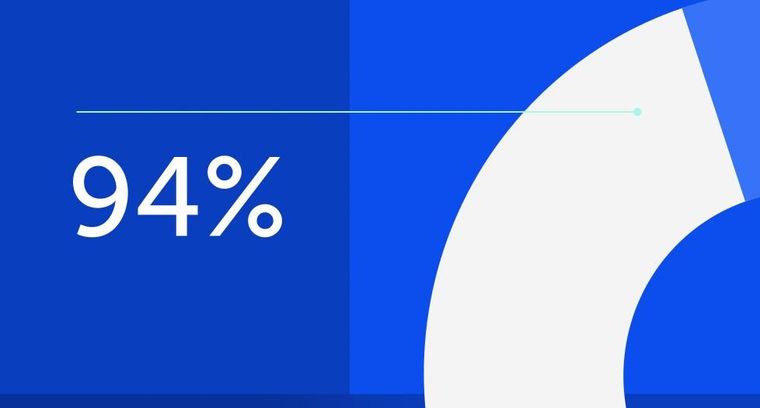
94% of researchers rate our articles as excellent or good
Learn more about the work of our research integrity team to safeguard the quality of each article we publish.
Find out more
ORIGINAL RESEARCH article
Front. Appl. Math. Stat., 22 March 2024
Sec. Mathematical Biology
Volume 10 - 2024 | https://doi.org/10.3389/fams.2024.1376507
This article is part of the Research TopicModeling Ecological and Epidemiological Interactions for Sustainable PracticesView all 5 articles
This study delves into the dynamics of Zika virus transmission by employing a mathematical model to explain virus spread with fractional order derivatives. The population is divided into two groups: the human group and the ticks group to accurately explain the transmission routes of the virus. The objective of this research is to protect susceptible individuals from infection and curb the spread of this endemic disease. To achieve this, we have included two control measures: the first is a sensibilization program, and the second is treatment. We investigate the use of optimal control strategies and fractional derivative techniques under the Caputo method to reduce the number of exposed and infected individuals. By employing the Pontryagin maximum principle to analyze and characterize the optimal controls, the proposed method is further validated through numerical simulations. The outcome of this study highlights the importance of containing the rate of dynamic dissemination in preventing the Zika epidemic.
Zika virus infection is a disease caused by a viral infection that spreads primarily through the bites of infected mosquitoes. While some person may not experience any noticeable symptoms, others may develop fever, rash, joint pain, or conjunctivitis (known as pink eye). The consequences of Zika virus infection can be particularly devastating for pregnant women, as it can lead to severe birth defects such as microcephaly, where a baby is born with an abnormally small head, as well as eye abnormalities. Apart from mosquito bites, the virus can also be transmitted through sexual contact, blood transfusion, and from a pregnant woman to her fetus before or during birth.1 Although symptoms, if any, are generally mild, healthcare professionals often consider a person's symptoms and recent history to suspect Zika virus infection. Vis–vis to confirm the diagnosis, blood or urine tests are usually performed.2
Zika virus infection can be effectively prevented by taking certain precautions. First, it is important to avoid mosquito bites completely. Although there is currently no specific treatment for Zika virus infection, there are measures that can help relieve symptoms.3 Adequate rest, drinking plenty of fluids, and using acetaminophen can help reduce fever and relieve pain. Zika virus spreads in a similar way to other arthropod-borne viruses such as dengue, yellow fever, and chikungunya. It is transmitted primarily by certain types of mosquitoes called Aedes mosquitoes, which lay their eggs in areas with stagnant water. These mosquitoes are particularly active during the day, both indoors and in shaded areas outdoors [1].
The Zika virus was first identified in the Zika Forest of Uganda in 1947. It remained relatively unknown until 2007 when significant outbreaks began occurring in the South Pacific islands. The situation escalated in May 2015 when cases of local transmission were reported in South America, followed by Central America and the Caribbean. Eventually, the virus reached Mexico in late November 2015. Local transmission refers to the transmission of the virus through mosquito bites in areas where people live or work, rather than contracting it during their travels. During the initial week of infection, the Zika virus can be detected in the bloodstream. When an infected person is bitten by a mosquito, the mosquito ingests the virus by feeding on the infected blood. The virus then multiplies within the mosquito's body, allowing it to transmit the virus to another person when it bites again after a few days. Consequently, individuals who have traveled to regions with a high prevalence of Zika virus infection may carry the virus in their bloodstream upon returning home, potentially leading to local transmission of the Zika virus [2].
Zika virus can be spread through various means, including sexual contact, mother-to-child transmission, blood transfusion or organ transplantation, and accidental exposure in laboratories. Understanding these modes of transmission is essential for implementing effective preventive measures against Zika virus [3].
To prevent Zika virus infection, it is important to take precautions and use acetaminophen to treat fever and relieve pain. Zika virus is spread by Aedes mosquitoes, similar to dengue, yellow fever, and chikungunya. These mosquitoes are most active during the day, especially a few hours after sunrise and before sunset. They can also sting at night. Zika virus was first identified in the Zika Forest, Uganda, in 1947, but gained more attention after a large outbreak in the South Pacific islands in 2007. It subsequently spread to South America, Central America, and the Pacific Rim, Caribbean, and Mexico. Local transmission occurs when infected mosquitoes bite people in their living or work areas. While cases have been reported in certain parts of the United States, as of December 2019, no new cases of local transmission have been recorded. However, Zika virus infections have been reported among travelers returning from affected countries. The Centers for Disease Control and Prevention (CDC) permanent diagnosis for travelers is essential [4–7].
The Zika virus has received significant attention because of its potential impact on public health. While many individuals infected with Zika virus do not experience any symptoms, others may develop a range of mild symptoms such as fever, conjunctivitis, joint and muscle pain, headache, and rash. In rare cases, the virus has been linked to the Guillain-Barr syndrome, a neurological disorder characterized by muscle weakness and sensory abnormalities. One of the most troubling aspects of Zika virus infection is its potential to cause serious complications in pregnant women and their unborn babies. In addition, children infected with the virus before birth may face various developmental challenges, including delayed speech and motor skills, intellectual disabilities, seizures, and movement difficulties [8, 9].
A number of contributions have been published in related topics. Tesla et al. [10] studied temperature drives Zika virus transmission: evidence from empirical and mathematical models. Agusto et al. [11] considered a mathematical model for Zika virus dynamics with sexual transmission route. Khan et al. [9] studied a dynamical model of asymptomatic carrier Zika virus with optimal control strategies. By replacing the ordinary normal equation with a fractional derivative equation, the fractional derivatives approach achieves an important goal of controlling the fact that the system dynamics are affected by memory. Ahmed et al. [12] studied equilibrium points, stability, and numerical solutions of fractional-order predator–prey and rabies models. Zeb et al. [13] studied the optimal campaign strategies in fractional-order smoking dynamics. Sardar et al. [14] proposed a mathematical model of dengue transmission with memory. Kouidere et al. [15] studied the analysis and optimal control of a mathematical modeling of the spread of African swine fever virus with a case study of South Korea and cost-effectiveness. Raza et al. studied and proposed the number model in Zika virus and the epedmic model related in it [16–20]. And papers related it [21–33].
Considering the above, they did not take into account the ability of the memory to contain the spreading of the Zika virus, as well as the transmission of the Zika virus model to the fractional derivative.
Among the models used for epidemic analysis of Zika virus, the majority was formulated using ODE's, while others were based on fractional calculus. Recall that the fractional calculus is applied in different directions of physics, mathematical biology, fluid mechanics, electrochemistry, signal processing, viscoelasticity, finance, and many others. Fractional derivatives were used in the literature to monitor the effect of memory on the system dynamics by replacing the normal derivative arrangement with a fractional derivative arrangement. In epidemic modeling, fractional derivatives and fractional integrals are important aspects, because the effect of memory plays an important role in the spread of the disease. The presence of memory effects on past events will affect the spread of the disease in the future so that the disease can be controlled in the future, and the distance of the memory effect indicates the date of the disease spread. Thus, the effects of memory on the spread of infectious diseases can be verified using fractional derivatives. The fractional calculus adds an extra dimension in the study of dynamics of epidemiological models, especially for COVID-19 pandemic. Therefore, the fractional version of many epidemic models has been investigated as in Ahmed et al. [12] and Khan and Atangana [34].
This article is organized using the following methodology: in the first section, we present some basic characteristics and definitions of the integral and fractional derivatives. Because of its applicability to the initial conditions of differential equations, we will apply Caputo's definition to fractional order differentiation. In Section 2, we describe the problem of optimal command on the basis of the suggested model, and we also characterize the terms of optimal command by utilizing the principle of Pontryagin's maximum. In Section 4, we performed some computational simulations. Finally, in Section 5, the article is terminated.
A mathematical model SPEPIPRPSMIM is considered, which represents the spread of the Zika virus through a population. As a result, we divided the N population into six compartments: To begin, the people susceptible SP, the people exposed EP, the people infected IP, the people recovered RP, the ticks susceptible SM, and the ticks infected IM.
In Figure 1, the considered model is graphically represented.
As a result, we present the fractional optimal command mathematical model of the Zika virus, which is governed by the system of differential equations shown below Equation 1:
where SP(0) ≥ 0, EP(0) ≥ 0, IP(0) ≥ 0, RP(0) ≥ 0, ST(0) ≥ 0 and IT(0) ≥ 0 are the initial state.
With
- ξP : recruitment rate of people susceptible.
- ξT : ratio of susceptible Zika being recruited.
- μP : death ratio in the population from natural causes.
- μT : death ratio in Zika due to natural causes.
- β1: infection ratio among people through contact with exposed people.
- β2: contamination ratio among people through contact with infected individuals.
- β3: infection ratio among people through contact with infected Zika.
- β4: infection rate among Zika through contact with infected individuals.
- α: the ratio of exposed becoming infected with the virus.
- σ: the ratio of infected become recovered from the virus.
- δ: death ratio caused by complications.
Our strategy for managing the Zika virus focuses on reducing its spread among the population. The aims is to minimize both the number of individuals exposed to the virus and the number of those who become infected. To accomplish this, we developed a program that employs two distinct controls, denoted as w1(t) and w2(t), for a time period of tinlbrack [0, T]. The control w1(t) represents an awareness campaign launched at a specific time t, whereas the control w2(t) represents treatment options available at the same time t.
with SP(0) ≥ 0, EP(0) ≥ 0, IP(0) ≥ 0, RP(0) ≥ 0, ST(0) ≥ 0 and IT(0) ≥ 0 are the initial state (Equation 3).
In this equation, we assign relative weights to the cost of implementing the awareness program and treatment controls using parameters A and B, where A and B are positive numbers. These weights assist us in determining the relative significance of the controls w1(t) and w2(t) at a given time t. To capture the non-linear interactions that arise from high levels of implementation, we use a quadratic cost function for the controls. This cost function includes the financial resources required for the treatment and implementation of an awareness campaign. The final time period in the implementation of the controls is represented by T.
To put it differently, we aim to find the optimal controls and that would minimize the cost function and meet the objectives of controlling the spread of the Zika virus.
To put it another way, we want to find the optimal controls and that minimize the cost function (Equation 4), while also controlling the spread of the Zika virus.
Where U is the set of admissible commands defined by U = {(w1, w2)/0 ≤ w1min ≤ w1i(t) ≤ w1max ≤ 1, 0 ≤ w2min ≤ w2i(t) ≤ w2max ≤ 1, /t ∈ [0, T]}.
The primary objective in FOCPs is to determine the optimal command pair (w1, w2) by minimizing the objective function:
Subject to the constraint
The following expression defines a modified objective function (Equation 6):
where H(SP, EP, IP, RP, ST, IT, w1, w2, t)
From Equations (5, 7), we can derive the following
With the transversality conditions at time T :
Equations (8, 9) outline the required conditions for the previously defined FOCP in terms of a Hamiltonian.
These conditions generate a series of fractional differential equations defined by the state variables SP, EP, IP, RP, ST, IT and commands w1, w2, and solving for the Lagrange multiplier ξi can be accomplished through analytical, numerical, or a blended approach.
The Pontryagain's principle [35] to solve the optimal commands problem is given below. This method converted this into a problem of minimizing a Hamiltonian H(t) at time t (Equation 10), which is defined by
That fi is the right side of the differential equation of the ith state variable of the system at time t.
Theorem 1. The optimals commands and the solutions , , , , and of the corresponding co-state system (Equation 2) are given, the adjoint variables ξ1(t), λ2(t), ξ3(t), ξ4(t), ξ5(t), and ξ6(t)satisfying:
We find the transversality conditions at time T: ξ1(T) = 0, λ2(T) = −1, ξ3(T) = −1, ξ4(T) = 0; ξ5(T) = 0 and ξ6(T) = 0.
Furthermore, for t ∈ [0, T], the optimal controls and are given by
Proof. Here, we applied the Pontryagain's principle [35] to solve the optimal command problem given below.
So, we defined the Hamiltonian H by Equation 13:
For t ∈ [0, T], the adjoint equations and transversality conditions can be obtained by using the Pontryagin's maximum principle [35–38] such that
With the transversality conditions at time T: ξ1(T) = 0, λ2(T) = −1, ξ3(T) = −1, ξ4(T) = 0; ξ5(T) = 0 and ξ6(T) = 0.
For, t ∈ [0, T] the optimal commands w1 and w2 can be solved from the optimality condition
We have
By the bounds in U of the commands, it is easy to obtain and , which are given by Equations (11, 12) in the form of system (Equation 8).
This section delves into the outcomes obtained through the numerical resolution of the optimality system. In our problem of control, the state variables have initial conditions and the adjoint conditions have terminal conditions. In other words, the optimality system is a boundary problem with two points and distinct boundary conditions for the time steps i = t0 and i = tf. The optimality problem is resolved iteratively, in which the state system is progressed forward and the adjoint system is progressed backward. We initially estimate the controls during the first iteration and subsequently update them using characterization before the next iteration commences. We proceed until we reach the convergence of the successive iterations. An optimality program is compiled and written in MATLAB according to the data below.
As the control and state functions have different magnitudes, the weight constant is set to the following values: A = 100 and B = 100.
In this scenario, we use any control.
Based on Figure 2 and the parameter values in Table 1, we noted an increase in the number of exposed and infected people with the Zika virus, which leads to an increase in the number of deaths due to the lack of any strategy to contain the disease and prevent its spread.
In this scenario, we use only the optimal control w1(t).
The number of people exposed to and infected with the Zika virus has decreased, as shown in Figure 2. Due to the implementation of the strategy, sensitization campaigns will be conducted for people who are likely to be infected. Moreover, due to the risks of mixing with people infected with the virus, this biological strategy aims to prevent the spread of the disease. The number of people exposed without control ranges from 150 (alpha = 0.3) to 3.03105 (alpha = 0.6) and from 150 (alpha = 0.4) to 3.42106 (alpha = 0.4) with control. The number of people infected without treatment ranges from 50* (alpha = 0.6) to 9.06, 104 (alpha = 0.3) with treatment, and from 50 (alpha = 0.4) to 8.14, 104 (alpha = 0.4) without treatment.
On the other hand, the fractional derivatives have a significant influence on the description of memory effects in dynamic systems. Memory's effects diminish as alpha approaches 1. Furthermore, in ordinary differential equations, the derivative order fractional alpha acts as a time delay. Figure 3 shows that the memory effect of the system increases when the order of the derivative alpha is equal to 1, and as a result, the number of infected people decreases over time.
Figure 3. The evolution of the number of exposed and infected people without and with control w1(t).
In this scenario, we use only the optimal control w2(t).
In Figure 4, we notice an important decrease in the number of people infected with a virus, that after 150 days, the number of exposed without control is 150 (α = 0.3) to 1.85.106 (α = 0.6) and 150 (α = 0.4) to 2.61.106 (α = 0.4) with control. The number of infected without control is 50 (α = 0.6) to 4.32.104 (α = 0.3) and 50 (α = 0.4) to 6, 04.104 (α = 0.4) with control. After applying this strategy of treatment as a vaccine and other treatments and quarantines, which will have important results in limiting the spread of the virus, the results were positive.
Figure 4. The evolution of the number of infected pigs and ticks susceptible to infection with and without controls w2(t).
Our study has successfully employed fractal derivatives to establish a theoretical and computational analysis for a mathematical model of Zika transmission through a population. The model is a fractional mathematical model called SPEPIPRPSTIT, and it depicts the spread of the Zika virus throughout the population, which contained two controls. Our study has effectively integrated two control measures, an awareness program and a treatment, into the mathematical model of Zika transmission.
We have also conducted a thorough investigation of the optimal control strategy, which aims to minimize the spread of the virus by reducing the number of exposed and infected individuals. Utilizing the principles of control theory, we were able to derive the characterization of optimal control. The numerical simulations of our results have confirmed the effectiveness of the proposed containment strategies. Our research has conclusively shown that controlling the rate of dynamic dissemination is critical in containing the Zika epidemic.
The raw data supporting the conclusions of this article will be made available by the authors, without undue reservation.
AK: Data curation, Methodology, Validation, Writing – original draft, Writing – review & editing. AE: Writing – original draft, Writing – review & editing. IM: Writing – original draft, Writing – review & editing. OB: Writing – original draft, Writing – review & editing. KA: Writing – original draft, Writing – review & editing.
The author(s) declare that no financial support was received for the research, authorship, and/or publication of this article.
The authors declare that the research was conducted in the absence of any commercial or financial relationships that could be construed as a potential conflict of interest.
All claims expressed in this article are solely those of the authors and do not necessarily represent those of their affiliated organizations, or those of the publisher, the editors and the reviewers. Any product that may be evaluated in this article, or claim that may be made by its manufacturer, is not guaranteed or endorsed by the publisher.
1. ^https://www.who.int/news-room/fact-sheets/detail/zika-virus?gclid=Cj0KCQjwuLShBhC_ARIsAFod4fKV2P6U3A_KwDudnmZawXdqhB7fwGDuSd412P5HnQHhJKP9_dh_gP8aAsMPEALw_wcB
2. ^https://www.who.int/westernpacific/health-topics/zika-virus-disease
3. ^https://www.who.int/news-room/feature-stories/detail/the-history-of-zika-virus
1. Yun S-I, Lee Y-M. Zika virus: an emerging flavivirus. J Microbiol. (2017) 55:204–19. doi: 10.1007/s12275-017-7063-6
2. Rezapour S, Mohammadi H, Jajarmi A. A new mathematical model for Zika virus transmission. Adv Diff Equ. (2020) 2020:1–15. doi: 10.1186/s13662-020-03044-7
3. Bjornstad ON, Shea K, Krzywinski M, Altman N. The SEIRS model for infectious disease dynamics. Nat Methods. (2020) 17:557–8. doi: 10.1038/s41592-020-0856-2
4. Iqbal S, Baleanu D, Javaid Ali HM, Younas M, Riaz B. Fractional analysis of dynamical novel COVID-19 by semi-analytical technique. Comput Model Eng Sci. (2021) 29:705–27. doi: 10.32604/cmes.2021.015375
5. Akbari N, Asheghi R. Optimal control of an HIV infection model with logistic growth, celluar and homural immune response, cure rate and cell-to-cell spread. Bound Value Probl. (2022) 2022:5. doi: 10.1186/s13661-022-01586-1
6. Gonzlez-Parra G, Daz-Rodrguez M, Arenas AJ. Optimization of the controls against the spread of Zika virus in populations. Computation. (2020) 8:76. doi: 10.3390/computation8030076
7. John Hodgkiss H, Man SH. Stock density and mortality assessment of Sarotherodon mossambicus (Cichlidae) in Plover Cove Reservoir, Hong Kong. Environ Biol Fishes. (1977) 1:171–80. doi: 10.1007/BF00000408
8. Elhia M, Rachik M, Benlahmar E. Optimal control of an SIR model with delay in state and control variables. ISRN Biomath. (2013) 2013:403549. doi: 10.1155/2013/403549
9. Khan MA, Wasim Shah A, Ullah S, Gmez-Aguilar JF. A dynamical model of asymptomatic carrier Zika virus with optimal control strategies. Nonlinear Anal Real World Appl. (2019) 50:144–70. doi: 10.1016/j.nonrwa.2019.04.006
10. Tesla B, Demakovsky LR, Mordecai EA, Ryan SJ, Bonds MH, Ngonghala CN, et al. Temperature drives Zika virus transmission: evidence from empirical and mathematical models. Proc R Soc B. (2018) 285:20180795. doi: 10.1098/rspb.2018.0795
11. Agusto FB, Bewick S, Fagan WF. Mathematical model for Zika virus dynamics with sexual transmission route. Ecol Complex. (2017) 29:61–81. doi: 10.1016/j.ecocom.2016.12.007
12. Ahmed E, El-Sayed A, El-Saka HA. Equilibrium points stability and numerical solutions of fractional-order predator–prey and rabies models. J Math Anal Appl. (2007) 325:542–53. doi: 10.1016/j.jmaa.2006.01.087
13. Zeb A, Zaman G, Jung IH, Khan M. Optimal campaign strategies in fractional-order smoking dynamics. Z Naturforschung A. (2014) 69:225–31. doi: 10.5560/zna.2014-0020
14. Sardar T, Rana S, Chattopadhyay J. A mathematical model of dengue transmission with memory. Commun Nonlinear Sci Numer Simul. (2015) 22:511–25. doi: 10.1016/j.cnsns.2014.08.009
15. Kouidere A, Balatif O, Rachik M. Analysis and optimal control of a mathematical modeling of the spread of African swine fever virus with a case study of South Korea and cost-effectiveness. Chaos, Solitons & Fractals. (2021) 146:110867. doi: 10.1016/j.chaos.2021.110867
16. Alfwzan WF, Raza A, Martin-VaqueroJ, Baleanu D, Rafiq M, Ahmed N, et al. Modeling and transmission dynamics of Zika virus through efficient numerical method. AIP Advan. (2023) 13:095221. doi: 10.1063/5.0168945
17. Raza A. Mathematical modelling of rotavirus disease through efficient methods. Comput Mater Contin. (2022) 72:4727–40. doi: 10.32604/cmc.2022.027044
18. Raza A, Arif MS, Rafiq MA. reliable numerical analysis for stochastic dengue epidemic model with incubation period of virus. Adv Differ Equ. (2019) 2019:1–19. doi: 10.1186/s13662-019-1958-y
19. Raza A, Rafiq M, Awrejcewicz J, Ahmed N, Mohsin M. Dynamical analysis of coronavirus disease with crowding effect, and vaccination: a study of third strain. Nonlinear Dyn. (2022) 107:3963–82. doi: 10.1007/s11071-021-07108-5
20. Raza A, Awrejcewicz J, Rafiq M, Ahmed N, Mohsin M. Stochastic analysis of nonlinear cancer disease model through virotherapy and computational methods. Mathematics. (2022) 10:368. doi: 10.3390/math10030368
21. Boyce WE, DiPrima RC. Elementary Differential Equations and Boundary Value Problems. New York, NY: John Wiley & Sons. (2009).
22. Birkhoff G, Rota GC. Ordinary Differential Equations, 4th edition. New York, NY: John Wiley & Sons (1989).
23. Beltrán-Alcrudo D, Arias M, Gallardo C, Kramer S, Penrith ML. African Swine Fever: Detection and Diagnosis – A Manual for Veterinarians. FAO Animal Production and Health Manual No. 19. Rome. Food and Agriculture Organization of the United Nations (2017). p. 88.
24. Bani-Yaghoub M, Gautam R, Shuai Z, van den Driessche P, Ivanek R. Reproduction numbers for infections with free-living pathogens growing in the environment. J Biol Dyn. (2012) 6:923–40. doi: 10.1080/17513758.2012.693206
25. Driessche PV, Watmough J. Reproduction numbers and sub-threshold endemic equilibria for compartmental models of disease transmission. Math Biosci. (2002) 180:29–48. doi: 10.1016/S0025-5564(02)00108-6
26. LaSalle JP. The stability of dynamical systems. In: Regional Conference Series in Applied Mathematics, Vol. 25. Philadelphia, PA: SIAM (1976).
27. Gumel AB, Shivakumar PN, Sahai BMA. mathematical model for the dynamics of HIV-1 during the typical course of infection. Nonlinear Anal. (2001) 47:17731783. doi: 10.1016/S0362-546X(01)00309-1
28. Karrakchou J, Rachik M, Gourari S. Optimal control and infectiology: application to an HIV/AIDS model. Appl Math Comput. (2006) 177:807818. doi: 10.1016/j.amc.2005.11.092
29. Agrawal OP. Formulation of Euler–Lagrange equations for fractional variational problems. J Math Anal Appl. (2002) 272:368–79. doi: 10.1016/S0022-247X(02)00180-4
30. Podlubny I. Fractional Differential Equations, Vol. 198 of Mathematics in Science and Engineering. San Diego, CA: Academic Press (1999).
31. Miller S, Ross B. An Introduction to the Fractional Calculus and Fractional Differential Equations. Hoboken, NJ: Wiley (1993). p. 480.
33. Fleming WH, Rishel RW. Deterministic and Stochastic Optimal Control. New York, NY: Springer (1975). doi: 10.1007/978-1-4612-6380-7
34. Khan MA, Atangana A. Modeling the dynamics of novel coronavirus (2019-nCoV) with fractional derivative. Alexandria Eng J. (2020) 599:2379–89. doi: 10.1016/j.aej.2020.02.033
35. Pontryagin LS, Boltyanskii VG, Gamkrelidze RV, Mishchenko EF. The Mathematical Theory of Optimal Processes. New York, NY: Wiley (1962).
36. Kouidere A, Balatif O, Ferjouchia H, Boutayeb A, Rachik M. Optimal control strategy for a discrete time to the dynamics of a population of diabetics with highlighting the impact of living environment. Discrete Dyn Nat Soc. (2019) 2019:5949303. doi: 10.1155/2019/6342169
37. Kada D, Kouidere A, Balatif O, Rachik M, Labriji EH. Mathematical modeling of the spread of COVID-19 among different age groups in Morocco: optimal control approach for intervention strategies. Chaos Solit Fractals. (2020) 141:110437. doi: 10.1016/j.chaos.2020.110437
38. Kouidere A, Kada D, Balatif O, Rachik M, Naim M. Optimal control approach of a mathematical modeling with multiple delays of the negative impact of delays in applying preventive precautions against the spread of the COVID-19 pandemic with a case study of Brazil and cost-effectiveness. Chaos Solit Fractals. (2020) 142:110438. doi: 10.1016/j.chaos.2020.110438
Keywords: Zika virus, optimal control, mathematical model, fractional-order model, epidemic
Citation: Kouidere A, El Bhih A, Minifi I, Balatif O and Adnaoui K (2024) Optimal control problem for mathematical modeling of Zika virus transmission using fractional order derivatives. Front. Appl. Math. Stat. 10:1376507. doi: 10.3389/fams.2024.1376507
Received: 25 January 2024; Accepted: 04 March 2024;
Published: 22 March 2024.
Edited by:
Sayooj Aby Jose, Phuket Rajabhat University, ThailandReviewed by:
Ali Raza, Government of Punjab, PakistanCopyright © 2024 Kouidere, El Bhih, Minifi, Balatif and Adnaoui. This is an open-access article distributed under the terms of the Creative Commons Attribution License (CC BY). The use, distribution or reproduction in other forums is permitted, provided the original author(s) and the copyright owner(s) are credited and that the original publication in this journal is cited, in accordance with accepted academic practice. No use, distribution or reproduction is permitted which does not comply with these terms.
*Correspondence: Abdelfatah Kouidere, a291aWRlcmU4OUBnbWFpbC5jb20=; Amine El Bhih, ZWxiaGloYW1pbmVAZ21haWwuY29t
†These authors have contributed equally to this work
Disclaimer: All claims expressed in this article are solely those of the authors and do not necessarily represent those of their affiliated organizations, or those of the publisher, the editors and the reviewers. Any product that may be evaluated in this article or claim that may be made by its manufacturer is not guaranteed or endorsed by the publisher.
Research integrity at Frontiers
Learn more about the work of our research integrity team to safeguard the quality of each article we publish.