- 1Instituto de Telecomunicações, AP-Lr, Leiria, Portugal
- 2Polytechnic Institute of Leiria, School of Technology and Management, Agueda, Portugal
- 3Department Teoría do Sinal e Communicacións, University of Vigo, Vigo, Spain
Introduction: This paper proposes an extension of a ray-tracing based model for radiowave propagation in the presence of vegetation, to account for the wind-induced channel dynamics in vegetated environments. The original propagation model uses various point scatterers with specific re-radiation and it has been proven to be suitable for a wide range of scenarios. However, the swaying motion of the tree branches and leaves, as an effect of the wind, creates a constantly changing environment that influences the propagating radio signals in both amplitude and phase, resulting in signal level fluctuations.
Methods: A large measurement campaign intending to record and characterize the effect of wind-induced dynamics in vegetated environments, was conducted in a controlled environment, inside an anechoic chamber. Experiments included various wind directions and at varying speeds: stationary (0 m/s), low (1.9 m/s) and high (4.7 m/s). The dynamic re-radiation pattern of trees present in the radio path were recorded at 20 and 62.4 GHz signal frequencies. The available experimental data was then used to develop a statistical model which is sought to characterize wind-induced dynamics of the point scatterers’ re-radiation function. Finally, the performance of the proposed dynamic model while predicting the received signal level fluctuations inside a tree formation scenario, was assessed against dynamic directional spectra measurements conducted in a controlled environment, for four different artificially generated wind incidences and two wind speeds, low (1.9 m/s) and high (4.7 m/s).
Results and discussion: This experiment proved that the proposed elementary model could be an asset on the characterization of the time-varying effects found in vegetation areas under wind influence.
1 Introduction
The presence of trees and vegetation areas will substantially degrade radio communication system performance, causing signal attenuation (absorption), scattering, and (de)polarization (Caldeirinha, 2001). Additionally, the swaying motion of the branches and leaves as an effect of the wind, creates a constantly changing environment that influences the propagating radio signals in both amplitude and phase, resulting in small-scale signal fluctuations. These phenomena become particularly critical at higher frequencies, in which the size of leaves and smaller branches present in the canopy, becomes comparable to the propagating signal wavelength (Rogers and et al., 2002).
In recent decades, several models addressing the propagation phenomena in the presence of trees and vegetation volumes have been proposed in the literature. One of the common modelling approaches is the empirical modelling. Empirical models (Weissberger, 1982; International Radio Consultative Committee, 1986; COST235, 1995; Meng et al., 2009; Seville and Craig, 1995), which are based on simple equations and developed based on specific on-site measurements, have successfully been used to characterise the well-known “dual-slope” attenuation effect in various scenarios and frequency bands. Nevertheless, due to their site-specific nature, they usually lack of accuracy outside the model scope and, in general, these models do not account for time-variant channel properties due to wind induced dynamics.
On the other hand, full wave theoretical models using deterministic approaches (Torrico et al., 1998; Torrico and Lang, 2012; Chee et al., 2013; Wang and Sarabandi, 2005; Wang, 2006) are able to provide very reliable propagation phenomena predictions in a wide range of vegetated environments. However, such models are often very complex in nature, requiring numerical approximations to provide accurate solutions, and its true applicability in real scenarios might be difficult, since this modelling approach requires an accurate electromagnetic description of the tree geometry, including parameters such as, leaf density, area, orientation and thickness, which are prohibitively time-consuming to obtain in a real-sized forests (Wang, 2006). This time-consumption problem quickly escalates whenever the wind-induced time-variability of the radio channel is to be considered, since a huge amount of simulations would be required as well as the respective time-variant input parameter extraction.
The Radiative Energy Transfer (RET) and discrete RET (dRET) based models have also been successfully used to characterise the propagation phenomena in the presence of vegetation. Indeed, several studies presented in (Fernandes, 2007; Fernandes et al., 2005; Caldeirinha et al., 2010)used the transfer theory to predict, not only the excess attenuation caused by the presence of vegetation volumes, but also the directional spectra inside various tree formations in both in- and outdoor environments. Additionally, early work aiming at the extension of the dRET model to account for the wind-induced channel dynamics has been proposed in (Morgadinho et al., 2012), in which a Multistate Markov model was used to provide small-scale signal fluctuation. However, the applicability of the transfer theory in forest environments, seems also to be limited. On one hand, the homogeneous medium consideration given in the original RET model (Johnson and Schwering, 1985) clashes with real forests, which usually exhibit free space gaps between adjacent trees. On the other hand, the discretized RET model (Didascalou et al., 2000), had overcome the homogeneous limitation of the original transfer theory but, since it requires the discretization of the vegetation volumes into small cells, it might become numerically intractable for large propagation distances (Wang and Sarabandi, 2007).
The point scatterer formulation based model is another modelling approach for radiowave propagation in vegetation, in which point scatterers are distributed within the computational volume to characterise the trees’ impact on the radiowave signal propagation. The referred modelling approach has been successively developed, extended and extensively validated in a wide range of scenarios, including various indoor tree formation scenarios with some degree of inhomogeneity within the vegetation volume (Leonor et al., 2014; Leonor et al., 2017); outdoor line-of-trees scenarios, including both in- and out-of-leaf foliation states and inhomogeneous outdoor tree formations with mixed species (Leonor et al., 2018); and indoor tree formation scenarios with oblique/slant incidence, i.e., three-dimension (3D) scenarios (Leonor et al., 2019). However, to the best of the authors’ knowledge, the effect of the wind, and the consequent signal level fluctuations over time, has not yet been accounted in this modelling approach. To this extent, this paper aims at the extension of the point scatterer formulation into a doubly-selective model for propagation in vegetation (Leonor, 2018).
The paper is then organised as follows. In Section 2, a brief overview of the point scatterer formulation modelling approach is presented. Section 3 aims at the characterisation of the effect of wind-induced dynamics on the received signal level. To this extent, measurements intending to record the dynamic re-radiation function of a tree specimen, were conducted in a controlled environment, inside a anechoic chamber, with artificially generated wind from various directions and speeds. Subsequently, the available experimental data and its statistical analysis was used to develop an elementary dynamic model characterising the radiowave propagation phenomena of a tree under wind influence. This dynamic elementary model was developed in two different stages. In the first stage, time-variant input propagation parameters were extracted directly from dynamic re-radiation measurements, yielding to a very reliable description of the signal level variations, specially in the forward scattering direction. However, its application to tree formation scenarios is cumbersome due to the synchronous nature of the input propagation parameters extraction method. To this extend a second dynamic model, based on well-known statistical distributions was proposed. Despite some limitations identified in this second method, it enabled the modelling approach to be applicable in complex vegetation structures, such as tree formations. The assessment of the statistical based dynamic model while predicting the received signal level fluctuations in a tree formation scenario is presented in Section 4. The performance of the proposed doubly-selective model was assessed against dynamic directional spectra measurements conducted inside an anechoic chamber, for four different artificially generated wind incidences and two wind speeds. Finally, Section 5, addresses some conclusions and raises directions for further work.
2 Original model overview
The ray-tracing based model proposed in (Leonor et al., 2014), and latter enhanced in Leonor et al. (2018) and in Leonor et al. (2019), uses point scatterer formulation to characterize the radiowave propagation phenomena of trees. In this model, trees present in the simulation radio channel are characterized using a set of point scatterers, which are distributed in various circles with varying heights and widths, providing a relatively homogeneous point scatterer distribution across the vegetation volume. A detailed description on algorithm defined for the point scatterer distribution, which will generate sort of a sphere which dimensions are dependent on the canopy height and width, can be found in Leonor et al. (2019). Figure 1 depicts an example of a small tree modelled using the point scatterer distribution.
To gather the interactions between all point scatterers present in the simulation volume a two-iteration ray-tracing algorithm is used. The first iteration of ray launching includes the direct component and also the rays travelling from the transmitter (TX) toward the scattered points and bounced back to the receiver (RX). In the second iteration, point scatterers illuminated with the rays of first iteration are assumed as new TXs, and the rays travelling from each point toward the other scatterers and subsequently bounced to the RX are included. Finally, the received signal complex envelope is calculated by coherently adding all the multipath components arriving at the receiving antenna, for each time-step.
Each tree, and its respective point scatterers, is characterized by a set of input propagation parameters, which includes.
•
•
The point scatterers’ re-radiation function is based on the normalized version of the well-known phase function model, originally presented in Ulaby et al. (1990), which is expressed by
where
Equation 1 is derived for two-dimension (2D) scenarios, however, based on the measurement results presented in Leonor et al. (2019), it was concluded that the symmetry around the forward scattering direction is found, not only in the azimuth plane, but also in the elevation plane. Therefore, to characterize the tree scattering phenomena in 3D scenarios, the
Additionally, rays travelling through the vegetation volume will account for excess attenuation. Considering a generic scenario composed by
where,
As far as input propagation parameter extraction is concerned, an empirical method was proposed in (Leonor et al. (2018)), which is based on two simple measurements that can be relatively easily conducted in an outdoor forest environment. The aim of such measurements, which are to be taken with the RX at positions
and
where
3 Measurement system overview
Measurements intending to record time-varying received signal level, caused by the swaying motion of the tree branches, twigs and leafs, were carried out at 20 and 62.4 GHz.
The 20 GHz measurement system, depicted in Figure 3 a through block diagram, consists of a Gunn diode voltage controlled oscillator (VCO), providing a continuous-wave (CW) tone of 20 GHz rated at 20 dB m, which is radiated to the radio path using a 10 dBi standard horn antenna with a half-power beamwidth (HPBW) of approximately
The 62.4 GHz measurement system was built using a phase locked loop oscillator (PLO) composed by a Gunn diode oscillator tuned at 20.8 GHz and a frequency multiplier used to triple the signal frequency. The ensemble oscillator-multiplier generates a RF signal tuned at 62.4 GHz and rated at 16 dB m. This RF signal is transmitted to the radio path over a waveguide attenuator and a 25 dBi standard horn antenna with a HPBW of approximately
At the RX end, a similar 25 dBi standard horn antenna was used to acquire the RF signal, which in turn, was transmitted to a frequency down-conversion set, using a waveguide attenuator. The frequency conversion stage consists of a balanced mixer and a VCO operating and 61.8 GHz, which converts the RF signal into a 600 MHz IF signal. The IF component is then amplified by a 44 dB LNA and filtered by a band-pass filter (BPF) centred at 600 MHz with a 5.38 MHz of bandwidth (−3 dB). Similarly to the 20 GHz measurement system, the IF signal was acquired by a LogAmp and DAQ.
The dynamic range specifications of the 20 and 62.4 GHz measurements system are summarized in Table 1. The noise levels of both systems were set by the LogAmps sensitivity and overall dynamic ranges of 147.8 and 170 dB were found for the 20 and 62.4 GHz measurements system, respectively. Although the saturation of the RX active devices is not considered here, during the actual course of the measurements it was prevented by means of the introduction of additional attenuation at the RX whenever it was needed.
4 Extension of the 3D ray-tracing based model to doubly-selective vegetated scenarios
In this section, the 3D ray-tracing based model for propagation through vegetation, presented in Leonor et al. (2019), is extended to include the time-varying received signal level, caused by the swaying motion of the tree branches, twigs and leafs. The development of this model extension was based on dynamic re-radiation measurements conducted in a controlled environment, inside an anechoic chamber, which included artificially generated wind from various directions and speeds, at 20 and 62.4 GHz signal frequencies.
A primary model validation is also presented in this section, including the comparison between both measured and simulated time-series of the received signal level, and the comparison of its respective first and secondary order statistics, namely, the cumulative distribution function (CDF), the probability density function (PDF), the average fade duration (AFD), the level crossing rate (LCR) and the autocorrelation function (ACF) received data samples obtained at the various conditions under consideration.
4.1 Methodology and measurement geometry
Measurements intending to record the time-varying re-radiation pattern of a single trees, were conducted inside an anechoic chamber, at 20 and 62.4 GHz. A TX was placed at a distance of 1.70 m from the centre of the tree under measurement (TUM), which is sufficient to ensure plane wave illumination, and the RX was made to rotate around the tree, from
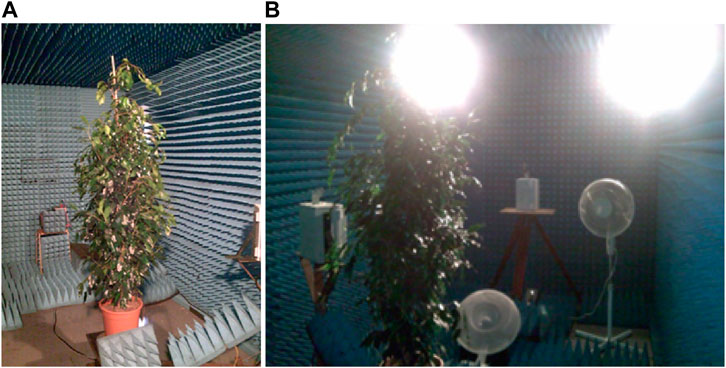
Figure 6. Picture of the measurement scenario: (A) Ficus tree inside the anechoic chamber; and (B) Tree measurement depicted from the receiver side with visible setups of wind fan at wind positions defined as A and B.
Considering the six wind directions (A to F), the three wind speeds (static, low and high), the angular range of the RX rotation (i.e.,
4.2 Measurement results and statistical analysis
An in-depth statistical analysis on the dynamic measurement results has been conducted. This analysis included the evaluation of the mean and standard deviation (STD) values of the 4,356 different time-series. The normalised mean signal level, with respect to the free space, obtained for wind incidences A and C at 20 GHz are depicted in Figure 7A) and b), respectively, while the same data obtained at 62.4 GHz is depicted in Figure 7C) and d). Figure 8 displays the STD values for same measurement scenarios. As may be observed, there is no evident influence of wind speed on the recorded STD values. Notwithstanding, given the relatively reduced size of the vegetation volume, and the low wind speeds artificially induced inside the anechoic chamber (less that 5 m/s), the obtained STD values are in line with the current International Telecommunication Union - Radiocommunication Sector (ITU-R) recommendation (Assembly, 2013), where STD variations of less than 1 dB were found for wind speeds of 5 m/s.
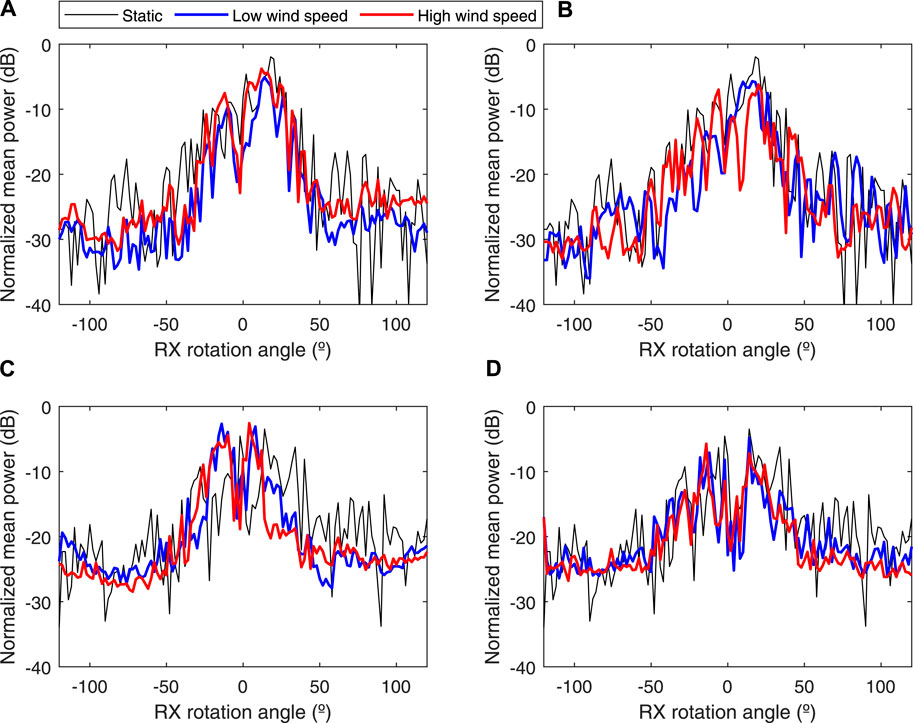
Figure 7. Normalised mean signal level for wind incidences: (A) A at 20 GHz; (B) C at 20 GHz; (C) A at 62.4 GHz; and (D) C at 62.4 GHz.
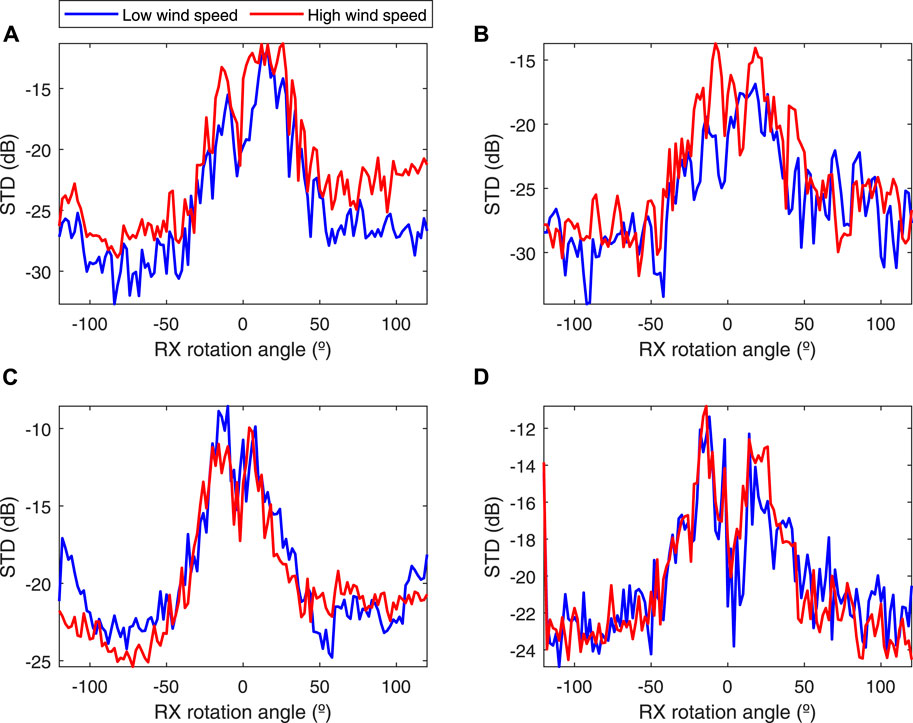
Figure 8. Standard deviation of the signal level for wind incidences: (A) A at 20 GHz; (B) C at 20 GHz; (C) A at 62.4 GHz; and (D) C at 62.4 GHz.
Based on the tree re-radiation pattern and the bi-static scattering angle, three propagation regions can be defined as the RX moves around the vegetation volume in the azimuthal plane. These are (Caldeirinha, 2001): forward scattering
4.3 Propagation model input parameter analysis
Given the availability of the time-series obtained at both RX positions required to apply empirical models (4) and (5), i.e.,
This method was able to provide remarkably accurate representation of the measured signal variability in the forward scattering direction. However, the time-varying
This analysis consisted of analysing the influence of the
where
Using the dynamic measurement data
The results obtained for the normalised power level (NPL) in the forward scattering region for a wind incidence from: a) B with low speed and b) F with high speed at 20 GHz; and c) C with low speed and d) E with high speed at 62.4 GHz, are depicted in Figure 10. As referred previously, the results obtained in the forward scattering direction yielded to a very faithful representation of the measured time-series, since
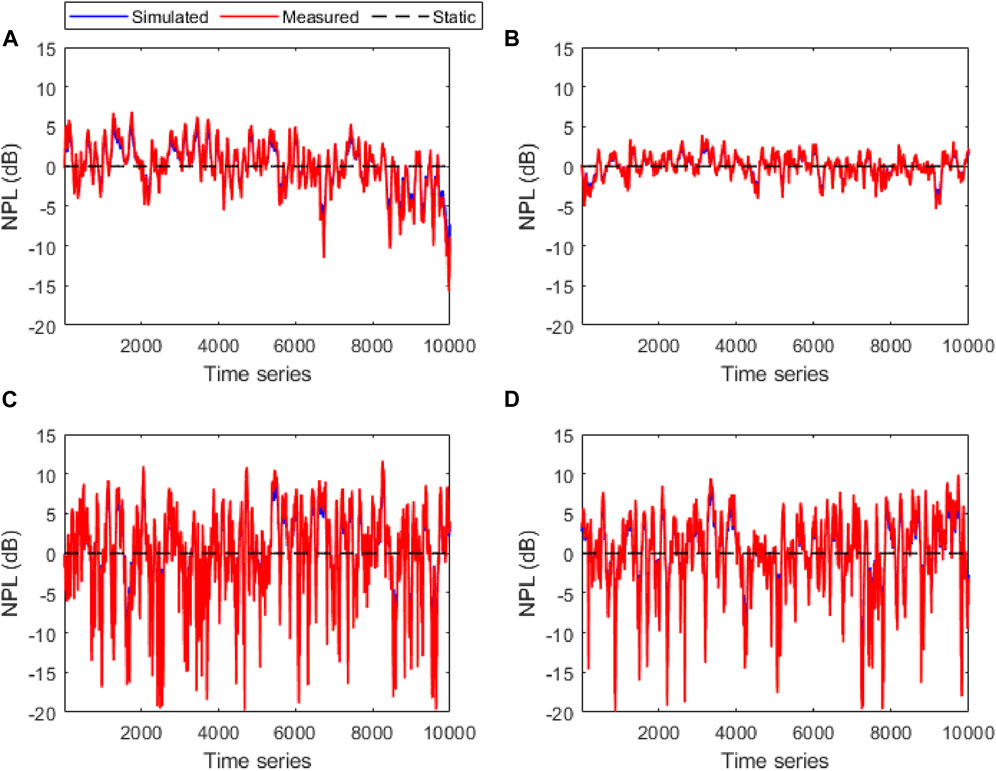
Figure 10. Time series obtained in the forward scattering direction
The results with same wind conditions obtained in the side scattering region are depicted in Figure 11. Despite the fact that the time-series representation provided by the proposed model is not as reliable as that obtained in the forward scattering region, the introduction of the time-varying
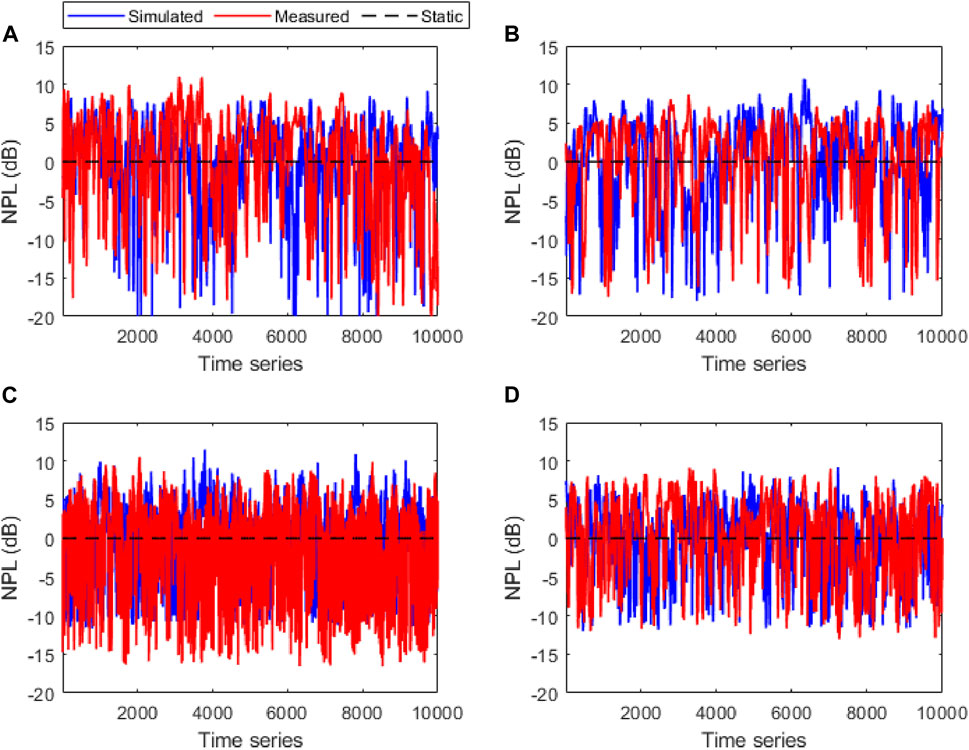
Figure 11. Time series obtained in the side scattering direction
The results provided by the proposed dynamic elementary model have been further analysed using first and second order statistics. These include the computation of CDF, PDF, AFD, LCR and ACF of both measured and simulated time-series, for all the scenarios taken into consideration. The results obtained in the forward scattering region
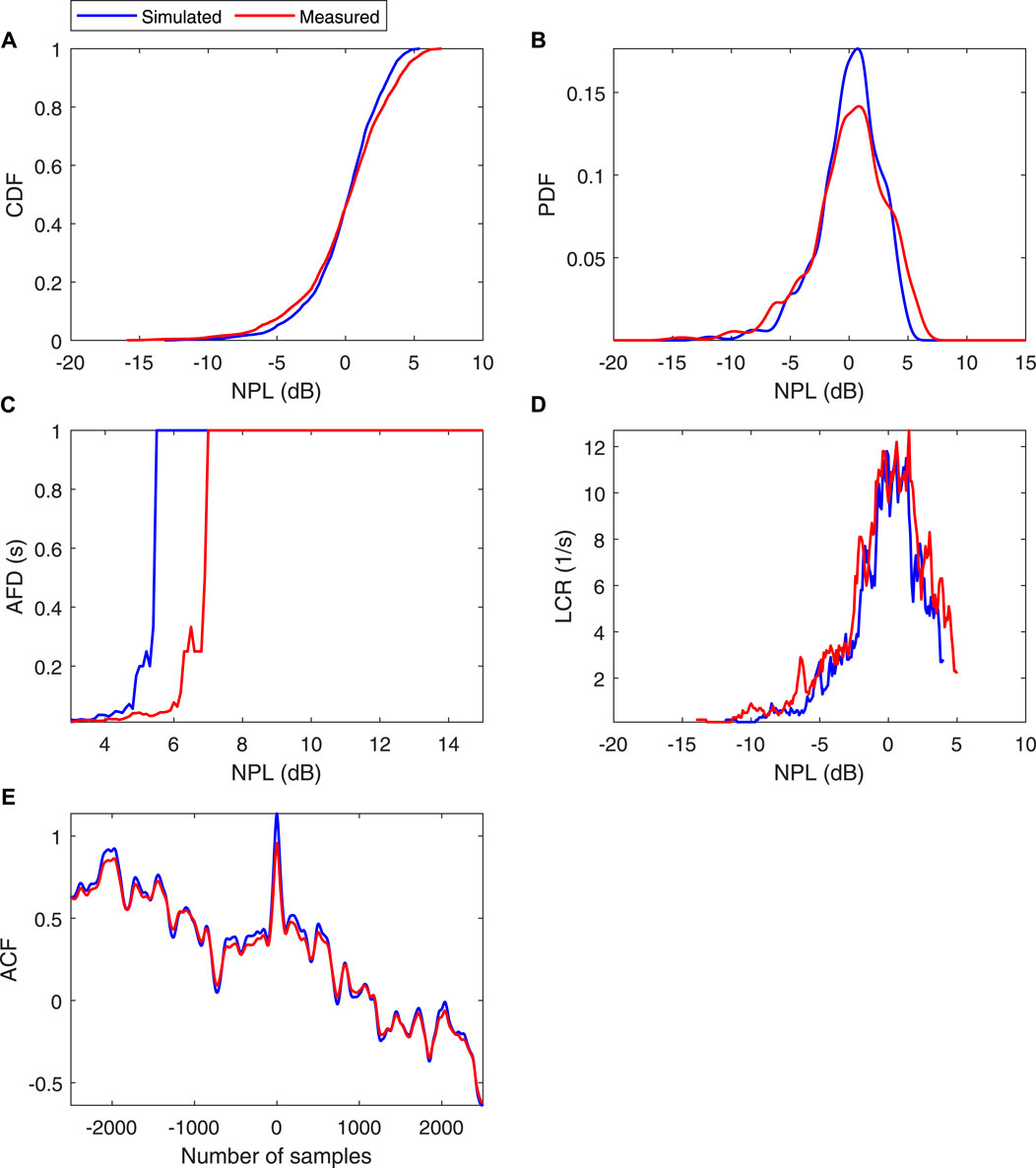
Figure 12. Statistical representation relative to the results obtained in the forward scattering direction
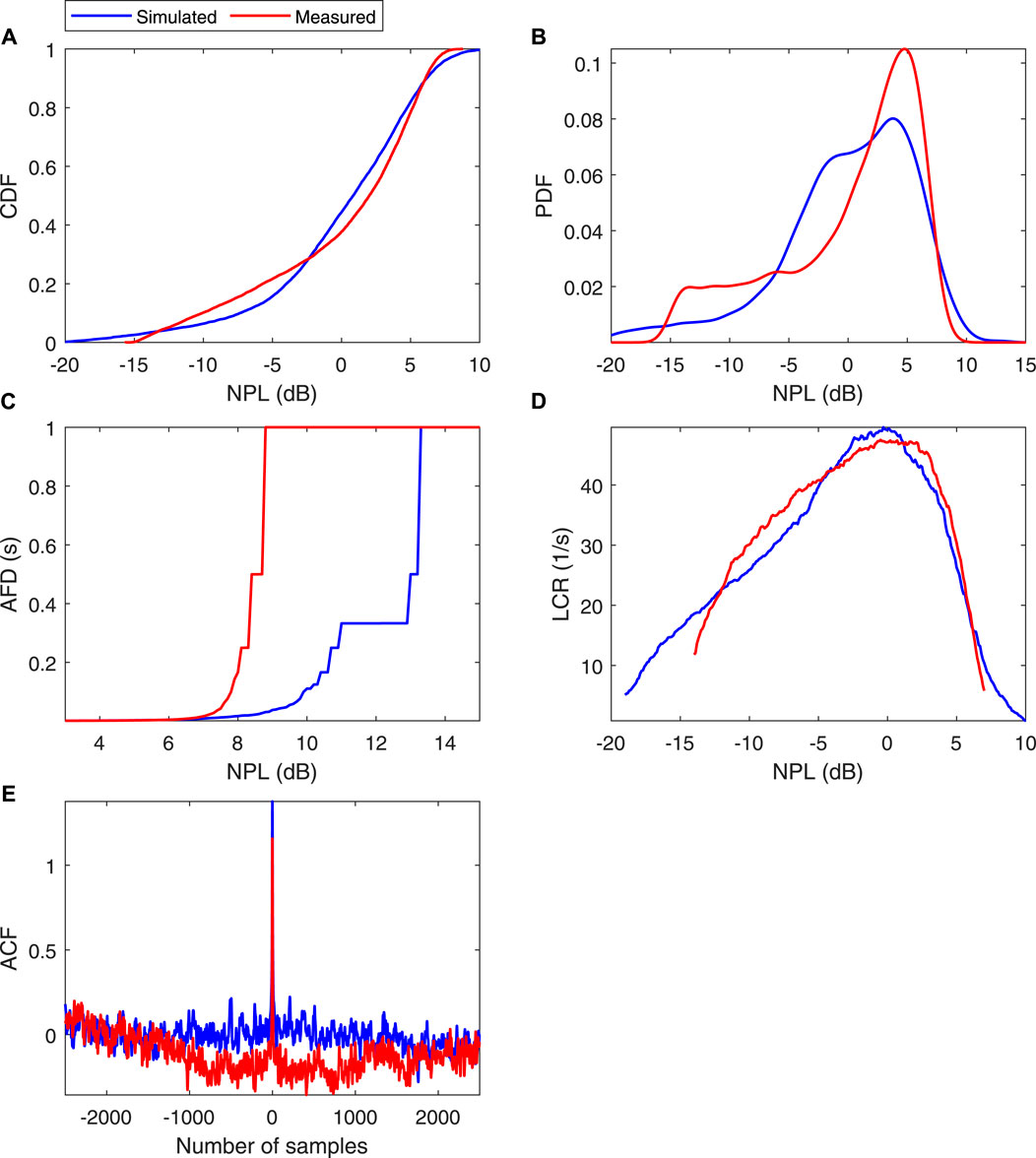
Figure 13. Statistical representation relative to the results obtained in the side scattering direction
Finally, the Kolmogorov-Smirnov (KS) test was used to assess the degree of similarity between the distribution functions of both measured and simulated time-series of the received signal level. Due to the huge amount of available data, the performance of the proposed model was evaluated in terms of averaged KS test result. Table 2 depicts the averaged KS test results obtained between both measured and simulated time-series. The averaged KS test result was consistently above the 80%, which is a fair good approximation, indicating that the signal level variation caused by the presence of the wind is being properly characterized by the proposed propagation model.
4.4 Statistical dynamic model development
Despite the fact that the time-variant input propagation parameters
This might cumbersome the model applicability in more complex scenarios, such as tree formations, in which trees present in a formation should primarily be characterised individually, and the extracted input propagation parameters are subsequently used in the ray-tracing algorithm allowing, not only the prediction of the dynamic properties of each individual tree, but also the interactions between different trees present in the simulation channel.
For that purpose, the statistics of the time-variability of the input parameters obtained in the previous section have been further investigated, yielding at the development of a statistical based model, in which the input propagation parameter are defined using random variables with properties of known distributions. This analysis consisted of using the KS test to assess the degree of similarity between the time-varying input parameters with known statistical distributions. With this analysis, it was concluded that the statistical distribution which better characterises the
Given the high degree of similarity found between the input parameter variation and these known distributions, the second part of this statistical analysis consisted of finding the specific input values for the these distributions which may generate values for
The subsequent section will address the performance analysis of this statistical model while characterising the signal level variations found for single and isolated trees under wind influence. To this extent, the input propagation parameters will be defined using the values obtained previously, i.e., acording to Equations 7–9.
where
4.5 Statistical dynamic model validation
The statistical distributions were used to characterise the input propagation parameters time-variability for all the dynamic re-radiation measurement scenarios taken into consideration, that is, wind incidences from A to F, low and high wind speeds, and at 20 and 62.4 GHz signal frequencies. Figure 14 depicts the results obtained at the forward scattering direction for a wind incidence from: a) B with low speed and b) F with high speed, at 20 GHz; and c) C with low speed and d) E with high speed, at 62.4 GHz. Since the model input parameters are now characterised by random variables and hence, they are not extracted directly from the time-varying tree insertion loss, the agreement between both measured and simulated time-series is not as great as that provided by the original dynamic model. This is due to the fast variation nature of a random variable. Similar behaviour was found for the side scattering region results depicted in Figure 15.
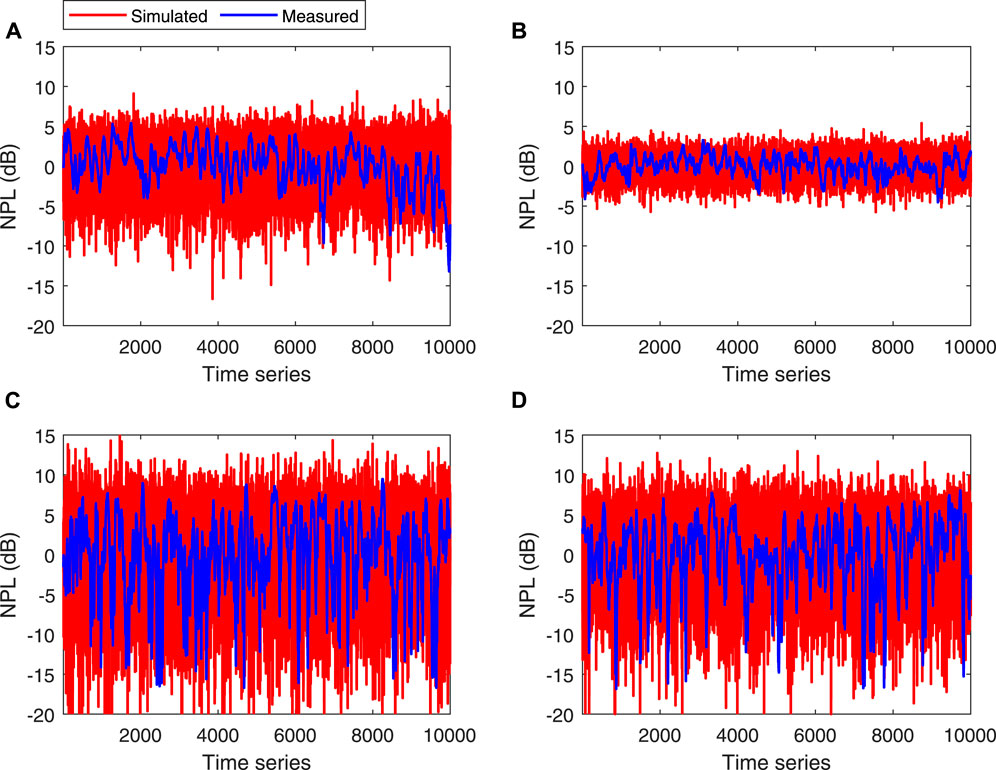
Figure 14. Time series obtained in the forward scattering direction
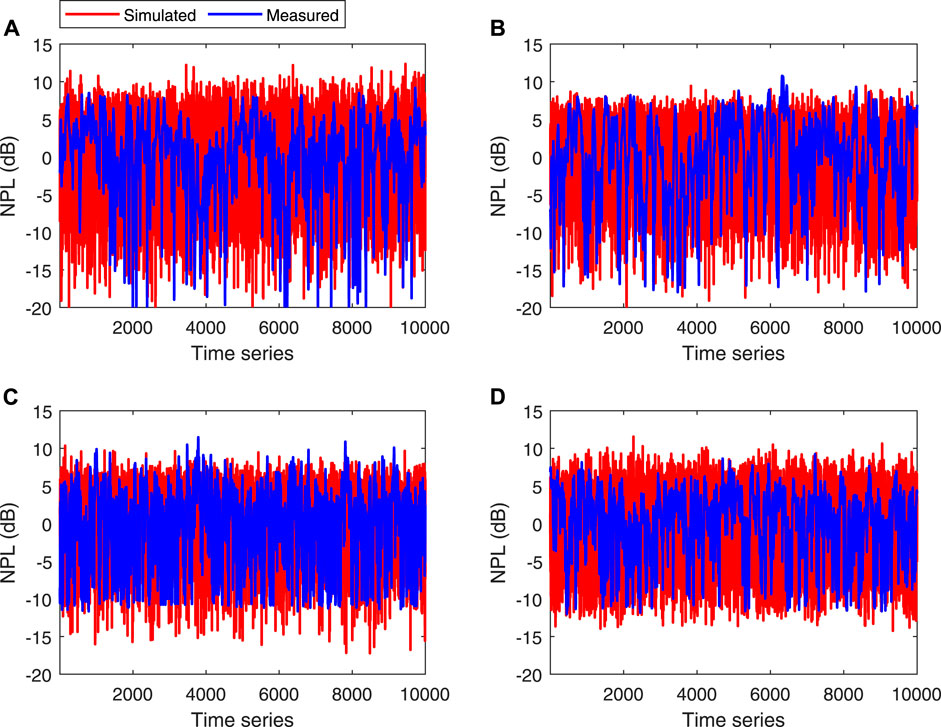
Figure 15. Time series obtained in the forward scattering direction
This lack of accuracy on the simulated time-series, specially found in the LCR and ACF analysis, suggests that the input propagation parameters should not be characterised using simple random distribution methods. Nevertheless, despite the overrated signal variation provided by the statistical model, the signal level variations of both measured and simulated time-series are roughly between same level range.
The performance of this statistical based dynamic model have been further analysed, including the evaluation of the first and second order statistics parameters, namely, the CDF, PDF and AFD. LCR and ACF parameters were not included due to the model limitation referred previously. Figure 16 depicts the statistical representation of the results obtained at the forward scattering direction
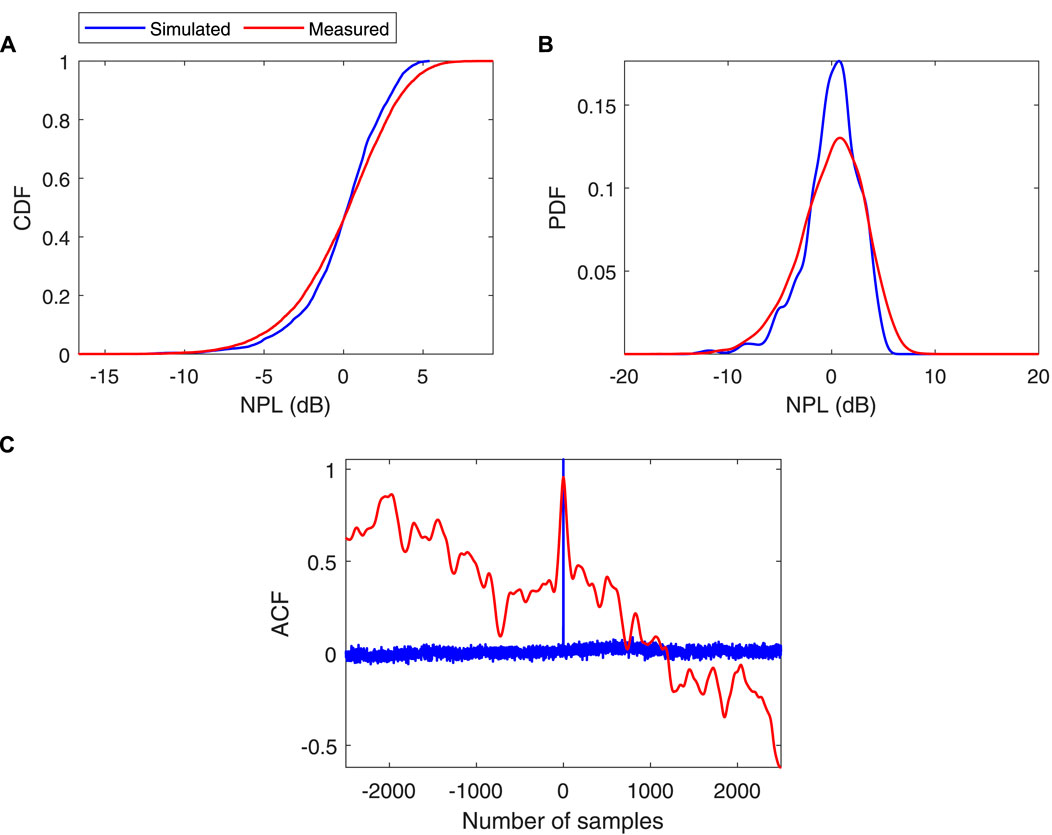
Figure 16. Statistical representation of the results obtained in the forward scattering direction
Similar model performance was found at the side scattering region, in which the simulated signal level is highly affected by the
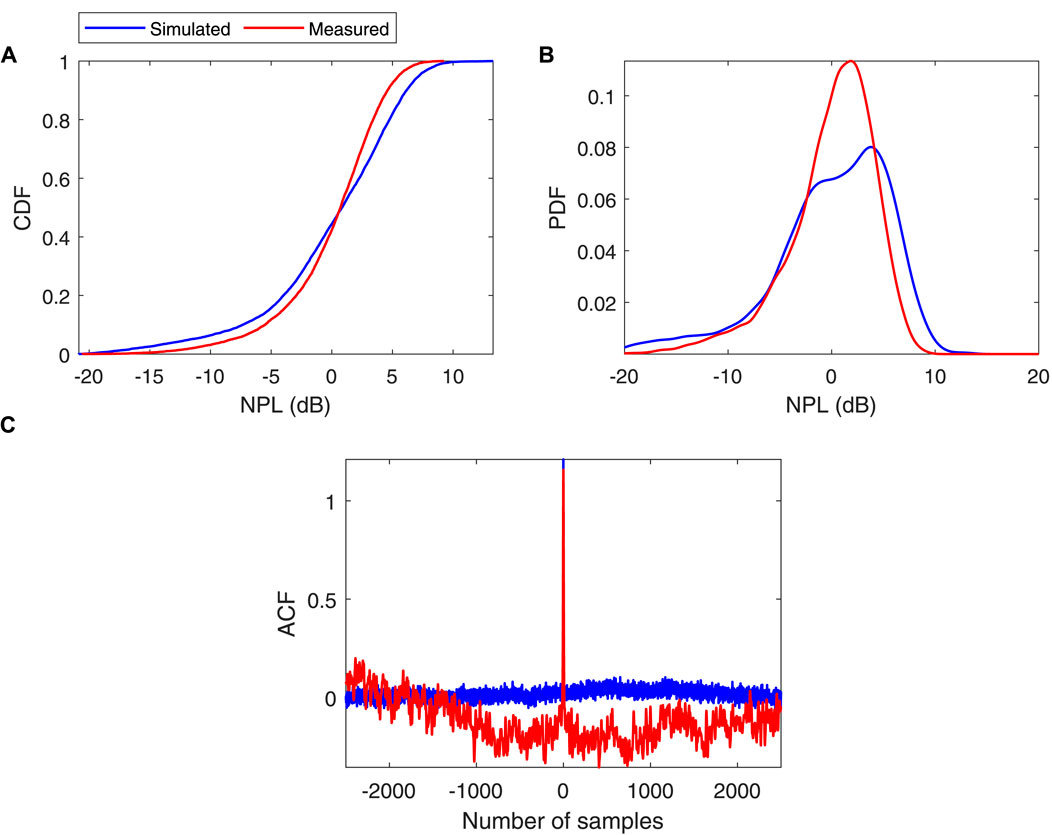
Figure 17. Statistical representation of the results obtained in the side scattering direction
For an overview of the overall model performance, the CDF and ACF statistics will be shown in three dimensions accounting for the RX rotation angle, fade or threshold levels and respective statistic magnitude. These will provide an insight view on the overall model performance while predicting the specific time-series with regard to all of the RX rotation angles. Results depicted in Figure 18 concerns to the measured and simulated data obtained with wind incidence from B, with low wind speed, at 20 GHz, whereas the results obtained wind incidence from B, with low wind speed, at 62.4 GHz, are depicted in Figure 19.
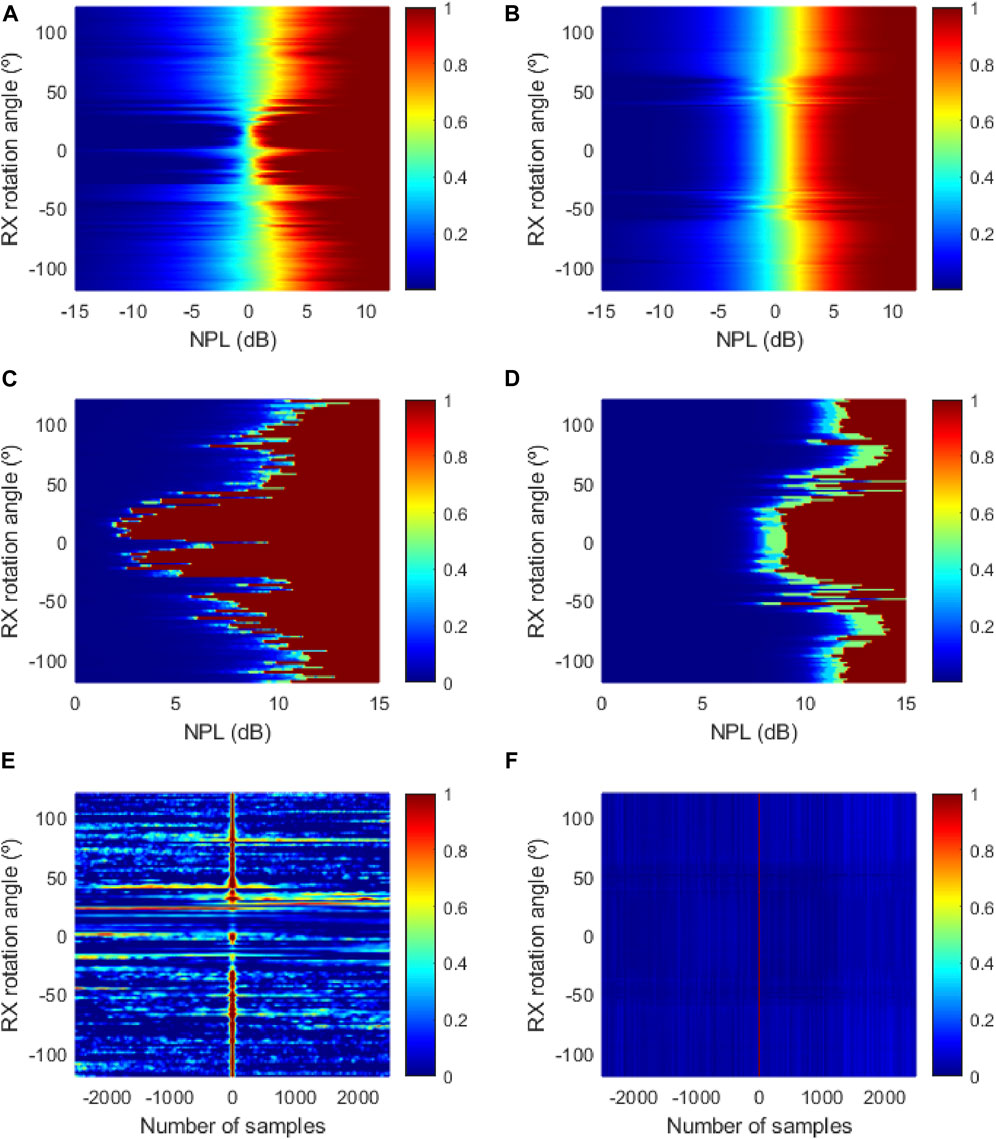
Figure 18. Statistical representation of both measured (left) and simulated (right) of the results obtained with wind incidence from B, low wind speed at 20 GHz. (A) Measured CDF of NPL (B) Simulated CDF of NPL (C) Measured AFD (D) Simulated AFD (E) Measured ACF (F) Simulated ACF.
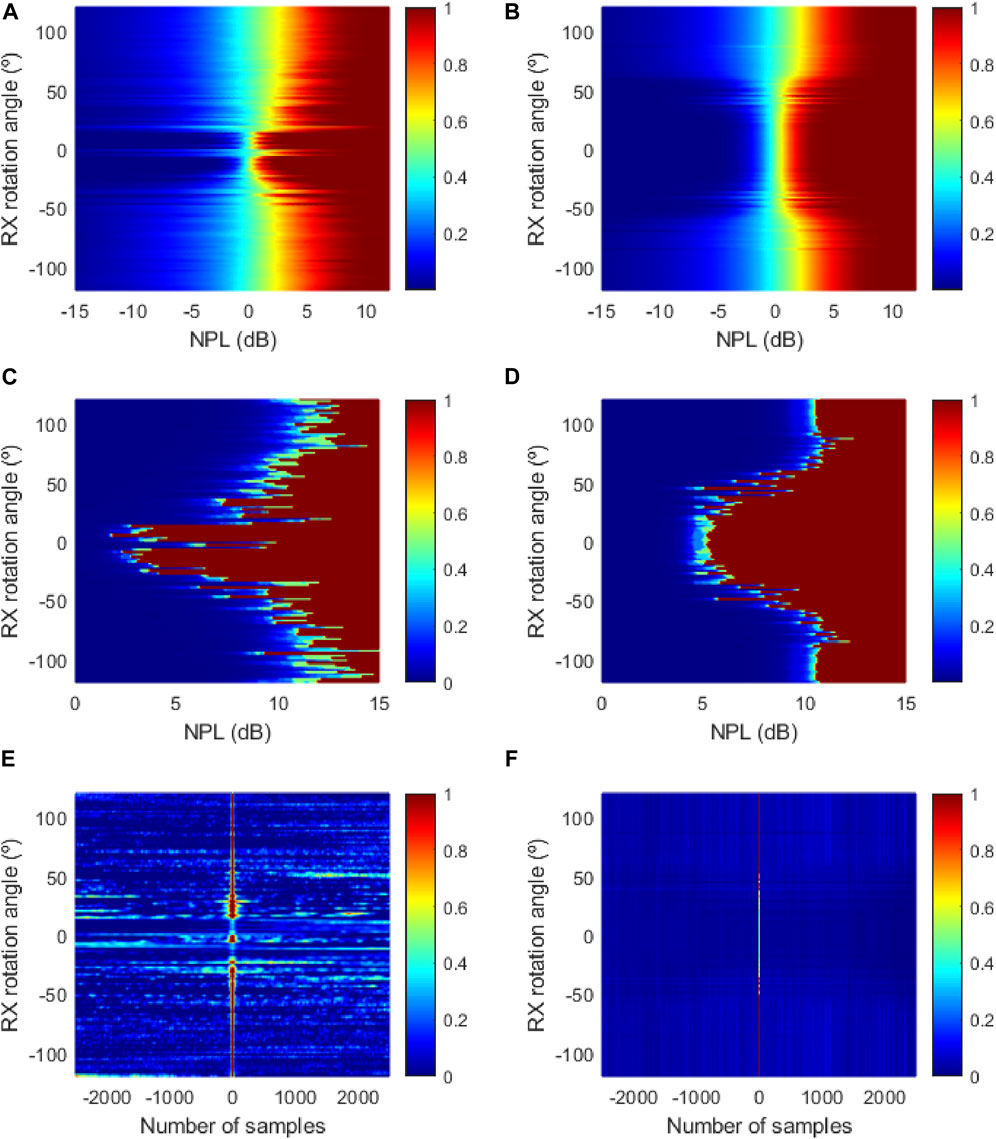
Figure 19. Statistical representation of both measured (left) and simulated (right) of the results obtained with wind incidence from B, low wind speed at 62.4 GHz. (A) Measured CDF of NPL (B) Simulated CDF of NPL (C) Measured AFD (D) Simulated AFD (E) Measured ACF (F) Simulated ACF.
Finally, the simulated time-series were compared against measurement results, using the KS test. Due to the huge amount of available data, the performance of the proposed model was evaluated in terms of averaged KS test result. Table 3 depicts the averaged KS test results obtained between both measured and simulated time-series. The averaged KS test result was consistently above the 80%, which is a fair good approximation.
As a final remark to the extension of the point scatterer formulation to include wind-induced dynamics, it is worth to emphasise that the original dynamic model using the input propagation parameters
5 Performance analysis in tree formation scenarios under wind influence
This section aims at the validation of the proposed statistical model in more complex scenario, such as tree formation, in which the ray-tracing based simulation platform must, not only to account the time-variability of the received signal level, caused by the swaying motion the branches, twigs and leafs of each tree, but also to account for the signal interaction between different trees and vegetation volumes. To this extend, the proposed statistical model was used to characterise the input propagation parameters
5.1 Measurement geometry
Figure 20 depicts the measurement geometry adopted for validation purposes, where the TX was located at 1.4 m from the vegetation structure, composed by 5 Ficus benjamina trees disposed in a
The dynamic directional spectra measurements were conducted in a controlled environment, inside an anechoic chamber, for four different artificially generated wind incidences, A to D, two wind speeds, i.e., low and high, and at 20 and 62.4 GHz signal frequency.
5.2 Simulation geometry
To assess the generic doubly-selective 3D vegetation model based on the point scatterer formulation, a simulation geometry similar to that depicted in Figure 20 was defined. To this extent, the TX was set to a distance of 1.4 m from the first row of trees, represented by the green circles in Figure 21, and four different RX positions were defined according with the measurement geometry. At each one of the RX positions, the
The dynamic re-radiation function of each individual tree was not available, therefore the detailed characterisation of the trees present in the simulation channel could not be established. However, all the trees used in the tree formation presented similar characteristics, i.e., height, radius, leaf density, and hence, the statistical parameters obtained for the Tree#1 in Section 4.4 at 20 GHz, were used to model all the trees in the simulation geometry.
5.3 Measurement results and analysis
As referred previously, the statistical based dynamic model overestimates the signal level variation rate, due to the very-fast variation nature of a random variable. Hence, the model performance analysis will be mostly focused in the signal level variation range of both measured and simulated time-series, e.g., CDFs, rather than their variation rate.
Figure 22 depicts both the measured and simulated results obtained at RX position RX1. In this position the RX is in LOS, however, when the RX is pointed to the Tree#2, i.e.,
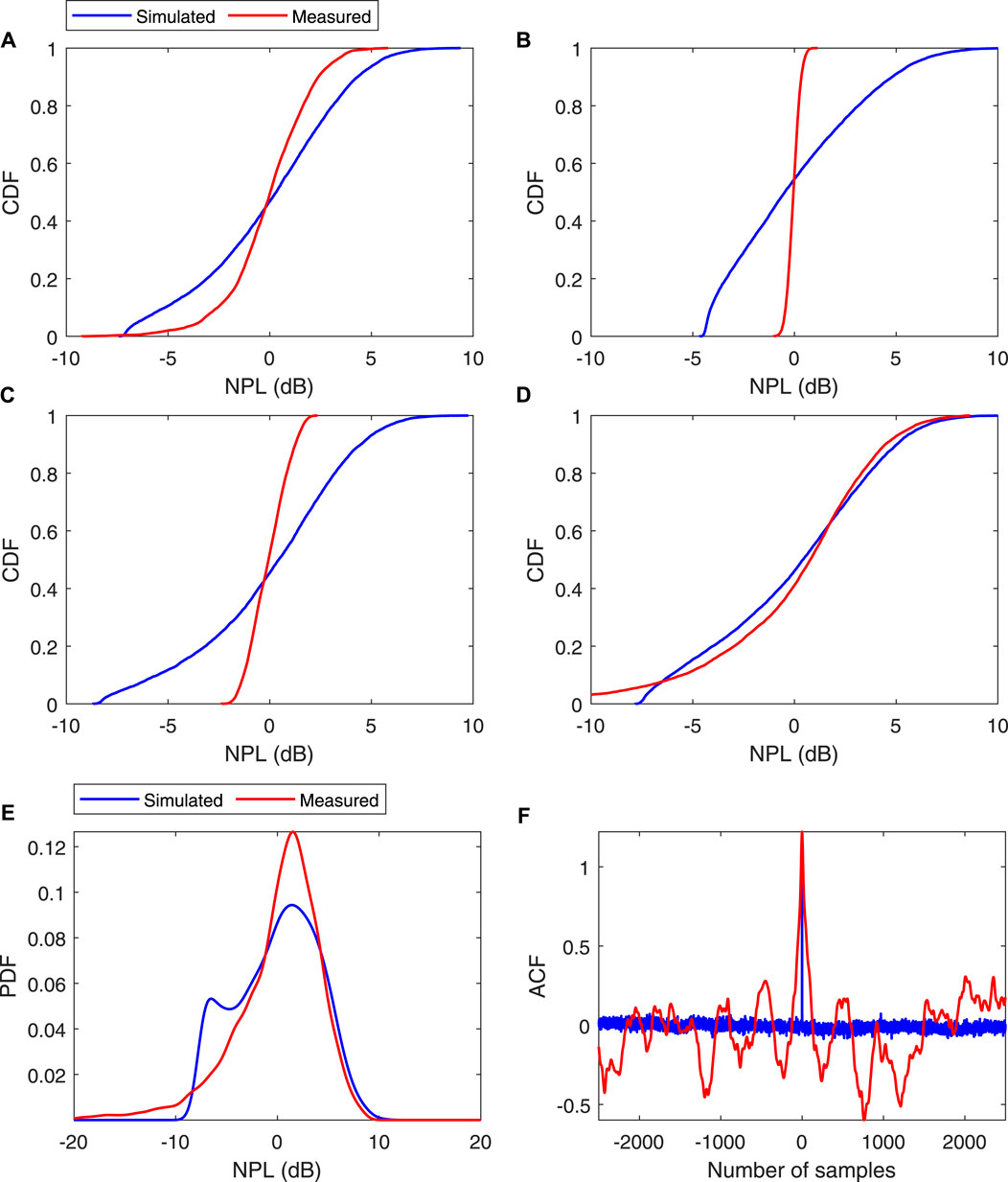
Figure 22. Results obtained for RX position RX1
This is related with the fact that all the trees in the simulation channel were modelled using time-varying input parameters, i.e., all the trees are assumed to be under wind influence. However, during the wind incidences from B and C, the Tree#2, the one that affects the measurement results the most, is covered by Tree#5 and Tree#4, respectively. Hence, the wind speeds arriving at the Tree#2 are weakened. A similar phenomenon was found for wind incidence from A, in which the wind speed arriving striking the Tree#2 is minimised by the Tree#1. Finally, the wind incidence from D strikes directly on Tree#2, hence, it will cause an enhanced swaying motion the tree branches, twigs and leafs, contributing to an increase of the signal level variation.
A more detailed analysis on the model accuracy while predicting the dynamic effect of the motion of the swaying trees, is depicted in Figure 22E) and f), where the statistical representation of the data recorded in RX position RX1, when pointed at Tree#2, i.e.,
The results obtained at RX2 when pointed directly to the TX, i.e.,
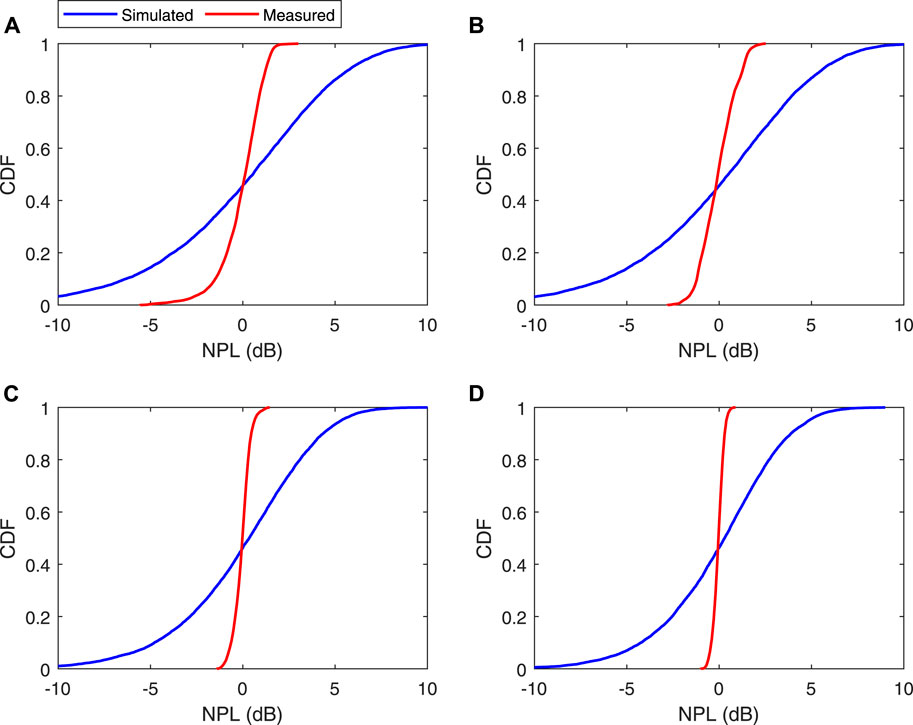
Figure 23. Results obtained for RX position RX2
When the RX2 is pointed at Tree#3, i.e.,
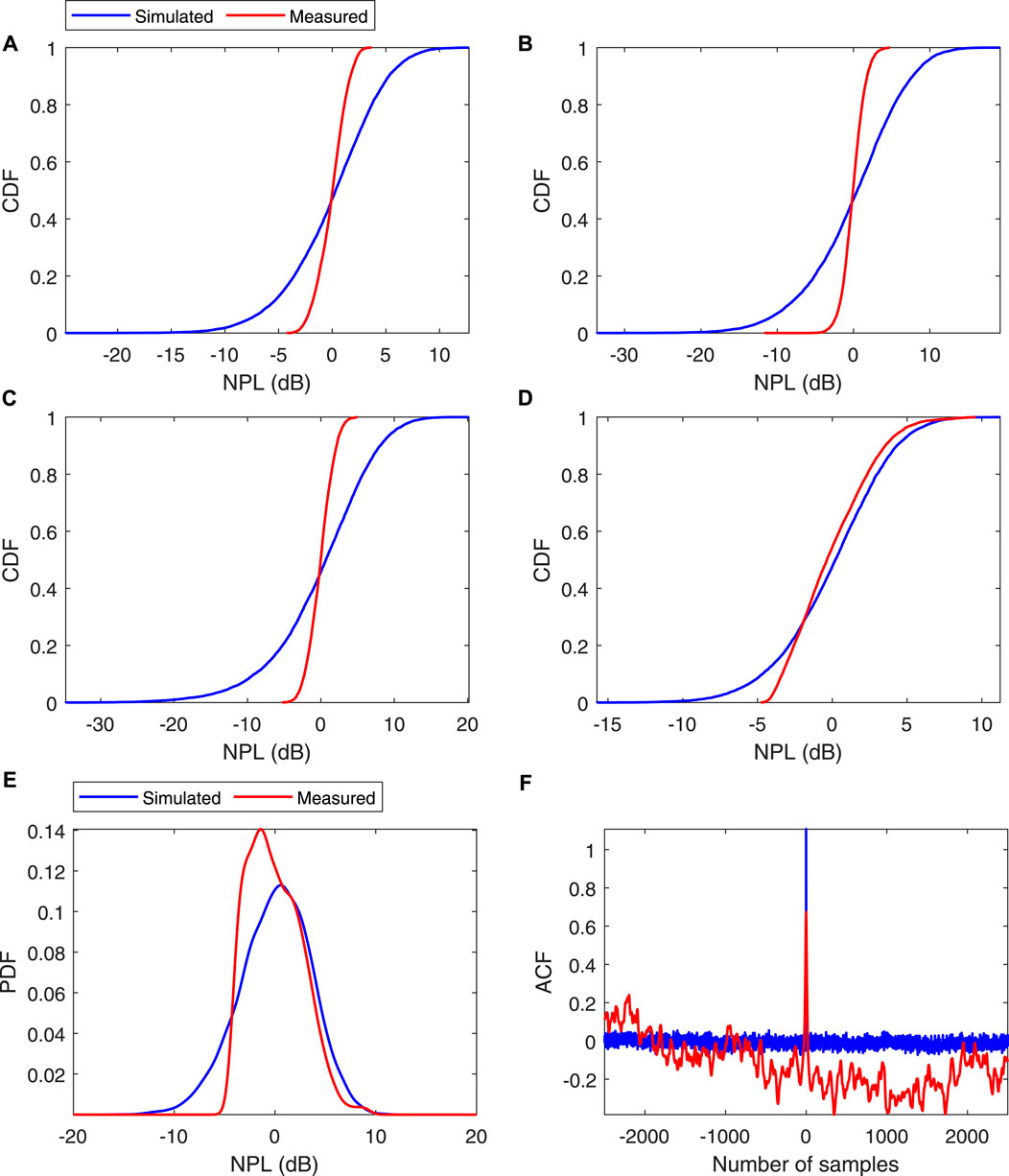
Figure 24. Results obtained for RX position RX2
Similar dependence of the overall model accuracy with the wind incidences was found for the remaining RX positions and pointing directions. Results obtained in RX position RX4 pointed at Tree#5, i.e.,
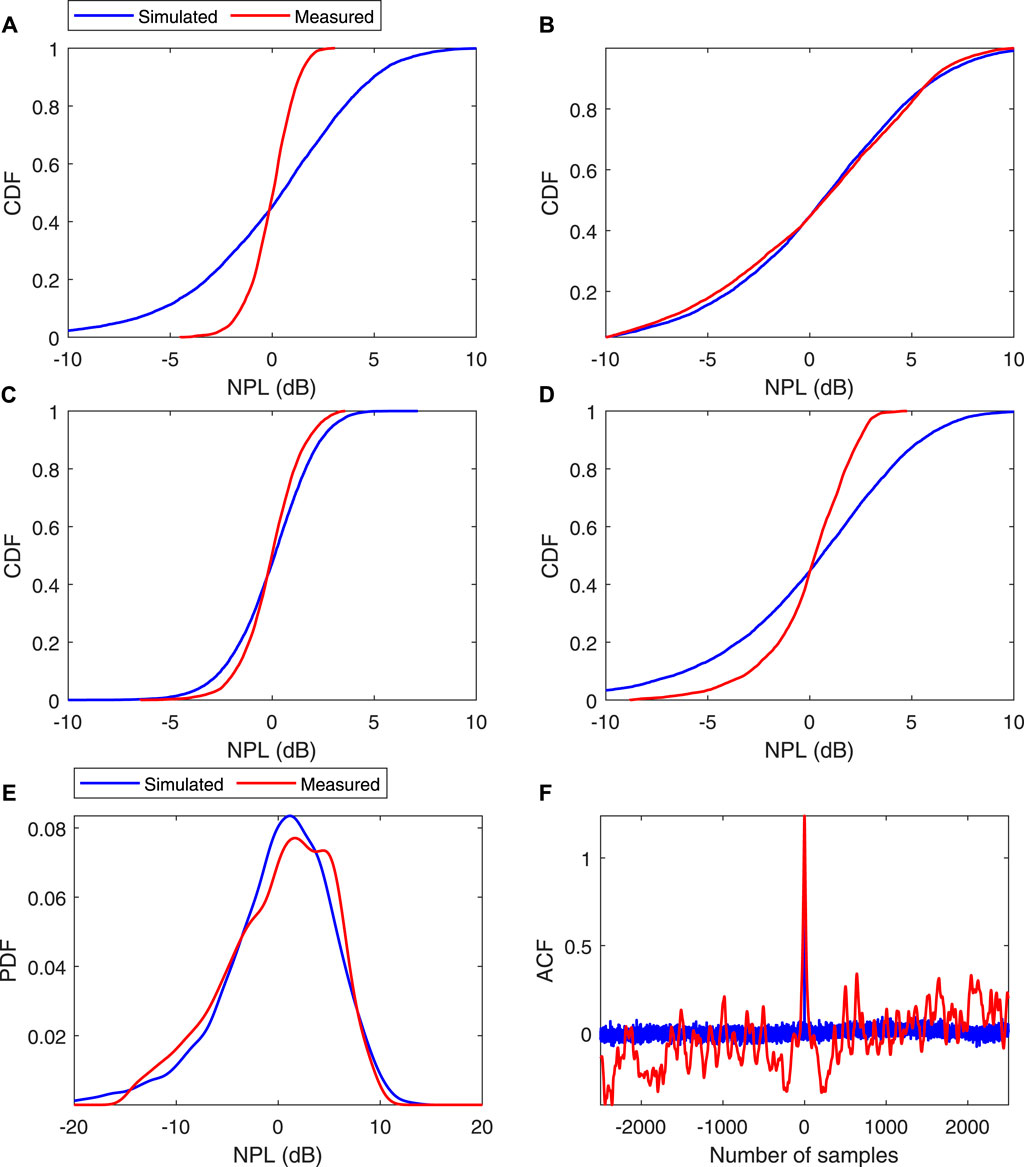
Figure 25. Results obtained for RX position RX4
6 Conclusion
In this paper, a 3D ray-tracing based doubly-selective model for propagation in vegetation, which accounts for the time-varying effects caused by the wind induced channel dynamics, is proposed. This dynamic model, at the outset, consisted of extracting the empirical input propagation parameters directly and synchronously from measured time-series of the dynamic re-radiation measurements, and yielded to a very reliable description of the signal level variations. However, its application in tree formation scenarios is limited due to the synchronous nature of the input propagation parameters extraction method, since the time instant that the input parameters are extracted, is different than that when the measurements in a tree formation scenario are conducted. Hence, this dynamic model was adapted to be based on the statistics of the signal level variation, in which the time-variability of the input propagation parameters was characterised using known statistical distributions, such as Lognormal and Weibull. Given the very-fast variation nature of a random variable, this second approach provided an accuracy reduction on the estimation of the signal time-series, particularly on the LCR and AFD analysis. However, such method enabled the model application in complex vegetation structures, such as tree formations, in which the distribution parameters,
The performance of the doubly-selective vegetation model while predicting the received signal level fluctuations, caused by the wind-induced swaying motion of the tree branches and leaves, in a tree formation scenario, was subsequently assessed against dynamic directional spectra measurements conducted in a controlled environment, inside an anechoic chamber. These measurements were performed at 20 and 62.4 GHz for four RX positions, four different artificially generated wind incidences and two wind speeds, i.e., low and high. The dynamic propagation model proved to be suitable to characterise the time-varying directional spectra providing a good representation of the measured time-series and its first and second order statistics.
The dynamic model developed to account for the wind induced channel time-variability, lacks of accuracy as far as the LCR and AFD analysis are concerned, due to the very-fast variation nature of the random variables used to characterise the time-varying input propagation parameters. Additionally, and as has been proven in Section 4, in tree formation scenarios, the wind will not affect all the trees equally. Thus, to effectively characterise the wind induced time-varying effect in such complex vegetation structures, wind propagation models and its effect on the variability of the input propagation parameters, should also be taken into account.
Data availability statement
The raw data supporting the conclusions of this article will be made available by the authors, without undue reservation.
Author contributions
NL: Writing–original draft, Writing–review and editing. TF: Writing–review and editing. MS: Writing–review and editing. RC: Writing–review and editing.
Funding
The author(s) declare that financial support was received for the research, authorship, and/or publication of this article. This work is part of the project UID/EEA/50008/2023, funded by the Portuguese Foundation for Science and Technology (FCT).
Conflict of interest
The authors declare that the research was conducted in the absence of any commercial or financial relationships that could be construed as a potential conflict of interest.
The author(s) declared that they were an editorial board member of Frontiers, at the time of submission. This had no impact on the peer review process and the final decision.
Publisher’s note
All claims expressed in this article are solely those of the authors and do not necessarily represent those of their affiliated organizations, or those of the publisher, the editors and the reviewers. Any product that may be evaluated in this article, or claim that may be made by its manufacturer, is not guaranteed or endorsed by the publisher.
Supplementary material
The Supplementary Material for this article can be found online at: https://www.frontiersin.org/articles/10.3389/fanpr.2025.1417976/full#supplementary-material
References
Assembly (2013). Radiocommunication. Recommendation itu-r p. 833-8, attenuation in vegetation. ITU-R.
Caldeirinha, R. (2001). “Radio Charaterisation of single trees at micro- and millimetre wave frequencies,”. Ph.D. thesis (UK: University of Glamorgan).
Caldeirinha, R., Fernandes, T., Leonor, N., and Ferreira, D. (2010). “Extension of the dret model to include scattering from tree trunks in microcell urban mobile scenarios,” in Proc. IEEE vehicular Technology conference (VTC-Spring) (Taipei, Taiwan).
International Radio Consultative Committee (1986). Influences of terrain irregularities and vegetation on tropospheric propagation. Reports and recommendations of the CCIR, 236–6.
Chee, K., Torrico, S., and Kurner, T. (2013). Radiowave propagation prediction in vegetated residential environments. IEEE Trans. Veh. Technol. 62, 486–499. doi:10.1109/tvt.2012.2226764
COST235 (1995). Radio propagation effects on next-generation fixed-service terrestrial Telecommunications systems. Luxembourg: Final Report.
Didascalou, D., Younis, M., and Wiesbeck, W. (2000). Millimeter-wave scattering and penetration in isolated vegetation structures. IEEE Trans. Geoscience Remote Sens. 38, 2106–2113. doi:10.1109/36.868869
Fernandes, T. (2007). “A discrete RET model for micro- and millimetre wave propagation through vegetation,”. Ph.D. thesis (U.K: University of Glamorgan).
Fernandes, T., Caldeirinha, R., Al-Nuaimi, M., and Richter, J. (2005). A discrete ret model for millimeter-wave propagation in isolated tree formations. IEICE Trans. Commun. E88-B, 2411–2418. doi:10.1093/ietcom/e88-b.6.2411
Johnson, R., and Schwering, F. (1985). A transport theory of millimeter wave propagation in woods and forest. Technical Report CECOM-TR-85-1.
Leonor, N. (2018). “A generic doubly-selective 3D vegetation model using point scatterers,”. Ph.D. thesis (Spain: Univ. of Vigo).
Leonor, N., Caldeirinha, R., Fernandes, T., Ferreira, D., and Sánchez, M. (2014). A 2d ray-tracing based model for micro- and millimeter-wave propagation through vegetation. IEEE Trans. Antennas Propag. 62, 6443–6453. doi:10.1109/tap.2014.2362124
Leonor, N., Caldeirinha, R., Sanchez, M., and Fernandes, T. (2017). A two-dimensional ray-tracing-based model for propagation through vegetation: a practical assessment using ornamental plants at 60 GHz. [Wireless corner]. IEEE Antennas Propag. Mag. 59, 145–150. doi:10.1109/map.2016.2629839
Leonor, N., Fernandes, T., Garcia Sanchez, M., and Caldeirinha, R. F. S. (2019). A 3d model for millimeter-wave propagation through vegetation media using ray-tracing. IEEE Trans. Antennas Propag. 67, 4313–4318. doi:10.1109/tap.2019.2905957
Leonor, N., Sanchez, M., Fernandes, T., and Caldeirinha, R. F. S. (2018). A 2d ray-tracing based model for wave propagation through forests at micro and millimeter wave frequencies. IEEE Access 6, 32097–32108. doi:10.1109/access.2018.2836223
Meng, Y., Lee, Y., and Ng, B. (2009). Empirical near ground path loss modeling in a forest at vhf and uhf bands. IEEE Trans. Antennas Propag. 57, 1461–1468. doi:10.1109/tap.2009.2016703
Morgadinho, S., Caldeirinha, R., Al-Nuaimi, M., Cuinas, I., Sanchez, M. G., Fernandes, T. R., et al. (2012). Time-variant radio channel characterization and modelling of vegetation media at millimeter-wave frequency. IEEE Trans. Antennas Propag. 60, 1557–1568. doi:10.1109/tap.2011.2180301
Rogers, N. C., Seville, A., Richter, J., Ndzi, D., Savage, N., Caldeirinha, R. F. S., et al. (2002). A generic model of 1-60 GHz radio propagation through vegetation - final report. UK: QINETIQ/KI/COM/CR020196/1.0.
Seville, A., and Craig, K. (1995). Semi-empirical model for millimetre-wave vegetationattenuation rates. Electron. Lett. 31, 1507–1508. doi:10.1049/el:19951000
Torrico, S., Bertoni, H., and Lang, R. (1998). Modeling tree effects on path loss in a residential environment. IEEE Trans. Antennas Propag. 46, 872–880. doi:10.1109/8.686776
Torrico, S., and Lang, H. (2012). “Wave attenuation prediction through a volume of random located lossy-dielectric branches – 3-d vector transport theory,” in 6th European conference on antennas and propagation (EUCAP) (prague), 3342–3345.
Ulaby, F., Haddock, T., and Kuga, Y. (1990). Measurement and modeling of millimeter wave scattering from tree foliage. Radio Sci. 25, 193–203. doi:10.1029/rs025i003p00193
Wang, F. (2006). “Physics-based modeling of wave Propagation for terrestrial and space communications,”. Ph.D. thesis (USA: University of Michigan).
Wang, F., and Sarabandi, K. (2005). An enhanced millimeter-wave foliage propagation model. IEEE Trans. Antennas Propag. 53, 2138–2145. doi:10.1109/tap.2005.850704
Wang, F., and Sarabandi, K. (2007). A physics-based statistical model for wave propagation through foliage. IEEE Trans. Antennas Propag. 55, 958–968. doi:10.1109/tap.2007.891841
Keywords: millimetre wave radio propagation, modelling, propagation measurements, scattering, vegetation
Citation: Leonor N, Fernandes TR, Sánchez MG and Caldeirinha RFS (2025) A 3D ray-tracing based model for radiowave simulations in vegetated environments with wind-induced dynamics. Front. Antennas Propag. 3:1417976. doi: 10.3389/fanpr.2025.1417976
Received: 15 April 2024; Accepted: 08 January 2025;
Published: 04 March 2025.
Edited by:
Ferdous Hossain, Multimedia University, MalaysiaReviewed by:
Jan M. Kelner, Military University of Technology (WAT), PolandOrlandino Testa, Sapienza University of Rome, Italy
Copyright © 2025 Leonor, Fernandes, Sánchez and Caldeirinha. This is an open-access article distributed under the terms of the Creative Commons Attribution License (CC BY). The use, distribution or reproduction in other forums is permitted, provided the original author(s) and the copyright owner(s) are credited and that the original publication in this journal is cited, in accordance with accepted academic practice. No use, distribution or reproduction is permitted which does not comply with these terms.
*Correspondence: Nuno Leonor, bnVuby5sZW9ub3JAaXBsZWlyaWEucHQ=