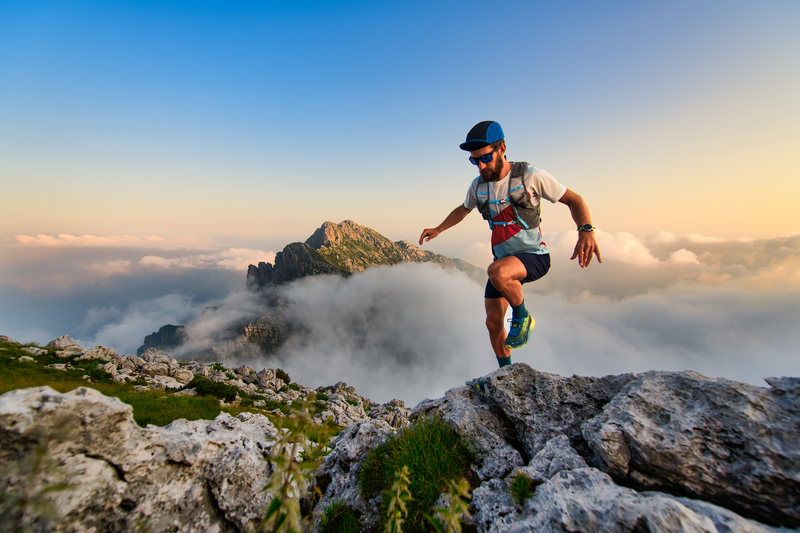
95% of researchers rate our articles as excellent or good
Learn more about the work of our research integrity team to safeguard the quality of each article we publish.
Find out more
PERSPECTIVE article
Front. Appl. Math. Stat. , 23 May 2022
Sec. Dynamical Systems
Volume 8 - 2022 | https://doi.org/10.3389/fams.2022.905807
This article is part of the Research Topic Patterns of synchrony in neuronal ensembles: a broad perspective View all 8 articles
Over the past few decades, neuroscience studies have elucidated the structural/anatomical network characteristics in the brain and their associations with functional networks and the dynamics of neural activity. These studies have been carried out at multiple spatial-temporal scale levels, including spikes at the neural microcircuit level, neural activity at the intra-brain regional level, and neural interactions at the whole-brain network level. One of the structural and functional neural characteristics widely observed among large spatial-temporal scale ranges is long-tail distribution, typified as power-low distribution, gamma distribution, and log-normal distribution. In particular, long-tailed distributions found in excitatory postsynaptic potentials (EPSP) induce various types of neural dynamics and functions. We reviewed recent studies on neural dynamics produced by the structural long-tailed characteristics of brain neural networks. In particular, the spiking neural network with a log-normal EPSP distribution was first introduced for the essential factors to produce spontaneous activity and was extended and utilized for studies on the association of neural dynamics with the network topology depending on EPSP amplitude. Furthermore, the characteristics of the response to a steady stimulus and its dependence on E/I balance, which are widely observed under pathological conditions, were described by the spiking neural networks with EPSP long-tailed distribution. Moreover, this spiking neural network has been utilized in modeling studies of mutual interactions among local microcircuit circuits. In future studies, the implementation of more global brain network architectures in modeling studies might reveal the mechanisms by which brain dynamics and brain functions emerge from the whole brain network architecture.
Over the past few decades, neuroscience studies have elucidated the characteristics of the structural/anatomical networks in the brain and their associations with functional networks and the dynamics of neural activity (reviewed in [1, 2]). These studies have been proceeded in multiple spatiotemporal scale levels among spikes at the neural microcircuit level, neural activity at the intra-brain regional level, and neural interactions at the whole-brain network level [3–9] (reviewed in [2, 10]).
One of the structural and functional neural characteristics widely observed among large spatial-temporal scale ranges is the long-tail distribution, typified as the power-low distribution, gamma distribution, and log-normal distribution (reviewed in [10–12]). In particular, the long-tailed distributions found in excitatory postsynaptic potentials (EPSP) [13, 14], spine size [3], and astrocytes [15] are the structural microcircuit level; ones for the size of links to connect the brain regions called node degree are the structural whole-brain network level [16, 17]. For functional neural activity, it was found that the spiking properties within fast temporal scales (10−3−10−1 s), such as inter-spike intervals [18] and the size of the synchronous spiking population called neuronal avalanche [12, 19, 20], and long-range temporal correlations at slow temporal scales (101−103 s) [21] obey the spatio-temporal long-tailed distribution. Recent findings have shown that these long-tailed characteristics of neural activity are produced by multiple network organizations composed of partially independent neural dynamics within modular structures [9]. Furthermore, long-tailed characteristics have been observed in higher cognitive processes (reviewed in [22]). In particular, in visual perception tasks, where an ambiguous figure with two different interpretations is presented, the period of dominant perception, which involves unilateral stimulus interpretation, follows a heavy-tailed gamma distribution [23–26].
In addition to the neuroimaging and electrophysiological approaches, to reveal the association between structural/anatomical network characteristics and functional neural characteristics, a modeling approach with high physiological validity, typified as spiking neural networks (reviewed in [27, 28]), is highly effective because the construction of a brain network model with a focus on structural network characteristics enables comprehensive simulation and bifurcation analysis to identify the structural causes of functional neural characteristics [29–33] (reviewed in [28, 34]). The modeling approach revealed that long-tailed structural network characteristics and mutual neural interactions in hierarchical module network structures produce long-tailed neural activity [29–32], which might support neural functions [35–38].
In this perspective, we summarize the progress of the modeling approach using spiking neural networks to elucidate the mechanism of long-tailed neural activity and its association with structural network characteristics, especially regarding long-tailed EPSP distribution, over the past decade. First, modeling studies focusing on synaptic connections and their topological characteristics at the microcircuit level were reviewed. Furthermore, we review modeling studies on the response characteristics of external stimuli and the mutual interactions between neural modules/populations. This review discusses the future perspectives of these studies, involving modeling studies on whole-brain level neural activity in large-scale spiking neural networks composed of brain regions.
In the microcircuits of the local cortical networks, a log-normal distribution of EPSPs in synaptic connections was found; i.e., EPSPs of the large majority of synaptic connections indicate sub-millivolts (mV), while EPSPs of a small minority of synaptic connections exhibit approximately 1–10 mV [13, 14]. Teramae et al. showed that this log-normal distribution causes spontaneous activity [39, 40], which is irregular and has a low firing rate (≲ 1.0 Hz) spiking activity and is sustained even in the absence of an external stimulus [41]. In this network with the EPSP log-normal distribution, spikes through weak synaptic connections and spikes through strong synaptic connections play a role in noise and signal, respectively, in the mechanism of stochastic resonance theory [41]. An adequate amount of spikes through small synapses realizes the “up-state” where the membrane potentials become higher (≈ −60 mV) than the “down-state” where the membrane potential is located at around the resting state (≈ −70 mV); in the up-state, even a single spike from the strong synapse achieves the emergence of the spike [41]. Therefore, among the major part of neuron pairs connected by weak synapses, the spikes do not synchronize, whereas, among the minor part of neuron pairs connected by strong synapses, the spikes synchronize. These properties are highly in line with physiological findings [42–44]. In several decades, many modeling approaches have been developed, such as an approach focusing on the existence of neurons with a low threshold [45], topological characteristics of small-worldness [46], and sparse random-networkness [47]. Among these studies, as the mechanism to describe the spontaneous activity, the approach focusing on the log-normal EPSP distribution exhibits the highest physiological validity (an overview of related studies on the log-normal EPSP distribution is shown in Table 1).
The mechanisms that produce the EPSP log-normal distribution have been investigated using physiological and modeling approaches (reviewed in [10]). Spike-timing-dependent plasticity (STDP) [50, 51] results in self-organized synaptic connections reflecting internal and exogenous spiking activity [52–54]. The proposed STDP rule is constructed by the mutual spike interaction between postsynaptic neurons and presynaptic neurons within several 100 ms [50, 51] and produces a separated synaptic distribution between significantly weak and strong synaptic weights [50, 52, 55]. However, the development of the size of the spine depending on the amplitude of EPSP [56] follows a longer timescale than that of this STDP rule [3]; the separated synaptic distribution is not congruent with the actual log-normal EPSP distribution [13, 14]. To describe these points, a synaptic weight-dependent STDP rule with sublinear weight-dependent depression, i.e., the large long-term depression for strong synapses and relatively small for long-term enhancement for strong synapses than for weak synapses is called log-STDP [57]. Along with the achievement of log-normal distribution, log-STDP realizes stable neural dynamics [57].
Moreover, the log-normal EPSP distribution produces many neural activities and functions [31, 35, 37, 48]. In particular, log-normal EPSPs induce a neural avalanche where the size of the synchronous spikes obeys a power law as long-tail characteristics of neural activity [48] (particularly, the theoretical analysis for the mechanism of producing neural avalanches by the long-tail distribution was described in Reference [58]) and bust spiking patterns to achieve hippocampal memory consolidation [37]. Nobukawa et al. reported that the complexity of spontaneous activity produced by log-normal EPSPs, detected by multifractal analysis, reflects the deterministic process in the spiking neural network [31]. Hiratani et al. showed that the EPSP log-normal distribution enhances memory capacity in a spiking neural network with an associative memory function [35].
In addition to synaptic amplitude, topological network characteristics change depending on the distribution of EPSPs [13, 59]. The major part of weak EPSPs synaptic connections constructs a random network, while the minor part of strong EPSPs synaptic connections forms clusters that are joined to each other, instead of isolated clusters, i.e., the strong EPSPs synaptic connections construct a small-world network [60]. In our previous study, the emergent temporal patterns of neural activity produced by this random and small-world dual-network topology were investigated through a simulation with a spiking neural network composed of excitatory and inhibitory neurons [30]. As a result, the clusters connected by strong synapses exhibit intermittent high-frequency spiking activity, which induces deterministic slow temporal neural activity (≲5 Hz), whereas, in a single topological random network, the neural activity drives with a faster temporal scale (mainly frequency component: ≳50 Hz) [30, 31]. In previous findings on local excitatory-inhibitory neural networks, through the mechanism of pyramidal-interneuron gamma (PING) [61] and interneuronal gamma (ING) [62, 63], the fast-spiking activity typified as gamma band activity becomes dominant. However, our findings of neural activity in the dual-network topology implied that the network even at the local microcircuit level can produce slow temporal-scale neural dynamics [30]. The physiological validity of these slow temporal-scale dynamics must be verified using an electrophysiological experimental approach.
In addition to elucidating the internal-neural activity and functions induced by the EPSP log-normal distribution at the local microcircuit level, the response to external stimuli and the interaction between neural populations in the spiking neural network with the EPSP log-normal distribution have been investigated [32, 49]. In particular, the EPSP log-normal distribution enhances the dependence of the response to external stimuli on the excitatory and inhibitory (E/I) balance [49] and produces a long-tailed distribution on mutual interactions among the activities of neural populations [32].
Sensory cortical neural activity is synchronized with a periodic stimulus with a specific frequency, which is known as the auditory steady-state response (ASSR) and steady-state visually evoked potentials [64–66]. The degree of this synchronization depends on the input frequency; the degree becomes remarkably high in gamma band activities, which play a role in perceptual function [64–66]. Neuroimaging studies using electroencephalography (EEG) and magnetoencephalography (MEG) revealed that E/I imbalance leads to an alteration of the steady-state response (reviewed in [67, 68]). Herein, these studies were conducted by comparing healthy (or typical development) subjects with psychiatric disorders, such as schizophrenia [69–71], bipolar disorder [72–75], and autism spectrum disorder [76–78], because E/I imbalance is a common neural basis for the impairment of brain functions widely observed in psychiatric disorders [79–82]. In modeling studies on E/I balance, although the influence of inhibitory synaptic amplitude on spatiotemporal patterns of neural activity has been reported, the characteristics of steady-state response and the relationship to log-normal EPSP distribution remain unclear [83–85]. Against this situation, our recent study with a spiking neural network with log-normal EPSP under steady stimulus showed that the existence of strong synaptic connections produced by log-normal EPSP distribution enhances the tendency to decrease the degree of steady-state response by increasing the excitatory ratio in the E/I balance [49]. In the input frequency characteristics, this decreasing tendency is significant in the gamma band (≈ 60 Hz) in comparison with other frequency bands [49], which is highly congruent with physiological findings under conditions of psychiatric disorders [69–78]. The causes of these response characteristics are that the autonomous irregular gamma-band activity of the internal network becomes dominant under the high excitatory ratio and the existence of strong excitatory synaptic connections in the EPSP log-normal distribution. Consequently, the response against external stimuli becomes desensitized, especially against the one with frequency components around the gamma band [49]. Although our study showed that the log-normal EPSP distribution causes a desensitized steady-state response with an increasing excitatory ratio [49], the log-normal EPSP distribution might also lead to the physiologically observed dependence of neural interaction between wide-range brain regions, i.e., functional whole-brain networks, on the E/I ratio [86–88]. This point must be evaluated in future studies.
In addition to the response to external stimuli, regarding the neural interactions among neural populations with log-normal EPSP distribution, we constructed a spiking neural network composed of two neural modules, where each module is composed of an excitatory neural population with log-normal EPSP and an inhibitory neural population as the local cortical network, and two modules are joined by the inter-lateral excitatory synaptic connections (refer to an overview of the network architecture in Figure 1) [32]. Each neural module, where the random network topology was used for the network topology, exhibits fast neural fluctuations dominantly with the gamma band frequency component (≈ 60 Hz) under isolated conditions [30, 32]. In two coupled neural modules, under the condition of moderate input stimulus and log-normal EPSP distribution involving a minor part of strong synaptic connections in intramodule synaptic connections, neural behaviors with wide frequency components, especially involving a slow frequency component (≲5 Hz), appear; this temporal behavior exhibits deterministic dynamical behaviors. Subsequently, this condition leads to an intermittent intermodule-alternative behavior where either neural activity becomes larger (activate state) than the other one (deactivate state) and its alternation duration follow a gamma distribution with long-tailed characteristics [32]. Alternation behaviors following the gamma distribution were widely observed in actual neural activity at several hierarchical levels [10, 23–26].
Figure 1. Spiking neural network composed of two neural modules where each module is composed of the excitatory neural population with log-normal EPSP, and inhibitory neural population as the local cortical network and the two modules are joined by inter-lateral excitatory synaptic connections [32]. In the two coupled neural modules, under the condition of moderate input stimulus and log-normal EPSP distribution in intermodule synaptic connections, neural behavior with wide frequency components appears, especially involving a slow frequency component (≲5 Hz). This slow neural behavior is produced from an intermittent intermodule-alternative behavior where either neural activity becomes larger (called the activated state) than the other one (deactivated state), and its alternation duration follows a gamma distribution with long-tailed characteristics.
This alternative behavior is produced by the following mechanism [32]: First, in the module of the activated state, the neural activities of intra excitatory and inhibitory neural populations strongly synchronize, i.e., the intra excitatory-inhibitory neural interaction produces the activated state. In the module of the deactivated state, the neural activity is driven by the activated module (intra excitatory-inhibitory synchronization is unlocked). Second, although both inhibitory neural activities between intermodules always synchronize through the inter excitatory synaptic connections, the large neural fluctuation due to the effect of intra log-normal EPSP distribution in the activated module unlocks the intra excitatory and inhibitory neural activities. Subsequently, by decreasing the input-driven inhibitory neural activity in the deactivate module, the deactivate state transits to the activated state. Previous studies using the neural network model showed that autonomous irregular neural activity typified as internal chaotic activity is essential to produce such alternation behavior [29, 89]. Under conditions without autonomous neural activity with large variability, either neural modulation becomes activated, and the other is suppressed; consequently, the alternation does not appear [90]. In previous studies on the chaotic neural activity for the emergence of this alternation, internal neural parameters typified as decay factors and parameters for neural connections between intramodule excitatory and inhibitory neural populations were focused on Nagao et al. [89], Kanamaru and Sekine [91], and Kanamaru [29]. Our approach focused on structural long-tailed characteristics to produce long-tailed characteristics for neural activity [32]. To verify the physiological validity of our approach in describing the long-tail characteristics of neural activity, future neuroimaging and electrophysiological studies are required. Even in the absence of external stimuli, the neural activity among regions of the brain and whole-brain functional networks exhibit large fluctuations, that is, high variability of resting-state neural activity [92–94], the dynamical transition of neural activity [95], and dynamic functional connectivity [96]. In addition to the autonomous state transition in two neural modules with a log-normal EPSP [32], the large variability of intermodule neural activity might cause autonomous whole-brain network dynamics. In future studies, modeling of large-scale whole-brain networks must be conducted.
We reviewed recent studies on neural dynamics produced by the structural long-tailed characteristics of brain neural networks. In particular, the spiking neural network with a log-normal EPSP distribution was first introduced by Teramae et al. for the essential factors to produce spontaneous activity [41] and was extended and used for studies on the association of neural dynamics with the network topology depending on EPSP amplitude [32]. Furthermore, the characteristics of the response to a steady stimulus and its dependence on the E/I balance, which are widely observed under pathological conditions, were described by the spiking neural networks with an EPSP long-tailed distribution [49]. Moreover, this spiking neural network has been utilized in modeling studies of mutual interactions among local microcircuit circuits [32]. In future studies, by implementing other network architectures such as global network centrality and small-worldness [1, 2], revealing the mechanisms by which brain dynamics and brain functions emerge from the whole-brain network architectures will be further progressed.
The original contributions presented in the study are included in the article/supplementary material, further inquiries can be directed to the corresponding author.
SN drafted the main manuscript text and prepared all figures.
This study was supported by JSPS KAKENHI for a Grant-in-Aid for Scientific Research (C) (Grant No. 22K12183) (SN). This study was partially supported by the JST CREST (Grant No. JPMJCR17A4).
The author declares that the research was conducted in the absence of any commercial or financial relationships that could be construed as a potential conflict of interest.
All claims expressed in this article are solely those of the authors and do not necessarily represent those of their affiliated organizations, or those of the publisher, the editors and the reviewers. Any product that may be evaluated in this article, or claim that may be made by its manufacturer, is not guaranteed or endorsed by the publisher.
2. Betzel RF, Bassett DS. Multi-scale brain networks. Neuroimage. (2017) 160:73–83. doi: 10.1016/j.neuroimage.2016.11.006
3. Yasumatsu N, Matsuzaki M, Miyazaki T, Noguchi J, Kasai H. Principles of long-term dynamics of dendritic spines. J Neurosci. (2008) 28:13592–608. doi: 10.1523/JNEUROSCI.0603-08.2008
4. van den Heuvel M, Mandl R, Luigjes J, Pol HH. Microstructural organization of the cingulum tract and the level of default mode functional connectivity. J Neurosci. (2008) 28:10844–51. doi: 10.1523/JNEUROSCI.2964-08.2008
5. Hagmann P, Cammoun L, Gigandet X, Meuli R, Honey CJ, Wedeen VJ, et al. Mapping the structural core of human cerebral cortex. PLoS Biol. (2008) 6:e159. doi: 10.1371/journal.pbio.0060159
6. Helmstaedter M, Briggman KL, Turaga SC, Jain V, Seung HS, Denk W. Connectomic reconstruction of the inner plexiform layer in the mouse retina. Nature. (2013) 500:168–74. doi: 10.1038/nature12346
7. Go ni J, Van Den Heuvel MP, Avena-Koenigsberger A, De Mendizabal NV, Betzel RF, Griffa A, et al. Resting-brain functional connectivity predicted by analytic measures of network communication. Proc Natl Acad Sci USA. (2014) 111:833–8. doi: 10.1073/pnas.1315529111
8. Barral J, Reyes AD. Synaptic scaling rule preserves excitatory-inhibitory balance and salient neuronal network dynamics. Nat Neurosci. (2016) 19:1690–6. doi: 10.1038/nn.4415
9. Zhigalov A, Arnulfo G, Nobili L, Palva S, Palva JM. Modular co-organization of functional connectivity and scale-free dynamics in the human brain. Netw Neurosci. (2017) 1:143–65. doi: 10.1162/NETN_a_00008
10. Buzsáki G, Mizuseki K. The log-dynamic brain: how skewed distributions affect network operations. Nat Rev Neurosci. (2014) 15:264–78. doi: 10.1038/nrn3687
11. Bullmore E, Sporns O. Complex brain networks: graph theoretical analysis of structural and functional systems. Nat Rev Neurosci. (2009) 10:186–98. doi: 10.1038/nrn2575
12. Mizuseki K, Buzsáki G. Preconfigured, skewed distribution of firing rates in the hippocampus and entorhinal cortex. Cell Rep. (2013) 4:1010–21. doi: 10.1016/j.celrep.2013.07.039
13. Song S, Sjöström PJ, Reigl M, Nelson S, Chklovskii DB. Highly nonrandom features of synaptic connectivity in local cortical circuits. PLoS Biol. (2005) 3:e68. doi: 10.1371/journal.pbio.0030068
14. Lefort S, Tomm C, Sarria JCF, Petersen CC. The excitatory neuronal network of the C2 barrel column in mouse primary somatosensory cortex. Neuron. (2009) 61:301–316. doi: 10.1016/j.neuron.2008.12.020
15. Gavrilov N, Golyagina I, Brazhe A, Scimemi A, Turlapov V, Semyanov A. Astrocytic coverage of dendritic spines, dendritic shafts, and axonal boutons in hippocampal neuropil. Front Cell Neurosci. (2018) 12: 248. doi: 10.3389/fncel.2018.00248
16. Hagmann P, Kurant M, Gigandet X, Thiran P, Wedeen VJ, Meuli R, et al. Mapping human whole-brain structural networks with diffusion MRI. PLoS ONE. (2007) 2:e597. doi: 10.1371/journal.pone.0000597
17. Chung MK, Hanson JL, Adluru N, Alexander AL, Davidson RJ, Pollak SD. Integrative structural brain network analysis in diffusion tensor imaging. Brain Connect. (2017) 7:331–46. doi: 10.1089/brain.2016.0481
18. Shinomoto S, Miura K, Koyama S. A measure of local variation of inter-spike intervals. Biosystems. (2005) 79:67–72. doi: 10.1016/j.biosystems.2004.09.023
19. Mizuseki K, Buzsaki G. Theta oscillations decrease spike synchrony in the hippocampus and entorhinal cortex. Phil Trans R Soc B. (2014) 369:20120530. doi: 10.1098/rstb.2012.0530
20. Beggs JM, Plenz D. Neuronal avalanches in neocortical circuits. J Neurosci. (2003) 23:11167–77. doi: 10.1523/JNEUROSCI.23-35-11167.2003
21. Linkenkaer-Hansen K, Nikouline VV, Palva JM, Ilmoniemi RJ. Long-range temporal correlations and scaling behavior in human brain oscillations. J Neurosci. (2001) 21:1370–7. doi: 10.1523/JNEUROSCI.21-04-01370.2001
23. Levelt WJ. Note on the distribution of dominance times in binocular rivalry. Br J Psychol. (1967) 58:143–5. doi: 10.1111/j.2044-8295.1967.tb01068.x
24. Borsellino A, De Marco A, Allazetta A, Rinesi S, Bartolini B. Reversal time distribution in the perception of visual ambiguous stimuli. Kybernetik. (1972) 10:139–44. doi: 10.1007/BF00290512
25. Walker P. Stochastic properties of binocular rivalry alternations. Percept Psychophys. (1975) 18:467–73. doi: 10.3758/BF03204122
26. Lehky SR. Binocular rivalry is not chaotic. Proc R Soc Lond B. (1995) 259:71–6. doi: 10.1098/rspb.1995.0011
28. Nobukawa S, Nishimura H. Synchronization of chaos in neural systems. Front Appl Math Stat. (2020) 6:19. doi: 10.3389/fams.2020.00019
29. Kanamaru T. Chaotic pattern alternations can reproduce properties of dominance durations in multistable perception. Neural Comput. (2017) 29:1696–720. doi: 10.1162/NECO_a_00965
30. Nobukawa S, Nishimura H, Yamanishi T. Temporal-specific complexity of spiking patterns in spontaneous activity induced by a dual complex network structure. Sci Rep. (2019) 9:1–12. doi: 10.1038/s41598-019-49286-8
31. Nobukawa S, Wagatsuma N, Nishimura H. Deterministic characteristics of spontaneous activity detected by multi-fractal analysis in a spiking neural network with long-tailed distributions of synaptic weights. Cogn Neurodyn. (2020) 14:829–36. doi: 10.1007/s11571-020-09605-6
32. Nobukawa S, Nishimura H, Wagatsuma N, Ando S, Yamanishi T. Long-tailed characteristic of spiking pattern alternation induced by log-normal excitatory synaptic distribution. IEEE Trans Neural Netw Learn Syst. (2021) 32:3525–37. doi: 10.1109/TNNLS.2020.3015208
33. Kopsick JD, Tecuatl C, Moradi K, Attili SM, Kashyap HJ, Xing J, et al. Robust resting-state dynamics in a large-scale spiking neural network model of area CA3 in the mouse hippocampus. Cognit Comput. (2022). doi: 10.1007/s12559-021-09954-2. [Epub ahead of print].
34. Rabinovich MI, Varona P, Selverston AI, Abarbanel HD. Dynamical principles in neuroscience. Rev Mod Phys. (2006) 78:1213. doi: 10.1103/RevModPhys.78.1213
35. Hiratani N, Teramae JN, Fukai T. Associative memory model with long-tail-distributed Hebbian synaptic connections. Front Comput Neurosci. (2013) 6:102. doi: 10.3389/fncom.2012.00102
36. Klinshov VV, Teramae Jn, Nekorkin VI, Fukai T. Dense neuron clustering explains connectivity statistics in cortical microcircuits. PLoS ONE. (2014) 9:e94292. doi: 10.1371/journal.pone.0094292
37. Omura Y, Carvalho MM, Inokuchi K, Fukai T. A lognormal recurrent network model for burst generation during hippocampal sharp waves. J Neurosci. (2015) 35:14585–601. doi: 10.1523/JNEUROSCI.4944-14.2015
38. Hiratani N, Fukai T. Hebbian wiring plasticity generates efficient network structures for robust inference with synaptic weight plasticity. Front Neural Circuits. (2016) 10:41. doi: 10.3389/fncir.2016.00041
39. McCormick DA. Spontaneous activity: signal or noise? Science. (1999) 285:541–3. doi: 10.1126/science.285.5427.541
40. Fiser J, Chiu C, Weliky M. Small modulation of ongoing cortical dynamics by sensory input during natural vision. Nature. (2004) 431:573–8. doi: 10.1038/nature02907
41. Teramae JN, Tsubo Y, Fukai T. Optimal spike-based communication in excitable networks with strong-sparse and weak-dense links. Sci Rep. (2012) 2:485. doi: 10.1038/srep00485
42. Softky WR, Koch C. The highly irregular firing of cortical cells is inconsistent with temporal integration of random EPSPs. J Neurosci. (1993) 13:334–50. doi: 10.1523/JNEUROSCI.13-01-00334.1993
43. Hromádka T, DeWeese MR, Zador AM. Sparse representation of sounds in the unanesthetized auditory cortex. PLoS Biol. (2008) 6:e16. doi: 10.1371/journal.pbio.0060016
44. Sakata S, Harris KD. Laminar structure of spontaneous and sensory-evoked population activity in auditory cortex. Neuron. (2009) 64:404–18. doi: 10.1016/j.neuron.2009.09.020
45. Destexhe A. Self-sustained asynchronous irregular states and up-down states in thalamic, cortical and thalamocortical networks of nonlinear integrate-and-fire neurons. J Comput Neurosci. (2009) 27:493. doi: 10.1007/s10827-009-0164-4
46. Vogels TP, Abbott LF. Signal propagation and logic gating in networks of integrate-and-fire neurons. J Neurosci. (2005) 25:10786–95. doi: 10.1523/JNEUROSCI.3508-05.2005
47. Guo D, Li C. Self-sustained irregular activity in 2-D small-world networks of excitatory and inhibitory neurons. IEEE Trans Neural Netw. (2010) 21:895–905. doi: 10.1109/TNN.2010.2044419
48. Samura T, Ikegaya Y, Sato YD. A neural network model of reliably optimized spike transmission. Cogn Neurodyn. (2015) 9:265–77. doi: 10.1007/s11571-015-9329-1
49. Nobukawa S, Wagatsuma N, Ikeda T, Hasegawa C, Kikuchi M, Takahashi T. Effect of steady-state response versus excitatory/inhibitory balance on spiking synchronization in neural networks with log-normal synaptic weight distribution. Cogn Neurodyn. (2021) 6:1–15. doi: 10.1007/s11571-021-09757-z
50. Song S, Miller KD, Abbott LF. Competitive Hebbian learning through spike-timing-dependent synaptic plasticity. Nat Neurosci. (2000) 3:919–26. doi: 10.1038/78829
51. Song S, Abbott LF. Cortical development and remapping through spike timing-dependent plasticity. Neuron. (2001) 32:339–50. doi: 10.1016/S0896-6273(01)00451-2
52. Izhikevich EM. Polychronization: computation with spikes. Neural Comput. (2006) 18:245–82. doi: 10.1162/089976606775093882
53. Nobukawa S, Nishimura H. Enhancement of spike-timing-dependent plasticity in spiking neural systems with noise. Int J Neural Syst. (2016) 26:1550040. doi: 10.1142/S0129065715500409
54. Nobukawa S, Nishimura H, Yamanishi T. Pattern classification by spiking neural networks combining self-organized and reward-related spike-timing-dependent plasticity. J Artif Intell Soft Comput Res. (2019) 9:283–91. doi: 10.2478/jaiscr-2019-0009
55. Masquelier T, Guyonneau R, Thorpe SJ. Spike timing dependent plasticity finds the start of repeating patterns in continuous spike trains. PLoS ONE. (2008) 3:e1377. doi: 10.1371/journal.pone.0001377
56. Asrican B, Lisman J, Otmakhov N. Synaptic strength of individual spines correlates with bound ca2+-calmodulin-dependent kinase ii. J Neurosci. (2007) 27:14007–11. doi: 10.1523/JNEUROSCI.3587-07.2007
57. Gilson M, Fukai T. Stability versus neuronal specialization for STDP: long-tail weight distributions solve the dilemma. PLoS ONE. (2011) 6:e25339. doi: 10.1371/journal.pone.0025339
58. Kuśmierz Ł, Ogawa S, Toyoizumi T. Edge of chaos and avalanches in neural networks with heavy-tailed synaptic weight distribution. Phys Rev Lett. (2020) 125:028101. doi: 10.1103/PhysRevLett.125.028101
59. Cossell L, Iacaruso MF, Muir DR, Houlton R, Sader EN, Ko H, et al. Functional organization of excitatory synaptic strength in primary visual cortex. Nature. (2015) 518:399–403. doi: 10.1038/nature14182
60. Watanabe K, Teramae Jn, Wakamiya N. Inferred duality of synaptic connectivity in local cortical circuit with receptive field correlation. In: International Conference on Neural Information Processing. Cham: Springer (2016). p. 115–22.
61. Börgers C, Kopell N. Synchronization in networks of excitatory and inhibitory neurons with sparse, random connectivity. Neural Comput. (2003) 15:509–38. doi: 10.1162/089976603321192059
62. Whittington MA, Traub R, Kopell N, Ermentrout B, Buhl E. Inhibition-based rhythms: experimental and mathematical observations on network dynamics. Int J Psychophysiol. (2000) 38:315–36. doi: 10.1016/S0167-8760(00)00173-2
63. Bartos M, Vida I, Frotscher M, Meyer A, Monyer H, Geiger JR, et al. Fast synaptic inhibition promotes synchronized gamma oscillations in hippocampal interneuron networks. Proc Natl Acad Sci USA. (2002) 99:13222–7. doi: 10.1073/pnas.192233099
64. Galambos R, Makeig S, Talmachoff PJ. A 40-Hz auditory potential recorded from the human scalp. Proc Natl Acad Sci USA. (1981) 78:2643–7. doi: 10.1073/pnas.78.4.2643
66. Hillyard SA, Mangun GR, Woldorff MG, Luck SJ. Neural systems mediating selective attention. In: Gazzaniga MS, editor. The Cognitive Neurosciences. Cambridge: The MIT Press (1995). p. 665–681.
67. Foss-Feig JH, Adkinson BD, Ji JL, Yang G, Srihari VH, McPartland JC, et al. Searching for cross-diagnostic convergence: neural mechanisms governing excitation and inhibition balance in schizophrenia and autism spectrum disorders. Biol Psychiatry. (2017) 81:848–61. doi: 10.1016/j.biopsych.2017.03.005
68. Mikanmaa E, Grent T, Hua L, Recasens M, Thune H, Uhlhaas PJ, et al. Towards a neurodynamical understanding of the prodrome in schizophrenia. Neuroimage. (2019) 190:144–53. doi: 10.1016/j.neuroimage.2017.11.026
69. O'Donnell BF, Vohs JL, Krishnan GP, Rass O, Hetrick WP, Morzorati SL. The auditory steady-state response (ASSR): a translational biomarker for schizophrenia. In: Supplements to Clinical neurophysiology. vol. 62. Amsterdam: Elsevier (2013). p. 101–12.
70. McNally JM, McCarley RW. Gamma band oscillations: a key to understanding schizophrenia symptoms and neural circuit abnormalities. Curr Opin Psychiatry. (2016) 29:202. doi: 10.1097/YCO.0000000000000244
71. Zhou TH, Mueller NE, Spencer KM, Mallya SG, Lewandowski KE, Norris LA, et al. Auditory steady state response deficits are associated with symptom severity and poor functioning in patients with psychotic disorder. Schizophr Res. (2018) 201:278–86. doi: 10.1016/j.schres.2018.05.027
72. O'Donnell BF, Hetrick WP, Vohs JL, Krishnan GP, Carroll CA, Shekhar A. Neural synchronization deficits to auditory stimulation in bipolar disorder. Neuroreport. (2004) 15:1369–72. doi: 10.1097/01.wnr.0000127348.64681.b2
73. Spencer KM, Salisbury DF, Shenton ME, McCarley RW. γ-Band auditory steady-state responses are impaired in first episode psychosis. Biol Psychiatry. (2008) 64:369–75. doi: 10.1016/j.biopsych.2008.02.021
74. Rass O, Krishnan G, Brenner CA, Hetrick WP, Merrill CC, Shekhar A, et al. Auditory steady state response in bipolar disorder: relation to clinical state, cognitive performance, medication status, and substance disorders. Bipolar Disord. (2010) 12:793–803. doi: 10.1111/j.1399-5618.2010.00871.x
75. Oda Y, Onitsuka T, Tsuchimoto R, Hirano S, Oribe N, Ueno T, et al. Gamma band neural synchronization deficits for auditory steady state responses in bipolar disorder patients. PLoS ONE. (2012) 7:e39955. doi: 10.1371/journal.pone.0039955
76. Wilson TW, Rojas DC, Reite ML, Teale PD, Rogers SJ. Children and adolescents with autism exhibit reduced MEG steady-state gamma responses. Biol Psychiatry. (2007) 62:192–7. doi: 10.1016/j.biopsych.2006.07.002
77. Rojas DC, Teale PD, Maharajh K, Kronberg E, Youngpeter K, Wilson LB, et al. Transient and steady-state auditory gamma-band responses in first-degree relatives of people with autism spectrum disorder. Mol Autism. (2011) 2:11. doi: 10.1186/2040-2392-2-11
78. Seymour RA, Rippon G, Gooding-Williams G, Sowman PF, Kessler K. Reduced auditory steady state responses in autism spectrum disorder. Mol Autism. (2020) 11:1–13. doi: 10.1186/s13229-020-00357-y
79. Gibson JR, Bartley AF, Hays SA, Huber KM. Imbalance of neocortical excitation and inhibition and altered UP states reflect network hyperexcitability in the mouse model of fragile a syndrome. J Neurophysiol. (2008) 100:2615–26. doi: 10.1152/jn.90752.2008
80. Chao HT, Chen H, Samaco RC, Xue M, Chahrour M, Yoo J, et al. Dysfunction in GABA signalling mediates autism-like stereotypies and Rett syndrome phenotypes. Nature. (2010) 468:263. doi: 10.1038/nature09582
81. Chattopadhyaya B, Di Cristo G. GABAergic circuit dysfunctions in neurodevelopmental disorders. Front Psychiatry. (2012) 3:51. doi: 10.3389/fpsyt.2012.00051
82. Glausier JR, Lewis DA. Dendritic spine pathology in schizophrenia. Neuroscience. (2013) 251:90–107. doi: 10.1016/j.neuroscience.2012.04.044
83. Guo D, Wang Q, Perc M. Complex synchronous behavior in interneuronal networks with delayed inhibitory and fast electrical synapses. Physical Review E. (2012) 85:061905. doi: 10.1103/PhysRevE.85.061905
84. Guo D, Chen M, Perc M, Wu S, Xia C, Zhang Y, et al. Firing regulation of fast-spiking interneurons by autaptic inhibition. EPL. (2016) 114:30001. doi: 10.1209/0295-5075/114/30001
85. Guo D, Wu S, Chen M, Perc M, Zhang Y, Ma J, et al. Regulation of irregular neuronal firing by autaptic transmission. Sci Rep. (2016) 6:1–14. doi: 10.1038/srep26096
86. Gu H, Hu Y, Chen X, He Y, Yang Y. Regional excitation-inhibition balance predicts default-mode network deactivation via functional connectivity. Neuroimage. (2019) 185:388–97. doi: 10.1016/j.neuroimage.2018.10.055
87. Bruining H, Hardstone R, Juarez-Martinez EL, Sprengers J, Avramiea AE, Simpraga S, et al. Measurement of excitation-inhibition ratio in autism spectrum disorder using critical brain dynamics. Sci Rep. (2020) 10:1–15. doi: 10.1038/s41598-020-65500-4
88. Ramírez-Toraño F, Bruña R, de Frutos-Lucas J, Rodríguez-Rojo I, Marcos de Pedro S, Delgado-Losada M, et al. Functional connectivity hypersynchronization in relatives of alzheimer's disease patients: an early E/I balance dysfunction? Cerebral Cortex. (2021) 31:1201–10. doi: 10.1093/cercor/bhaa286
89. Nagao N, Nishimura H, Matsui N. A neural chaos model of multistable perception. Neural Process Lett. (2000) 12:267–76. doi: 10.1023/A:1026511124944
90. Wagatsuma N, Potjans TC, Diesmann M, Fukai T. Layer-dependent attentional processing by top-down signals in a visual cortical microcircuit model. Front Comput Neurosci. (2011) 5:31. doi: 10.3389/fncom.2011.00031
91. Kanamaru T, Sekine M. Synchronized firings in the networks of class 1 excitable neurons with excitatory and inhibitory connections and their dependences on the forms of interactions. Neural Comput. (2005) 17:1315–38. doi: 10.1162/0899766053630387
92. Nobukawa S, Yamanishi T, Nishimura H, Wada Y, Kikuchi M, Takahashi T. Atypical temporal-scale-specific fractal changes in Alzheimer's disease EEG and their relevance to cognitive decline. Cogn Neurodyn. (2019) 13:1–11. doi: 10.1007/s11571-018-9509-x
93. Ando M, Nobukawa S, Kikuchi M, Takahashi T. Identification of Electroencephalogram signals in Alzheimer's disease by multifractal and multiscale entropy analysis. Front Neurosci. (2021) 15:667614. doi: 10.3389/fnins.2021.667614
94. Ando M, Nobukawa S, Kikuchi M, Takahashi T. Alteration of neural network activity with aging focusing on temporal complexity and functional connectivity within electroencephalography. Front Aging Neurosci. (2022) 14:793298. doi: 10.3389/fnagi.2022.793298
95. Nobukawa S, Ikeda T, Kikuchi M, Takahashi T. Dynamical characteristics of state transition defined by neural activity of phase in Alzheimer's disease. In: International Conference on Neural Information Processing. Cham: Springer (2021). p. 46–54.
Keywords: excitatory postsynaptic potential, log-normal distribution, spiking neural network, stochastic resonance, synchronization
Citation: Nobukawa S (2022) Long-Tailed Characteristics of Neural Activity Induced by Structural Network Properties. Front. Appl. Math. Stat. 8:905807. doi: 10.3389/fams.2022.905807
Received: 27 March 2022; Accepted: 27 April 2022;
Published: 23 May 2022.
Edited by:
Soumen Majhi, Bar-Ilan University, IsraelReviewed by:
Guanxiao Qi, Helmholtz Association of German Research Centres (HZ), GermanyCopyright © 2022 Nobukawa. This is an open-access article distributed under the terms of the Creative Commons Attribution License (CC BY). The use, distribution or reproduction in other forums is permitted, provided the original author(s) and the copyright owner(s) are credited and that the original publication in this journal is cited, in accordance with accepted academic practice. No use, distribution or reproduction is permitted which does not comply with these terms.
*Correspondence: Sou Nobukawa, bm9idWthd2FAY3MuaXQtY2hpYmEuYWMuanA=
Disclaimer: All claims expressed in this article are solely those of the authors and do not necessarily represent those of their affiliated organizations, or those of the publisher, the editors and the reviewers. Any product that may be evaluated in this article or claim that may be made by its manufacturer is not guaranteed or endorsed by the publisher.
Research integrity at Frontiers
Learn more about the work of our research integrity team to safeguard the quality of each article we publish.