023
Sec. Arachnid Microbiota and Diseases
Volume 2 - 2023 | https://doi.org/10.3389/frchs.2023.1215831
Arachnids cause human and animal afflictions. These range from poisoning, caused mainly by spiders (Lüddecke et al., 2022) and scorpions (Abroug et al., 2020), but also by ticks (Cabezas-Cruz and Valdés, 2014), to infectious diseases transmitted mainly by ticks (de la Fuente et al., 2017) and also by some mites such as Dermanyssus gallinae (Schiavone et al., 2022). Furthermore, mites can parasitize insects, with Varroa destructor, which affects honeybees, being a notable example of parasitism in Arachnida (Vilarem et al., 2021). Several arachnid species thus highlight the importance of this group in terms of economic loss and burden on animal and human health. It is inconceivable to speak today about the impact of vector-borne diseases without evoking Borrelia pathogens causing Lyme disease or relapsing fever and vectored by hard or soft ticks (Kurokawa et al., 2020; Faccini-Martínez et al., 2022), or referring to the global decline of honeybees that places Varroa mites (Vilarem et al., 2021) at the centre of the debate. Recently, a new condition called alpha-gal syndrome has emerged, which is a type of allergy to red meat triggered by bites from ticks like Amblyomma americanum or Ixodes ricinus, among others (Cabezas-Cruz et al., 2019; Román-Carrasco et al., 2021; Cabezas-Cruz et al., 2021). This discovery has expanded our knowledge about diseases transmitted by arachnids and has also posed challenges to our current understanding of allergies in general (Cabezas-Cruz et al., 2019; Román-Carrasco et al., 2021; Cabezas-Cruz et al., 2021). The study of arachnids today encompasses various research areas that contribute to our understanding of “infectious diseases,” “vectors,” “allergy,” “venoms,” and “microbiota,” among other topics.
Beyond the importance of vector-borne pathogens for human and animal health, studies on tick–pathogen interactions have shed light on the close relationships from which both vector and pathogen benefit (Cabezas-Cruz et al., 2017). Vector saliva assists pathogen transmission through specific molecular mechanisms (Ramamoorthi et al., 2005), while pathogens induce the expression of tick proteins (Neelakanta et al., 2010) that potentially increase vector survival in the environment (Cabezas-Cruz et al., 2017). This makes pathogen transmission a relevant evolutionary trait rather than a by-product of hematophagy. Departing from the existing paradigms in various fields of study within arachnid science and embracing arachnid biology and evolution as a whole can bring interesting, original, and unexpected insights that push forward our understanding of animal biology in general. For example, on one hand, a recent study challenged the concept of venom sterility, indicating the presence of bacterial pathogens in the venom of some spiders (Esmaeilishirazifard et al., 2022). On the other hand, the saliva of V. destructor, which is also a vector of pathogens affecting bees (Richards et al., 2011; Becchimanzi et al., 2020), contains proteins found in tick saliva and other venomous animals (Cabezas-Cruz and Valdés, 2014). Proteins in V. destructor saliva influence host immunity, preventing host infection by opportunistic pathogens (Becchimanzi et al., 2020). These studies suggest an intriguing ancestral evolutionary association between arachnids, their venom or saliva [tick saliva can be considered a special type of venom (Cabezas-Cruz and Valdés, 2014)], and the pathogens affecting their hosts. “Arachnid Microbiota and Diseases”, a specialty within Frontiers in Arachnid Science, creates an unrivalled opportunity to spark debate and creates a forum for studies in which knowledge from vector models (e.g., I. ricinus) can be transferred to other arachnids in which vector competence is still controversial (e.g., D. gallinae; Schiavone et al., 2022). Simultaneously, comparative studies can bring a bird’s eye view to the evolution of pathogen transmission or other complex traits across arachnids.
Certainly, more exciting discoveries are yet to come.
Recent technological developments in DNA sequencing (Malla et al., 2019; Yen and Johnson, 2021) have enabled “the microbiome revolution” (Blaser, 2014), a reformulation of scientific questions to include the microbiota as an essential player in biological phenomena, from animal and plant physiology to disease. The concept of species as a group of individuals sharing well-defined and unique properties is being challenged by the evidence-rich realization that all multicellular animals, and many unicellular organisms too (e.g., protozoans, Denoncourt et al., 2014), are inhabited by and/or associated with more than thousands (in some animals, trillions) of microorganisms (but see, Hammer et al., 2019) that influence every aspect of their biology, from metabolic health (Fan and Pedersen, 2021) to resistance (Ford and King, 2016) and susceptibility (Stevens et al., 2021) to pathogen infection. We can no longer rely simply on genetic definitions and germline-borne transmission of genes as the unique or key features to explain “typical” phenotypes carried and expressed by species, as the contribution of commensals and/or symbionts to almost all animal phenotypes permeate every attempt to study isolated traits within any given species.
Microbiota dynamics are relevant to arachnid biology and disease ecology. For example, studies have demonstrated that manipulation of microbiota decreased reproductive fitness in ticks such as Dermacentor andersoni (Clayton et al., 2015) and A. americanum (Zhong et al., 2007), as well as in the spider mite Tetranychus truncatus (Zhu et al., 2019). Furthermore, empirical studies under controlled laboratory conditions have shown that tick microbiota dysbiosis can cause a reduction in Borrelia burgdorferi colonization in Ixodes scapularis (Narasimhan et al., 2014) and higher transstadial transmission of Babesia microti in Haemaphysalis longicornis (Wei et al., 2021). Microbiota–pathogen interactions are bidirectional and, not only can dysbiosis affect pathogen life cycles, but infection can also modulate the vector microbiota (Abraham et al., 2017). Thus, mounting evidence suggests that the contribution of the tick microbiota to vector physiology and pathogen life cycle are so relevant that vectorial capacity or competence cannot be understood without considering the microbial communities associated to these vectors (Wu-Chuang et al., 2021; Maitre et al., 2022). The impact of the microbiota is not only relevant to individuals and populations, but it is also detected at the macroevolutionary scale, and evidence of phylosymbiosis has been documented in ticks (Díaz-Sánchez et al., 2019) and spiders (Dunaj et al., 2020), facilitating the merging of genetic and microbiological traits into conceptual unifications such as hologenomes (Díaz-Sánchez et al., 2019; Price et al., 2021) and holobionts (Díaz-Sánchez et al., 2019; Armstrong et al., 2022).
“Arachnid Microbiota and Diseases”, along with its sister sections “Arachnid Ecology and Behavior” (see, Schausberger, 2022), “Arachnid Diversity, Conservation and Biogeography” (see, Agnarsson, 2023), and “Arachnid Morphology, Systematics and Evolution”, makes Frontiers in Arachnid Science (see Kuntner, 2022) an exciting opportunity for scholars interested in bringing forward new transformative insights or incremental evidence of “old truths” to submit their scientific work in these fields.
We aspire to see the study of arachnid microbiota reach the same level of advancement as research on other organisms, and this field can greatly benefit from further exploration of microbiota diversity and functionality in Arachnida. Great challenges lie ahead, and we would like “Arachnid Microbiota and Diseases” to be a major player in shaping the future developments in the field. Here, we summarized what we consider are the two major challenges in articulating research programs that include the microbiota and its interplay with the biology and ecology of arachnids, and the diseases transmitted by them. These challenges are: (i) The Ontological Challenge, and (ii) the Epistemological Challenge. The first challenge refers to the knowledge of “what is what?” What concepts, categories, names, and definitions are the scientific community assigning to their subjects and objects of study? The second challenge concerns the preconditions and goals of knowledge, as well as how the knowledge on arachnid microbiota and diseases is generated (e.g., the methodologies available to study the subject).
Language is an evolving cultural production. Scientists, in their endeavor to describe complex phenomena for which words may not yet have been invented, must create their own language (and, it seems, enjoy doing so). Marchesi and Ravel proposed in their paper (Marchesi and Ravel, 2015) that “microbiota” was first coined by Lederberg and McCray in 2001 (Lederberg and Mccray, 2001) but the use of this word, based on Google records, dates back to 1928. Microbiota can be simply defined as “the assemblage of living microorganisms present in a defined environment” (Marchesi and Ravel, 2015). However, in contrast to other definitions in biology [e.g., that of “deoxyribonucleic acid” (DNA)] that describe a biochemical entity with a stable and well-defined chemical structure, the complexity that is intended to be defined with the word “microbiota” remains elusive and keeps resisting containment within an undisputed concept (Berg et al., 2020). For example, the word “microorganisms” includes bacteria, fungi, viruses, bacteriophages, and protozoans, and, accordingly, many papers use an expanded definition of microbiota as “the assemblage of bacteria, fungi, viruses, and protozoans present in a defined environment”. However, some argue that as viruses are not considered as living microorganisms (Dupré and O’Malley, 2009), they should not be included in the definition of “microbiota”. Another issue in this debate, for example, is that the most used technique to study the presence of bacteria and archaea in the ‘microbiota’ is 16S rRNA amplicon sequencing (see The Epistemological Challenge), which does not limit the detection to living microorganisms. This, if we stick to the definition above, renders 16S rRNA amplicon sequencing results as insufficient for strictly describing the “microbiota”.
Furthermore, within “microorganisms” there are “commensals”, “pathogens”, and “symbionts”, which are not interchangeable terms and are involved in well-defined interactions with their host (e.g., mites, ticks, or spiders) or environment (e.g., soil). Thus, placing “pathogens” [strictly speaking, “a pathogen is defined as an organism causing disease to its host, with the severity of the disease symptoms referred to as virulence” (Balloux and van Dorp, 2017)] within the concept of “microbiota” is confusing and misleading. A pathogen causes infection, and, while the microbiota can facilitate pathogen infection (see, Stevens et al., 2021), in principle, microbes in the microbiota should not cause disease directly as a pathogen does. To distinguish between “microbiota” and “microbiota + pathogens”, concepts such as “pathobiota” [i.e., microbes with potential pathogenic behavior (Bartoli et al., 2018)] and “pathobiome” [i.e., both healthcare-acquired and healthcare-adapted pathobiota (Defazio et al., 2014; Alverdy and Krezalek, 2017)] have been proposed.
An additional layer of confusion arises specifically in Arachnida. On one hand, non-pathogenic (i.e., non-pathogenic for humans or domestic animals) microbes found in ticks can be classified as commensals, facultative symbionts, or obligate symbionts. Frequently in the literature, the three types of non-pathogenic microbes are referred to as being part of the tick microbiota, and, subsequently, it is mentioned that the microbiota composition is under the influence of several factors including physiological stress from environmental traits, consumed blood, host species, tick immunity, and developmental stage (Wu-Chuang et al., 2021). However, an “obligate symbiont” within the microbiota composition (in the sense of the presence/absence of taxa) cannot be under the regular influence of the factors above because by definition, if an “obligate symbiont” is removed, the symbiont and host die or are significantly harmed (Bennett and Moran, 2015; Duron et al., 2018), unless the symbiont is replaced (Binetruy et al., 2020). On the other hand, tick-borne pathogens (i.e., pathogenic for humans or domestic animals) can be facultative symbionts for ticks (e.g., Anaplasma phagocytophilum; Neelakanta et al., 2010), which erodes potential notions of tick microbiota as including exclusively “non-pathogenic” microbes.
Altogether, the mélange of definitions colliding within the concept of “microbiota” creates difficulty in reaching an undisputed concept of “microbiota” and, more importantly, a concept that can be used unambiguously by all members of the scientific community. This challenge becomes greater when considering the potential definitions given to the “microbiota” by the lay public. Despite these definitions being important, as they represent public perceptions of this scientific field, they are not considered here.
As these conceptual distinctions are important for increasing the precision of research, a solution to this conundrum is needed. We propose one solution taken from linguistics, namely grammatology (Derrida, 1998). Considering the impossibility to assign a clear (i.e., unambiguous) meaning to the word ‘microbiota’, we propose the use of microbiota sous rature [in the sense used by the philosophers Martin Heidegger and Jacques Derrida (Derrida, 1998)] instead of “microbiota”. According to Heidegger, sous rature (under erasure) expresses the problem of the presence and absence of meaning in language. Heidegger put words “under erasure”, simultaneously recognizing and questioning the meaning of the term and its accepted use (Derrida, 1998). A word sous rature is “insufficient but necessary” (Derrida, 1998). Sous rature means that a particular signifier (e.g., a word) is not fully adapted to the concept it represents, but must be used because the constraints of our language offer nothing better (Derrida, 1998). Thus, the word microbiota hereafter designates a complex arrangement of concepts for which a meaning shall be defined and specified in each study as clearly as possible.
Epistemology is concerned with the preconditions and goals of knowledge, as well as with how knowledge is generated (Egger and Yu, 2022). What are the preconditions of knowledge in the study of arachnids microbiota? Should knowledge be generated exclusively from data, rendering theory no longer necessary, or shall theory play an important part in interpreting the results from algorithms and data analysis (Egger and Yu, 2022)? What are the paradigms [i.e., “world views representations underlying theories and methodology of a particular scientific field“ (Kuhn, 1962)] shaping theories and methodologies in the field of arachnids microbiota?
Based on the available scientific literature, several paradigms can be proposed. For example, ‘all arachnids have a microbiota’, ‘microbiota improves host fitness’, and ‘the microbiota and its host form an evolutionary unit’. These conceptions, in addition to setting an expectation and having predictive value, also influence the design of our experiments and thus shape the progression of this scientific field. Based on these paradigms, we would design experiments searching further evidence on how microbiota contributes to the survival of arachnids or organismal processes carried by these animals (e.g., molting), or how microbiota provides selective advantages to their host in different ecological or evolutionary contexts. However, these paradigms have been challenged (see, e.g., Moran and Sloan, 2015), and alternative paradigms have been proposed, such as ‘not every animal (which includes arachnids) has a microbiota’ (see, e.g., Hammer et al., 2019), 'presence of microbiota in a host does not equal co-evolution between host and microbiota’ (Moran and Sloan, 2015), and ‘microbiota can reduce host fitness’ (Stevens et al., 2021). This brings us to the question of what is the null hypothesis when designing our microbiota-related experiments. For example, Moran and Sloan (2015) called for a case-by-case analysis in which selection at the level of the symbiotic community should not be accepted as the null hypothesis to explain host–symbiont associations. It would be exciting to see scientific work providing examples of arachnid microbiota interactions that confirm or challenge the above paradigms. Better still, the diversity of arachnids offers the invaluable opportunity to find examples that break with the old established paradigms and open the door to new ones.
Some questions also concern the microbial ecology of arachnid microbiota. What factors shape the composition, diversity, and community assembly of microbiota in arachnids? What are the links between soil microbiota and that found in arachnids that spend extended periods of time in the soil and/or plants? A diverse community of microbes is established anew in each host generation (Coyte et al., 2021). The order [i.e., “priority effect” (Debray et al., 2022)] and identity of incoming microbes are critical in the assembly processes (Coyte et al., 2021). Is the community assembly in arachnids stochastic (Jones et al., 2022), predictable (Coyte et al., 2021), or both (Zhou and Ning, 2017)? Co-dependencies between members of the microbiota contribute to the assembly, as resident bacteria could facilitate the survival of a secondary-acquired microorganism if the two have cooperative interactions, while at the same time co-dependencies create a barrier to assembly (Coyte et al., 2021). In particular, the establishment of new microbes might fail owing to the absence of cooperative bacteria in the host microbiota (Coyte et al., 2021). Ecological theory predicts that hosts can overcome barriers to assembly by promoting uptake of multiple microbes in one step or by feeding key early colonizers (Coyte et al., 2021). It would be interesting to study how different arachnid species contribute to overcoming barriers to community assembly.
Studies on microbiota composition, diversity, and assembly can be carried out using field-collected arachnids (e.g., Zhang et al., 2019), laboratory colonies of arachnid species of interest to test specific hypothesis under controlled conditions (e.g., Abraham et al., 2017), or a combination of both approaches in which field-collected arachnids are placed in the laboratory for experimental manipulation (e.g., Gall et al., 2017). The research can further focus on interactions between arachnid hosts and single microbial species, such as obligate (Zhu et al., 2019; Ogawa et al., 2020) or facultative (Zhu et al., 2018) endosymbionts, or between these arthropods and the diverse microbial community associated with them (Zhu et al., 2019). Owing to the limitations of traditional culture methods for isolating microorganisms, in most cases, experimental designs include one or more “omics” technologies for the detection of one or more microbial biomolecules. The “omics” technologies more frequently used include: (i) amplification of specific regions of genetic markers, usually 16S rRNA genes (to detect bacteria and archaea) or 18S rRNA genes and the intergenic spacer (ITS) region (to detect protozoans or fungi); (ii) shotgun sequencing of microbial genomes (metagenomics) (Wang et al., 2015; Sergaki et al., 2018; Yen and Johnson, 2021); (iii) RNA sequencing for the detection of microbial transcripts (metatranscriptomics) (Wang et al., 2015; Sergaki et al., 2018); (iv) detection of microbial protein fragments (metaproteomics) (Wang et al., 2015; Sergaki et al., 2018); and (v) detection of microbial metabolites (metabolomics) (Wang et al., 2015; Sergaki et al., 2018).
High-throughput sequencing allows for the generation of vast amounts of “omics” data. In recent years, this technology has been combined with artificial intelligence (AI) to explore the connections between microbiota composition and function (Hernández Medina et al., 2022). AI has also been utilized for feature selection and biomarker identification (Marcos-Zambrano et al., 2021), as well as for integrating various types of data, including metagenomics, metatranscriptomics, and metabolomics (Li et al., 2022). For example, Chen et al. (2021) performed a robust prediction of human health status from longitudinal microbiota data using a comprehensive deep-learning-based framework. In another study, Wassan et al. (2019) proposed a novel integrative framework, Phy-PMRFI (phylogeny-aware modeling for prediction of metagenomic functions using random forest feature importance), to identify if phylogenetic relatedness is a good predictor of functional similarity in microbial communities. Inclusion of the phylogenetic measure in Phy-PMRFI maximized the classification of microbiota functions according to properties of taxa (Wassan et al., 2019). However, studies of arachnids microbiota using AI applications are scarce or non-existent in the literature.
Most arachnid microbiota studies available have used 16S rRNA amplicon sequence data to evaluate the taxonomic profiles of bacteria in the microbiota (see e.g., Dunaj et al., 2020; Wu-Chuang et al., 2021; Zheng et al., 2022). Microbiota data are compositional [i.e., describe relationships between components that are not independent and have an arbitrary sum (Quinn et al., 2018)], sparse [i.e., having excessive zero counts (Hu et al., 2018)], and high dimensional [(i.e., with a larger number of features per sample (Hernández Medina et al., 2022)], and, as such, require special treatment. For specifics on how to deal with microbiota data, the reader is referred to other publications (e.g., Chen et al., 2022; Lin and Peddada, 2020; Galloway-Peña and Hanson, 2020). Although a detailed discussion of this issue is out of the scope of the present editorial, we will refer to two examples in which methodological choice has a big impact on data interpretation. First, in many microbiota studies, prokaryotic taxa profiles are delineated using operational taxonomic units (OTUs). However, amplicon sequence variants (ASVs) allow the resolution of rare members of the community, which, among other reasons (Callahan et al., 2017), makes ASVs the ideal unit of marker gene analysis and reporting. Choosing ASVs over OTUs has a significant impact on the taxonomic profiles of the microbiota; as for example, given samples with equal microbial load and diversity, detected species richness may be higher when using ASVs. Second, if not accounted for, the sampling fraction, defined as “the ratio of expected abundance of taxa in a sample to the corresponding absolute abundance in the ecosystem”, may increase the false discovery rate in microbiota studies (Lin and Peddada, 2020). To eliminate any bias owing to differences in the sampling fractions, it is critical to normalize the data (Lin and Peddada, 2020) using rarefying- (Hughes and Hellmann, 2005), scaling- (Lin and Peddada, 2020), or log-ratio-based methods (Lin and Peddada, 2020). The later methods were inspired in Aitchison’s methodology for compositional data (Aitchison, 1982). The normalization method of choice will impact the numbers and sets of differentially abundant taxa (Lin and Peddada, 2020), with some methods outperforming others (see, e.g., Nearing et al., 2022).
The implementation and integration of different “omics” technologies and AI methodologies to further understand arachnid–microbiota interactions as well as the implementation of sound statistical analysis to deal with the complexity of microbiota data are important challenges (Rasmussen et al., 2022). “Arachnid Microbiota and Diseases” encourages submissions on the application of AI in the exploration of arachnids microbiota as well as on the use of metagenomics, metatranscriptomics, metaproteomics, and metabolomics to study the composition and function of microbiota in arachnids.
Although multiomic profiling studies integrating various “omics” technologies enable a comprehensive understanding of complex host–microbiota interactions (see, e.g., Rasmussen et al., 2022), 16S rRNA amplicon sequence data can be used to, in addition to taxonomic profiling, gain insights into the array of functional capabilities of microbial communities (Douglas et al., 2020) and explore complex microbe–microbe interactions using network analysis (Röttjers and Faust, 2018; Kaiser et al., 2022).
Available tools such as PICRUSt, PICRUSt2, Tax4Fun, and FaproTax can be used to infer microbial functional genes from 16S rRNA amplicon sequencing data (Douglas et al., 2020; Sun et al., 2020). In particular, PICRUSt2 has been applied to elucidate the functional profiles of microbiota from various animals (Wu-Chuang et al., 2022a; Aželytė et al., 2023; Corduneanu et al., 2023), including several tick species such as I. scapularis (Wu-Chuang et al., 2022b), I. ricinus (Mateos-Hernández et al., 2020; Mateos-Hernández et al., 2021), Amblyomma variegatum, Amblyomma hebraeum, and Hyalomma truncatum (Chigwada et al., 2022). Predicted metabolic profile analysis using PICRUSt2 revealed a high functional redundancy in the tick microbiota (Estrada-Peña et al., 2020). A limitation of amplicon-based functional predictions is that its accuracy varies across sample types and functional categories (Sun et al., 2020), as inferences are biased toward existing reference genomes and cannot provide resolution to distinguish strain-specific functionalities. Therefore, validation of the PICRUSt2 annotation is recommended. Recently, two studies used PCR to validate the functional predictions of ticks microbiota using PICRUSt2 (Mateos-Hernández et al., 2020; Mateos-Hernández et al., 2021), providing further support for the use of this tool to explore functional profiles in the microbiota of different arachnid species.
The 16S rRNA amplicon sequences can also be used to assess potential interactions between taxa in the microbiota. Network analysis is a powerful tool used in ecology to explore the complexity of microbial communities (Röttjers and Faust, 2018; Guseva et al., 2022). The graphical depiction of networks is a simplified representation of complex microbial systems. In microbial networks, the nodes represent taxa and the edges between them patterns of co-occurrence or correlations as a proxy for interactions (but see, Blanchet et al., 2020). Interestingly, in network analysis, taxa abundance is no longer a key metric for assessing the importance of taxa in a community, as rare microbes (i.e., low-abundance taxa) can have a central role in the network (Guo et al., 2022). Patterns of positive and negative co-occurrence between nodes in microbial networks can also be used to analyze the possible consequences of those interactions on the microbial fitness and metabolic functions of the microbiota (Berg et al., 2020). Furthermore, network centrality metrics (e.g., betweenness centrality and eigenvector centrality) (Newman, 2010) can be used to identify keystone taxa “with a major influence on microbiota structure and function at a particular space or time” (Banerjee et al., 2018). Keystone taxa were predicted in ticks microbiota based on high eigenvector centrality, high abundance, and ubiquity (Mateos-Hernández et al., 2020; Mateos-Hernández et al., 2021; Wu-Chuang et al., 2022c). The importance of keystone taxa in ticks microbiota was recently validated using anti-microbiota vaccines (Mateos-Hernández et al., 2020). Thus, network analysis is an ideal tool to assess complex microbial systems, including those found in arachnids. Network approaches can advance the understanding of microbial ecology in arachnids.
The study of microbiota in arachnids is in its infancy, which provides an opportunity for testing hypotheses already validated in other animal systems and to generate innovative experimental designs to test novel hypotheses. The mélange of concepts covered and colliding in the word “microbiota” requires the use of microbiota (sous rature) to highlight the conditionality of the term, for which a meaning shall be defined and specified in each study as clearly as possible.
Multiomic approaches have several advantages over studies relying solely on 16S rRNA amplicon sequencing for the characterization of the microbiota. However, combining 16S rRNA amplicon sequencing data with sound statistical methods and the integration of diverse data analysis pipelines can shed new light on arachnid–microbiota interactions and the microbial ecology of the multiple microorganisms inhabiting these animals.
Single-point microbiota composition analyses are predominant in the literature, whereas longitudinal microbiota data are rare. Longitudinal studies could help understanding of the dynamics microbiota of composition, taxa abundance, and assembly in arachnids. The application of AI algorithms can help uncover complex patterns in single-point and longitudinal microbiota data.
The author confirms being the sole contributor of this work and has approved it for publication.
I thank Dr. Matjaz Kuntner, Dr. Ingi Agnarsson, Dr. Snædís Huld Björnsdóttir, and the FAS editorial teams for useful suggestions for the improvement of this manuscript.
The author declares that they were an editorial board member of Frontiers at the time of submission. This had no impact on the peer review process and the final decision.
The author declares that the research was conducted in the absence of any commercial or financial relationships that could be construed as a potential conflict of interest.
All claims expressed in this article are solely those of the authors and do not necessarily represent those of their affiliated organizations, or those of the publisher, the editors and the reviewers. Any product that may be evaluated in this article, or claim that may be made by its manufacturer, is not guaranteed or endorsed by the publisher.
Abraham N. M., Liu L., Jutras B. L., Yadav A. K., Narasimhan S., Gopalakrishnan V., et al. (2017). Pathogen-mediated manipulation of arthropod microbiota to promote infection. Proc. Natl. Acad. Sci. U S A. 114 (5), E781–E790. doi: 10.1073/pnas.1613422114
Abroug F., Ouanes-Besbes L., Tilouche N., Elatrous S. (2020). Scorpion envenomation: state of the art. Intensive Care Med. 46 (3), 401–410. doi: 10.1007/s00134-020-05924-8
Agnarsson I. (2023). Grand challenges in research on arachnid diversity, conservation, and biogeography. Front. Arachn. Sci. 2. doi: 10.3389/frchs.2023.1101141
Aitchison J. (1982). The statistical analysis of compositional data. J. R Stat. Soc 44, 139–160. doi: 10.1111/j.2517-6161.1982.tb01195.x
Alverdy J. C., Krezalek M. A. (2017). Collapse of the microbiome, emergence of the pathobiome, and the immunopathology of sepsis. Crit. Care Med. 45 (2), 337–347. doi: 10.1097/CCM.0000000000002172
Armstrong E. E., Perez-Lamarque B., Bi K., Chen C., Becking L. E., Lim J. Y., et al. (2022). A holobiont view of island biogeography: unravelling patterns driving the nascent diversification of a Hawaiian spider and its microbial associates. Mol. Ecol. 31 (4), 1299–1316. doi: 10.1111/mec.16301
Aželytė J., Wu-Chuang A., Maitre A., Žiegytė R., Mateos-Hernández L., Obregón D., et al. (2023). Avian malaria parasites modulate gut microbiome assembly in canaries. Microorganisms 11 (3), 563. doi: 10.3390/microorganisms11030563
Balloux F., van Dorp L. (2017). Q&A: what are pathogens, and what have they done to and for us? BMC Biol. 15 (1), 91. doi: 10.1186/s12915-017-0433-z
Banerjee S., Schlaeppi K., van der Heijden M. G. A. (2018). Keystone taxa as drivers of microbiome structure and functioning. Nat. Rev. Microbiol. 16 (9), 567–576. doi: 10.1038/s41579-018-0024-1
Bartoli C., Frachon L., Barret M., Rigal M., Huard-Chauveau C., Mayjonade B., et al. (2018). In situ relationships between microbiota and potential pathobiota in arabidopsis thaliana. ISME J. 12 (8), 2024–2038. doi: 10.1038/s41396-018-0152-7
Becchimanzi A., Tatè R., Campbell E. M., Gigliotti S., Bowman A. S., Pennacchio F. (2020). A salivary chitinase of Varroa destructor influences host immunity and mite’s survival. PloS Pathog. 16 (12), e1009075. doi: 10.1371/journal.ppat.1009075
Bennett G. M., Moran N. A. (2015). Heritable symbiosis: the advantages and perils of an evolutionary rabbit hole. Proc. Natl. Acad. Sci. U S A. 112 (33), 10169–10176. doi: 10.1073/pnas.1421388112
Berg G., Rybakova D., Fischer D., Cernava T., Vergès M. C., Charles T., et al. (2020). Microbiome definition re-visited: old concepts and new challenges. Microbiome 8 (1), 103. doi: 10.1186/s40168-020-00875-0
Binetruy F., Buysse M., Lejarre Q., Barosi R., Villa M., Rahola N., et al. (2020). Microbial community structure reveals instability of nutritional symbiosis during the evolutionary radiation of Amblyomma ticks. Mol. Ecol. 29 (5), 1016–1029. doi: 10.1111/mec.15373
Blanchet F. G., Cazelles K., Gravel D. (2020). Co-Occurrence is not evidence of ecological interactions. Ecol. Lett. 23 (7), 1050–1063. doi: 10.1111/ele.13525
Blaser M. J. (2014). The microbiome revolution. J. Clin. Invest. 124 (10), 4162–4165. doi: 10.1172/JCI78366
Cabezas-Cruz A., Estrada-Peña A., Rego R. O., de la Fuente J. (2017). Tick-pathogen ensembles: do molecular interactions lead ecological innovation? Front. Cell Infect. Microbiol. 7. doi: 10.3389/fcimb.2017.00074
Cabezas-Cruz A., Román-Carrasco P., Mateos-Hernández L., Duscher G. G., Sinha D. K., Hemmer W., et al. (2019). Environmental and molecular drivers of the α-gal syndrome. Front. Immunol. 10, 1210. doi: 10.3389/fimmu.2019.01210
Cabezas-Cruz A., Valdés J. J. (2014). Are ticks venomous animals? Front. Zool 11, 47. doi: 10.1186/1742-9994-11-47
Cabezas-Cruz A., Hodžić A., Mateos-Hernández L., Contreras M., de la Fuente J. (2021). Tick-human interactions: from allergic klendusity to the α-gal syndrome. Biochem. J. 478 (9), 1783–1794. doi: 10.1042/BCJ20200915
Callahan B. J., McMurdie P. J., Holmes S. P. (2017). Exact sequence variants should replace operational taxonomic units in marker-gene data analysis. ISME J. 11 (12), 2639–2643. doi: 10.1038/ismej.2017.119
Chen X., Liu L., Zhang W., Yang J., Wong K. C. (2021). Human host status inference from temporal microbiome changes via recurrent neural networks. Brief Bioinform. 22 (6), bbab223. doi: 10.1093/bib/bbab223
Chen L., Wan H., He Q., He S., Deng M. (2022). Statistical methods for microbiome compositional data network inference: a survey. J. Comput. Biol. 29 (7), 704–723. doi: 10.1089/cmb.2021.0406
Chigwada A. D., Mapholi N. O., Ogola H. J. O., Mbizeni S., Masebe T. M. (2022). Pathogenic and endosymbiotic bacteria and their associated antibiotic resistance biomarkers in amblyomma and hyalomma ticks infesting nguni cattle (Bos spp.). Pathog. 11 (4), 432. doi: 10.3390/pathogens11040432
Clayton K. A., Gall C. A., Mason K. L., Scoles G. A., Brayton K. A. (2015). The characterization and manipulation of the bacterial microbiome of the rocky mountain wood tick, Dermacentor andersoni. Parasites Vectors 8, 632. doi: 10.1186/s13071-015-1245-z
Corduneanu A., Wu-Chuang A., Maitre A., Obregon D., Sándor A. D., Cabezas-Cruz A. (2023). Structural differences in the gut microbiome of bats using terrestrial vs. aquatic feeding resources. BMC Microbiol. 23 (1), 93. doi: 10.1186/s12866-023-02836-7
Coyte K. Z., Rao C., Rakoff-Nahoum S., Foster K. R. (2021). Ecological rules for the assembly of microbiome communities. PloS Biol. 19 (2), e3001116. doi: 10.1371/journal.pbio.3001116
Debray R., Herbert R. A., Jaffe A. L., Crits-Christoph A., Power M. E., Koskella B. (2022). Priority effects in microbiome assembly. Nat. Rev. Microbiol. 20 (2), 109–121. doi: 10.1038/s41579-021-00604-w
Defazio J., Fleming I. D., Shakhsheer B., Zaborina O., Alverdy J. C. (2014). The opposing forces of the intestinal microbiome and the emerging pathobiome. Surg. Clin. North Am. 94 (6), 1151–1161. doi: 10.1016/j.suc.2014.08.002
de la Fuente J., Antunes S., Bonnet A., Cabezas-Cruz A., Domingos A. G., Estrada-Peña A., et al. (2017). Tick-pathogen interactions and vector competence: identification of molecular drivers for tick-borne diseases. Front. Cell Infect. Microbiol. 7. doi: 10.3389/fcimb.2017.00114
Denoncourt A. M., Paquet V. E., Charette S. J. (2014). Potential role of bacteria packaging by protozoa in the persistence and transmission of pathogenic bacteria. Front. Microbiol. 5. doi: 10.3389/fmicb.2014.00240
Derrida J. (1998). Of grammatology. Baltimore (Baltimore, Maryland, United States:Johns Hopkins University Press).
Díaz-Sánchez S., Estrada-Peña A., Cabezas-Cruz A., de la Fuente J. (2019). Evolutionary insights into the tick hologenome. Trends Parasitol. 35 (9), 725–737. doi: 10.1016/j.pt.2019.06.014
Douglas G. M., Maffei V. J., Zaneveld J. R., Yurgel S. N., Brown J. R., Taylor C. M., et al. (2020). PICRUSt2 for prediction of metagenome functions. Nat. Biotechnol. 38 (6), 685–688. doi: 10.1038/s41587-020-0548-6
Dunaj S. J., Bettencourt B. R., Garb J. E., Brucker R. M. (2020). Spider phylosymbiosis: divergence of widow spider species and their tissues’ microbiomes. BMC Evol. Biol. 20 (1), 104. doi: 10.1186/s12862-020-01664-x
Dupré J. O., O’Malley M. A. (2009). Varieties of living things: life at the intersection of lineage and metabolism, in Vitalism and the scientific image in post-enlightenment life science; history, philosophy and theory of the life sciences. Eds. Normandin S., Wolfe C. (Dordrecht: Springer), 1800–2010.
Duron O., Morel O., Noël V., Buysse M., Binetruy F., Lancelot R., et al. (2018). Tick-bacteria mutualism depends on b vitamin synthesis pathways. Curr. Biol. 28 (12), 1896–1902.e5. doi: 10.1016/j.cub.2018.04.038
Egger R., Yu J. (2022). Epistemological challenges, in Applied data science in tourism. tourism on the verge. Ed. Egger R. (Cham: Springer). doi: 10.1007/978-3-030-88389-8_2
Esmaeilishirazifard E., Usher L., Trim C., Denise H., Sangal V., Tyson G. H., et al. (2022). Bacterial adaptation to venom in snakes and Arachnida. Microbiol. Spectr. 10 (3), e0240821. doi: 10.1128/spectrum.02408-21
Estrada-Peña A., Cabezas-Cruz A., Obregón D. (2020). Behind taxonomic variability: the functional redundancy in the tick microbiome. Microorganisms 8 (11), 1829. doi: 10.3390/microorganisms8111829
Faccini-Martínez Á.A., Silva-Ramos C. R., Santodomingo A. M., Ramírez-Hernández A., Costa F. B., Labruna M. B., et al. (2022). Historical overview and update on relapsing fever group borrelia in Latin America. Parasites Vectors 15, 196. doi: 10.1186/s13071-022-05289-5
Fan Y., Pedersen O. (2021). Gut microbiota in human metabolic health and disease. Nat. Rev. Microbiol. 19, 55–71. doi: 10.1038/s41579-020-0433-9
Ford S. A., King K. C. (2016). Harnessing the power of defensive microbes: evolutionary implications in nature and disease control. PloS Pathog. 12 (4), e1005465. doi: 10.1371/journal.ppat.1005465
Gall C. A., Scoles G. A., Magori K., Mason K. L., Brayton K. A. (2017). Laboratory colonization stabilizes the naturally dynamic microbiome composition of field collected Dermacentor andersoni ticks. Microbiome 5 (1), 133. doi: 10.1186/s40168-017-0352-9
Galloway-Peña J., Hanson B. (2020). Tools for analysis of the microbiome. Dig Dis. Sci. 65 (3), 674–685. doi: 10.1007/s10620-020-06091-y
Guo B., Zhang L., Sun H., Gao M., Yu N., Zhang Q., et al. (2022). Microbial co-occurrence network topological properties link with reactor parameters and reveal importance of low-abundance genera. NPJ Biofilms Microbiomes. 8 (1), 3. doi: 10.1038/s41522-021-00263-y
Guseva K., Darcy S., Simon E., Alteio L. V., Montesinos-Navarro A., Kaiser C. (2022). From diversity to complexity: microbial networks in soils. Soil Biol. Biochem. 169, 108604. doi: 10.1016/j.soilbio.2022.108604
Hammer T. J., Sanders J. G., Fierer N. (2019). Not all animals need a microbiome. FEMS Microbiol. Lett. 366 (10), fnz117. doi: 10.1093/femsle/fnz117
Hernández Medina R., Kutuzova S., Nielsen K. N., Johansen J., Hansen L. H., Nielsen M. (2022). Machine learning and deep learning applications in microbiome research. ISME Commun. 2, 98. doi: 10.1038/s43705-022-00182-9
Hu T., Gallins P., Zhou Y. H. (2018). A zero-inflated beta-binomial model for microbiome data analysis. Stat. (Int Stat. Inst). 7 (1), e185. doi: 10.1002/sta4.185
Hughes J. B., Hellmann J. J. (2005). The application of rarefaction techniques to molecular inventories of microbial diversity. Methods Enzymol. 397, 292–308. doi: 10.1016/S0076-6879(05)97017-1
Jones E. W., Carlson J. M., Sivak D. A., Ludington W. B. (2022). Stochastic microbiome assembly depends on context. Proc. Natl. Acad. Sci. U S A. 119 (7), e2115877119. doi: 10.1073/pnas.2115877119
Kaiser T., Jahansouz C., Staley C. (2022). Network-based approaches for the investigation of microbial community structure and function using metagenomics-based data. Future Microbiol. 17, 621–631. doi: 10.2217/fmb-2021-0219
Kuntner M. (2022). The seven grand challenges in arachnid science. Front. Arachn. Sci. 1, 1082700. doi: 10.3389/frchs.2022.1082700
Kurokawa C., Lynn G. E., Pedra J. H. F., Pal U., Narasimhan S., Fikrig E. (2020). Interactions between Borrelia burgdorferi and ticks. Nat. Rev. Microbiol. 18 (10), 587–600. doi: 10.1038/s41579-020-0400-5
Lederberg J., Mccray A. T. (2001)“Ome sweet `Omics–a genealogical treasury of words.” In: The scientist (Gale Academic OneFile) (Accessed 9 Nov. 2022).
Li P., Luo H., Ji B., Nielsen J. (2022). Machine learning for data integration in human gut microbiome. Microb. Cell Fact. 21 (1), 241. doi: 10.1186/s12934-022-01973-4
Lin H., Peddada S. D. (2020). Analysis of microbial compositions: a review of normalization and differential abundance analysis. NPJ Biofilms Microbiomes. 6 (1), 60. doi: 10.1038/s41522-020-00160-w
Lüddecke T., Herzig V., von Reumont B. M., Vilcinskas A. (2022). The biology and evolution of spider venoms. Biol. Rev. Camb Philos. Soc 97 (1), 163–178. doi: 10.1111/brv.12793
Maitre A., Wu-Chuang A., Mateos-Hernández L., Foucault-Simonin A., Moutailler S., Paoli J. C., et al. (2022). Rickettsia helvetica infection is associated with microbiome modulation in Ixodes ricinus collected from humans in Serbia. Sci. Rep. 12 (1), 11464. doi: 10.1038/s41598-022-15681-x
Malla M. A., Dubey A., Kumar A., Yadav S., Hashem A., Abd_Allah E. F. (2019). Exploring the human microbiome: the potential future role of next-generation sequencing in disease diagnosis and treatment. Front. Immunol. 9, 2868. doi: 10.3389/fimmu.2018.02868
Marchesi J. R., Ravel J. (2015). The vocabulary of microbiome research: a proposal. Microbiome 3, 31. doi: 10.1186/s40168-015-0094-5
Marcos-Zambrano L. J., Karaduzovic-Hadziabdic K., Loncar Turukalo T., Przymus P., Trajkovik V., Aasmets O., et al. (2021). Applications of machine learning in human microbiome studies: a review on feature selection, biomarker identification, disease prediction and treatment. Front. Microbiol. 12. doi: 10.3389/fmicb.2021.634511
Mateos-Hernández L., Obregón D., Maye J., Borneres J., Versille N., de la Fuente J., et al. (2020). Anti-tick microbiota vaccine impacts Ixodes ricinus performance during feeding. Vaccines (Basel). 8 (4), 702. doi: 10.3390/vaccines8040702
Mateos-Hernández L., Obregón D., Wu-Chuang A., Maye J., Bornères J., Versillé N., et al. (2021). Anti-microbiota vaccines modulate the tick microbiome in a taxon-specific manner. Front. Immunol. 12. doi: 10.3389/fimmu.2021.704621
Moran N. A., Sloan D. B. (2015). The hologenome concept: helpful or hollow? PloS Biol. 13 (12), e1002311. doi: 10.1371/journal.pbio.1002311
Narasimhan S., Rajeevan N., Liu L., Zhao Y. O., Heisig J., Pan J., et al. (2014). Gut microbiota of the tick vector Ixodes scapularis modulate colonization of the Lyme disease spirochete. Cell Host Microbe 15 (1), 58–71. doi: 10.1016/j.chom.2013.12.001
Nearing J. T., Douglas G. M., Hayes M. G., MacDonald J., Desai D. K., Allward N., et al. (2022). Microbiome differential abundance methods produce different results across 38 datasets. Nat. Commun. 13 (1), 342. doi: 10.1038/s41467-022-28034-z
Neelakanta G., Sultana H., Fish D., Anderson J. F., Fikrig E. (2010). Anaplasma phagocytophilum induces Ixodes scapularis ticks to express an antifreeze glycoprotein gene that enhances their survival in the cold. J. Clin. Invest. 120 (9), 3179–3190. doi: 10.1172/JCI42868
Newman M. E. J. (2010). “An introduction to the mathematical tools used in the study of networks, tools that will be important to many subsequent developments,” in Networks: an introduction. Ed. Newman M. (Oxford, UK:Oxford), 109–167. doi: 10.1093/acprof:oso/9780199206650.003.0006
Ogawa M., Takahashi M., Matsutani M., Takada N., Noda S., Saijo M. (2020). Obligate intracellular bacteria diversity in unfed Leptotrombidium scutellare larvae highlights novel bacterial endosymbionts of mites. Microbiol. Immunol. 64 (1), 1–9. doi: 10.1111/1348-0421.12745
Price D. C., Brennan J. R., Wagner N. E., Egizi A. M. (2021). Comparative hologenomics of two Ixodes scapularis tick populations in new Jersey. PeerJ 9, e12313. doi: 10.7717/peerj.12313
Quinn T. P., Erb I., Richardson M. F., Crowley T. M. (2018). Understanding sequencing data as compositions: an outlook and review. Bioinformatics 34 (16), 2870–2878. doi: 10.1093/bioinformatics/bty175
Ramamoorthi N., Narasimhan S., Pal U., Bao F., Yang X. F., Fish D., et al. (2005). The Lyme disease agent exploits a tick protein to infect the mammalian host. Nature 436 (7050), 573–577. doi: 10.1038/nature03812
Rasmussen J. A., Villumsen K. R., Ernst M., Hansen M., Forberg T., Gopalakrishnan S., et al. (2022). A multi-omics approach unravels metagenomic and metabolic alterations of a probiotic and synbiotic additive in rainbow trout (Oncorhynchus mykiss). Microbiome 10 (1), 21. doi: 10.1186/s40168-021-01221-8
Richards E. H., Jones B., Bowman A. (2011). Salivary secretions from the honeybee mite, Varroa destructor: effects on insect haemocytes and preliminary biochemical characterization. Parasitology 138 (5), 602–608. doi: 10.1017/S0031182011000072
Román-Carrasco P., Hemmer W., Cabezas-Cruz A., Hodžić A., de la Fuente J., Swoboda I. (2021). The α-gal syndrome and potential mechanisms. Front. Allergy 2. doi: 10.3389/falgy.2021.783279
Röttjers L., Faust K. (2018). From hairballs to hypotheses-biological insights from microbial networks. FEMS Microbiol. Rev. 42 (6), 761–780. doi: 10.1093/femsre/fuy030
Schausberger P. (2022). Grand challenges and bold opportunities in arachnid ecology and behavior. Front. Arachn. Sci. 1, 1097945. doi: 10.3389/frchs.2022.1097945
Schiavone A., Pugliese N., Domenico Otranto D., Rossella Samarelli R., Circella E., De Virgilio C., et al. (2022). Dermanyssus gallinae: the long journey of the poultry red mite to become a vector. Parasit Vectors. 15 (1), 29. doi: 10.1186/s13071-021-05142-1
Sergaki C., Lagunas B., Lidbury I., Gifford M. L., Schäfer P. (2018). Challenges and approaches in microbiome research: from fundamental to applied. Front. Plant Sci. 9. doi: 10.3389/fpls.2018.01205
Stevens E. J., Bates K. A., King K. C. (2021). Host microbiota can facilitate pathogen infection. PloS Pathog. 17 (5), e1009514. doi: 10.1371/journal.ppat.1009514
Sun S., Jones R. B., Fodor A. A. (2020). Inference-based accuracy of metagenome prediction tools varies across sample types and functional categories. Microbiome 8 (1), 46. doi: 10.1186/s40168-020-00815-y
Vilarem C., Piou V., Vogelweith F., Vétillard A. (2021). Varroa destructor from the laboratory to the field: control, biocontrol and IPM perspectives-a review. Insects 12 (9), 800. doi: 10.3390/insects12090800
Wang W. L., Xu S. Y., Ren Z. G., Tao L., Jiang J. W., Zheng S. S. (2015). Application of metagenomics in the human gut microbiome. World J. Gastroenterol. 21 (3), 803–814. doi: 10.3748/wjg.v21.i3.803
Wassan J. T., Wang H., Browne F., Zheng H. (2019). Phy-PMRFI: phylogeny-aware prediction of metagenomic functions using random forest feature importance. IEEE Trans. Nanobiosci. 18 (3), 273–282. doi: 10.1109/TNB.2019.2912824
Wei N., Cao J., Zhang H., Zhou Y., Zhou J. (2021). The tick microbiota dysbiosis promote tick-borne pathogen transstadial transmission in a babesia microti–infected mouse model. Front. Cell. Infect. Microbiol. 11. doi: 10.3389/fcimb.2021.713466
Wu-Chuang A., Bates K. A., Obregon D., Estrada-Peña A., King K. C., Cabezas-Cruz A. (2022a). Rapid evolution of a novel protective symbiont into keystone taxon in caenorhabditis elegans microbiota. Sci. Rep. 12 (1), 14045. doi: 10.1038/s41598-022-18269-7
Wu-Chuang A., Hodžić A., Mateos-Hernández L., Estrada-Peña A., Obregon D., Cabezas-Cruz A. (2021). Current debates and advances in tick microbiome research. Curr. Res. Parasitol. Vector Borne Dis. 1, 100036. doi: 10.1016/j.crpvbd.2021.100036
Wu-Chuang A., Obregon D., Estrada-Peña A., Cabezas-Cruz A. (2022b). Thermostable keystone bacteria maintain the functional diversity of the Ixodes scapularis microbiome under heat stress. Microb. Ecol. 84 (4), 1224–1235. doi: 10.1007/s00248-021-01929-y
Wu-Chuang A., Obregon D., Mateos-Hernández L., Cabezas-Cruz A. (2022c). Anti-tick microbiota vaccines: how can this actually work? Biologia 77, 1555–1562. doi: 10.1007/s11756-021-00818-6
Yen S., Johnson J. S. (2021). Metagenomics: a path to understanding the gut microbiome. Mamm Genome. 32 (4), 282–296. doi: 10.1007/s00335-021-09889-x
Zhang R., Huang Z., Yu G., Zhang Z. (2019). Characterization of microbiota diversity of field-collected Haemaphysalis longicornis (Acari: ixodidae) with regard to sex and blood meals. J. Bas. Microbiol. 59 (2), 215–223. doi: 10.1002/jobm.201800372
Zheng F., An X. L., Zhou G. W., Zhu D., Neilson R., Chen B., et al. (2022). Mite gut microbiome and resistome exhibited species-specific and dose-dependent effect in response to oxytetracycline exposure. Sci. Tot. Environ. 807 (Pt 2), 150802. doi: 10.1016/j.scitotenv.2021.150802
Zhong J., Jasinskas A., Barbour A. G. (2007). Antibiotic treatment of the tick vector Amblyomma americanum reduced reproductive fitness. PloS One 2 (5), e405. doi: 10.1371/journal.pone.0000405
Zhou J., Ning D. (2017). Stochastic community assembly: does it matter in microbial ecology? Microbiol. Mol. Biol. Rev. 81 (4), e00002–e00017. doi: 10.1128/MMBR.00002-17
Zhu Y. X., Song Y. L., Hoffmann A. A., Jin P. Y., Huo S. M., Hong X. Y. (2019). A change in the bacterial community of spider mites decreases fecundity on multiple host plants. Microbiologyopen 8 (6), e00743. doi: 10.1002/mbo3.743
Keywords: Arachnida, microbiota, vector-borne diseases, epistemology, ontology
Citation: Cabezas-Cruz A (2023) Grand challenges in arachnid microbiota and diseases. Front. Arachn. Sci. 2:1215831. doi: 10.3389/frchs.2023.1215831
Received: 02 May 2023; Accepted: 10 May 2023;
Published: 20 June 2023.
Edited and Reviewed by:
Matjaz Kuntner, National Institute of Biology (NIB), SloveniaCopyright © 2023 Cabezas-Cruz. This is an open-access article distributed under the terms of the Creative Commons Attribution License (CC BY). The use, distribution or reproduction in other forums is permitted, provided the original author(s) and the copyright owner(s) are credited and that the original publication in this journal is cited, in accordance with accepted academic practice. No use, distribution or reproduction is permitted which does not comply with these terms.
*Correspondence: Alejandro Cabezas-Cruz, YWxlamFuZHJvLmNhYmV6YXNAdmV0LWFsZm9ydC5mcg==
Disclaimer: All claims expressed in this article are solely those of the authors and do not necessarily represent those of their affiliated organizations, or those of the publisher, the editors and the reviewers. Any product that may be evaluated in this article or claim that may be made by its manufacturer is not guaranteed or endorsed by the publisher.
Research integrity at Frontiers
Learn more about the work of our research integrity team to safeguard the quality of each article we publish.