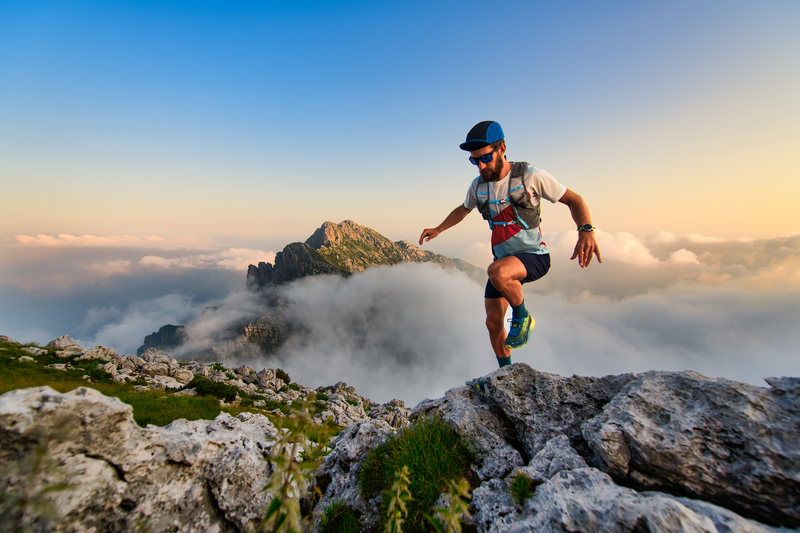
94% of researchers rate our articles as excellent or good
Learn more about the work of our research integrity team to safeguard the quality of each article we publish.
Find out more
OPINION article
Front. Anim. Sci.
Sec. Precision Livestock Farming
Volume 6 - 2025 | doi: 10.3389/fanim.2025.1556812
This article is part of the Research Topic Exploring Novel Data Sources to Improve Health and Welfare in Dairy Cattle View all 6 articles
The final, formatted version of the article will be published soon.
You have multiple emails registered with Frontiers:
Please enter your email address:
If you already have an account, please login
You don't have a Frontiers account ? You can register here
One of the most important environmental influences on a cow is the social environment induced by surrounding cows. Living in groups leads to affiliative and agonistic interactions between the individuals of a herd. Affiliative and aggressive interactions create balance and structure the herd (Tucker, 2017). Affiliative interactions, such as allogrooming and proximity, contribute to forming and maintaining positive social relationships within the herd (Val-Laillet et al., 2009). In contrast, negative interactions such as aggressive contacts, displacements or replacements, motivated by access to resources and housing conditions, among other factors, contribute to establishing dominance relationships between the individuals (Foris et al., 2021).These relationships can be affected by daily husbandry procedures at on dairy farms, such as re-grouping of animals according to age or production stage or insufficient space allowance (Bouissou et al., 2001;Rocha et al., 2020). Frequent regrouping challenges dominance relationships and social preferences, which can increase levels of aggression within the herd (Raussi et al., 2005;Schirmann et al., 2011). lead to high levels of aggression. Thus, daily husbandry practices on dairy farms can unintentionally disrupt these social interactions, impacting animal welfare and production (Bouissou et al., 2001;Thompson et al., 2022;von Keyserlingk et al., 2009) (Bouissou et al., 2001;Thompson et al., 2022). Moreover, social interactions play a role in the transmission of contagious diseases (Burke et al., 2022;de Freslon et al., 2019)(de Freslon et al., 2019). These studies described the relationship between social network structure and the incidence of respiratory and reproductive diseases in cattle.Due to the importance of social behavior in cattle's daily activities, scientific research has focused on understanding their social interactions. Visual observations have been the main tool to study social interactions in cattle (Sahu et al., 2020). Visual observations are a robust and valid method based on human assessments and decisions. However, they are sensitive to inter-observer variability, labor-intensive and generally typically limited by the duration of the observation and the number of animals that can be monitored simultaneously. Precision livestock farming (PLF) has opened new possibilities for monitoring and studying behavior in cattle. Real-time location systems (RTLS), for example, allow the continuous monitoring of area utilization (Ternman et al., 2019;Tullo et al., 2016) and proximity between individuals (Hansson et al., 2023)(Ben-Meir et al., 2023;Boyland et al., 2016;Chopra et al., 2020;Rocha et al., 2020).One of the main goals of the research program on cow social interaction and disease transmission (CSI:DT) was to develop data analysis tools for understanding social contact information which could concurrently consecutively to be used to minimize disease transmission within dairy farms. We summarize our publications (Table 1) and experiences from this research program into two opinion papers and give advice for future research within the field. Here, we focus on approaches to efficiently monitor and analyze social network information in dairy cattle and its future implications.Social interactionsAn RTLS based on ultra-wideband (UWB) technology works with tags attached to the cows which communicate with anchors placed in the barn's ceiling. The positioning of a cow is determined by the tag's connection to multiple anchors. Conducting in-depth analyses of animal movement and social contacts requires understanding the data quality by assessing the noise and accuracy of the position, where data is lost, and how accurately missing positions can be interpolated.In our studies, we have collected positioning data using the CowView system developed by GEA, which guarantees an error distance of approximately 0.50 m, with a reported accuracy of 16cm (Meunier et al., 2018). A validation study conducted in both a Swedish farm and a Dutch farm revealed a mean error distance of the positioning data of 0.78 m and 0.54 m for 13 and 21 fixed tags installed in each farm, respectively (Hansson et al., 2023). The quality of the positioning data was best in the center of the farm where the tags on the cows can easily connect to many anchors in the ceiling (Ren et al., 2021b). The system achieves a 95% accuracy in detecting zone-related behavioral activities based on the floorplans of the barn (Tullo et al., 2016). Ren et al. (2021b) found that missing data averaged 31.29% (~7.5 h/d) and 19.97% (~4.8 h/d) of the day for the Swedish and Dutch farm, with the most common scenario being a single second missing. Interpolating missing position data with the Modified Akima algorithm achieved an average interpolation accuracy of 0.17 m (Ren et al., 2022).Data collected using UWB systems seem satisfactory for detecting proximity between cows. Nevertheless, we removed proximity interactions that lasted less than 10 minutes over an entire day in our analysis to avoid considering stochastic short contacts due to the limited space of the barn. We attempted to classify affiliative and agonistic interactions by detecting displacements at the feeding tables using the UWB data and video recording but were unsuccessful. The main challenge was that the accuracy of the UWB system was insufficient to detect cow displacements in the open structure feeding bunks. Additionally, the CowView system's internal Kalman filter could delay the detection of animal movements, distorting the actual trajectory.The benefits of using RTLS for studying social contacts in livestock are evident, as it allows the continuous monitoring of proximity between individuals in the herd over long periods. However, proximity contacts do not imply social or physical interaction between individuals. Therefore, their applicability has been criticized in comparison to visual observational studies, which allow distinguishing between different types of social contacts. Nevertheless, preferential relationships in dairy cattle are expressed through social grooming or by spending more time in proximity (Val-Laillet et al., 2009). Previous studies have described a positive correlation between affiliative interactions and long-lasting proximity contacts between dairy cows and calves, whereas short encounters were associated with agonistic interactions in calves (Ben-Meir et al., 2023;Boyland et al., 2016).Scientific studies based on RTLS have described findings consistent with previous literature based on visual observations (e.g. Rocha et al., 2020;Hansson et al., 2023;Boyland et al., 2016), supporting the adoption of this technology to comprehend social behavior in dairy cattle. However, this approach requires some assumptions, such as time, distance and frequency thresholds. Social contacts have been investigated by using distance and cumulative interaction time information (Hansson et al., 2023;Marina et al., 2024c;Rocha et al., 2020) or distance and number of contacts (Boyland et al., 2016;Chopra et al., 2020;Raussi et al., 2010;Vázquez-Diosdado et al., 2023). These studies individually validated the proximity thresholds adopted by varying the temporal and spatial thresholds, supporting the robustness of using proximity to quantify social contacts.Pairwise interaction data can be used to construct social networks. This information is usually modeled by applying individual-or relational-level models (O' Malley and Marsden, 2008). Hansson et al. (2023) applied individual-level models to explore the relationship between different cow's characteristics and the contact rate on social networks. Applying these models allowed the authors to define the association between parity and days in milk with the number of contacts a cow establishes daily with its herd mates. Marina et al. (2024c) applied a relational-level model known as the separable temporal exponential random graph model described by Krivitsky and Goodreau (2016), to explore longitudinal networks and study the formation and persistence of social contacts in dairy cattle. Implementing this longitudinal relational-level model allowed the authors to describe the kindergarten effect: cows born within seven days of each other showed more persistent contact with each other than with the rest of the herd. Considering these factors together with the internal structure of social networks is crucial to understanding the occurrence and persistence of social contacts in dairy cattle (Marina et al., 2024a).RTLS data can also be used to discover cubicle occupancy patterns of dairy cows in the barn. Churakov et al. (2021) used the aggregated positioning data within the CowView system with predicted activity (e.g., walking, eating, in-cubicle). They found that cubicle occupancy patterns are related to parity and lactation stage and that cows choose cubicles close to individuals with similar attributes. High parity cows used the cubicles close to the milking area more frequently, while first lactation cows occupied less busy barn areas. In addition, Marina et al. (2024b) demonstrated the importance of considering the spatial preferences of individuals when studying social interactions in dairy cattle.Only a few studies have investigated the genetic component of sociability in dairy cattle and its association with other traits of interest to the dairy industry (e.g. (Gutiérrez-Gil et al., 2008;Marina et al., 2024c;Rönnegård et al., 2022;Wang et al., 2023). Hansson et al. (2023) investigated the repeatability of social behavior in dairy cows over consecutive days by estimating the proportion of variation in contact rate between individuals relative to the total variance, and determined that it was approximately 30%. Rönnegård et al. (2022) used these data to investigate how much of the variation between animals was due to genetics and estimated heritabilities (proportion of genetic variance relative to total variance) of 0 to 0.20, albeit with large standard errors. Similar heritability estimates were reported in Bos indicus for other social measures (dominance value and social hierarchy), with values of 0.23 and 0.25, respectively (de Paula Soares Valente et al., 2023). These estimates correspond well to heritability estimates of similar traits in pigs (Agha et al., 2022a(Agha et al., , 2022b)).Not only is the number of contacts a cow has heritable, but the effect a cow has on other cows' milk production might be heritable too. This is known as indirect genetic effects (IGEs) (Moore et al., 1997). IGEs can make a significant contribution to heritable variation in livestock species, helping to reduce disease transmission, stress levels and overall herd health issues (Peeters et al., 2012), for instance. There are several examples of the benefits of understanding IGEs in different livestock species, such as the cannibalistic behavior in laying hens (Alemu et al., 2016), tail biting in pigs (Camerlink et al., 2015), and the outcome of dyadic contacts in deer (Wilson et al., 2011). Using methods that target both the direct and indirect genetic effects for genetic selection, is a promising tool to simultaneously improve production and welfare through the selection programs in dairy cattle (Ellen et al., 2014).The continuous collection of proximity and area utilization data for all individuals over time has allowed us to gain new insights into the behaviour of dairy cattleWe have gained new insights into social contacts between dairy cattle in free-stall barns using UWB data. Insights, thatThe UWB data have facilitated the have description of allowed us to analyze how cubicle occupancy varies within and between lactations, and that have revealedshown the heterogeneity of social networks in dairy cattle and how long-lasting preferences for social contacts between cows that continue even between lactations. Similar technologies could have been used to obtain this information, such as Bluetooth wireless technology or proximity loggers. However, UWB systems provide fairly accurate positions compared to Bluetooth (see Huhtala et al., 2007;Bloch and Pastell, 2020). Spatial proximity loggers could also provide temporal proximity information, although; however, the area of the barn where these interactions occurred will be challenging to determine.The findings from this project could have benefited from combining UWB data with video information and data from proximity loggers to overcome the limitations of the system and increase the accuracy of social networks. The integration of UWB data with video image analysis could provide specific information about certain behaviours and allow the detection of the affiliative and agonistic social interactions (McDonagh et al., 2021;Meunier et al., 2018;Ren et al., 2021a)As UWB data is not sufficient to obtain information on the direction of contacts between individual cows in a social network (e.g. licking a social partner), we do foresee the potential of combining UWB data with image analysis of videos. In addition, the fusion of indoor and outdoor sensors would allow continuous monitoring of all individuals throughout the year also in production systems with pasture access, enhancing the use of this information to improve farm management practices and gain valuable information on animal behavior. Altogether, RTLS data is a valuable tool that could contribute to understanding the social behavior of dairy cattle, helping to optimize farm management practices, and improve animal health, production and welfare and production, and control disease transmission within dairy farms of dairy cattle.. Table 1. Scientific publications published during the project. 355
Keywords: dairy cows, Social network, Ultra-Wide Band, real-time location system, indirect genetic effects
Received: 07 Jan 2025; Accepted: 19 Mar 2025.
Copyright: © 2025 Marina, Hansson, Ren, Fikse, Gussmann, Nielsen, Skarin, Woudstra and Rönnegård. This is an open-access article distributed under the terms of the Creative Commons Attribution License (CC BY). The use, distribution or reproduction in other forums is permitted, provided the original author(s) or licensor are credited and that the original publication in this journal is cited, in accordance with accepted academic practice. No use, distribution or reproduction is permitted which does not comply with these terms.
* Correspondence:
Lars Rönnegård, Dalarna University, Falun, Sweden
Disclaimer: All claims expressed in this article are solely those of the authors and do not necessarily represent those of their affiliated organizations, or those of the publisher, the editors and the reviewers. Any product that may be evaluated in this article or claim that may be made by its manufacturer is not guaranteed or endorsed by the publisher.
Research integrity at Frontiers
Learn more about the work of our research integrity team to safeguard the quality of each article we publish.