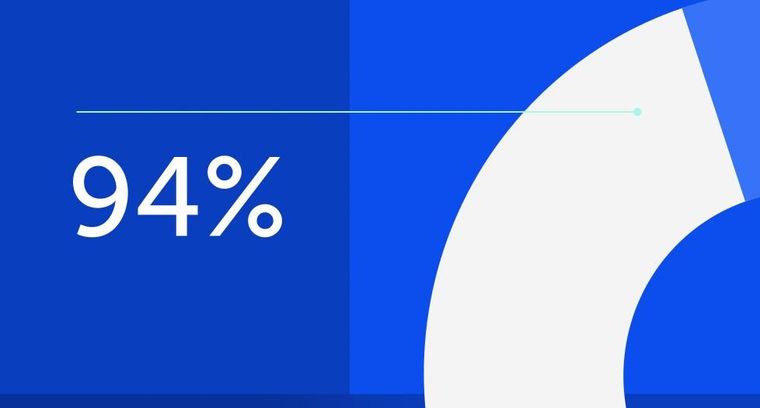
94% of researchers rate our articles as excellent or good
Learn more about the work of our research integrity team to safeguard the quality of each article we publish.
Find out more
ORIGINAL RESEARCH article
Front. Anim. Sci., 31 March 2025
Sec. Precision Livestock Farming
Volume 6 - 2025 | https://doi.org/10.3389/fanim.2025.1547395
This article is part of the Research TopicTechnological and Economic Advancement for Efficient and Sustainable Dairy Farming in Large RuminantsView all 4 articles
Introduction: The aim of this study was to evaluate the transformative potential of precision livestock farming (PLF) in dairy production, nutrition, and reproductive efficiency by implementing proactive management strategies that utilize real-time data.
Methods: Data were gathered from a commercial dairy farm, employing a 3D accelerometer system attached to ear-tags to monitor activity and rumination behavior. Additionally, information on animal-related events such as oestrus, artificial insemination, clinical diseases, and treatments was recorded.
Results: It was observed that there were differences in rumination between positive and negative cows in the days following artificial insemination (AI). Specifically, from day 0 to day 16, negative cows exhibited a higher rumination time compared to positive cows, with this contrast being particularly significant on days 9 and 10. Over the duration of the study, cows that tested negative produced, on average, 0.78 kg more milk per day.
Discussion: This suggests that animals with higher milk production were less likely to be pregnant, potentially due to increased nutrient allocation to the mammary gland, resulting in a more pronounced negative energy balance and consequently lower conception rates. Furthermore, the accelerometer utilized in this study was capable of distinguishing differences in daily lying time between pregnant and non-pregnant animals on the days of oestrus return. This finding highlights the potential for developing a system alert to identify “probable pregnant” and “probable non-pregnant” animals.
In contemporary dairy farming, optimizing reproductive efficiency is essential for maintaining profitability and safeguarding herd well-being (Cardoso Consentini et al., 2021). The intricate interplay among disease, pregnancy losses, and reproductive performance significantly impacts milk yields and overall operational success in dairy farms (McDougall et al., 2014). Increased rates of disease and pregnancy losses directly undermine reproductive outcomes, resulting in lower calving rates and increased costs associated with involuntary culling and replacement. Therefore, the economic sustainability of dairy farms relies heavily on their ability to uphold optimal reproductive health within their cattle herds (Buonaiuto et al., 2023; 2024).
To address these challenges and enhance both productivity and profitability, PLF has emerged as a promising paradigm (Bianchi et al., 2022; Lamanna et al., 2024a). PLF utilizes cutting-edge technologies, including ear tag devices, to collect real-time data from animals across production stages (Das et al., 2023). This data, analyzed using specialized software, provides invaluable insights into the nutritional, health, and reproductive status of individual animals as well as the entire herd (Lanzoni et al., 2021; Lamanna et al., 2024b). Among the various behavioral parameters, rumination and lying behaviors have gained increasing attention for their potential to serve as reliable indicators of early pregnancy detection (Saint-Dizier and Chastant-Maillard, 2015). Both rumination and lying time are intricately linked to physiological changes associated with conception and pregnancy maintenance (Cangar et al., 2008; Schirmann et al., 2013). Specifically, alterations in these behaviors can reflect shifts in hormonal profiles, metabolic demands, and overall health status during early gestation (Reith and Hoy, 2012; Paudyal, 2021). Early pregnancy detection using behavioral data such as rumination and lying times offers a non-invasive and real-time approach to assess reproductive status (Cangar et al., 2008; Marques et al., 2024). Variations in rumination time during early gestation may indicate physiological adaptations to pregnancy, while lying behavior changes may highlight differences in comfort and metabolic status, both of which are pivotal for successful conception and embryo development (Santos et al., 2022).
In the context of PLF and different forage type rations, careful monitoring of rumination time emerges as a crucial indicator of dairy cow health and nutritional balance (Heinrichs et al., 2021). While total mixed rations (TMR) provide essential nutrients, they also pose challenges related to digestibility and overall rumen health (Song et al., 2023). Leveraging PLF technologies like individual rumination monitoring systems enables dairy farmers to obtain real-time insights into cow rumination patterns. Changes in rumination time can serve as early indicators of potential health issues, suboptimal ration digestibility, or stressors within the herd (Cavallini et al., 2021; 2022; Soriani et al., 2012). Within different forage-based rations, fluctuations in rumination time may indicate differences in forage quality, consistency, or intake, thereby facilitating proactive adjustments in feeding regimes to optimize rumen function and overall cow health (Schirmann et al., 2012). The integration of rumination and lying behavior monitoring into PLF systems provides dairy farmers with actionable insights into reproductive outcomes. By leveraging these behaviors as early signals, producers can optimize reproductive management protocols, reduce pregnancy losses, and enhance herd profitability, particularly in systems relying on silage/hay-mix TMR rations (Szenci, 2022; Lin et al., 2023).
The aim of this study was to evaluate the potential of PLF in improving reproductive efficiency in free-range dairy cattle through real-time monitoring of behavioral parameters. Specifically, we investigated the use of ear-tag accelerometer data to detect variations in rumination and lying time associated with pregnancy status, with the objective of developing predictive indicators for early pregnancy detection.
The experimental protocol was approved by the Ethic Committee of the Veterinary Medicine Department of the University of Teramo with the number 18528/2022. The present study was conducted from January 2021 to December 2022 on a free-stall farm located 30 km from Teramo at an altitude of 1073 m above sea level. The farm raised a total of 313 Italian Holstein dairy cows, divided into pens based on age and reproductive status: lactating cows (divided into three different groups based on the number of lactation and days in milk; DIM), post-partum transition cows, dry cows, and heifers.
A total of 291 Italian Holstein lactating dairy cows were included in the study. The cows had an average (mean ± standard deviation) of 1.77 ± 1.50 lactations, with an average lactation period of 153.90 ± 68.08 DIM. At the beginning of the trial, their average body weight (BW) was approximately 700 ± 25 kg. The average milk yield was recorded as 46.07 ± 5.35 kg. All the cows were monitored for health status along the trial by an expert veterinarian to avoid occurrence of pathologies, such as ketosis, acidosis, lameness and hypocalcemia (Magro et al., 2024). Each cow had access to a cubicle structured with a concrete base and two side briskets. The cubicles were covered with a bottom layer of compacted sand (approximately 20 cm deep), with the addition of daily renewed straw spread across the entire cubicle. In addition to benefiting from good natural ventilation due to the structure of the barn, the farm also has an automatic ventilation system connected to control units and sensors installed in various areas of the facility: when the Temperature-Humidity Index (THI) inside the barn exceeds 65, the ventilation system activates automatically. Furthermore, in the waiting area of the milking parlor, there is a wetting and drying system that activates automatically when the THI exceeds 70.
The diets were prepared every day using a mixer wagon Matrix Rover Jumbo (Italmix SRL, Italy) and fed ad libitum (daily orts 3–5%) once a day at 0800 h. Tables 1 and 2 present the diet composition and chemical analysis, respectively, of the ration provided to enrolled cows. TMR samples were collected weekly from the feed bunk, at the start, middle, and end. After the collection these samples were then combined, dried in an oven at 65°C for 24 hours, and initially milled using a Retsch MS 100 mill with a 4 mm sieve. Subsequently, they were further milled with a Cyclotec 1093 mill to achieve a particle size of 1 mm. Approximately 2 grams of dried samples underwent analysis using near-infrared spectroscopy (NIRS) with Bruker-Tango instruments (Buonaiuto et al., 2021a). Feed samples were analyzed with a wavelength range between 400 and 2,498 nm, for the following parameters: crude protein, ash, starch, amylase-treated neutral detergent fiber (NDF), acid detergent fiber, acid detergent lignin, undigested NDF at 24, 30, 120 and 240 h in vitro fermentation were also analyzed in the TMR. More details in the employed methods are reported in a previous paper (Vettori et al., 2023; Felini et al., 2024; Koakoski et al., 2024). Near-infrared spectra (log 1/reflectance) were recorded for each 2 nm range. Applied NIRS calibrations have already been published and validated in a precedent full paper works (Buonaiuto et al., 2021a).
Table 1. Composition of the experimental rations fed during the research (kg DM and % of dietary DM).
Rumination and activity were monitored using the Smartbow system (Smartbow GmbH; Roland et al., 2018). Each cow was equipped with an ear tag that included an accelerometer, positioned on the upper part of the left ear (Wolfger et al., 2017). The tag measured 5.2 × 3.6 × 1.7 cm and weighed 34.1 grams, capable of transmitting data over an unobstructed range of 300 meters. Data transmission to wall-mounted receivers occurred at a frequency of 1 Hz. These receivers were installed 2.7 meters above the ground and spaced approximately 15 meters apart to ensure comprehensive coverage throughout the barn. Data collected by the receivers (Wallpoints), were sent to a central server (Station), located in the office area. This server calculated each cow’s precise location by measuring the distance from the nearest four sensors. Data transmission was contingent upon direct detection of the tag by at least four sensors, aiming to maintain consistent updates every second. The server then relayed the positional data to a computer system where it was collected and stored for further analysis.
The system facilitated real-time access to the data via both fixed and mobile devices, as discussed by Reiter et al. (2018). It enables detailed evaluations of various aspects of animal behavior, including real-time tracking of animal locations, the duration of time spent in different areas, rumination, and postural behaviors (standing and lying down). Additionally, it assesses the level of activity or inactivity exhibited by the animals. For each cow, data on rumination time and postural behaviors were recorded minutes per day. Activity levels are categorized into two types: active and inactive. Inactive time accounts for periods during which the cow is not engaged in any motor or rumination behaviors and may include both standing and lying phases. In contrast, active time encompasses natural behaviors such as walking, rumination, feeding, and social interactions with other cows.
Cows were milked three times a day, at 0400, 1200, and 1900 hours, in a 16-unit herringbone milking parlor. The milking parlor was equipped with Metatron electronic milk meters, P21 bail controllers, and electronic identification tags (Metatron DairyPlan, GEA GmbH). For each cow, milk production data, concerning the days following the fertilization event, was extracted directly from the company’s software, implemented at the milking facility. The animals were identified and recognized through a pedometer and a collar placed on them. The computer communicated with the milking parlor, and upon the entry of a cow into the parlor, the system automatically detected the pedometer and transferred the information to the milk meter installed at the milking point, which in turn communicated and provided the data to the software. This way, the following were recorded: the animal’s company number, the duration of milking, and the milk production in kilograms. The milk production control provided by the software, using milk meters, allowed constant and complete control of the production process directly from the milking parlor. Once the production data had been extracted on the farm, we then proceeded to process it on an Excel (2019) spreadsheet. Here, the daily productions for each animal were associated with ear tag data, taking into account the date of the latter. We then proceeded to calculate the actual lactation days, using the date of the last calving. Monthly, the milk of all the cows was sampled and analyzed according to previously published paper (Buonaiuto et al., 2021b).
At the farm, the reproduction protocol operated as follows: following parturition, the voluntary waiting period for artificial insemination was set at 70 days. Heat detection was performed either by farm operators or by the ear tag device alarm. Cows that did not exhibit natural heat after the voluntary waiting period were induced using a synchronization protocol (PFG2α). Artificial insemination (AI) could occur after either natural or induced heat. Thirty-two days after AI, pregnancy status was assessed via ultrasound echography (Draminsky iScan 2). Positive pregnancies progressed normally, while negative cases (non-pregnant) were pharmacologically induced until pregnancy confirmation.
Reproductive data and health status were retrieved from the national monitoring system (Si@llEvA, Italian Farmer Association, Rome, Italy). Evaluated parameters included: dates of drug administration for synchronization protocols, calving dates and corresponding insemination dates, dates of postpartum heat surveys, dates of postpartum inseminations, dates of pregnancy diagnosis confirmation (positive or negative), and any subsequent post-diagnosis detections of confirmed positive pregnancies (Table 3).
Table 3. Summary of the included animals and events in the study, with their characteristics (PGF yes or not, positive or negative pregnancy) and distribution along the year.
All data were analyzed using the statistical software JMP Pro v 16 (SAS, NY). A preliminary model assessed whether there was a greater or lesser effectiveness of the type of oestrus (natural or induced). A Chi-square model was used for this purpose. The results indicated no differences between the groups based on the type of oestrus (p=0.68). Therefore, all fertilization events were included in the study. The normal distribution of the data was verified using the Shapiro-Wilk test. Followed statistical analysis was based on the use of mixed models with repeated measures. The fixed effects considered were the outcome (successful vs unsuccessful insemination), post-fertilization days (32 days), their interaction, and seasonality. The cow, along with the type of oestrus (induced or natural), was considered a random effect. Each cow, associated with the lactation day and the single insemination event (715), was considered as the experimental unit and included as a random effect. In the graphs, the results of the tested parameters are expressed as the least squares mean and standard error of the mean. Results were considered trends for p ≤ 0.10, significant for p ≤ 0.05, and highly significant for p ≤ 0.01. To analyze differences on individual days or the effect of seasonality, if the interaction between fixed effects was significant, the Tukey test was used. In the graphs, significant differences were denoted by the non-overlap of the error bars, in the text, with different superscript letters.
In the years 2021–2022, a total of 715 fertilization events involving 291 animals were selected. Among these, 206 were induced and 85 occurred during natural oestrus. Roughly half of these events resulted in successful fertilization. The success rate of these events was influenced by seasonality, with the highest success observed in winter and the lowest in summer and autumn.
The animals selected for the study were, on average, fertilized at 138 ± 61 DIM. This group comprised both primiparous and multiparous cows. Among these animals, 36% had undergone a single AI prior to a positive diagnosis, while 26% had undergone 2 AIs, 16% had undergone 3 AIs, 8% had undergone 4 AIs, and 13% had undergone more than 4 AIs.
The average total milk production of our animals was 45.27 ± 3.38 kg/day (Figure 1). This production varied significantly by group, time, and seasonality but not by their interactions. Over the study period, cows that tested negative produced, on average, 0.78 kg more milk per day (p<0.01). There was a higher peak in milk production on days 2 and 5 after AI, with average productions of 49 kg for negative cows and 44 kg for positive cows. Spring exhibited the highest production levels with 53 kg, while the other seasons ranged between 43 and 46 kg.
Figure 1. Daily milk yield recorded in positive (blue) and negative (red) cows after the AI. The absence of overlap in the error bar (SEM) indicates a static difference.
On average, negative cows spent 771 minutes per day lying down, while positive cows averaged 781 minutes per day, as depicted in Figure 2. Statistical differences in behavior across various days post-insemination were observed (p<0.01). A decrease in lying time among negative cows was noted from the 18th to the 25th day, with significant differences observed on the 21st, 22nd, and 23rd days, with nearly an hour’s disparity between the groups. Additionally, seasonality showed high significance (p<0.01). During autumn, cows exhibited a higher lying time (804 min/day), while in winter, the time was intermediate (790 min/day), followed by spring with a resting time similar to winter (783 min/day). In contrast, summer showed a lower and distinct lying time compared to the other seasons (725 min/day).
Figure 2. Daily lying time pattern recorded in positive (blue) and negative (red) cow after the AI. The absence of overlap in the error bar (SEM) indicates a static difference.
Rumination time fell within the normal range for healthy dairy cattle, averaging 553 and 546 minutes in 24 hours for negative and positive cows respectively (p<0.01). Examining the rumination pattern (see Figure 3), a statistically significant difference between successful and unsuccessful inseminations emerged in the days following AI (p<0.01). Notably, from day 0 to day 16, unsuccessful inseminations exhibited a higher rumination time compared to successful inseminations, with this difference being significant on days 9 and 10. Furthermore, this significance re-emerged concerning seasonality, with rumination time being higher during autumn (556 min/day), equal during spring (553 min/day), and lower in winter (544 min/day) and summer (544 min/day).
Figure 3. Daily rumination time pattern recorded in positive (blue) and negative (red) cows after the AI. The absence of overlap in the error bar (SEM) indicates a static difference.
The inactive time did not exhibit significant differences between the two groups of animals. Similarly, to the previously analyzed data, we observed significance and an influence of seasonality (p<0.01). It is notable that autumn and spring exhibited comparable and higher inactive times (401 and 399 min/day respectively), while in winter and summer, cows displayed lower levels of inactivity (389 and 381 min/day, respectively).
Concerning active time, a statistical significance was found between the two study groups, unsuccessful and successful inseminations, throughout the entire study period. Unsuccessful inseminations were more active (923 vs 914 min/day for unsuccessful and successful inseminations respectively, p<0.01). Moreover, the influence of seasonality on this parameter (p<0.01) is noteworthy. Seasonality equally influenced the activity of the cows, leading to increased activity during autumn and spring (941 and 926 min/day respectively), followed by winter and summer (906 and 903 min/day, respectively).
The present study aims to explore how precision livestock enhance reproductive efficiency through proactive management approaches rooted in real-time data analysis. By leveraging ear tag monitoring techniques and embracing cutting-edge technologies, dairy farmers employing silage/hay-mix TMR can effectively address issues related to reproductive success.
Lying time, referring to the duration dairy cows spend lying down, is crucial for rest, rumination, and overall cow comfort (Tucker et al., 2021). It has been more frequently utilized in assessing cow welfare and comfort compared to commonly used parameters for determining estrus, such as the number of steps and time spent standing (Thompson et al., 2017). However, it has now also been recognized as a factor capable of predicting the timing of heat. Lying time also undergoes changes according to the season (Brzozowska et al., 2014). Observing the various seasons in detail, we noticed that lying time was highest in autumn and winter, with intermediate values reported in spring, while summer exhibited lower and distinct lying times compared to other seasons. This observation is consistent with findings from other authors (De Palo et al., 2006; Brzozowska et al., 2014). The reduction in lying time during summer can be associated with climatic conditions, primarily caused by the increase in THI typical of the summer period (> 68). Increased ambient temperature during summer can lead to greater standing time as it allows for increased heat dissipation through increased body surface area (Cook et al., 2007).
Active and inactive time data provide dairy farmers with valuable insights into the health, reproductive status, nutrition, and welfare of their cows, enabling them to make informed management decisions and optimize herd performance. Activity time encompasses the period during which cows engage in various locomotive behaviors such as walking, standing, and social interactions, while inactive time refers to periods when cows are not engaged in active behaviors such as feeding, rumination, or locomotion.
In the present study, regarding seasonality, we observed that in spring and autumn, animals showed higher activity compared to winter, and even less in summer, as reported in the literature (e.g., Toledo et al., 2023). During summer, animals tend to spend more time standing but move less. This observation aligns with studies indicating increased lying behavior and steps taken by animals during summer, suggesting increased activity. Although many authors (Brzozowska et al., 2014; Toledo et al., 2023) demonstrate a decrease in activity with increasing temperatures to reduce heat produced by muscle metabolism, our study results show a similar trend. Rumination time serves as a valuable indicator of gastrointestinal health, nutritional adequacy, metabolic status, and reproductive performance in dairy cow management. By monitoring rumination patterns, farmers can proactively address potential health issues, optimize feeding practices, and enhance the overall well-being and productivity of their herds. Rumination time typically falls within a normal range for healthy animals (Soriani et al., 2012; Heinrichs et al., 2021).
However, there are significant variations in the average rumination threshold considered “normal” among different devices available on the market. These differences can primarily be attributed to the specific algorithms used by each device to analyze the collected data. One of the key factors contributing to variations in rumination thresholds is the diversity in data analysis algorithms implemented by each manufacturer. For example, devices like SCR, CowMed, and SmartBow may adopt different approaches in interpreting rumination data and determining what constitutes a favorable rumination threshold. In the case of SCR devices, the average rumination threshold considered desirable might be around 500 minutes per day, based on historical data analysis and the average rumination behavior of livestock. Conversely, CowMed devices might set an average optimal rumination threshold around 400 minutes per day, based on specific analysis criteria and algorithms developed by the company.
Regarding SmartBow devices, as implemented in the present study, the achieved normal rumination threshold for a lactating cow producing approximately 40 liters of milk per day and at around 100 days in lactation may vary but was found to be around 550 minutes per day. This data is comparable to findings from other studies (e.g., an average of 575 min/day according to Martin et al., 2021) that used the same technology to measure rumination. However, it could range from 400 to 650 minutes per day, taking into account the specific conditions and physiological behavior of the livestock in these circumstances. It is important to note that defining a “normal” rumination threshold depends on various factors, including the breed of livestock, individual health status, milk production level, and other environmental factors. Furthermore, differences between optimal thresholds established by different devices may reflect variations in data analysis approaches and methodologies for assessing livestock health (Wolfger et al., 2017; Roland et al., 2018).
The average rumination time in our study is lower for cows during the summer and winter compared to autumn and spring, where the time was higher, likely due to the mild climate favored by the altitude of the evaluated farm and appropriate stable management. This behavioral variation recorded in summer is consistent with findings in the literature (Moallem et al., 2010; Moretti et al., 2017; Müschner-Siemens et al., 2020; Tapkı and Şahin, 2006), which demonstrate how this behavior is influenced by the season and temperature. Specifically, the increase in ambient temperature seems to be associated with a reduction in rumination time. Finally, we can affirm that cows, in response to an increase in temperature, reduce feed intake, and consequently rumination time, to limit the production of endogenous heat.
Based on previously reported results, we propose a protocol for evaluating non-pregnant cows at 25 days after AI (see Figure 4). To conduct this evaluation, we need to combine the data obtained from the ear tag device regarding daily rumination time and lying time. In practical terms, to analyze this large dataset effectively, we need to develop machine learning techniques capable of retrospectively evaluating each cow starting from day 25 after AI. As shown in Figure 4, we need the combination of three events to trigger a negative pregnancy alarm for the cows enrolled in the trial. The first event is a peak in rumination time (+20 minutes per day compared to the day of AI) on days 9 and 10 after AI. The second event is a drop in daily lying time for at least 4–6 days between days 18–24 after AI. The last event is a drop-in rumination time between days 23–25 after AI (equivalent to the rumination time on the day of AI). The occurrence of these three events, in the specified sequence and on the listed days, in the cows enrolled in the trial, produced a significant outcome of non-pregnancy.
Figure 4. Evaluation methods to detect non-pregnant cows using ear tag devices (enrolled cows with negative events, red line).
Based on our hypothesis, the results we have obtained have enabled us to distinguish a distinct postural behavior from the day of artificial insemination, regardless of whether the cows were confirmed pregnant (positive) or not (negative). On the day of artificial insemination, there is a significantly lower resting time compared to the entire observation period, which can be attributed to the heightened activity of cows during heat (Stevenson, 2021). Thus, both lying time and standing time can serve as early indicators of fertilization outcome. A recent study by Stevenson (2021), consistent with our findings, has highlighted the significant effect of the gestational status of the animals (confirmed positive or negative) on resting behavior. Specifically, cows subsequently confirmed pregnant tended to rest more during the peri-oestrous period (−3, +3 days), except for the day of oestrus, where they exhibited a decrease of approximately 11% in resting time.
Our findings indicate that positive animals demonstrated an increased rumination time 9–10 days after AI. Preceding this timeframe, several significant events occur: the embryo’s arrival in the uterus (4–5 days after AI), the morphological transformation from morula to blastocysts (7–8 days after AI), and the simultaneous transformation of the follicle into corpus luteum, initiating progesterone production, followed by implantation (Bruinjé et al., 2019; Ma et al., 2022). These sequential occurrences may correlate with the observed slight reduction in rumination time among positive cows. Further research in this aspect is warranted.
In contrast to our study, where a significant difference in resting time between pregnant and non-pregnant cows was observed on the 21st and 22nd day post-insemination, Stevenson (2021) found no significant differences during the period from 17 to 32 days post-insemination. Additionally, the author further categorized cows into two groups: those with a negative pregnancy diagnosis who had exhibited oestrus, and those who had not. In this context, animals displaying oestrus tended to rest significantly less during the presumed oestrus period compared to the other group. This observation aligns with our study findings, where negative cows exhibited much less resting time from the 20th to the 25th days post-insemination, presumably corresponding to the oestrus period.
However, the assessment of the averages of both groups included in our study has allowed the identification of a typical behavior highlighted also by a recent study by Silper et al. (2017). In this study, Silper et al. (2017) hypothesized an increase in motor activity during oestrus, which consequently also influenced lying and standing behavior during this period. According to the aforementioned study, the extent and intensity of the behavioral change that occurred would have been characterized by a higher ovulation rate and probability of pregnancy per insemination. The results of Silper et al. (2017) demonstrate that the association between variations in lying time during oestrus and fertility achieved after timed artificial insemination is similar to that between activity and fertility described by other studies (Madureira et al., 2015) during spontaneous oestrus. This suggests that behavioral measurements (lying down, standing, and activity), if used in combination, are valuable predictors of fertility. Ultimately, the two factors (lying down and standing) work best when analyzed together in assessing fertility. Further studies, integrating hormonal values as well, could clarify the influence of lying and standing behavior on oestrus expression, the association between the intensity of oestrus expression and fertility, and behavioral changes during the days following artificial insemination in both positive and negative cows.
As previously mentioned, according to our initial hypothesis, positive animals tend to exhibit a numerically greater inactive time, during which lying time increased (from the 20th to the 25th day). This could be attributed to hormonal characteristics and early signs of pregnancy, which tend to increase inactive time to preserve the product of conception. Conversely, we hypothesized that negative animals would show a lower activity parameter due to the resumption of two or three follicular waves, the new cycle, and related hormonal variations. According to Wang et al. (2020), to provide a stable environment for fetal growth, daily activity gradually reduces during pregnancy by the animal, which may also lead to an increase in body weight. Therefore, the change in cow activity after insemination (between 21–25 days after AI) could, according to this study, serve as a reference for pregnancy diagnosis. This result could be consistent with an increase in motor behavior associated with the oestrus of animals that subsequently tested negative in the pregnancy diagnosis. However, the heat monitoring and signaling system did not show activity attributable to oestrus, nor did it reveal differences between cows that tested positive or negative later. This might suggest silent oestrus in cows that later tested negative. Our results are consistent with those reported by Wang et al. (2020), as pregnant animals tended numerically to be less inactive than negative ones. Stevenson (2021) also reports longer periods of inactivity/rest in pregnant cows, focusing exclusively on the lying time of the cows, while the inactivity parameter analyzed by our ear tag device is not associated with any motor behavior but with animals maintaining a lying or standing posture.
Regarding rumination time, upon careful observation of Figure 3, it can be noted that there is no statistical difference between positive and negative animals. However, as previously emphasized, we can observe a minimum value during the day of oestrus/AI, which is justified by the fact that when cows are in heat, rumination time decreases. The result reported by us may be consistent with a recent study by Roland et al. (2018), which analyzed rumination time during the peri-oestrus period. In cows exhibiting natural oestrus, it was demonstrated that rumination decreased starting from one day before oestrus, reaching a nadir on the day of oestrus; this did not occur in the group of cows with induced oestrus, where no decrease in rumination was detected. Despite the fact that the animals in our study did not show statistical differences between animals with synchronization protocol or natural oestrus, we nevertheless observed the same decrease. In accordance with the study by Roland et al. (2018), even for rumination time, according to our assessments, it was not possible to highlight a significant difference between the two study groups. Indeed, the study stated that cows subjected to synchronization, whether subsequently pregnant or empty, did not seem to have any difference in rumination time during the peri-oestrus period. A significant difference in rumination time based on gestational status was also identified, highlighting how negative cows tended to ruminate for a shorter time between days 22 and 26, indicating a possible return to heat (Stevenson, 2021). From the analysis of our results, it was possible to detect rumination behavior as a predictive signal of pregnancy occurrence, as from the 20th to the 26th day, we found a difference in rumination time of about 20 minutes between the two groups under examination.
The influence of forage ration sources on rumination time, lying time, activity time, and inactive time in dairy cows can be substantial and is often influenced by various factors such as silage and hay quality, nutritional composition, and feeding management practices (Gomez and Cook, 2010; Cavallini et al., 2018; Iqbal et al., 2023). High fiber content in rations stimulates rumination activity in dairy cows, resulting in longer rumination times compared to diets with lower fiber content (Beauchemin et al., 1994; Zebeli et al., 2010). While concentrates are more frequent the component of the raions imported from outside the farm and the production area (Gasparini et al., 2024; Vastolo et al., 2024). Silage-based rations, typically made from fermented forage crops like corn or grass, offer a rich source of dietary fiber crucial for rumen health and function (Salfer et al., 2018). The fibrous nature of silage encourages prolonged chewing, promoting saliva production, and buffering rumen acidity, thus aiding in optimal digestion and nutrient absorption.
Palatability plays a crucial role in ensuring cows consume the ration effectively, thereby influencing their activity and inactive time patterns. Indeed, feed palatability is a key factor in animal nutrition (Vinassa et al., 2020). A ration meeting the cow’s nutritional requirements can also promote optimal inactive time by facilitating efficient rumination and digestion. However, inadequate or poorly fermented silage rations, or rations low in fiber content, regardless of their nutritional content, may increase inactive time as cows experience digestive disturbances or seek alternative nutrition sources (Cavallini et al., 2021). For these reasons, forage type and quality significantly influence rumination time, activity time, and lying time in dairy cows, emphasizing the specificity of findings observed in this research. The composition and palatability of the silage/hay-mix diet, alongside management practices, play pivotal roles in shaping cow behavioral patterns (Schirmann et al., 2012; Stone et al., 2017). While high milk yield is advantageous for dairy production, it can induce physiological and metabolic stress in dairy cows, affecting various factors crucial for reproductive success (Allen et al., 2015; Paudyal et al., 2016; Miller-Cushon and DeVries, 2017). Implementing effective management practices to optimize nutrition, body condition, and reproductive performance is vital for maintaining high pregnancy rates in high milk yield dairy herds (Buonaiuto et al., 2024). Our assessments align with those reported by Stevenson (2021), which found no association between cows’ pregnancy status and changes in milk production during the periestral period (−3, +3). However, our study reveals that despite all cows presumably experiencing a negative energy balance postpartum, negative cows produce on average 0.78 kilos more than positive cows, with peaks of +5 kilos, potentially influencing the resumption of reproductive activity. Regarding seasonality’s impact on production performance, existing literature illustrates how high temperatures detrimentally affect milk production in terms of quantity and quality, leading to reductions in fat and protein percentages in the milk (Baul et al., 2014; Bernabucci et al., 2015). In our study, no differences were observed during the summer period, but a significantly higher production was noted during the spring season. It is possible to hypothesize that the animals examined during the spring period in our study were likely the most productive ones on the farm. The observation that high milk yield and seasonal variations can influence rumination time, lying time, and activity time suggests an important consideration for interpreting the results of the study. These factors imply that the behaviors and activities of dairy cows are not static but are influenced by physiological and environmental factors.
One of the most promising aspects is the potential of these technologies to assist farmers. By analyzing data provided by PLF devices, technicians can make more informed and timely decisions regarding dairy cow management (Berckmans, 2014; 2017). For example, monitoring resting time, rumination time, and activity levels can help identify early signs of health or fertility issues, enabling targeted preventive interventions. Furthermore, social media plays a crucial role in disseminating these technologies to farmers and students (Muca et al., 2022; 2023; Lamanna et al., 2024c). This facilitates knowledge dissemination and promotes greater adoption of these technologies in the agricultural sector. However, there are still challenges to be addressed. For instance, the complexity of data generated by PLF devices requires specialized skills for effective interpretation and analysis. Therefore, investing in training for farmers and technicians is essential to maximize the benefits of these technologies.
This study demonstrates that PLF devices provide valuable real-time data for assessing dairy cow reproductive status. By integrating behavioral monitoring into reproductive management strategies, it is possible to develop predictive indicators for early pregnancy detection based on rumination and lying behaviors. Our findings indicate that increased rumination on days 9–10 post-AI, combined with reduced lying time between days 18–25 post-AI, may serve as early indicators of non-pregnancy. However, further validation is necessary to refine predictive models and assess their applicability across diverse farm conditions. Future research should focus on integrating these behavioral indicators with machine learning techniques to enhance the accuracy and practicality of PLF-based reproductive management systems.
The raw data supporting the conclusions of this article will be made available by the authors, without undue reservation.
The animal study was approved by the Ethic Committee of the Veterinary Medicine Department of the University of Teramo. The study was conducted in accordance with the local legislation and institutional requirements.
DC: Data curation, Software, Writing – review & editing. MG: Conceptualization, Methodology, Writing – review & editing. GB: Validation, Writing – original draft. GV: Visualization, Writing – review & editing. JD: Visualization, Writing – review & editing. ML: Visualization, Writing – review & editing. PP: Formal analysis, Methodology, Writing – review & editing. AF: Visualization, Writing – review & editing. IF: Conceptualization, Funding acquisition, Project administration, Resources, Supervision, Writing – review & editing. RC: Writing – review & editing.
The author(s) declare that financial support was received for the research and/or publication of this article. This work has been funded by the European Union -NextGenerationEU, Mission 4, Component 1, under the Italian Ministry of University and Research (MUR) National Innovation Ecosystem grant ECS00000041 -VITALITY -CUP: C43C22000380007.
The authors declare that the research was conducted in the absence of any commercial or financial relationships that could be construed as a potential conflict of interest.
The author(s) declared that they were an editorial board member of Frontiers, at the time of submission. This had no impact on the peer review process and the final decision.
The author(s) declare that no Generative AI was used in the creation of this manuscript.
All claims expressed in this article are solely those of the authors and do not necessarily represent those of their affiliated organizations, or those of the publisher, the editors and the reviewers. Any product that may be evaluated in this article, or claim that may be made by its manufacturer, is not guaranteed or endorsed by the publisher.
Allen J. D., Hall L. W., Collier R. J., Smith J. F. (2015). Effect of core body temperature, time of day, and climate conditions on behavioral patterns of lactating dairy cows experiencing mild to moderate heat stress. J. Dairy Sci. 98, 118–127. doi: 10.3168/jds.2013-7704
Baul S., Cziszter L., Acatincai S., Gavojdian D., Erina S., Marcu A., et al. (2014). Seasonal influences on milk yield and composition dynamics during a normal lactation in dairy cows: milk yield, fat and protein precentage. Sci. Papers: Anim. Sci. Biotechnologies 46, 260–265.
Beauchemin K. A., Farr B. I., Rode L. M., Schaalje G. B. (1994). Effects of alfalfa silage chop length and supplementary long hay on chewing and milk production of dairy cows. J. Dairy Sci. 77, 1326–1339. doi: 10.3168/jds.S0022-0302(94)77072-7
Berckmans D. (2014). Precision livestock farming technologies for welfare management in intensive livestock systems. Rev. Sci. Tech. 33.1, 189. doi: 10.20506/rst.33.1.2273
Berckmans D. (2017). General introduction to precision livestock farming. Anim. Front. 7, 6–11. doi: 10.2527/af.2017.0102
Bernabucci U., Basiricò L., Morera P., Dipasquale D., Vitali A., Piccioli Cappelli F., et al. (2015). Effect of summer season on milk protein fractions in Holstein cows. J. Dairy Sci. 98, 1815–1827. doi: 10.3168/jds.2014-8788
Bianchi M. C., Bava L., Sandrucci A., Tangorra F. M., Tamburini A., Gislon G., et al. (2022). Diffusion of precision livestock farming technologies in dairy cattle farms. animal 16, 100650. doi: 10.1016/j.animal.2022.100650
Bruinjé T. C., Colazo M. G., Ribeiro E. S., Gobikrushanth M., Ambrose D. J. (2019). Using in-line milk progesterone data to characterize parameters of luteal activity and their association with fertility in Holstein cows. J. Dairy Sci. 102, 780–798. doi: 10.3168/jds.2018-14654
Brzozowska A., Łukaszewicz M., Sender G., Kolasińska D., Oprządek J. (2014). Locomotor activity of dairy cows in relation to season and lactation. Appl. Anim. Behav. Sci. 156, 6–11. doi: 10.1016/j.applanim.2014.04.009
Buonaiuto G., Cavallini D., Mammi L. M. E., Ghiaccio F., Palmonari A., Formigoni A., et al. (2021a). The accuracy of NIRS in predicting chemical composition and fibre digestibility of hay-based total mixed rations. Ital. J. Anim. Sci. 20, 1730–1739. doi: 10.1080/1828051X.2021.1990804
Buonaiuto G., Lopez-Villalobos N., Costa A., Niero G., Degano L., Mammi L. M. E., et al. (2023). Stayability in Simmental cattle as aected by muscularity and body condition score between calvings. Front. Vet. Sci. 10. doi: 10.3389/fvets.2023.114128
Buonaiuto G., Palmonari A., Ghiaccio F., Visentin G., Cavallini D., Campidonico L., et al. (2021b). Effects of complete replacement of corn flour with sorghum flour in dairy cows fed Parmigiano Reggiano dry hay-based ration. Ital. J. Anim. Sci. 20, 826–833. doi: 10.1080/1828051X.2021.1916408
Buonaiuto G., Visentin G., Costa A., Niero G., Degano L., Cavallini D., et al. (2024). The effect of first-lactation calving season, milk production, and morphology on the survival of Simmental cows. animal 18, 101128. doi: 10.1016/j.animal.2024.101128
Cangar O., Leroy T., Guarino M., Vranken E., Fallon R., Lenehan J., et al. (2008). Automatic real-time monitoring of locomotion and posture behaviour of pregnant cows prior to calving using online image analysis. Comput. Electron. Agric. 64, 53–60. doi: 10.1016/j.compag.2008.05.014
Cardoso Consentini C. E., Wiltbank M. C., Sartori R. (2021). Factors that optimize reproductive efficiency in dairy herds with an emphasis on timed artificial insemination programs. Anim. (Basel) 11, 301. doi: 10.3390/ani11020301
Cavallini D., Mammi L. M. E., Buonaiuto G., Palmonari A., Valle E., Formigoni A. (2021). Immune-metabolic-inflammatory markers in Holstein cows exposed to a nutritional and environmental stressing challenge. J. Anim. Physiol. Anim. Nutr. 105, 42–55. doi: 10.1111/jpn.13607
Cavallini D., Mammi L. M. E., Fustini M., Palmonari A., Heinrichs A. J., Formigoni A. (2018). Effects of ad libitum or restricted access to total mixed ration with supplemental long hay on production, intake, and rumination. J. Dairy Sci. 101, 10922–10928. doi: 10.3168/jds.2018-14770
Cavallini D., Mammi L. M. E., Palmonari A., García-González R., Chapman J. D., McLean D. J., et al. (2022a). Effect of an immunomodulatory feed additive in mitigating the stress responses in lactating dairy cows to a high concentrate diet challenge. Animals 12, 2129. doi: 10.3390/ani12162129
Cavallini D., Penazzi L., Valle E., Raspa F., Bergero D., Formigoni A., et al. (2022b). When Changing the Hay Makes a Difference: A Series of Case Reports. J. Equine Vet. Sci., 113. doi: 10.1016/j.jevs.2022.103940
Cook N. B., Mentink R. L., Bennett T. B., Burgi K. (2007). The effect of heat stress and lameness on time budgets of lactating dairy cows. J. Dairy Sci. 90, 1674–1682. doi: 10.3168/jds.2006-634
Das S., Shaji A., Nain D., Singha S., Karunakaran M., Baithalu R. K. (2023). Precision technologies for the management of reproduction in dairy cows. Trop. Anim. Health Prod 55, 286. doi: 10.1007/s11250-023-03704-2
De Palo P., Tateo A., Zezza F., Corrente M., Centoducati P. (2006). Influence of free-stall flooring on comfort and hygiene of dairy cows during warm climatic conditions. J. Dairy Sci. 89, 4583–4595. doi: 10.3168/jds.S0022-0302(06)72508-5
Felini R., Cavallini D., Buonaiuto G., Bordin T. (2024). Assessing the impact of thermoregulatory mineral supplementation on thermal comfort in lactating Holstein cows. Veterinary Anim. Sci. 24, 100363. doi: 10.1016/j.vas.2024.100363
Gasparini M., Brambilla G., Menotta S., Albrici G., Avezzù V., Vitali R., et al. (2024). Sustainable dairy farming and fipronil risk in circular feeds: insights from an Italian case study. Food Additives Contaminants - Part A. 41 (12), 1582–1593. doi: 10.1080/19440049.2024.2414954
Giorgino A., Raspa F., Valle E., Bergero D., Cavallini D., Gariglio M., et al. (2023). Effect of dietary organic acids and botanicals on metabolic status and milk parameters in mid-late lactating goats. Animals 13, 797. doi: 10.3390/ani13050797
Girolami F., Barbarossa A., Badino P., Ghadiri S., Cavallini D., Zaghini A., et al. (2022). Effects of turmeric powder on aflatoxin M1 and aflatoxicol excretion in milk from dairy cows exposed to aflatoxin B1 at the EU maximum tolerable levels. Toxins 14 (7). doi: 10.3390/toxins14070430
Gomez A., Cook N. B. (2010). Time budgets of lactating dairy cattle in commercial freestall herds. J. Dairy Sci. 93, 5772–5781. doi: 10.3168/jds.2010-3436
Heinrichs A. J., Heinrichs B. S., Cavallini D., Fustini M., Formigoni A. (2021). Limiting total mixed ration availability alters eating and rumination patterns of lactating dairy cows. JDS Commun. 2, 186–190. doi: 10.3168/jdsc.2020-0074
Iqbal M. W., Draganova I., Henry Morel P. C., Todd Morris S. (2023). Variations in the 24 h temporal patterns and time budgets of grazing, rumination, and idling behaviors in grazing dairy cows in a New Zealand system. J. Anim. Sci. doi: 10.1093/jas/skad038
Koakoski D. L., Bordin T., Cavallini D., Buonaiuto G. A. (2024). Preliminary study of the effects of gaseous ozone on the microbiological and chemical characteristics of whole-plant corn silage. Fermentation 10, 398. doi: 10.3390/fermentation10080398
Lamanna M., Buonaiuto G., Dalla Favera F., Romanzin A., Formigoni A., Cavallini D. (2024a). Precision livestock farming: bridging the gap between media narratives and scientific realities in climate change impact 1673–1680. doi: 10.3168/jds.2024-25347
Lamanna M., Marliani G., Buonaiuto G., Cavallini D., Accorsi P. A. (2024b). Personality traits of dairy cows: relationship between boldness and habituation to robotic milking to improve precision and efficiency. J. Dairy Sci. 640–647.
Lamanna M., Muca E., Buonaiuto G., Formigoni A., Cavallini D. (2024c). From posts to practice - instagram’s role in veterinary dairy cow nutrition education: how does the audience interact and apply knowledge? A survey study. J. Dairy Sci. 108 (2), 1659–1671. doi: 10.3168/jds.2024-25347
Lanzoni L., Chincarini M., Giammarco M., Fusaro I., Gloria A., Contri A., et al. (2021). Maternal and neonatal behaviour in italian mediterranean buffaloes. Animals 11, 1584. doi: 10.3390/ani11061584
Lin D., Kenéz Á., McArt J. A. A., Li J. (2023). Transformer neural network to predict and interpret pregnancy loss from activity data in Holstein dairy cows. Comput. Electron. Agric. 205, 107638. doi: 10.1016/j.compag.2023.107638
Ma J., Burgers E. E. A., Kok A., Goselink R. M. A., Lam T. J. G. M., Kemp B., et al. (2022). Consequences of extending the voluntary waiting period for insemination on reproductive performance in dairy cows. Anim. Reprod. Sci. 244, 107046. doi: 10.1016/j.anireprosci.2022.107046
Madureira A. M. L., Silper B. F., Burnett T. A., Polsky L., Cruppe L. H., Veira D. M., et al. (2015). Factors affecting expression of estrus measured by activity monitors and conception risk of lactating dairy cows. J. Dairy Sci. 98, 7003–7014. doi: 10.3168/jds.2015-9672
Magro S., Costa A., Cavallini D., Chiarin E., de Marchi M. (2024). Phenotypic variation of dairy cows’ hematic metabolites and feasibility of non-invasive monitoring of the metabolic status in the transition period. Front. Veterinary Sci. 11. doi: 10.3389/fvets.2024.1437352
Marques T. C., Marques L. R., Fernandes P. B., de Lima F. S., do Prado Paim T., Leão K. M. (2024). Machine learning to predict pregnancy in dairy cows: an approach integrating automated activity monitoring and on-farm data. Anim. (Basel) 14, 1567. doi: 10.3390/ani14111567
Martin M. J., Dórea J. R. R., Borchers M. R., Wallace R. L., Bertics S. J., DeNise S. K., et al. (2021). Comparison of methods to predict feed intake and residual feed intake using behavioral and metabolite data in addition to classical performance variables. J. Dairy Sci. 104, 8765–8782. doi: 10.3168/jds.2020-20051
McDougall S., Heuer C., Morton J., Brownlie T. (2014). Use of herd management programmes to improve the reproductive performance of dairy cattle. animal 8, 199–210. doi: 10.1017/S1751731114000457
Miller-Cushon E. K., DeVries T. J. (2017). Short communication: Associations between feed push-up frequency, feeding and lying behavior, and milk yield and composition of dairy cows. J. Dairy Sci. 100, 2213–2218. doi: 10.3168/jds.2016-12004
Moallem U., Altmark G., Lehrer H., Arieli A. (2010). Performance of high-yielding dairy cows supplemented with fat or concentrate under hot and humid climates. J. Dairy Sci. 93, 3192–3202. doi: 10.3168/jds.2009-2979
Moretti R., Biffani S., Chessa S., Bozzi R. (2017). Heat stress effects on Holstein dairy cows’ rumination. Animal 11, 2320–2325. doi: 10.1017/S1751731117001173
Muca E., Buonaiuto G., Lamanna M., Silvestrelli S., Ghiaccio F., Federiconi A., et al. (2023). Reaching a wider audience: instagram’s role in dairy cow nutrition education and engagement. Animals 13, 3503. doi: 10.3390/ani13223503
Muca E., Cavallini D., Odore R., Baratta M., Bergero D., Valle E. (2022). Are veterinary students using technologies and online learning resources for didactic training? A mini-meta analysis. Educ. Sci. 12. doi: 10.3390/educsci12080573
Müschner-Siemens T., Hoffmann G., Ammon C., Amon T. (2020). Daily rumination time of lactating dairy cows under heat stress conditions. J. Thermal Biol. 88, 102484. doi: 10.1016/j.jtherbio.2019.102484
Paudyal S. (2021). Using rumination time to manage health and reproduction in dairy cattle: a review. Veterinary Q. 41, 292–300. doi: 10.1080/01652176.2021.1987581
Paudyal S., Maunsell F., Richeson J., Risco C., Donovan A., Pinedo P. (2016). Peripartal rumination dynamics and health status in cows calving in hot and cool seasons. J. Dairy Sci. 99, 9057–9068. doi: 10.3168/jds.2016-11203
Reiter S., Sattlecker G., Lidauer L., Kickinger F., Öhlschuster M., Auer W., et al. (2018). Evaluation of an ear-tag-based accelerometer for monitoring rumination in dairy cows. J. Dairy Sci. 101, 3398–3411. doi: 10.3168/jds.2017-12686
Reith S., Hoy S. (2012). Relationship between daily rumination time and estrus of dairy cows. J. Dairy Sci. 95, 6416–6420. doi: 10.3168/jds.2012-5316
Roland L., Schweinzer V., Kanz P., Sattlecker G., Kickinger F., Lidauer L., et al. (2018). Technical note: Evaluation of a triaxial accelerometer for monitoring selected behaviors in dairy calves. J. Dairy Sci. 101, 10421–10427. doi: 10.3168/jds.2018-14720
Saint-Dizier M., Chastant-Maillard S. (2015). Methods and on-farm devices to predict calving time in cattle. Veterinary J. 205, 349–356. doi: 10.1016/j.tvjl.2015.05.006
Salfer I. J., Morelli M. C., Ying Y., Allen M. S., Harvatine K. J. (2018). The effects of source and concentration of dietary fiber, starch, and fatty acids on the daily patterns of feed intake, rumination, and rumen pH in dairy cows. J. Dairy Sci. 101, 10911–10921. doi: 10.3168/jds.2018-15071
Santos C. A., Landim N. M. D., de Araújo H. X., Paim T do P. (2022). Automated systems for estrous and calving detection in dairy cattle. AgriEngineering 4, 475–482. doi: 10.3390/agriengineering4020031
Schirmann K., Chapinal N., Weary D. M., Heuwieser W., von Keyserlingk M. A. G. (2012). Rumination and its relationship to feeding and lying behavior in Holstein dairy cows. J. Dairy Sci. 95, 3212–3217. doi: 10.3168/jds.2011-4741
Schirmann K., Chapinal N., Weary D. M., Vickers L., von Keyserlingk M. A. G. (2013). Short communication: Rumination and feeding behavior before and after calving in dairy cows. J. Dairy Sci. 96, 7088–7092. doi: 10.3168/jds.2013-7023
Silper B. F., Madureira A. M. L., Polsky L. B., Soriano S., Sica A. F., Vasconcelos J. L. M., et al. (2017). Daily lying behavior of lactating Holstein cows during an estrus synchronization protocol and its associations with fertility. J. Dairy Sci. 100, 8484–8495. doi: 10.3168/jds.2016-12160
Song J., Ma Y., Zhang H., Wang L., Zhang Y., Zhang G. (2023). Fermented total mixed ration alters rumen fermentation parameters and microbiota in dairy cows. Animals 13, 1062. doi: 10.3390/ani13061062
Soriani N., Trevisi E., Calamari L. (2012). Relationships between rumination time, metabolic conditions, and health status in dairy cows during the transition period1. J. Anim. Sci. 90, 4544–4554. doi: 10.2527/jas.2011-5064
Stevenson J. S. (2021). Daily activity measures and milk yield immediately before and after a fertile estrus and during the period of expected return to estrus after insemination in dairy cows. J. Dairy Sci. 104, 11277–11290. doi: 10.3168/jds.2021-20325
Stone A. E., Jones B. W., Becker C. A., Bewley J. M. (2017). Influence of breed, milk yield, and temperature-humidity index on dairy cow lying time, neck activity, reticulorumen temperature, and rumination behavior. J. Dairy Sci. 100, 2395–2403. doi: 10.3168/jds.2016-11607
Szenci O. (2022). Accuracy to predict the onset of calving in dairy farms by using different precision livestock farming devices. Anim. (Basel) 12, 2006. doi: 10.3390/ani12152006
Tapkı İ., Şahin A. (2006). Comparison of the thermoregulatory behaviours of low and high producing dairy cows in a hot environment. Appl. Anim. Behav. Sci. 99, 1–11. doi: 10.1016/j.applanim.2005.10.003
Thompson A. J., Weary D. M., von Keyserlingk M. A. G. (2017). Technical note: Mining data from on-farm electronic equipment to identify the time dairy cows spend away from the pen. J. Dairy Sci. 100, 3975–3982. doi: 10.3168/jds.2016-11713
Toledo I. M., Casarotto L. T., Dahl G. E. (2023). Seasonal effects on multiparous dairy cow behavior in early lactation. JDS Commun. doi: 10.3168/jdsc.2022-0358
Tucker C. B., Jensen M. B., de Passillé A. M., Hänninen L., Rushen J. (2021). Invited review: Lying time and the welfare of dairy cows. J. Dairy Sci. 104, 20–46. doi: 10.3168/jds.2019-18074
Vastolo A., Serrapica F., Cavallini D., Fusaro I., Atzori A. S., Todaro M. (2024). Editorial: Alternative and novel livestock feed: reducing environmental impact. Front. Vet. Sci. 11. doi: 10.3389/fvets.2024.1441905
Vettori J.M., Cavallini D., Giammarco M., Lanzoni L., Vignone O., Jalal H., et al. (2023). Parity and nutrient total-tract digestibility in dairy cows during transition period. Veterinary Anim. Sci. 22, 100313. doi: 10.1016/j.vas.2023.100313
Vinassa M., Cavallini D., Galaverna D., Baragli P., Raspa F., Nery J., et al. (2020). Palatability assessment in horses in relation to lateralization and temperament. Appl. Anim. Behav. Sci. 232, 105110. doi: 10.1016/j.applanim.2020.105110
Wang S., Zhang H., Kou H., Chen X., Lu Y., Li L., et al. (2020). Early pregnancy diagnoses based on physiological indexes of dairy cattle: a review. Trop. Anim. Health Prod 52, 2205–2212. doi: 10.1007/s11250-020-02230-9
Wolfger B., Jones B. W., Orsel K., Bewley J. M. (2017). Technical note: Evaluation of an ear-attached real-time location monitoring system. J. Dairy Sci. 100, 2219–2224. doi: 10.3168/jds.2016-11527
Keywords: precision livestock farming, sensors, rumination, reproductive efficiency, farm management
Citation: Cavallini D, Giammarco M, Buonaiuto G, Vignola G, De Matos Vettori J, Lamanna M, Prasinou P, Colleluori R, Formigoni A and Fusaro I (2025) Two years of precision livestock management: harnessing ear tag device behavioral data for pregnancy detection in free-range dairy cattle on silage/hay-mix ration. Front. Anim. Sci. 6:1547395. doi: 10.3389/fanim.2025.1547395
Received: 18 December 2024; Accepted: 07 March 2025;
Published: 31 March 2025.
Edited by:
Lorenzo Serva, University of Padua, ItalyReviewed by:
Francesco Bonavolontà, University of Naples Federico II, ItalyCopyright © 2025 Cavallini, Giammarco, Buonaiuto, Vignola, De Matos Vettori, Lamanna, Prasinou, Colleluori, Formigoni and Fusaro. This is an open-access article distributed under the terms of the Creative Commons Attribution License (CC BY). The use, distribution or reproduction in other forums is permitted, provided the original author(s) and the copyright owner(s) are credited and that the original publication in this journal is cited, in accordance with accepted academic practice. No use, distribution or reproduction is permitted which does not comply with these terms.
*Correspondence: Giovanni Buonaiuto, Z2lvdmFubmkuYnVvbmFpdXRvQHVuaWJvLml0
Disclaimer: All claims expressed in this article are solely those of the authors and do not necessarily represent those of their affiliated organizations, or those of the publisher, the editors and the reviewers. Any product that may be evaluated in this article or claim that may be made by its manufacturer is not guaranteed or endorsed by the publisher.
Research integrity at Frontiers
Learn more about the work of our research integrity team to safeguard the quality of each article we publish.