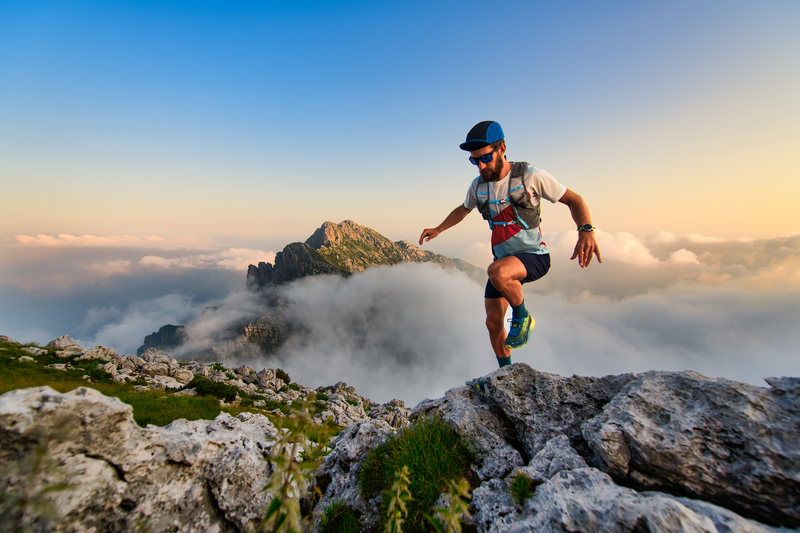
94% of researchers rate our articles as excellent or good
Learn more about the work of our research integrity team to safeguard the quality of each article we publish.
Find out more
METHODS article
Front. Appl. Math. Stat. , 17 October 2022
Sec. Statistics and Probability
Volume 8 - 2022 | https://doi.org/10.3389/fams.2022.969068
Nonresponse is a major problem encountered by surveyors when conducting sampling surveys. The present study suggested a naïve modified Searls method for the elevated estimation of the population mean of the primary variable under investigation by utilizing the known auxiliary parameters. The bias along with the mean squared error (MSE) of the introduced estimator is calculated up to the approximation of the first order. We compared the presented estimator with a competing usual unbiased estimator and other competing population mean estimators regarding the issue of nonresponse. The efficiency criteria of the introduced estimator outperforming the other estimators in the competition are determined and verified using five real data sets. The MSEs for the introduced estimator and the other estimators in the competition are calculated for the five considered populations. The estimator with the least MSE or highest percentage relative efficiency (PRE) is recommended for practical exercise in different areas of applications.
Because of time and financial constraints, sampling is important when the population is big. Furthermore, many sampling studies use the mail questionnaire to acquire factual information due to financial constraints. The problem of nonresponse is common with mail questionnaires, and unexplained bias might be a major factor in these cases. Because of nonresponse, surveys may produce estimates that are biased with large variance. Nonresponse in a sample survey occurs when the inquirer is not able to get observations from some of the units of the population for a variety of reasons, including when someone refuses to answer, when respondents are unavailable, and the possibility that the respondents did not get the question due to a lack of interest or an inability to comprehend what was asked in the questionnaire. As a result, the researcher must be extra cautious when creating the survey questionnaire to ensure that such inaccuracies are minimized. Personal interviews often produce a more full response, although they are more expensive than the postal questionnaire approach. The goal of the investigator is to provide a system that incorporates the advantages of both strategies. When combining both strategies, the questionnaire form of the survey is typically addressed to larger targeted respondents rather than the size in need in the hope that the total returned forms will be larger than expected [1].
The most suitable estimator for any parameter under consideration is the related statistic when it comes to population parameter estimation. The sample mean of the primary variable, Y, for example, is the best estimator of the population mean, Ȳ. Although the estimator ȳ is unbiased for Ȳ, it has a significant sampling variance. As a result, we probed for a biased estimator with a lower mean squared error (MSE). This goal of finding a more efficient estimator is acquired by using an auxiliary variable that is highly associated with the main variable under study. When auxiliary variable,X is combined with data on Y to improve Ȳ estimation, it is expected that each sample unit has complete fact-based information on both variables and that they are accurately measured. However, it is a typical observation in many surveys that nonresponse happens in a variety of ways. Nonresponse can be caused by the refusal of the respondent to answer a specific question, their non-availability, information loss because of the negligence of the investigator or an accident, and lost observations. The most typical strategy for dealing with nonresponse to questionnaires is to have a personal interview with these nonrespondents in order to gather the maximum possible information. Many authors helped estimate Ȳ utilizing X with non-response for various nonresponse scenarios on Y and X.
Using mailed questionnaires, El-Badry [2] advocated estimating Ȳ of a research variate. Estimation of Ȳ through ratio, product, and regression estimators in the no response condition was examined by Rao and Khare and Srivastava [3–5]. Tracy and Osahan [6] explored how to get a better estimate of Ȳ with no response on Y and X. For the no response issue, [7] worked on a regression estimator for estimating Ȳ while [8] proposed an advanced estimation of Ȳ. Under the challenge of non-respond, [9] worked on a general class of exponential ratio estimators of Ȳ using transformed X. In a survey sample with replacement strategy, [10] proposed the estimation of Ȳ for random non-respond. In the context of nonrespond, [11] introduced an elevated ratio estimator for estimating Ȳ. For the issue of non-respond, [12] proposed an exponential type generalized estimator of Ȳ. While some observations on Y and X are missing, [13, 14] investigated the challenge of estimating the ratio of two Ȳ in survey sampling. In case of nonresponse, [15, 16] proposed employing multi-auxiliary characteristics to improve estimation of the ratio of the two Ȳ.
The estimation of Ȳ in a multi-phase sampling technique with nonresponse error was carried out by Srinath [17]. In a two-phase sampling design, [18, 19] proposed enhanced estimators of Ȳ with the problem of nonresponse. Shabbir and Khan [20] investigated estimating Ȳ, utilizing a known couple of X in double sampling in the presence of nonresponse. In a double sampling scheme, Yadav et al. [21] investigated the improved Ȳ estimation, utilizing the known parameters of Ȳ in the case of nonresponse and measurement errors. To overcome the challenge of measurement errors in sample surveys, Sud and Srivastava [22] and Srivastava and Shalabh [23] introduced the modified ratio and the regression type estimators of Ȳ. Kumar et al. [24] and Kumar [25] investigated the challenge of estimating Ȳ in the case of nonresponse and measurement error, and Singh et al. [26] worked on the same issue. In the case of nonrespose on Y along with X, [27] used the modified estimators with exponential functions for estimating Ȳ. Yadav et al. [28] worked on the problem of estimating Ȳ using information from highly correlated X in the face of nonresponse on one or both of the variables and proposed an improved estimator in three different nonresponse scenarios. Sharma and Kumar [29] proposed an estimator for estimating the Ȳ, with known parameters of X in sample surveys in the case of nonresponse. Unal and Kadilar [27] proposed the estimators class for estimating Ȳ, utilizing exponential function in the nonresponse condition for a couple of cases. In the condition of nonresponse on Y and X, Unal and Kadilar [27] adopted an exponential type estimator for Ȳ. Unal and Kadsilar [30] introduced a new class of exponential estimators in the case of nonresponse and Unal and Kadilar [31] worked on a novel population mean estimator for the nonresponse problem. Jaiswal et al. [32] suggested an elevated procedure for the estimation of population mean in the case of nonresponse. Some recent contributions for elevated estimation of Ȳ for the nonresponse problem were made by Ahmed at al. [33], Yadav et al. [34], Ahmed and Shabir [35], Hussain et al. [36], Unal and Kadilar [30], and Ahmad et al. [37].
The research studies showed that the estimators are getting better, as evidenced by decreasing MSE. The estimator with the sampling distribution having lower MSE was closer to the genuine, Ȳ. Thpresent investigation aimed to create an estimator that may enhance the true Ȳ estimation when there is a nonresponse error. We suggested a naive estimator of Ȳ, utilizing known information on X in the case of nonresponse error. We also noticed the large sample properties of the suggested estimator up to the first-degree approximation. The remainder of this study is laid out as below: The review of competing estimators is presented in Section Review of competing estimators, and introduced estimators are discussed in Section Proposed class of estimators. The suggested estimator is detailed in Section Efficiency conditions, while the efficiency comparison is discussed in Section Numerical illustrations. The numerical investigation is described in Section Simulation study, and the results and comments are presented in Sections Results and discussion and Conculsion, respectively.
Hansen and Hurwitz [1] worked on the issue of nonresponse by introducing a novel subsampling procedure for the units which have not responded. In their technique, they considered that the population U = (U1, U2, ..., UN) is the N finite and identifiable population units such that N = N1 + N2, where N1 are the number of units with response and N2 are the units with no response in the population respectively. Further a sample having n units is taken from the population under investigation using simple random sampling without replacement (SRSWOR) procedure. This sample is such that, n = n1 + n2, where n1 have given response and n2 has not given response in the sample. Let Y be the main characteristic under investigation and X as auxiliary variable. Further (yi, xi) are values on the ith(i = 1, 2, ..., N) observations on Y and X for the population and a sub-sample of size random observations from n2 nonrespondents are taken with h as inverse sampling ratio for the sample from the second phase. The population's proportions of responders and nonresponders are, and respectively.
In the context of the nonresponse issue, Hansen and Hurwitz [1] suggested the unbiased estimator of Ȳ as,
Where, and are the sample proportions of responders and nonresponders respectively with the ȳ1 and ȳ2(r) as the sample means for n1 and r units for the respondents and nonrespondents respectively.
The sampling variance of t1 for the approximation of first order is,
where
, , and is the square of the coefficient of variation (CV) of Y for the N2 population's nonresponders.
It is very common phenomenon in practice that we face the issue of nonrespond on Yand X both. In the present investigation, we are dealing with the issue of nonrespond on Y and X both.
Singh et al. [38] introduced the traditional ratio estimator of Ȳ with the non-respond on Y and X, utilizing the known and having high positive correlation between Y and X as,
Where, and
The MSE of t2 till the approximation of degree one is,
where
, Cyx = ρyxCyCx, , Cyx(2) = ρyx(2)Cy(2)Cx(2) with the ρyx and ρyx(2) as the population correlation coefficients for the respondents and nonrespondents, respectively.
Searls [39] worked on the exponential ratio estimator with the nonresponse on Y and X both and having somewhat weak positive correlation between Y and X as,
The MSE of t3 for the approximation of order one is,
Singh et al. [38] worked on the usual regression estimator for the problem of nonrespond on Y and X presented estimator as,
Where, is the sample regression coefficient of the regression line of Y and X for the nonrespondents in the sample.
The MSE of t4 for the approximation of degree one is,
where, Cyx(2) = ρyx(2)Cy(2)Cx(2)
Unal and Kadilar [27] worked on the following modified exponential ratio estimator of Ȳ with the issue of nonrespond on both Y and X as,
The MSE of t5 for the approximation of order one is,
The optimal α for which the MSE of t5 is least is,
Where, and
The least MSE of t5 for the optimal α is,
Motivated by Khare and Kumar [40] and Unal and Kadilar [27], we introduced the following modified generalized class for enhanced estimation of Ȳ with the problem of nonresponse on Y and X as
where, π1 and π2 (π1 + π2 ≠ 1) are the characterizing scalars that are obtained such that the MSE of tp is least possible.
Following are some of the interesting observations about the introduced family of estimators:
(1) For π1 = 1 and π2 = 0, the proposed class becomes ȳ* estimator of Ȳ with the issue of nonresponse.
(2) For π2 = 0, the suggested family of estimators reduces to the estimator introduced by Khare and Kumar [40] with the problem of nonresponse.
(3) For π1 = 0 and π2 = 1, the introduced class of estimators takes the form of the exponential ratio estimator of ȳ introduced by Searls [39] for the problem of nonresponse.
(4) For π1 + π2 = 1, the suggested family reduces to the class of estimators of Ȳ introduced by Unal and Kadilar [27] for the issue of nonresponse.
For sampling properties of the introduced estimator, tp, we used the standard notations given below:
and with and , ,
Expressing tp in terms of (i = 0, 1), we have
Subtracting Ȳ from two sides of above equation, we have,
By taking expectations on two sides of (7) and substituting the values of corresponding expectations, we have the bias of tp as
Squaring on both sides of (7), simplifying and occluding the terms for the approximation of degree one, we get
By taking expectations on two sides of the above equation and substituting the values of corresponding expectations, we obtain the MSE of tp as
where
The optimal π1 and π2 values which minimizes the MSE of tp respectively are,
The least MSE of tp for these optimal π1 and π2 is,
Where,
The efficiency of the proposed and competing estimators have been compared theoretically in the case of nonresponse on both Y and X showed that the efficiency condition of the suggested estimator is superior to the competing estimators. The MSEs of the estimators are used to assess their efficiency. Any estimator ta is said to be more efficient or better than the estimator, tb, if the condition MSE(tb) − MSE(ta) > 0 is satisfied.
The suggested estimator t1 performs better than the estimator proposed by Hansen and Hurwitz [1] under the condition:
if V(t1) − MSE(tp) > 0, or
The proposed estimator tp has lesser MSE than the estimator t2 proposed by Ahmed and Shabeer [35] for the following condition:
if MSE(t2) − MSEmin(tp) > 0, or
The introduced estimator tp is more efficient than the exponential ratio estimator t3 proposed by Yadav et al. [34] under the following condition:
if MSE(t3) − MSEmin(tp) > 0, or
The introduced estimator tp outperforms the competitor's estimator t4 of Ahmed and Shabeer [35] under the following condition:
if MSE(t4) − MSEmin(tp) > 0, or
The proposed estimator tp has lesser MSE than the estimator t5 proposed by Unal and Kadilar [27] for the following stipulation:
if MSE(t5) − MSEmin(tp) > 0, or
In this section, the theoretical efficiency conditions were verified using five real natural data congregations. To see the executions of the introduced and the competing estimators of Ȳ in the presence of nonresponse on both Yand X, we considered all five natural real populations [27]. The parameters of all five considered populations along with their sources are presented in Table 1.
All the above seven populations under consideration were natural real-world populations on which the applications of the suggested estimator along with the competing estimators were carried out in the presence of nonresponse. For instance, we described the seven real-world populations in Yadav et al.'s study [45] as follows:
The data were collected from 923 districts of Turkey from different private teaching institutions in 2006. The study variable Ywas taken as the number of successful students in the student selection examination for secondary schools and the auxiliary variableXwas taken as the number of teachers. It should be mentioned that only 261 homogenous districts for the above population were considered as we used simple random sampling in this study and a sample of size 90 was drawn from the above population of size 261. Here, we faced the problem of nonresponse in both the study and the auxiliary variables. A total of 25% of the units (65 units) was represented in the group of nonresponse.
In the last population, the primary data set, also presented in Yadav et al. [45], for simple random sampling on the production of peppermint yield, which is located at Banikodar block of Barabanki district at Uttar Pradesh state in India in 2019, was collected. It was observed that approximately 20% of nonresponse occurred while collecting data for the targeted units of the population under consideration, and the data were collected on 150 farmers with peppermint yield as the primary variable in kilogram and the auxiliary variable as an area of the field in Bigha (2529.3 Square Meter). Thus, out of 150 units, 120 units were considered in the response group and 20 in the nonresponse group. The parameters of this population are presented in Table 1.
The percentage relative efficiency (PRE) of the suggested and competing estimators with respect to the estimator t1 was calculated using the following formula:
The percentage relative efficiencies of the suggested estimator tp and competing estimators over t1 for all five populations for different values of hare presented in Tables 2–8.
Table 2. Percentage relative efficiency (PRE) of different estimators with respect to t1 for Population 1.
To visualize the performances of the introduced and the competing estimators on the large population, we generated a large artificial population. A simulated population was generated through the R programming language to compare the outcomes of competing estimators and the introduced estimator for the artificial population. For the simulated population, we used the parameters of the real Population 1 given in the numerical study section. A bivariate normal distribution with mean vectors and a variance-covariance matrix to construct the population are as follows:
Means of [Y, X] as μ = [185.22, 1807.23]
Correlation ρyx = 0.90
The following steps were used for the simulation of the required population:
(a) A bivariate normal distribution of X and Y of size N = 5000 has been generated through these parameters using the R Program.
(b) The parameters have been computed for this simulated population of size N = 5000 with N1 = 3500 and N2 = 1500.
(c) A sample of size n with n1 and n2 = n − n1 has been selected from this simulated population.
(d) Sample statistics, which are the sample mean, sample variance, and the values of the introduced and competing estimators ti of Ȳ, are calculated for this sample.
(e) Steps (c) and (d) are repeated m = 50, 000 times.
(f) The MSE of every estimator ti is calculated using the formula, .
(g) The PRE of each of the estimator ti with respect to t1 has been calculated using the formula:
Table 9 represents the PRE of different estimators of Ȳ with respect to t1 for the simulated population.
It can be verified from Table 2 that the PREs of the competing estimators over t1 lie in the intervals [301.6963, 491.1458], [310.1851, 463.1675], [306.3844, 447.5542], [276.1563, 438.3615], and [254.0789, 432.8506] for different values of h from 2 to 6, respectively, for Population 1. From Table 3, it can be observed that the PREs of the estimators in competition over the estimator t1 lie in the intervals [148.0884, 220.6768], [144.4216, 214.9752], [142.6821, 212.6081], [141.6667, 211.3493], and [141.0011, 210.5799] for different values of h from 2 to 6, respectively, for Population 2. It can be verified from Table 4 that the PREs of the competing estimators over t1 lie in the intervals [124.3555, 210.8401], [108.5754, 189.2205], [98.8639, 176.1734], [92.2849, 167.4633], and [87.5332, 161.2454] for different values of h from 2 to 6, respectively, for Population 3. It can be observed from Table 5 that the PREs of the competing estimators over t1 lie in the intervals [233.9950, 359.4776], [232.7577, 351.3462], [232.0054, 346.5934], [231.4997, 343.4756], and [231.1363, 341.2729] for different values of h from 2 to 6, respectively, for Population 4. It can be verified from Table 6 that the PREs of the competing estimators over t1 estimator lie in the intervals [38.8760, 133.8633], [37.0780, 140.6118], [35.8300, 146.1823], [34.9130, 150.8558], and [34.2109, 154.8319] for different values of h from 2 to 6, respectively, for Population 5. From Table 7, it is evident that the PREs of the competing estimators over t1 estimator lie in the intervals [334.7728, 1735.3152], [340.5247, 1739.5428], [346.0438, 1745.3751], [351.8249, 1749.6817], and [357.4681, 1754.8894] for different values of h from 2 to 6, respectively, for Population 6. From Table 8, it can be observed that the PREs of the competing estimators over the estimator t1 lie in the intervals [150.5897, 383.9387], [125.4893, 383.8675], [118.8892, 383.8323], [115.8396, 383.8108], and [114.0844, 383.7792] for different values of h from 2 to 6, respectively, for Population 7. The PREs of the competing estimators with respect to t1 for the simulated population for different values of h from 2 to 6 lie in the interval [269.1084, 502.3164]. On the other hand, the PREs of the introduced estimator over t1 for different values of h from 2 to 6, respectively, are (506.2415, 482.3257, 473.5124, 470.6528, and 471.0254) for Population 1, (228.4691, 225.3594, 224.2563, 222.2874, and 221.9566) for Population 2, (223.4526, 215.2046, 188.8459, 183.9876, and 181.0087) for Population 3, (418.5783, 416.6584, 414.6217, 412.5894, and 412.01365) for Population 4, (139.2657, 151.4568, 157.3259, 163.4628, and 168.8413) for Population 5, (2054.8142, 2066.5743, 2074.2057, 2083.9104, 2087.7764) for Population 6, and (454.8142, 454.5743, 453.8057, 453.6104, and 452.7764) for Population 7. The PREs of the competing estimators with respect to t1 for the simulated population for different values of from 2 to 6 lie in the interval [483.2057, 529.9382].
We introduced a naive family of estimators for more efficient estimation of Ȳ using known auxiliary parameters in the case of nonresponse on both Yand X in the current study. For the approximation till order one, the bias and MSE of the introduced estimator were investigated. The optimum values of the characterizing scalars and the minimum value of the MSE of the introduced estimator were determined. The proposed estimator was theoretically compared to competing population mean estimators, and the efficiency criteria for the proposed estimator over competing estimators were determined. These efficiency criteria are verified using the five natural populations and one simulated population under investigation. The presented estimator is proven to be the most efficient estimator when compared to the class of competing estimators Hansen and Hurwitz [1], Khare and Kumar [40], Searls [39], and Unal and Kadilar [27], as it has the least MSE among these competing estimators for all seven real-world natural and one simulated data sets. As a result, the recommended estimators can be used in a variety of fields, such as Agricultural Science, Biological Sciences, Business, Commerce, Economics, Engineering, Fisheries, Mathematical Sciences, Medical Sciences, Poultry, and Social Sciences, in the case of nonresponse.
The original contributions presented in the study are included in the article/supplementary material, further inquiries can be directed to the corresponding authors.
All authors listed have made a substantial, direct, and intellectual contribution to the work and approved it for publication.
The authors express their heartfelt thanks to the Associate Editor and the learned referees for their valuable comments which improved the earlier draft. The authors are also thankful to the Deanship of Scientific Research, Qassim University for funding the publication of this project.
The authors declare that the research was conducted in the absence of any commercial or financial relationships that could be construed as a potential conflict of interest.
All claims expressed in this article are solely those of the authors and do not necessarily represent those of their affiliated organizations, or those of the publisher, the editors and the reviewers. Any product that may be evaluated in this article, or claim that may be made by its manufacturer, is not guaranteed or endorsed by the publisher.
1. Hansen MH, Hurwitz WN. The problem of non-response in sample surveys. J Am Stat Assoc. (1946) 41:517–29. doi: 10.1080/01621459.1946.10501894
2. El-Badry MA. A sampling procedure for mailed questionnaires. J Am Stat Assoc. (1956) 51:209–27. doi: 10.1080/01621459.1956.10501321
3. Rao SRS. Ratio estimation with sub sampling the non-respondents. Surv Methodol. (1986) 12:217–30.
4. Khare BB, Srivastava S. Study of conventional and alternative two phase sampling ratio, product and regression estimators in presence of nonresponse. Proc Natl Acad Sci India Sect. (1995) 65:195–204.
5. Khare BB, Srivastava S. Transformed ratio type estimators for the population mean in the presence of nonresponse. Commun Stat. (1997) 7:1779–91. doi: 10.1080/03610929708832012
6. Tracy DS, Osahan SS. Random non-response on study variable versus on study as well as auxiliary variables. Statistica. (1994) 54:163–8.
7. Singh HP, Kumar S. A regression approach to the estimation of the finite population mean in the presence of non-response Australian and New Zealand. J Statistics. (2008) 4:395–408. doi: 10.1111/j.1467-842X.2008.00525.x
8. Kumar S, Bhougal S. Estimation of the population mean in presence of non-response. Commun Stat. (2011) 18:537–48. doi: 10.5351/CKSS.2011.18.4.537
9. Grover LK, Kaur P. A generalized class of ratio type exponential estimators of population mean under linear transformation of auxiliary variable. Commun Stat. (2014) 43:1552–74. doi: 10.1080/03610918.2012.736579
10. Bii NK, Onyango CO. Odhiambo J. Estimating a finite population mean under random non response in two stage cluster sampling with replacement. J. Physics: Conference Series. (2018) 1132:012071. doi: 10.1088/1742-6596/1132/1/012071
11. Sharma P, Pal SK. Estimation of population mean in presence of random non-response. J Stat Manag Syst. (2018) 21:1105–19. doi: 10.1080/09720510.2018.1478623
12. Muneer S, Shabbir J, Khalil A. A generalized exponential type estimator of population mean in the presence of non-response. Stat Transit. (2018) 19:259–76. doi: 10.21307/stattrans-2018-015
13. Toutenburg H, Srivastava VK. Estimation of ratio of population means in survey sampling when some observations are missing. Metrika. (1998) 48:177–87. doi: 10.1007/PL00003973
14. Toutenburg H, Srivastava VK. Efficient estimation of population mean using incomplete survey data on study and auxiliary characteristics. Statistica. (2003) 63:223–36.
15. Khare BB, Sinha RR. Estimation of the ratio of the two population means using multi auxiliary characteristics in the presence of non-response. In: Statistical Techniques in Life Testing. Reliability, Sampling Theory and Quality Control. Warsaw: Główny Urza̧d Statystyczny (2007). p. 63–171.
16. Khare BB, Sinha RR. On the class of estimators for population mean using multi auxiliary characters in the presence of non-response. Stat Transit. (2009) 10:3–14. doi: 10.21307/stattrans-2019-025
17. Srinath KP. Multiphase sampling in non-response problems. J Am Stat Assoc. (1971) 66:583–6. doi: 10.1080/01621459.1971.10482310
18. Tabasum R, Khan IA. Double sampling for ratio estimator for the population mean in the presence of non-response. Assam Statistical Review. (2006) 20:73–83.
19. Singh HP, Kumar S, Kozak M. Improved estimation of finite population mean using sub-sampling to deal with non response in two phase sampling scheme. Commun Stat Theory Method. (2010) 39:791–802. doi: 10.1080/03610920902796056
20. Shabbir J, Khan NS. On estimating the finite population mean using two auxiliary variables in two phase sampling in the presence of non response. Commun Stat Theory Method. (2013) 42:4127–45. doi: 10.1080/03610926.2011.643851
21. Yadav DK, Devi MM, Yadav SK. Estimation of finite population mean using known coefficient of variation in the simultaneous presence of non-response and measurement errors under double sampling scheme. J Reliability Stat Stud. (2018) 11:51–66.
22. Sud UC, Srivastava SK. Estimation of population mean in repeat surveys in the presence of measurement errors. J Indian Soc Agric Stat. (2000) 53:125–33.
23. Srivastava K. and Shalabh, Effect of measurement errors on the regression method of estimation in survey sampling. J Stat Res. (2001) 35:35–44.
24. Kumar S, Bhougal S, Nataraja NS, Viswanathaiah M. Estimation of population mean in the presence of non-response and measurement error. Revista Colombiana de Estadistica. (2015) 38:145–61. doi: 10.15446/rce.v38n1.48807
25. Kumar S. Improved estimation of population mean in presence of nonresponse and measurement error. J Stat Theory Pract. (2016) 10:707–20. doi: 10.1080/15598608.2016.1216488
26. Singh P, Singh R, Bouza CN. Effect of measurement error and non-response on estimation of population mean. Revista Investigacion Operacional. (2018) 39:108–20.
27. Unal C, Kadilar C. Adapted exponential type estimator in the presence of non-response. J Reliability Stat Stud. (2021) 14:311–22. doi: 10.13052/jrss0974-8024.14115
28. Yadav SK, Yadav OP, Yadav DK. Dexterous estimation of population mean in survey sampling under non-response error. Int J Math Eng Manag Sci. (2019) 4:1307–27. doi: 10.33889/IJMEMS.2019.4.6-103
29. Sharma V, Kumar S. Estimation of population mean using transformed auxiliary variable and non-response. Revista Investigacion Operacional. (2020) 41:438–44.
30. Unal C, Kadilar C, A. New Family of exponential type estimators in the presence of non-response. J Math Fundam Sci. (2021) 53:1–15. doi: 10.5614/j.math.fund.sci.2021.53.1.1
31. Unal C, Kadilar C, A. new population mean estimator under non-response cases. J Taibah Uni Sci. (2022) 16:111–9. doi: 10.1080/16583655.2022.2034343
32. Jaiswal AK, Singh GN, Pandey AK. Improved procedures for mean estimation under non-response. Alexandria Eng J. (2022) 61:12813–28. doi: 10.1016/j.aej.2022.06.031
33. Ahmed S, Shabbir J, Gupta S. Use of scrambled response model in estimating the finite population mean in presence of non response when coefficient of variation is known. Commun Stat Theory Method. (2017) 46:8435–49. doi: 10.1080/03610926.2016.1183782
34. Yadav DK, Devi M, Yadav SK. Estimation of finite population mean using known coefficient of variation in the simultaneous presence of non-response and measurement errors under double sampling scheme. J Reliability Stat Stud. (2018) 11:51–66.
35. Ahmed S. Shabbir J. Model based estimation of population total in presence of non-ignorable non-response. PLoS ONE. (2019) 14:e0222701. doi: 10.1371/journal.pone.0222701
36. Hussain S, Ahmad S, Akhtar S, Javed A, Yasmeen U. Estimation of finite population distribution function with dual use of auxiliary information under non-response. PLoS ONE. (2020) 15:e0243584. doi: 10.1371/journal.pone.0243584
37. Ahmad S, Hussain S, Aamir M, Khan F, Alshahrani MN, Alqawba M. Estimation of finite population mean using dual auxiliary variable for non-response using simple random sampling. AIMS Mathematics. (2022) 7:4592–613. doi: 10.3934/math.2022256
38. Singh R, Kumar M, Chaudhary MK, Smarandache F. Estimation of mean in presence of non-response using exponential estimator. arXiv. (2009) 0906.2462. doi: 10.48550/arXiv.0906.2462
39. Searls DT. The utilization of a known coefficient of variation in the estimation procedure. J Am Stat Assoc. (1964) 59:1225–6. doi: 10.1080/01621459.1964.10480765
40. Khare BB, Kumar S. Utilization of coefficient of variation in the estimation of population mean using auxiliary character in the presence of non-response. Natl Acad Sci Lett. (2009) 32:235–41.
41. Sinha RR, Kumar V. Estimation of mean using double sampling the non-respondents with known and unknown variance. Int J Comput Sci Math. (2015) 6:442–58. doi: 10.1504/IJCSM.2015.072963
42. Khare BB, Srivastava S. Estimation of population mean using auxiliary character in presence of non-response. Natl Acad Sci Lett. (1993) 16:111–4.
43. Sinha RR, Kumar V. Families of estimators for finite population variance using auxiliary character under double sampling the non-respondents. Natl Acad Sci Lett. (2015) 38:501–5. doi: 10.1007/s40009-015-0380-6
44. Satici E, Kadilar C. Ratio estimator for the population mean at the current occasion in the presence of non-response in successive sampling. Hacettepe J Math Stat. (2011) 40:115–24.
Keywords: primary variable, auxiliary variable, non-response, bias, MSE, PRE
Citation: Ahmadini AAH, Yadav T, Yadav SK and Al Luhayb ASM (2022) Restructured searls family of estimators of population mean in the presence of nonresponse. Front. Appl. Math. Stat. 8:969068. doi: 10.3389/fams.2022.969068
Received: 14 June 2022; Accepted: 10 August 2022;
Published: 17 October 2022.
Edited by:
Shakeel Ahmed, National University of Sciences and Technology (NUST), PakistanReviewed by:
Usman Shahzad, Pir Mehr Ali Shah Arid Agriculture University, PakistanCopyright © 2022 Ahmadini, Yadav, Yadav and Al Luhayb. This is an open-access article distributed under the terms of the Creative Commons Attribution License (CC BY). The use, distribution or reproduction in other forums is permitted, provided the original author(s) and the copyright owner(s) are credited and that the original publication in this journal is cited, in accordance with accepted academic practice. No use, distribution or reproduction is permitted which does not comply with these terms.
*Correspondence: Abdullah Ali H. Ahmadini, YWFobWFkaW5pQGphemFudS5lZHUuc2E=; S. K. Yadav, ZHJza3lzdGF0c0BnbWFpbC5jb20=; Asamh Saleh M. Al Luhayb, YS5hbGx1aGF5YkBxdS5lZHUuc2E=
Disclaimer: All claims expressed in this article are solely those of the authors and do not necessarily represent those of their affiliated organizations, or those of the publisher, the editors and the reviewers. Any product that may be evaluated in this article or claim that may be made by its manufacturer is not guaranteed or endorsed by the publisher.
Research integrity at Frontiers
Learn more about the work of our research integrity team to safeguard the quality of each article we publish.