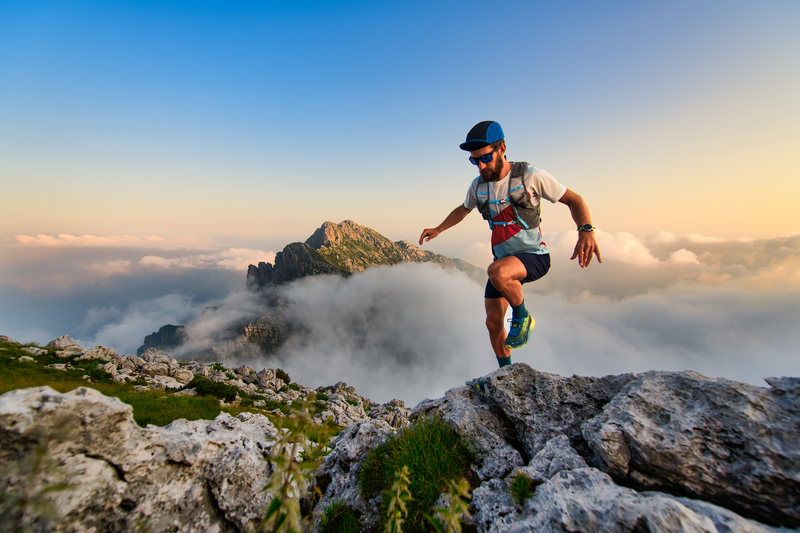
94% of researchers rate our articles as excellent or good
Learn more about the work of our research integrity team to safeguard the quality of each article we publish.
Find out more
ORIGINAL RESEARCH article
Front. Appl. Math. Stat. , 22 April 2021
Sec. Dynamical Systems
Volume 7 - 2021 | https://doi.org/10.3389/fams.2021.662221
This article is part of the Research Topic From Structure to Function in Neuronal Networks: Effects of Adaptation, Time-Delays, and Noise View all 14 articles
We analyze the influence of an external sound source in a network of FitzHugh–Nagumo oscillators with empirical structural connectivity measured in healthy human subjects. We report synchronization patterns, induced by the frequency of the sound source. We show that the level of synchrony can be enhanced by choosing the frequency of the sound source and its amplitude as control parameters for synchronization patterns. We discuss a minimum model elucidating the modalities of the influence of music on the human brain.
Synchronization phenomena are well-known regarding dynamical activities of the brain. A high degree of synchronization is related to (slow-wave) sleep [1, 2] or transitions from wakefulness to sleep [3, 4]. Recently, partial synchronization has become a clue to explain the first-night effect [5] and unihemispheric sleep [1, 6–8]. Moreover, synchronized dynamics play an important role in the dynamics of epileptic seizures [9], where the synchronization of a part of the brain causes dangerous consequences for the persons concerned. In contrast, synchronization is also used to explain brain processes which subserve for development of syntax and its perception [10–12]. In general, synchronization theory is highly important to analyze and understand musical acoustics and music psychology [13–17]. While the neurophysiological processes when listening to music remain ongoing research, it is presumed that a certain degree of synchrony can be observed while listening to music and building up expectations. Event-related potentials (ERPs), measured by electroencephalography (EEG) of participants while listening to music, show synchronized dynamics between different brain regions [18, 19]. These studies indicate that the increase of synchronization represents musical large-scale form perception. Moreover, it has been observed that areas of the whole brain are involved regarding neuronal dynamics during perception [10]. Therefore, we propose to investigate the general influence of sound on empirical brain networks. We model the spiking dynamics of the neurons by the paradigmatic FitzHugh–Nagumo model, and investigate possible partial synchronization patterns induced by an external sound source, which is connected to the auditory cortex of the human brain. Furthermore, It is a well-known fact that an important feature of musical sound perception is tonal fusion [20]. Although sound has in general a rich overtone spectrum, subjects perceive only one musical pitch which is a fusion of all partials of the spectrum. Against this background, we concentrate our general study on an external sound source with an amplitude and a single frequency, neglecting the complexity of music and its distinct effects in different frequency bands within the brain oscillations. Within the scope of this work, we have restricted ourselves to a minimal model with no node-specific behavior to reveal the impact of a periodic perturbation.
An intriguing synchronization phenomenon in networks is relay (or remote) synchronization between layers which are not directly connected, and interact via an intermediate (relay) layer [21]. The simplest realization of such a system is a triplex network where a relay layer in the middle acts as a transmitter between the two outer layers. Remote synchronization, a regime where pairs of nodes synchronize despite their large distances on the network graph, has been shown to depend on the network symmetries [22–26]. Recently the notion of relay synchronization has been extended from completely synchronized states to partial synchronization patterns in the individual layers of a three-layer multiplex network. It has been shown that the three-layer structure of the network allows for (partial) synchronization of chimera states in the outer layers via the relay layer [27–31]. Going towards more realistic models, time-delay plays an important role in the modeling of the dynamics of complex networks. In brain networks, the communication speed will be affected by the distance between regions and therefore a stimulation applied to one region needs time to reach a different region. In such delayed system, it is possible to predict if the effects of stimulation remain focal or spread globally [32]. More generally, time delays due to propagation over the white-matter tracts have been shown to organize the brain network synchronization dynamics for different types of oscillatory nodes [33]. Within the scope of this paper, we focus on the requirements for a simple model to exhibit partial synchronization patterns, which have been experimentally observed [18, 19]. Therefore, we defer the consideration of time delays for now.
We consider an empirical structural brain network shown in Figure 1 where every region of interest is modeled by a single FitzHugh–Nagumo (FHN) oscillator.
FIGURE 1. (color online) Model for the hemispheric brain structure: Weighted adjacency matrix
The weighted adjacency matrix
The structural connectivity matrices serve as a realistic input for modeling, rather than as exact information concerning the existence and strength of each connection in the human brain. The pipeline for constructing such connectivity information using diffusion tractography is known to face a range of challenges [37]. While some estimates of the strength and direction of structural connections from measurements of brain activity can in principle be attempted, the relation of these can vary dramatically with (experimentally unknown) parameters of the local dynamics and coupling function [38].
The auditory cortex is the part of the temporal lobe that processes auditory information in humans. It is a part of the auditory system, performing basic and higher functions in hearing and is located bilaterally, roughly at the upper sides of the temporal lobes, i.e., corresponding to the AAL indexing
Each node corresponding to a brain region is modeled by the FitzHugh–Nagumo (FHN) model with external stimulus, a paradigmatic model for neuronal spiking [39–41]. Note that while the FitzHugh-Nagumo model is a simplified model of a single neuron, it is also often used as a generic model for excitable media on a coarse-grained level [42, 43]. Thus the dynamics of the network reads:
where
with coupling phase
We explore the dynamical behavior by calculating the mean phase velocity
Thus
is calculated by means of an abstract dynamical phase
to estimate the general dynamical behavior of the system over time. Similarly, the temporal mean
can be considered, and compared with the spatially averaged mean phase velocity.
We investigate synchronization scenarios emerging from an external periodic stimulus in the auditory cortices of both hemispheres (
FIGURE 2. (color online) Synchronization tongues in brain network with external stimulus: (A) The temporal mean of the Kuramoto order parameter
It turns out that by taking the frequency
FIGURE 3. (color online) Dynamical scenarios: dynamics inside and outside the synchronization regions (marked as black dots in Figure 2) by the Kuramoto order parameter R (left column) and the mean phase velocities
For a better insight, Figure 4 shows the space-time plot of the variable uk for the corresponding parameter values in Figure 3. In Figures 4B,D, the dynamics inside the two synchronization regions is depicted. The perturbation in the mean phase velocity profile in the right panel of Figure 3D, can be detected also in the corresponding perturbations in Figure 4D. Comparing Figures 4A,C, we can see an increase of synchronized time segments. This increase will be analyzed quantitatively in more detail in the inset of Figure 5.
FIGURE 4. (color online) Synchronized and desynchronized dynamics: Shown are the space-time plot of the variable
FIGURE 5. (color online) Transition scenarios: (A) temporal mean of the Kuramoto order parameter
There are two frequencies which play an important role for the dynamics of the system. On the one hand, in Figure 2A a broad synchronization region is located at a frequency
where
The local dynamics of Eq. 1 is governed by a slow-fast system (FitzHugh-Nagumo oscillator), where the slow part essentially determines the period of the oscillations. Hence, considering the slow motion on the falling branches of the u-nullcline (
the time derivative of the falling branches yields with
The separation of the variables gives
where
This could explain on one side the fact that we observe a synchronization tongue at
In Figure 5A, both transitions are depicted in dependence on the frequency ω for a fixed amplitude
The existence of two synchronization regions depends on the choice to which nodes the external stimulus is supplied. In case of a different input, for instance k = 1,45 in contrast to k = 41,86, the light grey curves in Figure 5A depict the corresponding dependence of the Kuramoto order parameter
In the following, we analyze the region between the two synchronization areas in more detail. Figure 6 depicts the dynamical behavior when we approach the synchronization region by increasing the frequency ω of the external stimulus in the neighborhood of the synchronization region at ω = 2.6. For ω = 2.47 in Figure 6A, the time series of the Kuramoto order parameter shows familiar temporal fluctuations with only short episodes of synchronization
FIGURE 6. (color online) Transition scenarios: The increase of the regularity and duration of synchronized time intervals shown by the temporal evolution of the Kuramoto order parameter R (left column) and the mean phase velocities
Finally, the mean phase velocities in the right column of Figure 6 display the transition to frequency synchronization. While the frequency of the two driven nodes (k = 41, 86) converges to the frequency of an uncoupled FHN oscillator
We have investigated the influence of an external sound source on the dynamics of a network with empirical structural connectivity. It has been found that depending on the frequency and amplitude of the sound source, synchronization can be induced in the dynamics of the system. We have shown that two frequencies play an important role for synchronization, particularly the natural frequency of the uncoupled oscillator and the frequency of the coupled system. Moreover, the degree of synchronization is gradually increased when the frequency of the uncoupled oscillator or multiple values of it are approached. Furthermore, we have analyzed the linear dependence of the synchronization borders upon the amplitude of the external sound, which can also be characterized as the volume of the sound. This has resulted in the observation that the synchronization region can be enlarged by increasing volume. We have demonstrated the dynamical behavior of the system in the transition to synchronization. By tuning the frequency of the external sound appropriately, we have shown that the level of synchrony can be increased.
These results are in accordance with experiments of Bader’s group [18, 19] that music induces a certain degree of synchrony in the human brain. This group has shown that listening to music can have remarkable influence on the brain dynamics, in particular, a periodic alternation between synchronization and desynchronization. Moreover, such an alternation reflects the variability of the system; this can be seen as a critical state between a fully synchronized and a desynchronized state. It is known that the brain is operating in a critical state at the edge of different dynamical regimes [45], exhibiting hysteresis and avalanche phenomena as seen in critical phenomena and phase transitions [48–50]. By choosing appropriate parameters, we have reported an intriguing dynamical behavior regarding the transition to synchronization, and have observed the induced alternation between high and low degrees of synchronization. To sum up, an external sound source connected to the brain allows for synchronization dynamics, which may be used to model the effect of music on the human brain.
The original contributions presented in the study are included in the article, further inquiries can be directed to the corresponding author.
JS did the numerical simulations and the theoretical analysis. ES supervised the study. All authors designed the study and contributed to the preparation of the article. All the authors have read and approved the final article.
This work was supported by the Deutsche Forschungsgemeinschaft (DFG, German Research Foundation, project No. 429685422).
The authors declare that the research was conducted in the absence of any commercial or financial relationships that could be construed as a potential conflict of interest.
We are grateful to Antonín Škoch and Jaroslav Hlinka (National Institute of Mental Health, Klecany, Czech Republic) for providing the sample structural connectivity matrices, and to Rolf Bader and Lenz Hartmann (University of Hamburg) for stimulating discussions.
1. Rattenborg, NC, Amlaner, CJ, and Lima, SL. Behavioral, neurophysiological and evolutionary perspectives on unihemispheric sleep. Neurosci Biobehav Rev (2000). 24:817–42. doi:10.1016/s0149-7634(00)00039-7
2. Steriade, M, McCormick, DA, and Sejnowski, TJ. Thalamocortical oscillations in the sleeping and aroused brain. Science (1993). 262:679–85. doi:10.1126/science.8235588
3. Moroni, F, Nobili, L, De Carli, F, Massimini, M, Francione, S, Marzano, C, et al. Slow eeg rhythms and inter-hemispheric synchronization across sleep and wakefulness in the human hippocampus. Neuroimage (2012). 60:497–504. doi:10.1016/j.neuroimage.2011.11.093
4. Schwartz, JRL, and Roth, T. Neurophysiology of sleep and wakefulness: basic science and clinical implications. Curr Neuropharmacol (2008). 6:367–78. doi:10.2174/157015908787386050
5. Tamaki, M, Bang, JW, Watanabe, T, and Sasaki, Y. Night watch in one brain hemisphere during sleep associated with the first-night effect in humans. Curr Biol (2016). 26:1190–4. doi:10.1016/j.cub.2016.02.063
6. Mascetti, GG. Unihemispheric sleep and asymmetrical sleep: behavioral, neurophysiological, and functional perspectives. Nat Sci Sleep (2016). 8:221. doi:10.2147/NSS.S71970
7. Ramlow, L, Sawicki, J, Zakharova, A, Hlinka, J, Claussen, JC, and Schöll, E. Partial synchronization in empirical brain networks as a model for unihemispheric sleep. EPL (2019). 126:50007. doi:10.1209/0295-5075/126/50007
8. Rattenborg, NC, Voirin, B, Cruz, SM, Tisdale, R, Dell’Omo, G, Lipp, HP, et al. Evidence that birds sleep in mid-flight. Nat Commun (2016). 7:12468. doi:10.1038/ncomms12468
9. Gerster, M, Berner, R, Sawicki, J, Zakharova, A, Skoch, A, Hlinka, J, et al. FitzHugh-Nagumo oscillators on complex networks mimic epileptic-seizure-related synchronization phenomena. Chaos (2020). 30:123130. doi:10.1063/5.0021420
10. Bader, R.. Neural coincidence detection strategies during perception of multi-pitch musical tones. arXiv:2001 (2020). (Accessed Jan 17, 2020). 06212v1
11. Koelsch, S, Rohrmeier, M, Torrecuso, R, and Jentschke, S. Processing of hierarchical syntactic structure in music. Proc Natl Acad Sci U.S.A (2013). 110:15443. doi:10.1073/pnas.1300272110
12. Large, EW, Herrera, JA, and Velasco, MJ. Neural networks for beat perception in musical Rhythm. Front Syst Neurosci (2015). 9:159. doi:10.3389/fnsys.2015.00159
13. Bader, R.. Nonlinearities and synchronization in musical acoustics and music psychology. Berlin, BE: Springer (2013). p. 458.
14. Hou, YS, Xia, GQ, Jayaprasath, E, Yue, DZ, and Wu, ZM. Parallel information processing using a reservoir computing system based on mutually coupled semiconductor lasers. Appl Phys B (2020). 126:40. doi:10.1007/s00340-019-7351-
15. Joris, PX, Carney, LH, Smith, PH, and Yin, TCT. Enhancement of neural synchronization in the anteroventral cochlear nucleus. I. responses to tones at the characteristic frequency. J Neurophysiol (1994). 71:1022. doi:10.1152/jn.1994.71.3.1022
16. Sawicki, J, Omelchenko, I, Zakharova, A, and Schöll, E. Delay controls chimera relay synchronization in multiplex networks. Phys Rev E (2018a). 98:062224. doi:10.1103/physreve.98.062224
17. Shainline, JM. Fluxonic processing of photonic synapse events. IEEE J Sel Top Quan Electron. (2020). 26:7700315. doi:10.1109/jstqe.2019.2927473
18. Hartmann, L, and Bader, R. Neuronal synchronization of musical large scale form: an eeg-study. Proc Meetings Acoust (2014). 22:1.
19. Hartmann, L, and Bader, R.. Neural synchronization of music large-scale form.arXiv:2005 (2020). (Accessed May 14 2020). 06938v1
20. Schneider, A.. Pitch and Pitch Perception. 1st ed. Heidelberg, HH: Springer HandbooksSpringer (2018).
21. Leyva, I, Sendiña-Nadal, I, Sevilla-Escoboza, R, Vera-Avila, VP, Chholak, P, and Boccaletti, S. Relay synchronization in multiplex networks. Sci Rep (2018). 8:8629. doi:10.1038/s41598-018-26945-w
22. Bergner, A, Frasca, M, Sciuto, G, Buscarino, A, Ngamga, EJ, Fortuna, L, et al. Remote synchronization in star networks. Phys Rev E (2012). 85:026208. doi:10.1103/physreve.85.026208
23. Gambuzza, LV, Cardillo, A, Fiasconaro, A, Fortuna, L, Gómez-Gardeñes, J, and Frasca, M. Analysis of remote synchronization in complex networks. Chaos (2013). 23:043103. doi:10.1063/1.4824312
24. Nicosia, V, Valencia, M, Chavez, M, Díaz-Guilera, A, and Latora, V. Remote synchronization reveals network symmetries and functional modules. Phys Rev Lett (2013). 110:174102. doi:10.1103/physrevlett.110.174102
25. Zhang, L, Motter, AE, and Nishikawa, T. Incoherence-mediated remote synchronization. Phys Rev Lett (2017). 118:174102. doi:10.1103/physrevlett.118.174102
26. Zhang, Y, Nishikawa, T, and Motter, AE. Asymmetry-induced synchronization in oscillator networks. Phys Rev E (2017). 95:062215. doi:10.1103/physreve.95.062215
27. Drauschke, F, Sawicki, J, Berner, R, Omelchenko, I, and Schöll, E. Effect of topology upon relay synchronization in triplex neuronal networks. Chaos (2020). 30:051104. doi:10.1063/5.0008341
28. Sawicki, J, Abel, M, and Schöll, E. Synchronization of organ pipes. Eur Phys J B (2018). 91:24. doi:10.1140/epjb/e2017-80485-8
29. Sawicki, J, Omelchenko, I, Zakharova, A, and Schöll, E. Synchronization scenarios of chimeras in multiplex networks. Eur Phys J Spec Top (2018b). 227:1161. doi:10.1140/epjst/e2018-800039-y
30. Sawicki, J.. Delay controlled partial synchronization in complex networks. Heidelberg, HH: Springer ThesesSpringer (2019). doi:10.1007/978-3-030-34076-6˙5
31. Winkler, M, Sawicki, J, Omelchenko, I, Zakharova, A, Anishchenko, V, and Schöll, E. Relay synchronization in multiplex networks of discrete maps. EPL (2019). 126:50004. doi:10.1209/0295-5075/126/50004
32. Muldoon, SF, Pasqualetti, F, Gu, S, Cieslak, M, Grafton, ST, Vettel, JM, et al. Stimulation-based control of dynamic brain networks. Plos Comput Biol (2016). 12:e1005076. doi:10.1371/journal.pcbi.1005076
33. Petkoski, S, and Jirsa, VK. Transmission time delays organize the brain network synchronization. Phil Trans R Soc A (2019). 377:20180132. doi:10.1098/rsta.2018.0132
34. Melicher, T, Horacek, J, Hlinka, J, Spaniel, F, Tintera, J, Ibrahim, I, et al. White matter changes in first episode psychosis and their relation to the size of sample studied: a DTI study. Schizophr Res (2015). 162:22–8. doi:10.1016/j.schres.2015.01.029
35. Chouzouris, T, Omelchenko, I, Zakharova, A, Hlinka, J, Jiruska, P, and Schöll, E. Chimera states in brain networks: empirical neural vs. modular fractal connectivity. Chaos (2018). 28:045112. doi:10.1063/1.5009812
36. Tzourio-Mazoyer, N, Landeau, B, Papathanassiou, D, Crivello, F, Etard, O, Delcroix, N, et al. Automated anatomical labeling of activations in SPM using a macroscopic anatomical parcellation of the MNI MRI single-subject brain. Neuroimage (2002). 15:273–89. doi:10.1006/nimg.2001.0978
37. Schilling, KG, Daducci, A, Maier-Hein, K, Poupon, C, Houde, J-C, Nath, V, et al. Challenges in diffusion MRI tractography - Lessons learned from international benchmark competitions. Magn Res Imaging (2019). 57:194–209. doi:10.1016/j.mri.2018.11.014
38. Hlinka, J, and Coombes, S. Using computational models to relate structural and functional brain connectivity. Eur J Neurosci (2012). 36:2137–45. doi:10.1111/j.1460-9568.2012.08081.x
39. Bassett, DS, Zurn, P, and Gold, JI. On the nature and use of models in network neuroscience. Nat Rev Neurosci (2018). 19:566–78. doi:10.1038/s41583-018-0038-8
40. FitzHugh, R. Impulses and physiological states in theoretical models of nerve membrane. Biophys J (1961). 1:445–66. doi:10.1016/s0006-3495(61)86902-6
41. Nagumo, J, Arimoto, S, and Yoshizawa, S. An active pulse transmission line simulating nerve axon. Proc IRE (1962). 50:2061–70. doi:10.1109/jrproc.1962.288235
42. Chernihovskyi, A, and Lehnertz, K. Measuring synchronization with nonlinear excitable media. Int J Bifurc Chaos (2007). 17:3425–9. doi:10.1142/s0218127407019159
43. Chernihovskyi, A, Mormann, F, Müller, M, Elger, CE, Baier, G, and Lehnertz, K. EEG analysis with nonlinear excitable media. J Clin Neurophysiol (2005). 22:314–29. doi:10.1097/01.wnp.0000179968.14838.e7
44. Omelchenko, I, Omel’chenko, OE, Hövel, P, and Schöll, E. When nonlocal coupling between oscillators becomes stronger: patched synchrony or multichimera states. Phys Rev Lett (2013). 110:224101. doi:10.1103/physrevlett.110.224101
45. Massobrio, P, de Arcangelis, L, Pasquale, V, Jensen, HJ, and Plenz, D. Criticality as a signature of healthy neural systems. Front Syst Neurosci (2015). 9:22. doi:10.3389/fnsys.2015.00022
46. Petkoski, S, Iatsenko, D, Basnarkov, L, and Stefanovska, A. Mean-field and mean-ensemble frequencies of a system of coupled oscillators. Phys Rev E (2013). 87:032908. doi:10.1103/physreve.87.032908
47. Sawicki, J, Omelchenko, I, Zakharova, A, and Schöll, E. Delay-induced chimeras in neural networks with fractal topology. Eur Phys J B (2019). 92:54. doi:10.1140/epjb/e2019-90309-6
48. Kim, H, Moon, J-Y, Mashour, GA, and Lee, U. Mechanisms of hysteresis in human brain networks during transitions of consciousness and unconsciousness: theoretical principles and empirical evidence. Plos Comput Biol (2018). 14:e1006424. doi:10.1371/journal.pcbi.1006424
49. Ribeiro, TL, Copelli, M, Caixeta, F, Belchior, H, Chialvo, DR, Nicolelis, MA, et al. Spike avalanches exhibit universal dynamics across the sleep-wake cycle. PLoS One (2010). 5:e14129. doi:10.1371/journal.pone.0014129
Keywords: synchronization, coupled oscillators, neuronal network dynamics, pattern formation, external driven
Citation: Sawicki J and Schöll E (2021) Influence of Sound on Empirical Brain Networks. Front. Appl. Math. Stat. 7:662221. doi: 10.3389/fams.2021.662221
Received: 31 January 2021; Accepted: 26 March 2021;
Published: 22 April 2021.
Edited by:
Alessandro Torcini, Université de Cergy-Pontoise, FranceReviewed by:
Spase Petkoski, INSERM U1106 Institut de Neurosciences des Systèmes, FranceCopyright © 2021 Sawicki and Schöll. This is an open-access article distributed under the terms of the Creative Commons Attribution License (CC BY). The use, distribution or reproduction in other forums is permitted, provided the original author(s) and the copyright owner(s) are credited and that the original publication in this journal is cited, in accordance with accepted academic practice. No use, distribution or reproduction is permitted which does not comply with these terms.
*Correspondence: Jakub Sawicki, emVyZ29uQGdteC5uZXQ=
Disclaimer: All claims expressed in this article are solely those of the authors and do not necessarily represent those of their affiliated organizations, or those of the publisher, the editors and the reviewers. Any product that may be evaluated in this article or claim that may be made by its manufacturer is not guaranteed or endorsed by the publisher.
Research integrity at Frontiers
Learn more about the work of our research integrity team to safeguard the quality of each article we publish.