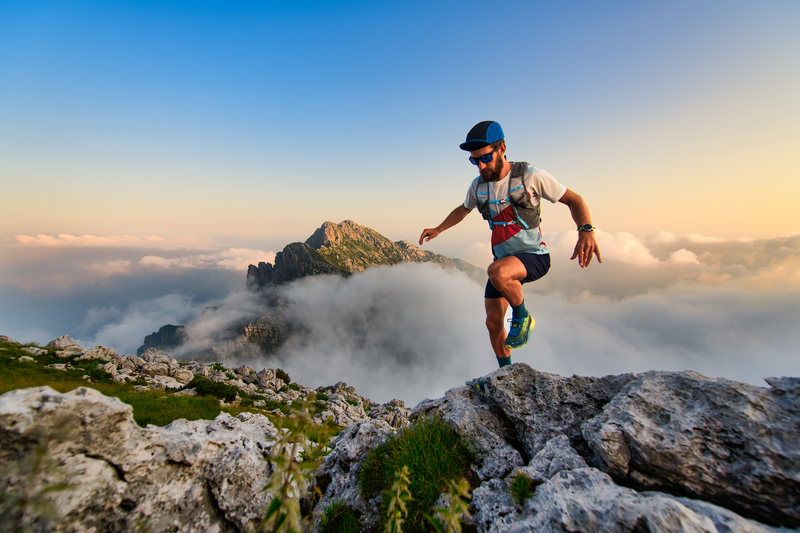
95% of researchers rate our articles as excellent or good
Learn more about the work of our research integrity team to safeguard the quality of each article we publish.
Find out more
ORIGINAL RESEARCH article
Front. Appl. Math. Stat.
Sec. Mathematical Finance
Volume 11 - 2025 | doi: 10.3389/fams.2025.1554144
The final, formatted version of the article will be published soon.
You have multiple emails registered with Frontiers:
Please enter your email address:
If you already have an account, please login
You don't have a Frontiers account ? You can register here
We propose a dynamic fractional volatility model that incorporates a time-varying Hurst exponent estimated via Daubechies-4 wavelet analysis on 252-day rolling windows to capture evolving market memory effects in equity markets. This approach overcomes the limitations of traditional GARCH-type (Engle, 1982) and static fractional volatility models (Baillie et al., 1996), which assume a constant memory parameter and struggle during regime shifts and market stress. The model is applied to daily closing prices of the S&P 500 Index over 1,258 trading days from January 1, 2015 to December 31, 2019, yielding statistically significant improvements in forecasting performance. Empirical results indicate a 12.3% reduction in RMSE and a 9.8% improvement in MAPE, with an out-of-sample R-squared exceeding 0.72 compared to benchmark models. Maximum likelihood estimation with Fisher scoring is used for daily parameter updates, ensuring the model remains responsive to rapidly changing market conditions. Additionally, the model achieves an average absolute option pricing error of 1.8%, markedly lower than that of traditional specifications. These enhancements are further corroborated by pairwise Diebold-Mariano tests, which confirm the statistical significance of the forecast improvements. Overall, this framework offers a rigorous and computationally efficient method for real-time volatility forecasting that delivers substantial benefits for risk management, derivative pricing, and automated trading strategies, thereby extending the theoretical foundations established by Cont (2005), Mandelbrot andHudson (2004), andPercival andWalden (2000).
Keywords: time-varying Hurst exponent, Volatility modeling, fractal dynamics, wavelet analysis, Adaptive Market Hypothesis, Stochastic volatility, Equity markets
Received: 01 Jan 2025; Accepted: 31 Mar 2025.
Copyright: © 2025 Webb. This is an open-access article distributed under the terms of the Creative Commons Attribution License (CC BY). The use, distribution or reproduction in other forums is permitted, provided the original author(s) or licensor are credited and that the original publication in this journal is cited, in accordance with accepted academic practice. No use, distribution or reproduction is permitted which does not comply with these terms.
* Correspondence:
Abe Webb, Westchester Research, Los Angeles, United States
Disclaimer: All claims expressed in this article are solely those of the authors and do not necessarily represent those of their affiliated organizations, or those of the publisher, the editors and the reviewers. Any product that may be evaluated in this article or claim that may be made by its manufacturer is not guaranteed or endorsed by the publisher.
Research integrity at Frontiers
Learn more about the work of our research integrity team to safeguard the quality of each article we publish.