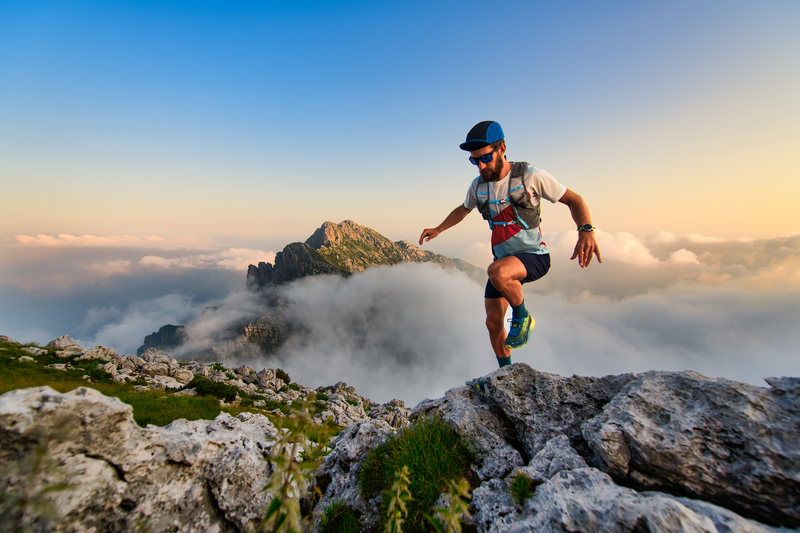
94% of researchers rate our articles as excellent or good
Learn more about the work of our research integrity team to safeguard the quality of each article we publish.
Find out more
EDITORIAL article
Front. Appl. Math. Stat. , 03 September 2021
Sec. Optimization
Volume 7 - 2021 | https://doi.org/10.3389/fams.2021.756949
This article is part of the Research Topic Data Science Applications to Inverse and Optimization Problems in Earth Science View all 9 articles
Editorial on the Research Topic
Data Science Applications to Inverse and Optimization Problems in Earth Science
Solving inverse and optimization problems that are encountered in the earth sciences is often challenging because of the computational cost of simulating models, the nonlinearity of forward models, the frequently large number of uncertain parameters or decision options and the limited information provided by data. These challenges have motivated a significant investment of effort into the development of efficient methods to improve the efficacy and reduce the overall computational costs of inversion and optimization workflows.
In recent years, the recent developments in data science (including machine learning) have attracted increased attention from researchers and practitioners from both academia and industry, for its proven ability to construct useful predictive models from large numbers of data. In comparison to data science, inverse and optimization theories have a relatively longer history within earth science. While various inverse and optimization methods have been well established and successfully applied to real-world problems, there is still room to further improve and strengthen their performance and applicability in terms of e.g., accuracy, computational efficiency, and uncertainty representation. The papers in this topic address various challenges in earth sciences, combining data science, inverse and/or optimization theories.
Gao et al. present an extension of the distributed Gauss-Newton method to optimization problems that allows for large numbers of controls by use of a limited-memory BFGS scheme. In the distributed optimization approach, multiple parameter or control solutions are simultaneously updated in an iterative manner. The updates exploit information gathered in a growing database of intermediate solutions that allows for learning from distributed data. In contrast to ensemble methods, by selecting data based on distance, solutions are able to converge towards different modes, resulting in multiple distinct solutions.
The presence of multiple modes in the posterior distribution is addressed in the context of inverse problems by Conjard and Omre. They define a selection Kalman model (SKM) as an extension of the traditional Kalman model for Gaussian distributions towards (spatially) multimodal distributions for linear-Gaussian forward models. In synthetic experiments, the SKM is found to outperform the traditional Kalman model, which tends to produce blurred distributions because of its tendency towards Gaussianity. The new approach could be the initial step towards an ensemble version that supports nonlinear forward models.
Coutinho et al. consider the reduction of computational cost for expensive model-based workflows by use of proxy models. The idea here is to replace the large online cost associated with applying iterative procedures to many model realizations by a single offline training stage in which fast models are trained using machine learning techniques. The authors consider an extension of the so-called Embed to Control (E2C) approach, introduced into the geosciences for the purpose of simulating reservoir flow by Jin et al. [1]. In particular, various options for prediction and conditioning to well data are compared and the authors demonstrate that improved predictions can be obtained relative to those obtained with previously proposed reduced-order model approaches.
Nasir et al. combine fast proxy models based on convolutional neural networks with deep-reinforcement learning techniques to inform improved solutions for optimization problems that involve decisions on where to place wells to develop subsurface reservoir systems. By considering a very large training database constructed from randomly sampled model parameters, operational constraints and economic conditions, it is expected that valid optimized results can be generated almost instantly for new scenarios within the range of training data.
Nezhadali et al. consider the use of reduced models obtained by multiple levels of domain coarsening in ensemble inversion workflows. The loss of accuracy associated with the models of multilevel fidelities could be balanced by an increased ensemble size, leading to lower Monte Carlo errors. A scheme is introduced to estimate and (approximately) account for the multilevel modelling error. The resulting workflow is applied to experiments with synthetic reservoir flow models, which suggest that the multilevel approach with error correction outperforms the conventional approach.
Fablet et al. consider the challenges associated with the sparse sampling of observation data by earth-orbiting satellites. In particular, they investigate if it is possible to learn a representation of the processes underlying the observed data that could be used to interpolate to times that are not directly observed. The interpolation problem can be formulated as an optimization problem where parameters of a model is estimated such that some measure of the variance (or energy) in the interpolated model state is minimized. The authors consider neural network representations of an energy state and apply their proposed methodology to interpolate sea surface temperature and height data.
Jiang et al. present a study in which the recently developed data-space inversion (DSI) method is extended with a parameterization technique based on a recurrent autoencoder (RAE). The DSI method enables fast updates of predictions based on new data without the need for explicit forward modelling. Instead, prior forecasts are added to the state vector and updated directly. The proposed parameterization enables a low-dimensional representation of the time series forecast data that can be seen as an alternative to more traditional PCA representations. The methodology is applied to a complex fractured reservoir system, which is operated with a detailed management logic that results in frequent changes to the wells in the reservoir.
Lin et al. investigate the impact of ensemble-based inversion on forecast degradation caused by the introduction of shocks through the update of the dynamic model state. They propose an incremental update solution, as also adopted in stochastic ensemble smoothers that are frequently applied to parameter estimation problems, but in this case for the class of deterministic filter methods such as the Ensemble Transform Kalman Filter (ETKF). The new scheme is tested with a shallow-water model.
The papers in this research topic cover a diverse range of application domains, fundamental and applied research, workflows (interpolation, inversion, optimization), and methods. They demonstrate that the earth sciences remain a fertile ground for exciting and promising new developments in advanced computational methods.
The research topic was initiated by XL. All topic editors have contributed to the formulation of the scope and the editorial process.
AE was employed by Petrobras (Brazil).
The remaining authors declare that the research was conducted in the absence of any commercial or financial relationships that could be construed as a potential conflict of interest.
All claims expressed in this article are solely those of the authors and do not necessarily represent those of their affiliated organizations, or those of the publisher, the editors and the reviewers. Any product that may be evaluated in this article, or claim that may be made by its manufacturer, is not guaranteed or endorsed by the publisher.
Keywords: data science, data assimilation, optimization, inversion, earth sciences, machine learning
Citation: Leeuwenburgh O, Emerick AA, Jafarpour B, Zhang D and Luo X (2021) Editorial: Data Science Applications to Inverse and Optimization Problems in Earth Science. Front. Appl. Math. Stat. 7:756949. doi: 10.3389/fams.2021.756949
Received: 11 August 2021; Accepted: 24 August 2021;
Published: 03 September 2021.
Edited and reviewed by:
Hong-Kun Xu, Hangzhou Dianzi University, ChinaCopyright © 2021 Leeuwenburgh, Emerick, Jafarpour, Zhang and Luo. This is an open-access article distributed under the terms of the Creative Commons Attribution License (CC BY). The use, distribution or reproduction in other forums is permitted, provided the original author(s) and the copyright owner(s) are credited and that the original publication in this journal is cited, in accordance with accepted academic practice. No use, distribution or reproduction is permitted which does not comply with these terms.
*Correspondence: Xiaodong Luo, eGx1b0Bub3JjZXJlc2VhcmNoLm5v
Disclaimer: All claims expressed in this article are solely those of the authors and do not necessarily represent those of their affiliated organizations, or those of the publisher, the editors and the reviewers. Any product that may be evaluated in this article or claim that may be made by its manufacturer is not guaranteed or endorsed by the publisher.
Research integrity at Frontiers
Learn more about the work of our research integrity team to safeguard the quality of each article we publish.