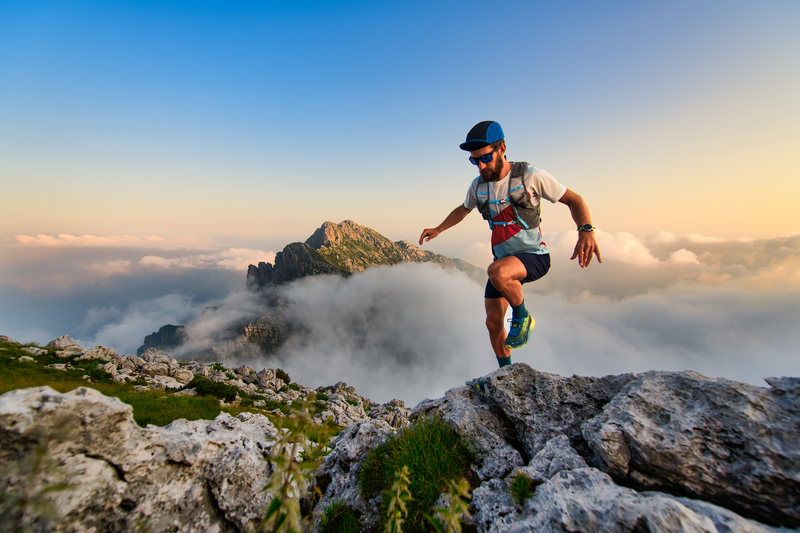
95% of researchers rate our articles as excellent or good
Learn more about the work of our research integrity team to safeguard the quality of each article we publish.
Find out more
EDITORIAL article
Front. Appl. Math. Stat. , 25 February 2025
Sec. Mathematical Finance
Volume 11 - 2025 | https://doi.org/10.3389/fams.2025.1573606
This article is part of the Research Topic Applied Quantitative Methods for Corporate Finance and Investments View all 5 articles
Editorial on the Research Topic
Applied quantitative methods for corporate finance and investments
In the rapidly evolving world of corporate finance and investments, the demand for advanced analytical methods to guide decision-making processes has never been greater. Traditionally, finance relied heavily on theoretical models, but today's dynamic financial markets and intricate corporate structures require more robust, data-driven solutions. The field of applied quantitative methods has become an essential tool for financial professionals seeking to make more accurate predictions, optimize portfolios, and enhance corporate governance. By leveraging statistical models, computational techniques, and data analytics, specialists in finance can better manage risk, evaluate investment opportunities, and maximize shareholder value.
Quantitative methods in corporate finance are grounded in a variety of mathematical and statistical techniques that provide insights into the financial world. These methods, which include regression analysis, time series analysis, stochastic processes, and optimization algorithms, allow financial analysts to make sense of vast amounts of financial data. Moreover, data analytics and machine learning have introduced an entirely new dimension to corporate finance and investment management. By analyzing big data, companies can uncover patterns and trends that were previously hidden, helping them make more informed decisions. Algorithms can detect market anomalies, identify investment opportunities, and even automate trading strategies. As machine learning continues to evolve, its potential to transform financial markets is immense, making the integration of artificial intelligence (AI) into financial decision-making an exciting frontier. Nevertheless, despite the advantages of applied quantitative methods, there are challenges that come with their use. Financial models are based on assumptions that may not always hold true in the real world. Therefore, over-reliance on quantitative methods without considering qualitative factors can lead to flawed decision-making. Besides, there is a growing need for transparency and ethical considerations in the use of such advanced methods, especially as automation and algorithmic trading become more pervasive in the financial industry.
The Research Topic “Applied Quantitative Methods for Corporate Finance and Investments” focuses on the integration of advanced quantitative techniques in corporate finance, investment analysis, and financial modeling. The research emphasizes the practical application of these methods in addressing contemporary challenges in finance and investment strategies.
Zhu et al. analyzes the impact of digital financial agglomeration on the economic resilience of 30 Chinese provinces (excluding Hainan) from 2011 to 2020, using panel data and spatial correlation tests. The study finds that digital financial agglomeration positively influences economic resilience by increasing consumption scale. It has both a direct effect on the region's economic resilience and an indirect spillover effect on neighboring regions. The research also highlights that the positive impacts of digital financial agglomeration, consumption scale, and their interaction are significant. To enhance these effects, the article suggests strengthening financial support, improving infrastructure, encouraging consumption, and promoting coordinated regional financial development, with tailored policies to address varying levels of digital financial concentration across cities.
The article of Zhang and Yin uses machine learning algorithms to predict the green innovation performance of micro-entities in China, examining the impact of internal drivers, external regulations, and financial capabilities on corporate green innovation. Key findings show that the gradient ascent algorithm best predicts performance, with financial indicators and operational capacity playing crucial roles in driving green innovation. Internal mechanisms, such as financial growth, have a positive effect, while certain regulatory tools, like administrative penalties and pollution control investments, incentivize green innovation. However, excessive environmental taxes can hinder progress. The study suggests governments shape policies for micro-entities, enforce regulations, improve financing mechanisms, and adjust tax structures. Enterprises, on the other hand, should optimize internal resources, comply with regulations, and increase transparency to enhance green competitiveness and encourage sustainable development.
Osterrieder and Seigne discuss the gap in academic research regarding the execution phase of share buybacks. The article explores various complexities like financial anomalies, market biases, and advanced computational techniques such as genetic algorithms and neural networks. There are revealed inefficiencies in how buybacks are executed, which has significant implications for both practitioners and policymakers. For practitioners, this knowledge gap could lead to financial losses, distorted markets, and damage to corporate reputation, urging them to adopt better strategies. For policymakers, there is a need to update regulatory frameworks to ensure fairness, transparency, and efficiency in buyback executions, especially as technological advancements continue to reshape the market landscape.
The research of Oudat et al. aimed to explore the relationship between capital, liquidity, and operational risks and financial performance indicators (return on assets and return on equity) in United Arab Emirates (UAE) banks, considering the impact of bank size. The study analyzed data from 10 banks over 7 years (2015–2021). Findings revealed a positive correlation between bank size and return on equity, with larger banks generally yielding higher returns. Capital risk positively influenced return on assets, while liquidity and operational risks had no significant impact. Regression analysis showed that both capital risk and bank size were predictive of return on assets, but liquidity and operational risks did not predict return on equity. The study advises UAE banks to focus on managing capital risk to improve return on assets and consider expanding operations to increase return on equity. However, bank expansion should be managed carefully due to potential operational and liquidity risks. This research provides valuable insights into UAE bank's risk management and performance, helping policymakers and bank executives enhance industry efficiency.
Finally, as financial markets become more complex and interconnected, the future of corporate finance and investments lies in the continuous integration of innovative quantitative tools. Financial institutions and corporations must stay ahead of the curve by adopting these methods, whether through in-house expertise or external consultants. Academic research and collaboration between the finance industry and quantitative disciplines will drive further advancements, ensuring that businesses remain competitive and resilient in an increasingly volatile global marketplace. Also, the ability to harness the power of quantitative methods will not only enhance financial performance but also drive innovation and create new opportunities in the corporate finance and investment sectors.
ŞCG: Conceptualization, Data curation, Formal analysis, Funding acquisition, Investigation, Methodology, Project administration, Resources, Software, Supervision, Validation, Visualization, Writing – original draft, Writing – review & editing. LNS: Conceptualization, Data curation, Formal analysis, Funding acquisition, Investigation, Methodology, Project administration, Resources, Software, Supervision, Validation, Visualization, Writing – original draft, Writing – review & editing.
The authors declare that the research was conducted in the absence of any commercial or financial relationships that could be construed as a potential conflict of interest.
All claims expressed in this article are solely those of the authors and do not necessarily represent those of their affiliated organizations, or those of the publisher, the editors and the reviewers. Any product that may be evaluated in this article, or claim that may be made by its manufacturer, is not guaranteed or endorsed by the publisher.
Keywords: corporate finance, investments, quantitative methods, capital structure, firm risk, company performance, stock return
Citation: Gherghina ŞC and Simionescu LN (2025) Editorial: Applied quantitative methods for corporate finance and investments. Front. Appl. Math. Stat. 11:1573606. doi: 10.3389/fams.2025.1573606
Received: 09 February 2025; Accepted: 13 February 2025;
Published: 25 February 2025.
Edited and reviewed by: Young Shin Aaron Kim, Stony Brook University, United States
Copyright © 2025 Gherghina and Simionescu. This is an open-access article distributed under the terms of the Creative Commons Attribution License (CC BY). The use, distribution or reproduction in other forums is permitted, provided the original author(s) and the copyright owner(s) are credited and that the original publication in this journal is cited, in accordance with accepted academic practice. No use, distribution or reproduction is permitted which does not comply with these terms.
*Correspondence: Ştefan Cristian Gherghina, c3RlZmFuLmdoZXJnaGluYUBmaW4uYXNlLnJv
Disclaimer: All claims expressed in this article are solely those of the authors and do not necessarily represent those of their affiliated organizations, or those of the publisher, the editors and the reviewers. Any product that may be evaluated in this article or claim that may be made by its manufacturer is not guaranteed or endorsed by the publisher.
Research integrity at Frontiers
Learn more about the work of our research integrity team to safeguard the quality of each article we publish.