- Department of Mathematics, Kotebe University of Education, Addis Ababa, Ethiopia
The main finding of this study is the derivation of a new probability distribution that reveals interesting properties, especially with various asymmetry and kurtosis behavior. We call this distribution the asymmetric generalized error distribution (AGED). AGED is a new contribution to the field of statistical theory, offering more flexible probability density functions, cumulative distribution functions, and hazard functions than the base distribution. The AGED also includes normal, uniform, Laplace, asymmetric Laplace, and generalized error distribution (GED) as special cases. The mathematical and statistical features of the distribution are derived and discussed. Estimators of the parameters of the distribution are obtained using the maximum likelihood approach. In a simulation study, random samples are generated from the new probability distribution to illustrate what ideal data looks like. Using real data from diverse applications such as health, industry, and cybersecurity domains, the performance of the new distribution is compared to that of other distributions. The new distribution is found to be a better fit for the data, showing great adaptability in the context of real data analysis. We expect the distribution to be applied to many more real data, and the findings of the study can be used as a basis for future research in the field.
1 Introduction
There has been a growing interest in the construction of flexible parametric families of distributions that exhibit asymmetry and peakedness differing from those of symmetric distributions (1–3). Many of these methods center around overcoming the assumptions of normality found in the empirical analysis of many parametric models.
An empirical analysis in various studies suggests that the assumption of normality of real data is often untenable (4, 5), and asymmetry is commonly observed (6, 7). It is highly acknowledged that data with heavy-peaked distribution are encountered in the empirical analysis (8), as is asymmetric distribution (9, 10). In all cases, it is important to adopt a flexible distribution that can directly address asymmetry and peakedness (6, 9).
There has been a different approach to develop asymmetric counterparts of symmetric distributions. Many of these approaches centered on overcoming assumptions of normality (11, 12). In many works of literature, asymmetry is achieved via the transformation of the skewing function (9, 10), which lacks a wide range of skewness and kurtosis. Moreover, the technique of creating asymmetric counterparts of symmetric distributions has a longer history (13–15).
The approach that is commonly considered for constructing classes of asymmetric distributions from symmetric distribution is authored by Azzalini (16, 17). The initial idea appeared in O’hagan and Leonard (18) in the context of base distribution. Azzalini (16, 17) introduced asymmetric distributions called skew-normal (SN). The idea was further extended in Azzalini (13) introduced multivariate asymmetry distributions. An ideal class of distributions obtained from this methodology includes symmetric distributions, mathematical tractability, and a wide range of skewness and kurtosis. The theoretical and statistical properties of the methodology have been studied by various researchers (2, 3, 19, 20).
As noted in (5, 12) the generalized error distribution (GED) has short tails, making it unsuitable for modeling data with heavier tails. One method to solve this problem, as suggested in Azzalini (16), is to use an asymmetric pdf with flexible tails and excess kurtosis. Azzalini’s methodology generates distributions with flexible tails and excess kurtosis.
In this study, we follow Azzalini’s methodology to introduce a new distribution that is flexible enough for modeling data with heavier tails and excess kurtosis. More data with heavier tails and excess kurtosis are adequately modeled to the distribution and play an important role in this context. This new distribution is called asymmetric generalized error distribution (AGED) and is denoted by , where represents the asymmetry parameter so that corresponds to the generalized error distribution. We outline some properties of the distribution, provide a graphical representation of the distribution, and discuss some inferences.
2 Generalized error distribution
The GED is a symmetric and unimodal member of the exponential family of distribution introduced by Subbotin (22) and has been used by different authors with different parameterizations (23, 24). A random variable have a generalized error distribution if its probability density function (pdf) is expressed by (21):
where is the location parameter, is the scale parameter, and is the shape parameter. Here, is the Euler gamma function. We denote it by .
It is convenient to work with the alternative expression given in Eq. 1, which allows for mean zero and variance unity (25). The variance of the GED is a function of (26, 27). To rescale its variance, a scaling parameter η is introduced, and a substitution is made for in Eq. 1 to get the following equivalent pdf (25):
Or
3 The newly suggested asymmetric generalized error distribution
In this section, the method of generating an asymmetric distribution from a symmetric distribution is presented to develop the new asymmetric generalized error distribution (AGED). Here, the method of constructing classes of asymmetric distributions suggested by Azzalini (16, 17) is used. The authors introduced a methodology that can be used to derive an asymmetric distribution from an existing symmetric distribution. This is expressed in Proposition 1.
3.1 Proposition 1
Let and be pdf and cdf of the random variable , respectively, and characterizing symmetric distribution such that , , for all . Then, the random variable has an asymmetric probability density function expressed in the form of:
where is the asymmetry parameter and is an asymmetric version of a symmetric base pdf.
In this study, we derived a new asymmetric distribution called the AGED. The approach of Azzalini (16, 17) is used with the base distribution of the generalized error distribution in Eq. 3.
3.2 Theorem 1
For the generalized error distribution, GED, in Eq. 3, the new asymmetric generalized error distribution (AGED), has probability density and cumulative distribution functions expressed as follows:
where , and is Owen’s function (28). Here, Γ is the Euler gamma function. The parameters determine the degree of asymmetry, which can generate distributions with flexible tail behavior and excess kurtosis. We denote it by . See Ref. (29–31).
3.3 Proof
Suppose is a pdf of GED defined in Eq. 3 and cdf, obtained as:
We have two cases to consider.
Case 1: for
Let,
Case 2: for , similarly
3.4 Corollary 1
A linear combination of the AGED is also asymmetric. In particular, the inclusion of and variance is possible using the transformation, , where have AGED with mean zero and variance 1. Then, a random variable is said to have an asymmetric generalized error distribution, , and it has pdf expressed by:
Where and are the pdf and cdf of the symmetric base distribution, respectively, is the asymmetry parameters, and is the asymmetric version made from the symmetric distribution.
The theorem 2 shows a pdf of the AGED with shape parameter and asymmetry parameter , which is generated using the representation given in Eq. 12.
3.5 Theorem 2
Let and be symmetric random variables such that and . Then, the representation of the new asymmetric generalized error distribution is
We call the distribution of the asymmetric generalized error distribution (AGED).
3.6 Proof
Let Then
But, and are symmetric random variables, and following that:
Since and have symmetric pdf, we have:
Then, has a pdf of , which is defined in Eq. 5.
3.7 Corollary 2
Let and , where and . Then, for , random variable converges in distribution to .
3.8 Proof
Since and , that is . Therefore, by applying Slutsky’s lemma (32) to to obtain:
That is, for decreasing value of , converges in distribution to .
Using the distribution, reliability measures can be assessed. Identification of a system’s important components and estimation of the effects of component failure are important in reliability measures (33). Therefore, it is essential to derive the functions of the AGED reliability measures, an important quantity characterizing life phenomena (34).
For a random variable with probability density function and cumulative distribution function and defined in Eqs 5, 6, respectively, survival and hazard functions can be defined as and , respectively (35).
4 Plots of the asymmetric generalized error distribution
Graphs of probability density and cumulative distribution function of AGED are illustrated in Figure 1 for some values of parameters that give possible shapes of function. The asymmetry parameter controls the magnitude of the asymmetry exhibited by the probability density function. The AGED can take a number of forms, including symmetric, near symmetric, and asymmetric. As , the asymmetric generalized error distribution converges in distribution to the half asymmetric generalized error distribution, and for , the distribution reduced to the generalized error distribution. However, for , the generalized error distribution converges to the asymmetric generalized error distribution (Figure 2).
Extremely illustrated properties instantly follow from definition 1 and Figure 1 is as follows:
If , then the following properties are concluded directly from theorem (1) and Figures 1, 3, 4:
• If , then : The distribution reduced to the generalized error distribution with location parameter , scale parameter , and shape parameter (21).
• If , then : The distribution becomes a half asymmetric generalized error distribution with location parameter , scale parameter , and shape parameter .
• If and 1, then : The distribution is asymmetric Laplace distribution with location parameter and scale parameters and (27).
• If , then : The AGED distribution goes to asymmetric student distribution with location parameter , scale parameter , and shape parameters and (26).
5 Moment and its measures
Let be a random variable from AGED with pdf defined in Eq. 5, the moments of the random variable is obtained as follows (36):
where . However, for a random variable with pdf given in Eq. 5, it follows the form of the binomial theorem:
Consider a random variable with pdf in Eq. 5, then
where and similarly,
and moment of becomes:
Therefore, for a random variable , the moment for a random variable can be defined as:
In particular, the first four moments of a random variable are defined as:
The skewness and kurtosis of the asymmetric generalized error distribution are functions of , and . However, the actual equations in terms of , and are quite expansive. In compact form, we can write the variance , skewness , and kurtosis of using the standardized moments of and defined as:
We perform a brief comparison illustrating that the tails of the AGED are heavier than those of the GED. Table 1 noted that the AGED has much heavier tails than the GED, as Figure 1 depicts the AGED for different values of parameters.
Similarly, the degree of asymmetry and peakedness of the AGED for different values of and are shown, and for small values of , the kurtosis coefficient increases in the AGED. The ranges of both coefficients are smaller in GED. Thus, the AGED is more flexible for modeling data with larger coefficients of asymmetry and kurtosis.
6 Estimation of the parameters
In this section, we go over how to estimate the AGED parameters using the maximum likelihood approach.
6.1 Maximum likelihood estimation
Let be an independent and identically distributed (i.i.d.) random variable and having the density function defined in Eq. 5, then the likelihood function of AGED is defined as (36):
The maximum likelihood estimator is the value that maximizes the likelihood function (36). Rather than the likelihood function, the log-likelihood function of AGED is given as:
where is the natural logarithm function, and .
By differentiating Eq. 25 with respect to the parameters and and equating them to 0, we obtain:
Solving Eqs 26–29, we get MLEs of and . However, there is no explicit form for the solutions to these equations; thus, we obtain the MLEs numerically using the fitdistrplus package in R (37).
Maximum likelihood estimators are consistent in the sense that as and asymptotically normally distributed: such that , where is the variance–covariance matrix and can be obtained by inverting the Fisher information matrix (38).
We now take the second partial derivatives of Eqs 26–29, and the observed hessian matrix of the AGED distribution can be obtained and is given by:
Based on the above, the observed Fisher information matrix , from which we can derive the estimated dispersion matrix as:
In addition, for ,3,4. The asymptotic normality distribution of MLEs is guaranteed. More precisely, the random vector of follows the multivariate normal distribution .
7 Simulations studies
To establish the performance of an estimator, we conduct a simulation study. We choose parameter values that are consistent with the graph depicted in Figure 1. The effect of various shape parameter values on the distribution is shown in Figure 1.
The simulations of the AGED are done based on the accept-reject method. Three designs are presented and used to generate random samples from AGED for a parameter considered. The designs for parameters of the AGED considered are , , and for designs 1, 2, and 3, respectively. We use three values of the asymmetry parameter, to cover the cases where the distribution is asymmetric. The realization plot, histogram, and density plot are assessed.
7.1 The acceptance-rejection method
We use a very clever method known as the acceptance-rejection method (39, 40). The acceptance-rejection (A-R) method is one of the standard methods used for generating random samples from distributions (41, 42). We generate a random sample of size hundred thousand from the target density AGED, defined in Eq. 5, and density, which we choose to be the standard normal distribution.
Numerically maximized, there exists a finite constant , such that , and record a maximum value as . Then, define . The acceptance-rejection algorithm is:
1. Generate from the standard normal distribution, , i.e., .
2. Generate from uniform distribution and independent of .
3. If accept as candidate samples; otherwise, reject , and go back to step (1).
4. Repeat step (1) to (3), until is successfully generated.
Figures 5, 6 show the results of the A-R algorithm for the parameter considered. The histograms associated with samples of size hundred thousand generated from AGED and the fitted pdf of AGED to the random samples are illustrated.
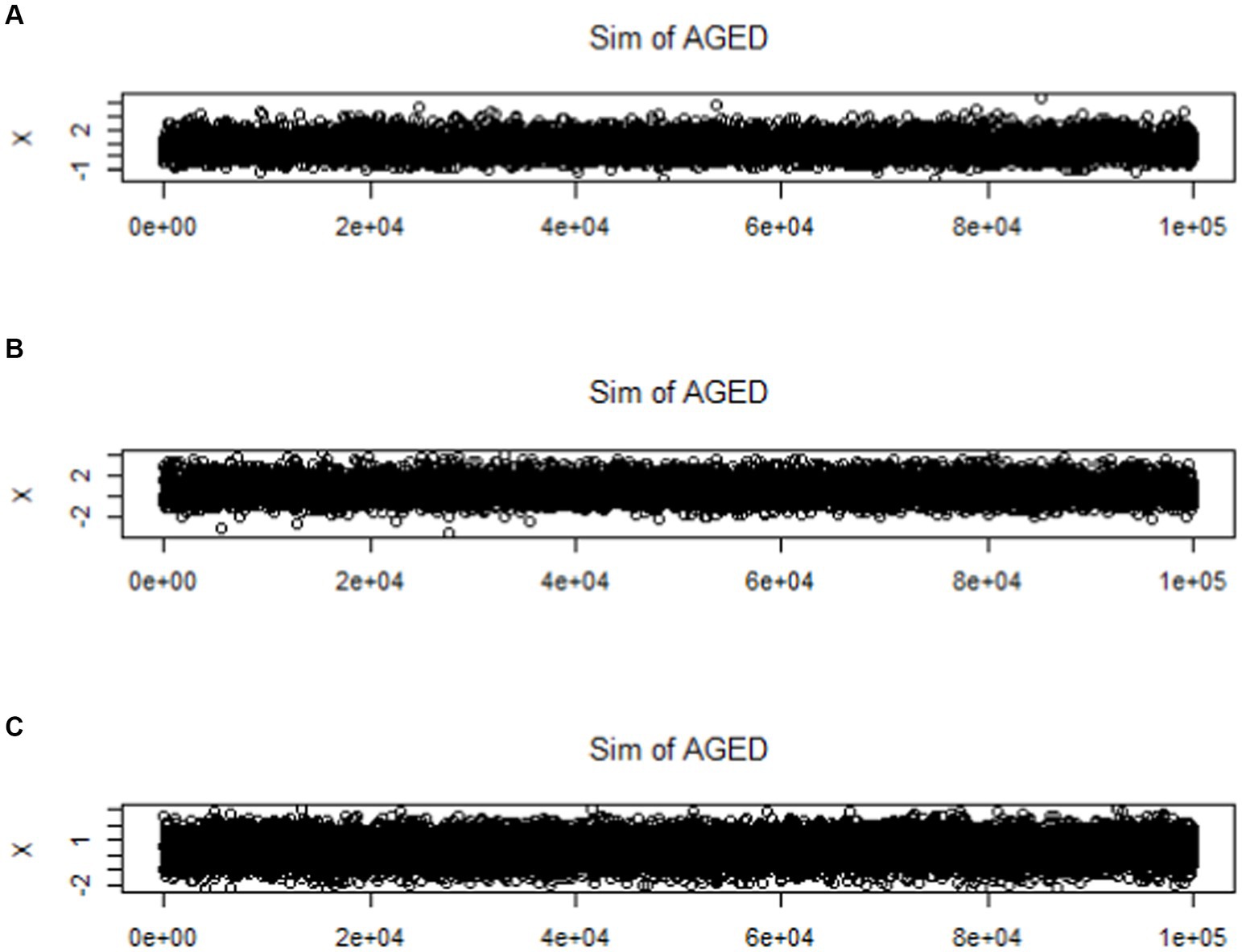
Figure 5. Graphs of realized random samples of size 100,000 taken from AGED with the corresponding pdf (5): (A) design 1, (B) design 2, and (C) design 3.
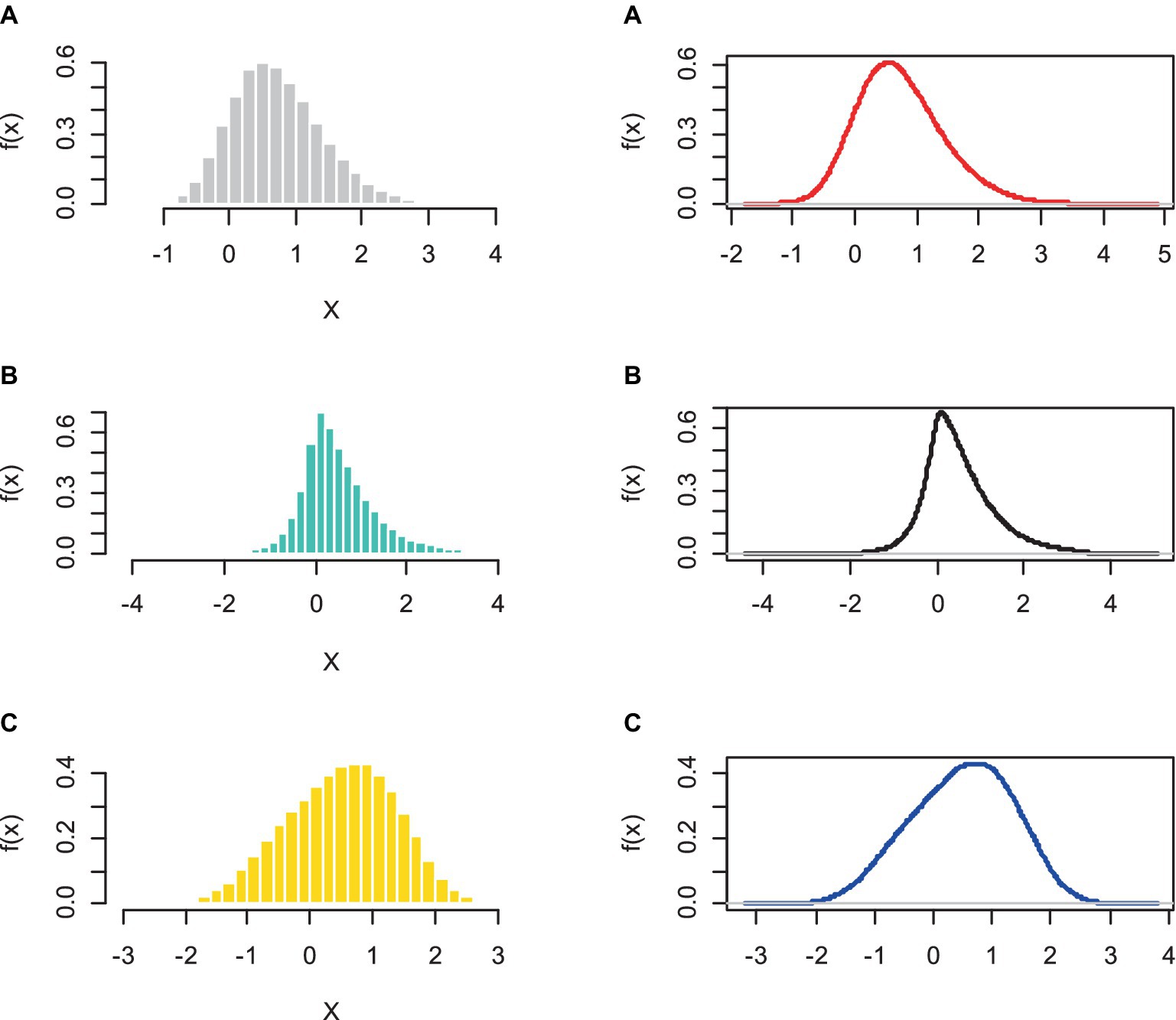
Figure 6. Histograms (left) and density (right) of random samples of size 100,000 taken from AGED with corresponding pdf (5): for (A) design 1, (B) design 2, and (C) design 3, respectively.
The histogram and density of the AGED are plotted. All points under the curve are an accepted random sample and have coordinated distributed AGED. The points above the curve are rejected.
8 Parameter estimation using the MLE method
8.1 Applications—fitting to simulated data
In this section, we study and evaluate the long-term performance of the maximum likelihood estimators (MLEs) of AGED parameters based on finite random samples. Several finite samples of sizes , 500, 1,000, and 100,000 are considered. Three different designs for parameters and are considered. Thus, asymmetry and kurtosis are constructed.
For each sample size and the specified values of the parameters defined in the simulation design, datasets are generated from the AGED, as per Eq. 5. From each dataset, the estimates of the parameters are obtained by the maximum likelihood method. For comparing the performance of the estimators, we use bias and mean square error (MSE) (43).
The average estimates of the parameters, bias, and MSE are calculated using an optimization algorithm in R software. The result verifies the consistency of MLEs. The consistency of MLE can be verified as bias, and the MSE of the estimators is reasonable and diminished for increasing sample size, indicating that estimated values of parameters tend to their true value (Tables 2–4).
8.2 Applications—fitting to real data
In this section, we illustrate the modeling performance of the asymmetric generalized error distribution (AGED) by modeling data with asymmetry and excess kurtosis. Three practical datasets are used to assess the performance of AGED compared to other distributions.
8.2.1 Datasets
Three practical datasets are considered. The first data are the cyber attacks, which are measured as the average length time of cyber attacks per week. It consists of an average time of attacks of 363 weeks and is obtained from (44).
The second dataset is heart failure data. This dataset comprises a substantial number of individuals diagnosed with heart failure and its associated factors, which consists of 304 patients following treatment and was taken from (45). In this respect, we model a number of cholesterol levels in heart failure patients. Statistical measures and the ML estimates of the AGED are obtained and compared with the competing distributions.
The third dataset is reported in (46), which includes 63 observations of the strengths of 1.5 cm of glass fiber, originally obtained from workers at the National Physical Laboratory, England, and used in the work (47). We have utilized these data to present the modeling performance of the AGED compared to other competing distributions. Table 5 reports the summary of data, whereas the goodness of fit (GOF) statistics can be viewed in Tables 6–8.
Some descriptive statistics for the data, including skewness and kurtosis coefficients, are displayed in Table 5, where and denote skewness and kurtosis coefficients, respectively. In this respect, we highlight the peakedness and asymmetry of the data.
Second, different distributions are considered to model these datasets. There are many distributions that have been proposed; however, distributions having special cases for the suggested pdf would be used. The generalized error distribution, the Laplace distribution, and the normal distribution are used to fit the data and are compared with AGED.
We examined the performance of the AGED. Using mostly the prominent goodness-of-fit statistics, Kolmogorov–Smirnov statistics (K-S), consistent Akaike’s information criteria (CAIC), Hannan–Quinn information criteria (HQIC), and Bayesian information criterion (BIC) (48), we compared the competing distribution with AGED.
When the estimates of parameters are computed, we examine via GOF statistics which of the four pdfs is the best fit for the data. The lower those values, the better the fit (48–50). The corresponding maximum likelihood estimates and goodness-of-fit (GOF) statistics are presented in Tables 6–8.
It can be seen that the GOF statistic values of the AGED are lower than those of competing distributions, indicating its superiority in fitting all datasets compared to competing distributions. In light of this, we can conclude that the AGED provides a better fit than a competing distribution.
Figures 7–9 display the histogram of the three practical datasets with the estimated pdf of the AGED along with the competing distributions. The figures show that a closer fit to the data was provided by the AGED for all datasets. In light of this, we can draw the conclusion that the AGED is a better fit for all datasets compared to competing distributions.
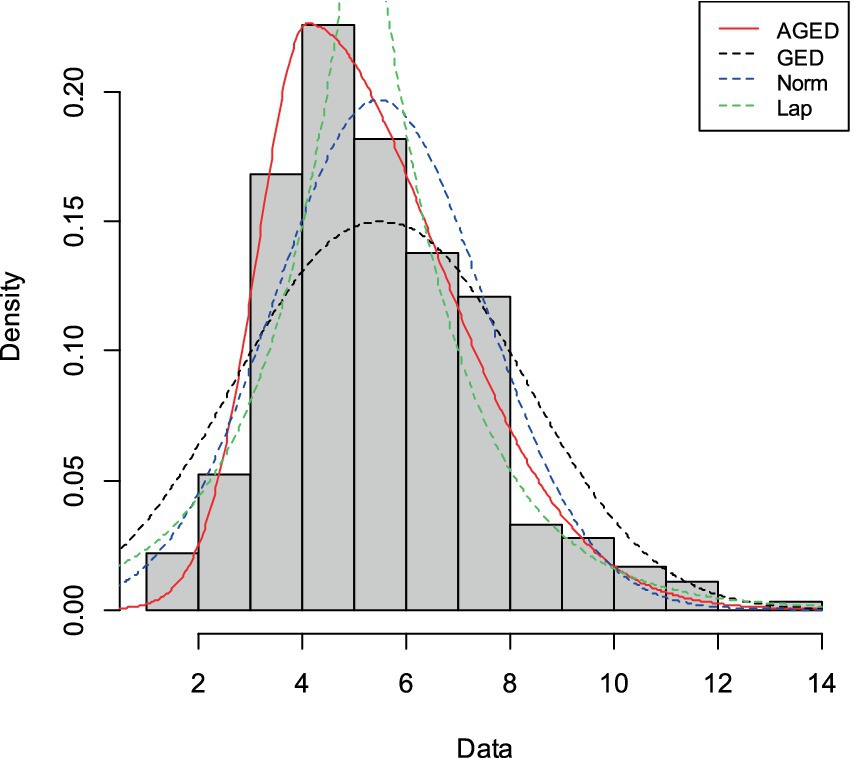
Figure 7. Histogram and fitted probability density function of AGED, GED, Normal, and Laplace distribution for cyber dataset.
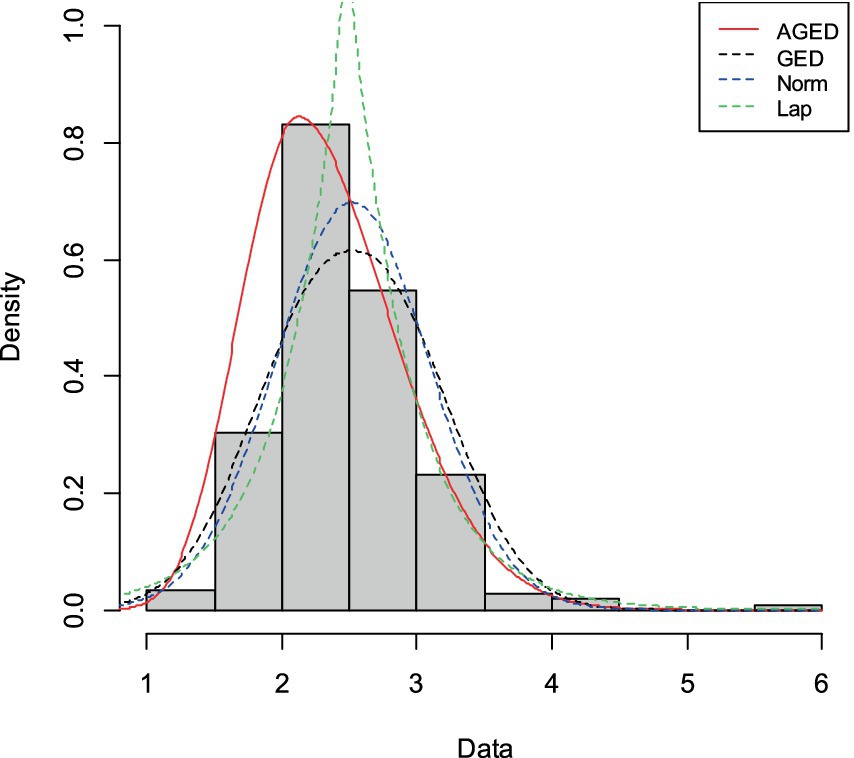
Figure 8. Histogram and fitted probability density function of AGED, GED, Normal, and Laplace distribution for heart failure dataset.
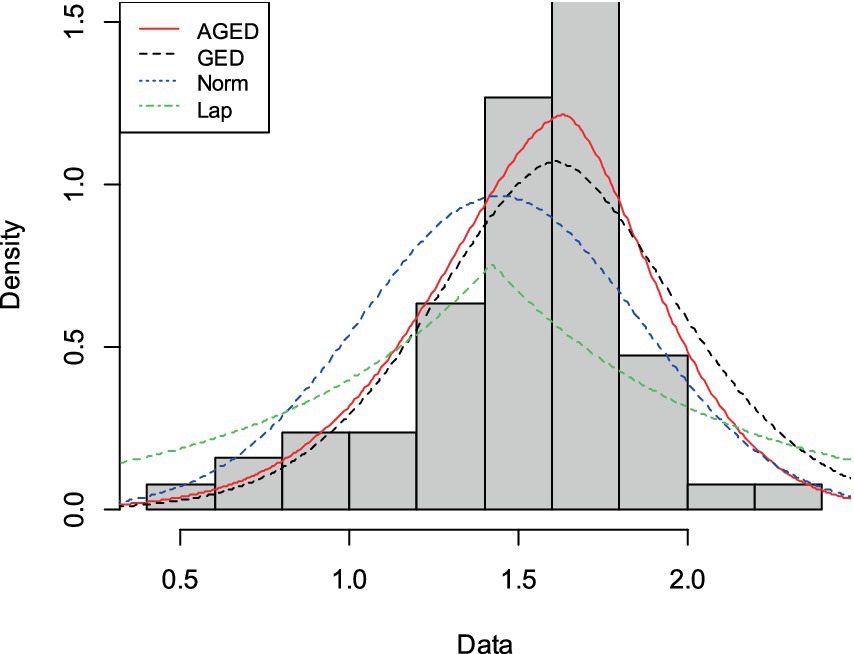
Figure 9. Histogram and fitted probability density function of AGED, GED, Normal, and Laplace distribution for strengths of glass fibers dataset.
For all datasets, Figures 7–9 show that AGED fits better than the competing distributions. In particular, the peakedness can be fitted. The asymmetry illustrated in Table 5 has also been fitted, as unequally distributed histograms around the location in the figures can show that there is an asymmetry in the datasets.
9 Conclusion
The main finding of this study is the derivation of a new probability distribution that reveals interesting properties, especially with various asymmetry and kurtosis behaviors; we call it the asymmetric generalized error distribution (AGED). AGED is a new contribution to the field of statistical theory and provides a more flexible pdf, cdf, and hazard function than the base distribution. The mathematical and statistical features of the distribution are derived and discussed. To estimate the distribution parameters, maximum likelihood estimators are derived. A simulation study is done using the acceptance-rejection algorithm. In the applications, the datasets have high kurtosis and skewness. The criteria indicate that the AGED provides better fits to the datasets. This implies that the new distribution is a good alternative for modeling data with asymmetric and excess kurtosis behavior. We expect that the distribution can be applied to many more real datasets, and the findings of the study can be used as the basis for future research in the field.
Data availability statement
The raw data supporting the conclusions of this article will be made available by the authors, without undue reservation.
Author contributions
TA: Conceptualization, Investigation, Software, Data curation, Formal analysis, Methodology, Visualization, Writing – original draft, Writing – review & editing. AG: Conceptualization, Software, Data curation, Formal analysis, Methodology, Visualization, Writing – original draft, Writing – review & editing.
Funding
The author(s) declare that no financial support was received for the research, authorship, and/or publication of this article.
Acknowledgments
We thank Kotebe University of Education for providing necessary facilities. The authors also gratefully acknowledge the Editor and referees for their insightful comments and constructive suggestions that led to a marked improvement of the article.
Conflict of interest
The authors declare that the research was conducted in the absence of any commercial or financial relationships that could be construed as a potential conflict of interest.
Publisher’s note
All claims expressed in this article are solely those of the authors and do not necessarily represent those of their affiliated organizations, or those of the publisher, the editors and the reviewers. Any product that may be evaluated in this article, or claim that may be made by its manufacturer, is not guaranteed or endorsed by the publisher.
References
1. Cahoy, DO (2015). Some skew-symmetric distributions which include the bimodal ones. Commun Stat Theory Methods 44:554–63. doi: 10.1080/03610926.2012.746986
2. Arellano-Valle, RB, Gómez, HW, and Quintana, FA (2005). Statistical inference for a general class of asymmetric distributions. J Stat Plan Infer 128:427–43. doi: 10.1016/j.jspi.2003.11.014
3. Fernández, C, and Steel, MF (1998). On Bayesian modelling of fat tails and skewness. J Am Stat Assoc 93:359–71. doi: 10.1080/01621459.1998.10474117
4. Abdulah, E, and Elsalloukh, H (2013). Analyzing skewed data with the epsilon skew gamma distribution. J Stat Appl Probab 2:195. doi: 10.12785/jsap/020302
5. Theodossiou, P (2015). Skewed generalized error distribution of financial assets and option pricing. Multinatl Financ J 19:223–66. doi: 10.17578/19-4-1
6. Fagiolo, G, Napoletano, M, and Roventini, A (2008). Are output growth-rate distributions fat-tailed? Some evidence from OECD countries. J Appl Econ 23:639–69. doi: 10.1002/jae.1003
7. Hampel, FR, Ronchetti, EM, Rousseeuw, PJ, and Stahel, WA. (2011). Robust statistics: The approach based on influence functions. New York, USA: John Wiley & Sons.
8. Arellano-Valle, RB, and Genton, MG (2005). On fundamental skew distributions. J Multivar Anal 96:93–116. doi: 10.1016/j.jmva.2004.10.002
9. Weldensea, M. (2019). Bayesian analysis of the epsilon skew exponential power distribution. New York, USA: University of Arkansas at Little Rock.
10. Hutson, AD (2019). An alternative skew exponential power distribution formulation. Commun Stat Theory Methods 48:3005–24. doi: 10.1080/03610926.2018.1473600
11. Jia, P, Liao, X, and Peng, Z (2015). Asymptotic expansions of the moments of extremes from general error distribution. J Math Anal Appl 422:1131–45. doi: 10.1016/j.jmaa.2014.09.030
12. Pewsey, A (2000). Problems of inference for Azzalini’s Skewnormal distribution. J Appl Stat 27:859–70. doi: 10.1080/02664760050120542
13. Azzalini, A, and Valle, AD (1996). The multivariate skew-Normal distribution. Biometrika 83:715–26. doi: 10.1093/biomet/83.4.715
14. Mudholkar, GS, and Hutson, AD (2000). The epsilon–skew–Normal distribution for analyzing near-Normal data. J Stat Plan Infer 83:291–309. doi: 10.1016/S0378-3758(99)00096-8
15. Olmos, NM, Varela, H, Gómez, HW, and Bolfarine, H (2012). An extension of the half-Normal distribution. Stat Pap 53:875–86. doi: 10.1007/s00362-011-0391-4
16. Azzalini, A (1985). A class of distributions which includes the Normal ones. Scand J Stat :171–8. doi: 10.6092/ISSN.1973-2201
17. Azzalini, A (1986). Further results on a class of distributions which includes the Normal ones. Statistica 46:199–208. doi: 10.6092/issn.1973-2201/711
18. Ohagan, A, and Leonard, T (1976). Bayes estimation subject to uncertainty about parameter constraints. Biometrika 63:201–3. doi: 10.1093/biomet/63.1.201
19. Salinas, HS, Arellano-Valle, RB, and Gómez, HW (2007). The extended skew-exponential power distribution and its derivation. Commun Stat Theory Methods 36:1673–89. doi: 10.1080/03610920601126118
20. Diciccio, TJ, and Monti, AC (2004). Inferential aspects of the skew exponential power distribution. J Am Stat Assoc 99:439–50. doi: 10.1198/016214504000000359
21. Evans, M, Hastings, N, and Peacock, B (2001). Statistical distributions. Meas Sci Technol 12:117–7. doi: 10.1088/0957-0233/12/1/702
24. Bottazzi, G, and Secchi, A (2011). A new class of asymmetric exponential power densities with applications to economics and finance. Ind Corp Chang 20:991–1030. doi: 10.1093/icc/dtr036
25. Giller, G. L. (2005). A generalized error distribution. Giller Investments Research. Available at: https://dx.doi.org/10.2139/ssrn.2265027.
26. Davis, C. (2015). The skewed generalized T distribution tree package vignette. Chicago: R Foundation for Statistical Computing.
27. Catherine, F, Merran, E, Nicholas, H, and Brian, P. (2011). Statistical distributions. Hoboken: John Wiley & Sons Inc (2011).
28. Owen, DB (1956). Tables for computing bivariate Normal probabilities. Ann Math Stat 27:1075–90. doi: 10.1214/aoms/1177728074
29. Young, J, and Minder, CE (1974). Algorithm AS 76: an integral useful in calculating non-central T and bivariate Normal probabilities. Appl Stat :455–7. doi: 10.2307/2347148
30. Hill, I (1978). A remark on algorithm AS 76: an integral useful in calculating non-central T and bivariate Normal probabilities. J R Stat Soc C Appl Stat 27:379–9. doi: 10.1111/j.1467-9876.1978.tb01084.x
31. Boys, RJ (1989). Algorithm AS R80: a remark on algorithm AS 76: an integral useful in calculating noncentral T and bivariate Normal probabilities. J R Stat Soc C Appl Stat 38:580–2. doi: 10.2307/2347894
34. Astorga, JM, Reyes, J, Santoro, KI, Venegas, O, and Gómez, HW (2020). A reliability model based on the incomplete generalized Integro-exponential function. Mathematics 8:1537. doi: 10.3390/math8091537
35. Fox, J, Weisberg, S, Adler, D, Bates, D, Baud-Bovy, G, Ellison, S, et al. (2012). Package ‘car’. Vienna: R Found. Statist. Comput. 16:333.
37. Team, RDC. (2010). R: a language and environment for statistical computing. R Foundation for Statistical Computing.
38. Arellano Valle, R. B., Gómez Geraldo, H., and Salinas, H. S. (2013). A note on the fisher information matrix for the skew-generalized-Normal model. SORT-Statistics and Operations Res. Trans. 37, 19–28.
39. Lange, K, Chambers, J, and Eddy, W. (2010). Numerical analysis for statisticians, vol. 1 New York: Springer.
40. Nguyen, N, and Ökten, G (2016). The acceptance-rejection method for low-discrepancy sequences. Monte Carlo Methods Appl 22:133–48. doi: 10.1515/mcma-2016-0104
42. Moskowitz, B, and Caflisch, RE (1996). Smoothness and dimension reduction in quasi-Monte Carlo methods. Math Comput Model 23:37–54. doi: 10.1016/0895-7177(96)00038-6
43. Ahmed, AA, Algamal, ZY, and Albalawi, O (2024). Bias reduction of maximum likelihood estimation in Exponentiated Teissier distribution. Front Appl Math Stat 10:1351651. doi: 10.3389/fams.2024.1351651
44. Kaggle. (2023). Heart Failure Datasets. Available at: https://www.kaggle.com/Datasets/Vaibhavsuman/Heart-Failure-Dataset
45. Gjika, E. Fitting probability distribution in R. Dataset Cyber Data (2021). Available at: https://rpubs.com/Eraldagjika/715261.
46. Smith, RL, and Naylor, J (1987). A comparison of maximum likelihood and Bayesian estimators for the three-parameter Weibull distribution. J R Stat Soc C Appl Stat 36:358–69. doi: 10.2307/2347795
47. Baharith, LA, and Aljuhani, WH (2021). New method for generating new families of distributions. Symmetry 13:726. doi: 10.3390/sym13040726
48. Akaike, H (1974). A new look at the statistical model identification. IEEE Trans Autom Control 19:716–23. doi: 10.1109/TAC.1974.1100705
Keywords: AR algorithms, asymmetric generalized error distribution, generalized error distribution, maximum likelihood estimation, probability, statistics, symmetric distribution
Citation: Abebe TN and Goshu AT (2024) Asymmetric generalized error distribution with its properties and applications. Front. Appl. Math. Stat. 10:1398137. doi: 10.3389/fams.2024.1398137
Edited by:
Han-Ying Liang, Tongji University, ChinaReviewed by:
Zakariya Yahya Algamal, University of Mosul, IraqDiganta Mukherjee, Indian Statistical Institute, India
Copyright © 2024 Abebe and Goshu. This is an open-access article distributed under the terms of the Creative Commons Attribution License (CC BY). The use, distribution or reproduction in other forums is permitted, provided the original author(s) and the copyright owner(s) are credited and that the original publication in this journal is cited, in accordance with accepted academic practice. No use, distribution or reproduction is permitted which does not comply with these terms.
*Correspondence: Tayu Nigusie Abebe, dGF5dXRhdGEyQGdtYWlsLmNvbQ==