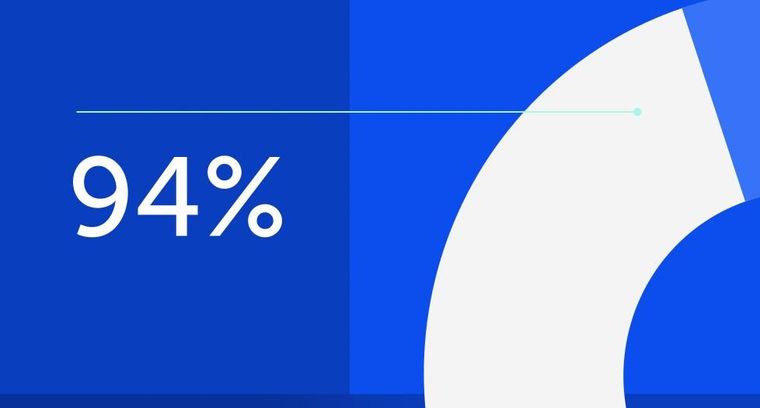
94% of researchers rate our articles as excellent or good
Learn more about the work of our research integrity team to safeguard the quality of each article we publish.
Find out more
ORIGINAL RESEARCH article
Front. Anim. Sci., 14 April 2025
Sec. Precision Livestock Farming
Volume 6 - 2025 | https://doi.org/10.3389/fanim.2025.1543490
This study investigates key physiological, genetic, and environmental factors influencing maternal success in sheep to enhance lamb survival and maternal quality. Using data from native and crossbred prolific ewes in a high-altitude, cold-climate region, we applied machine learning models to predict mothering scores based on dam characteristics, birth conditions, and lamb attributes. Pregnant ewes were monitored 24 hours per day, beginning three days before parturition, with minimal human intervention. Predictor variables included dam breed, body weight, age, litter size, lamb genotype, lambing season, time of lambing, parturition duration, and lambing assistance. Several machine learning algorithms, including Random Forest, Decision Trees, Logistic Regression, and Support Vector Machines (SVM), were evaluated for predictive accuracy. The Random Forest model achieved the highest accuracy (67.2%) and demonstrated the best overall performance with a 0.41 Kappa statistic and the lowest mean absolute error (0.59). Feature importance analysis identified dam weight at birth, parturition duration, and lamb birth weight as the strongest predictors of maternal success. The Decision Tree model highlighted time of lambing, lamb genotype, and lambing assistance as key decision points for classifying mothering ability. Further analysis revealed that shorter parturition durations (≤ 38 min), unassisted lambing, and smaller litter sizes were associated with higher mothering scores. Breed-specific maternal differences were also observed, with crossbred prolific ewes exhibiting stronger maternal instincts. These findings provide actionable insights for precision livestock farming, emphasizing the importance of genetic selection, birthing management, and environmental monitoring to enhance maternal efficiency and lamb survival.
It is well-documented that maternal effects play a highly significant role in offspring, even though in sexually reproducing animals, both parents are equally likely to affect the phenotype of offspring (Chenoweth et al., 2014). It has been emphasized that the offspring’s phenotype can be substantially influenced by the parental phenotype, with significant impacts on growth, survival, and adaptation, since mothers are able to transfer important information about the environment to their offspring (Räsänen and Kruuk, 2007). Neonate survival is dependent on the coordinated expression of appropriate behaviors from both the mother and the lamb, and these behavioral interactions are much more important for prolific sheep with larger litter sizes (Dwyer, 2003; Welsh et al., 2006; Everett-Hincks et al., 2004; Thonney et al., 2007).
Maternal quality in sheep is a multifaceted concept that encompasses various aspects of an ewe’s ability to provide care and support for her offspring. Some of the key factors that contribute to maternal quality include reproductive performance, milk production, and maternal behavior. Reproductive performance is a crucial component of maternal quality, as it determines the ewe’s ability to conceive, carry, and deliver healthy lambs. Factors such as body condition, prolificacy, and the management of breeding and lambing processes can all influence an ewe’s reproductive performance and, by extension, her maternal quality (Gibbons et al., 2019). Ultimately, the ewe’s maternal behavior, encompassing her capacity to bond with her lambs, offer sufficient care and safe-guarding, and respond to their needs, represents a pivotal element of maternal quality.
The Awassi breed, classified as a dairy sheep, is renowned for its high milk production and exceptional adaptability to hot and arid climates (Gootwine, 2011). These sheep possess a calm temperament, primarily due to their frequent handling by humans for milking and dairy management, making them easier to manage in intensive production systems (Galal et al., 2008). Their strong maternal instincts and high milk yield contribute positively to lamb survival and growth. The Morkaraman breed, categorized as a local fat-tailed sheep, is native to the mountainous regions of Eastern Anatolia and exhibits strong resilience to extreme environmental conditions (Akçapınar, 2000; Gürsoy, 2006). Morkaraman sheep are characterized by good temperament and adaptability to extensive grazing systems, which make them a preferred breed in semi-arid and cold environments. Their maternal ability and hardiness allow them to perform well under traditional flock management conditions. Pure and crossbred Romanov ewes belong to the prolific sheep category, renowned for their exceptional reproductive efficiency, early sexual maturity, and high lambing rates (Kutluca et al., 2011). Originating from Russia, the Romanov breed is highly valued for its ability to produce multiple lambs per lambing cycle, significantly enhancing flock productivity (Bodin, 2018). The Tuj breed, classified as a high temperament sheep, is indigenous to cold, high-altitude regions of Turkey and the Caucasus (Karakuş, 2010). Due to the harsh climate and limited feed resources in their native habitat, Tuj sheep exhibit distinct adaptive traits and breeding potential shaped by their environment (Aydin et al., 2024). While their strong survival instincts and robustness make them well-suited for extensive grazing systems, their temperament requires careful handling in intensive production environments. These behavioral and environmental adaptations significantly influence maternal qualities, lamb survival, and flock management strategies. Understanding the specific characteristics of each breed allows for improved selection strategies, management practices, and breeding programs tailored to optimize maternal performance and reproductive success.
Machine learning and animal breeding share key objectives, particularly in prediction, making ML algorithms highly relevant for genomic prediction (Hamadani and Ganai, 2023). In the context of lamb survival, ML methods can analyze large datasets to identify influential factors, aiding in assumption development and informed decision-making (González-Recio and Forni, 2011). Additionally, ML has significant potential in assessing maternal quality in sheep, offering valuable insights for livestock management. Various algorithms, including regression models, decision trees, and neural networks, have been explored to enhance predictive accuracy and decision-making (Hamadani and Ganai, 2023; Fogarty et al., 2021).
This study aimed to apply advanced machine learning algorithms to identify and analyze the key physiological, genetic, and environmental factors influencing maternal quality in sheep. By focusing on predicting mothering scores based on dam characteristics, birth conditions, and lamb attributes, the research sought to enhance the understanding of maternal success. The objective was to develop data-driven insights that could support informed decision-making in sheep breeding and management, ultimately contributing to improved reproductive efficiency and maternal care strategies in precision livestock farming.
This study was conducted in Eastern Anatolia, Turkey, at an altitude of 1,850 meters above sea level, where sheep are traditionally managed under semi-intensive production systems. The region is characterized by long, harsh winters, with temperatures ranging from -10°C to -20°C in January, and short, mild summers, with temperatures between 15°C and 25°C in July. Annual precipitation varies between 400–600 mm, with significant snowfall during the winter months.
The study was carried out over the 2016–2017 lambing seasons, covering spring (March–May), summer (June–August), autumn (September–November), and winter (December–February). Ewes were housed in a semi-open system, designed to provide protection from extreme weather conditions while allowing natural ventilation and outdoor access. Lambing took place in a controlled shed environment designed to provide optimal conditions for maternal care and neonatal survival. Pregnant ewes were fed alfalfa hay and a balanced concentrate ration, formulated to meet their nutritional requirements during late gestation and early lactation. Fresh water was provided ad libitum. Prior to the study, comprehensive health checks were conducted to ensure all ewes were clinically healthy, maintaining a uniform health status across the flock.
The dataset comprised 314 lambs from individual lambing events, representing four distinct breed categories: Awassi (n = 52) as a dairy breed, Morkaraman (n = 68) as a local fat-tailed breed, Romanov and its crossbreeds (n = 23) as prolific breeds, and Tuj (n = 78) as a high-temperament breed. Estrus synchronization protocols were applied to standardize mating periods for synchronized lambing. Ewes were assigned to one of three breeding strategies: (1) natural mating with sires of the same breed to maintain genetic integrity for the lambs (106 lambs), (2) laparoscopic artificial insemination (AI) with frozen Romanov semen for crossbreeding fat-tailed dams to enhance lamb vigor in the crossbred lambs (141 lambs), and (3) frozen embryo transfer (ET), where ewes acted as surrogate mothers for Charollais (25 lambs) and Romanov (42 lambs) embryos, ensuring no genetic relationship between the recipient dams and the resulting lambs. These breeding strategies facilitated the evaluation of maternal responses to different lamb genotypes, with a focus on maternal behavior, lambing duration, and birth assistance requirements under controlled production conditions.
The dataset includes 12 variables, which are categorized into dam-related factors (e.g., dam breed, dam age, dam weight at birth, lambing assistance) and lamb-related factors (e.g., lamb birth weight, lamb genotype, litter size, lamb sex). Additionally, environmental factors such as lambing season and time of lambing were incorporated to assess their influence on maternal score (Table 1).
Maternal behaviors were evaluated through structured observations conducted by researchers trained by the methodology described by Dwyer (2003) to ensure standardized assessment with minimal interference. Observations were carried out in three daily shifts, each lasting eight hours, starting three days before parturition and continuing until 48 hours post-parturition. Researchers closely monitored key maternal behaviors, including lamb grooming, standing to allow suckling, maternal vocalizations, and responsiveness to lamb distress, while providing assistance only when necessary to ensure the welfare of the ewe and lamb. To ensure scoring consistency and minimize subjective bias, two researchers were assigned to each shift, and scores were cross-validated. Any discrepancies in scoring were resolved through consensus discussions.
Maternal care quality was assessed using a standardized behavioral scoring system based on observable ewe-lamb interactions, following the methodology described by Dwyer (2003) and Emsen et al. (2012). Maternal behavior was classified into three levels: Score 1 (Low Maternal Care): The ewe kicks or butts the lamb, actively preventing suckling, displaying rejection behaviors such as avoiding the lamb, showing aggression, or failing to engage in maternal care. The ewe does not respond to lamb distress and does not attempt to bond with the newborn; Score 2 (Medium Maternal Care): The ewe approaches the lamb but does not initiate physical contact and may circle or move away when the lamb attempts to suckle. Maternal responses are inconsistent or passive, with limited grooming and delayed acceptance of the lamb. The ewe may allow suckling but does not actively facilitate it; Score 3 (High Maternal Care): The ewe gets up within three minutes of lamb expulsion, vigorously grooms the lamb, stays in close proximity, and actively facilitates suckling by standing still and allowing the lamb to latch. The ewe exhibits strong maternal instincts, frequently vocalizing to the lamb and responding immediately to lamb distress. Additional qualitative observations were recorded during the 48 hours following parturition, categorizing behaviors such as vocalization type (low-pitched, high-pitched, or none), lamb rejection (present or absent), prolonged lying, and aggressive responses towards lambs or researchers. These behavioral traits were systematically documented and incorporated into the maternal behavior scoring system to provide a comprehensive assessment of maternal success. The structured observation period allowed for a detailed evaluation of early ewe-lamb interactions, ensuring the identification of both positive and negative maternal responses. A complete list of qualitative variables is provided in Appendix 1.
Machine learning classification models were implemented using Python-based frameworks, ensuring efficient data processing, model training, and evaluation. These models were applied to classify maternal success and predict lamb survival based on maternal behavioral traits, physiological parameters, and environmental factors. The dataset included variables such as dam breed, dam body weight at lambing, dam age, litter size at birth, lamb genotype, lamb sex, parturition duration, time of lambing, and lambing assistance. To ensure consistency and optimal model performance, the dataset underwent preprocessing before model training. Categorical variables (e.g., dam breed, lambing season, lamb genotype) were one-hot encoded, while binary categorical variables (e.g., lamb sex) were numerically transformed. Continuous variables (e.g., dam body weight, parturition duration, and lamb birth weight) were normalized to standardize input data. Missing values were handled through imputation, with the mean used for continuous variables and the mode for categorical variables.
A set of classification algorithms was applied, including Random Forest, Decision Tree, Support Vector Machines (SVM), and Logistic Regression, to determine the most effective approach for predicting maternal success. Model performance was evaluated using accuracy, precision, recall, F1-score, and the Kappa statistic. A 10-fold cross-validation procedure was implemented to assess model reliability and prevent overfitting. To optimize predictive performance, hyperparameter tuning was performed using a grid search approach, adjusting parameters such as the number of trees (Random Forest), maximum tree depth, and minimum samples per split (Decision Tree). Feature importance was assessed using Random Forest, which ranks predictors based on their contribution to classification accuracy. Additionally, a Decision Tree model was applied to interpret hierarchical decision-making patterns in maternal success classification.
This study was conducted in accordance with the guidelines for the care and use of animals and was approved by the Institutional Review Board of Atatürk University (protocol code 118, approval date 30.06.2016).
The classification models used to predict maternal ability varied in their performance, as summarized in Table 2. The Random Forest model achieved the highest classification accuracy (67.2%), followed by the Decision Tree (60.5%), Logistic Regression (58.7%), and Support Vector Machine (55.9%). The Random Forest model also had the highest Kappa statistic (0.41), indicating moderate agreement between predicted and actual mothering scores, whereas the Kappa values for the Decision Tree (0.32), Logistic Regression (0.30), and SVM (0.27) were lower, reflecting greater misclassification.
In terms of error metrics, the Random Forest model had the lowest mean absolute error (0.50) and root mean squared error (1.01), indicating superior predictive precision. In contrast, the Decision Tree model exhibited higher error rates, with a mean absolute error of 0.78 and a root mean squared error of 1.12, suggesting a less accurate classification. Logistic Regression performed slightly better than the Decision Tree, while SVM had the highest relative absolute error (83.6%), demonstrating the least effective performance. These findings confirm that ensemble learning methods, such as Random Forest, provide better generalization and robustness in classifying maternal behavior.
Feature importance analysis within the Random Forest model identified dam weight at birth as the most influential predictor of mothering ability, followed by parturition duration and lamb birth weight (Figure 1). This suggests that larger and healthier dams exhibit better maternal behaviors, potentially due to enhanced metabolic reserves and overall robustness. Similarly, lamb birth weight was among the top predictors, with heavier lambs exhibiting stronger maternal interactions. This supports the hypothesis that larger lambs are more viable, have a stronger suckling drive, and receive better maternal attention, reducing rejection risks. Additionally, shorter labor durations were associated with higher maternal scores, indicating that prolonged labor may negatively affect maternal bonding and postnatal care.
Other notable predictors included dam age, lambing season, and genetic background. Older ewes exhibited higher mothering scores, supporting the hypothesis that maternal experience enhances caregiving behaviors. The season of birth also influenced maternal ability, with lambs born in spring showing better maternal care, likely due to favorable environmental conditions and improved feed availability. Genetic factors played a role, as dam breed and lamb genotype significantly contributed to model predictions, suggesting that maternal behavior exhibits a strong hereditary component. Notably, Local Fat-Tailed breeds demonstrated a strong association with improved mothering scores. This could be linked to evolutionary adaptations in native breeds, which may have developed superior maternal instincts to cope with farming conditions. Interestingly, time of lambing and lambing assistance also emerged as relevant factors. Lambing during specific times of the day was linked to better maternal scores, possibly due to circadian rhythms or hormonal influences on parturition timing. Night-time lambing may be an adaptive behavior to minimize predation risks or align with circadian rhythms influencing oxytocin release. Additionally, ewes requiring birthing assistance showed weaker maternal behaviors, reinforcing the importance of early intervention and dystocia management in optimizing maternal success. Additionally, litter size (twin-bearing ewes) was identified as a relevant factor. Twin lambs compete for maternal resources, potentially impacting maternal responsiveness and suckling success. This finding suggests that nutritional supplementation and management interventions could help optimize maternal care in multiple births.
The Decision Tree model provided a structured classification of maternal ability, identifying the most critical decision rules for predicting mothering scores (Figure 2). Time of lambing emerged as the primary decision node, indicating that birth timing strongly influences maternal success. Lambing occurring during specific periods was associated with higher maternal care scores, possibly due to hormonal and environmental factors regulating parturition. The next key decision rule involved lamb genotype, which significantly influenced ewe responses. The tree further branched based on dam age and parturition duration, highlighting that ewes younger than 6.5 years and shorter labor durations (<38 min) were associated with better maternal performance. Additionally, lambing assistance was a critical determinant, as unassisted births were more likely to result in stronger mothering behaviors.
Breed-related influences were also evident, as seen in the final decision branches, which included dam breed category and litter size. Smaller litter sizes (e.g., single and twin lambs) were associated with higher maternal care scores, likely due to reduced competition for maternal resources. These findings highlight the complex interplay of physiological, genetic, and environmental factors in determining maternal success. The results underscore the importance of selective breeding and targeted reproductive management strategies to improve maternal success and lamb survival.
The findings of this study provide valuable insights into the factors influencing maternal ability in sheep, with direct implications for flock management and breeding programs. The feature importance analysis identified dam weight at birth, parturition duration, and lamb birth weight as the most significant predictors of mothering ability. These results align with previous studies indicating that maternal body condition, birth-related stress, and neonatal viability are key determinants of successful mothering behaviors in livestock (Dwyer, 2008; Cloete and Scholtz, 1998). Dam weight at birth emerged as the strongest predictor of maternal ability, suggesting that larger dams develop superior maternal instincts and provide better care for their offspring. This finding is consistent with research indicating that ewe body condition is positively correlated with milk production, lamb viability, and maternal attentiveness (Nowak and Poindron, 2006). Moreover, older ewes demonstrated stronger maternal behaviors, reinforcing the notion that maternal experience plays a significant role in lamb survival and ewe-lamb bonding (Dwyer and Lawrence, 2005).
Parturition duration was another major predictor, with longer labor times associated with lower mothering scores. Prolonged labor can lead to increased stress, fatigue, and delayed maternal bonding, which may result in higher lamb rejection rates and lower suckling success (Mora-Medina et al., 2016). These findings highlight the importance of monitoring dystocia cases and providing timely intervention to support maternal responsiveness postpartum.
Lamb birth weight was a key determinant of mothering success, with heavier lambs receiving stronger maternal care. This supports prior studies suggesting that larger lambs have higher survival rates due to improved thermoregulation and stronger suckling reflexes (Dwyer et al., 2016). The role of lambing season in maternal success was also evident, with ewes lambing in Spring exhibiting better maternal scores than those lambing in Winter, likely due to more favorable environmental conditions and improved nutritional availability (Nowak, 1996). Breed-specific differences further shaped maternal behaviors. Local fat-tailed breeds, such as Morkaraman, exhibited stronger maternal responses compared to prolific breeds like Romanov, which aligns with prior research indicating that highly prolific breeds often require more human intervention due to weaker maternal instincts (Cloete and Scholtz, 1998). The Decision Tree model highlighted the influence of time of lambing on mothering success, reinforcing the idea that hormonal cycles and natural rhythms may influence parturition timing and subsequent maternal responses (Dwyer, 2013).
The application of machine learning in maternal behavior assessment demonstrates the potential for precision livestock farming (PLF) technologies to improve reproductive management. The high predictive power of the Random Forest model suggests that data-driven selection of maternal traits can optimize flock productivity and lamb survival. However, as noted in previous studies, machine learning models alone cannot fully capture the complexity of maternal care (Goursaud and Nowak, 1999). Future studies should integrate real-time behavioral monitoring, hormonal profiling, and sensor-based tracking to enhance the accuracy of maternal assessments (Mora-Medina et al., 2016).
While the models successfully identified key predictors, maternal behavior is a multifaceted trait influenced by various environmental, physiological, and genetic interactions. The current approach did not include hormonal indicators of maternal stress or oxytocin levels, which have been shown to influence ewe-lamb bonding and milk production (Keverne et al., 1992). Future studies should incorporate biological markers alongside behavioral observations to refine predictive models further.
This study demonstrates that maternal ability in sheep is significantly influenced by dam weight at birth, parturition duration, lamb birth weight, and breed-specific traits. The Random Forest model proved to be the most reliable machine learning algorithm for predicting maternal success, offering potential applications in selective breeding and reproductive management strategies. However, the findings also highlight the need for a holistic approach that combines data-driven insights with qualitative behavioral assessments to capture the full complexity of maternal care. Future research should explore integrating real-time monitoring, hormonal profiling, and environmental factors to further refine predictive frameworks and enhance precision livestock farming applications.
The raw data supporting the conclusions of this article will be made available by the authors, without undue reservation.
The animal study was approved by In-stitu-tional Review Board (Ethics Committee) of Atatürk University (protocol code 118, approval date 30.06.2016), Erzurum, Türkiye. The study was conducted in accordance with the local legislation and institutional requirements.
EE: Conceptualization, Funding acquisition, Methodology, Project administration, Resources, Supervision, Validation, Writing – review & editing. BO: Conceptualization, Data curation, Formal analysis, Investigation, Methodology, Software, Validation, Visualization, Writing – original draft. MK: Conceptualization, Formal analysis, Methodology, Resources, Validation, Writing – review & editing.
The author(s) declare that financial support was received for the research and/or publication of this article. This research was partly funded by the Innovation for Sustainable Sheep and Goat Production in Europe (iSAGE-679302).
The authors declare that the research was conducted in the absence of any commercial or financial relationships that could be construed as a potential conflict of interest.
The author(s) declare that Generative AI was used in the creation of this manuscript. The authors verify and take full responsibility for the use of generative AI in the preparation of this manuscript. Generative AI was used to refine language, improve grammar, and organize citations while ensuring all scientific content, analysis, and original text were produced by the authors.
All claims expressed in this article are solely those of the authors and do not necessarily represent those of their affiliated organizations, or those of the publisher, the editors and the reviewers. Any product that may be evaluated in this article, or claim that may be made by its manufacturer, is not guaranteed or endorsed by the publisher.
The Supplementary Material for this article can be found online at: https://www.frontiersin.org/articles/10.3389/fanim.2025.1543490/full#supplementary-material
Aydin K. B., Ye B., Brito L. F., Ulutaş Z., Morota G. (2024). Review of sheep breeding and genetic research in Türkiye. Frontiers in Genetics 15, 1308113. doi: 10.3389/fgene.2024.1308113
Bodin L., Raoul J., Bunter K. L., Swan A., Janssens S., Brito L. (2018). Distribution of sheep litter size: a worldwide survey. In: Proceedings of the 69th Annual Meeting of the European Federation of Animal Science (EAAP), 27–31 August 2018 Dubrovnik, Croatia: Wageningen Academic Publishers 24, 705–546.
Chenoweth P. J., Landaeta-Hernández A., Flöercke C. (2014). Reproductive and maternal behavior of livestock. In: Grandin T. (Ed.), Genetics and the Behavior of Domestic Animals (Amsterdam, The Netherlands: Elsevier BV) 159–194.
Cloete S. W. P., Scholtz A. J. (1998). Lamb survival in relation to lambing and neonatal behavior in medium wool Merino lines divergently selected for multiple rearing ability. Aust. J. Exp. Agric. 38, 801–811. doi: 10.1071/EA97155
Dwyer C. M. (2003). Behavioural development in the neonatal lamb: Effect of maternal and birth-related factors. Theriogenology 59, 1027–1050. doi: 10.1016/S0093-691X(02)01147-8
Dwyer C. M. (2008). The welfare of the neonatal lamb. Small Ruminant Res. 76, 31–41. doi: 10.1016/j.smallrumres.2007.12.011
Dwyer C. M. (2013). Maternal behaviour and lamb survival: From neuroendocrinology to practical application. Animal 8, 102–112. doi: 10.1017/S175173111300194
Dwyer C. M., Lawrence A. B. (2000). Maternal behaviour in domestic sheep (Ovis aries): Constancy and change with maternal experience. Behaviour 137 (10), 1391–1413. doi: 10.1163/156853900501999
Dwyer C. M., Conington J., Corbière F., Holmøy I. H., Muri K., Nowak R., et al (2016). Invited review: Improving neonatal survival in small ruminants: science into practice. Animal 10 (3), 449–459. doi: 10.1017/S1751731115001974
Emsen E., Diaz C. A. G., Yaprak M., Koycegiz F., Kutluca M., Emsen H. (2012). Effect of inter-breed embryo transfer on lamb growing performance and survival. Reprod. Domest Anim. 47, 8–11. doi: 10.1111/j.1439-0531.2008.01200.x
Everett-Hincks J. M., López-Villalobos N., Blair H. T., Stafford K. J. (2004). The effect of ewe maternal behavior score on lamb and litter survival. Livest Prod Sci. 93, 51–61. doi: 10.1016/j.livprodsci.2004.07.001
Fogarty E. S., Swain D. L., Cronin G. M., Moraes L., Bailey D. W., Trotter M. (2021). Developing a simulated online model that integrates GNSS accelerometer and weather data to detect parturition events in grazing sheep: A machine learning approach. Animals 11, 303. doi: 10.3390/ani11020303
Galal S., Gürsoy O., Shaat I. (2008). Awassi sheep as a genetic resource and efforts for their genetic improvement. Small Ruminant Res. 79, 99–108. doi: 10.1016/j.smallrumres.2008.07.018
Gibbons A., Fernández J., Bruno-Galarraga M. M., Spinelli M. V., Cueto M. (2019). Technical recommendations for artificial insemination in sheep. Arq Bras. Med. Vet. Zootec 16, 803–809. doi: 10.1590/1678-4162-10894
González-Recio Ó., Forni S. (2011). Genome-wide prediction of discrete traits using Bayesian regressions and machine learning. Genet. Sel Evol. 43, 9. doi: 10.1186/1297-9686-43-9
Gootwine E. (2011). Breeding Awassi and Assaf sheep for diverse management conditions. Trop. Anim. Health Prod 43, 1289–1296. doi: 10.1007/s11250-011-9852-y
Goursaud A. P., Nowak R. (1999). Colostrum mediates the development of mother preference by newborn lambs. Physiol. Behav. 67, 49–56. doi: 10.1016/S0031-9384(99)00043-9
Gürsoy O. (2006). Economics and profitability of sheep and goat production in Turkey under new support regimes. Small Ruminant Res. 62, 181–191. doi: 10.1016/j.smallrumres.2005.08.013
Hamadani A., Ganai N. A. (2023). Artificial intelligence algorithm comparison and ranking for weight prediction in sheep. Sci. Rep. 13, 5427. doi: 10.1038/s41598-023-32438-w
Ölmez M., Riaz R., Karadağoğlu Ö., Şahin T., Şerbetçi İ., Yılmaz B., et al (2023). Effect of SOD-rich melon supplement on performance, serum biochemical, antioxidant, and meat quality characteristics of Tuj lambs. Agriculture 13, (3), 625. doi: 10.3390/agriculture13030625
Keverne E. B., Kendrick K. M., Hinton M. R. (1992). Oxytocin and maternal behavior in sheep. Ann. N Y Acad. Sci. 689, 192–203.
Kutluca M., Emsen E., Koycegiz F., Gimenez-Diaz A. C., Aslan A. F. (2011). Reproductive performance and milk traits of F1 Romanov ewes. Small Ruminant Res. 100, 34–36. doi: 10.1016/j.smallrumres.2011.05.016
Mora-Medina P., Orihuela A., Arch-Tirado E., Roldán-Santiago P., Terrazas A., Mota-Rojas D. (2016). Sensory factors involved in mother-young bonding in sheep: A review. Vet. Med. 61, 595–611. doi: 10.17221/52/2016-VETMED
Nowak R. (1996). Neonatal survival: Contributions from behavioral studies in sheep. Appl. Anim. Behav. Sci. 49, 61–72. doi: 10.1016/0168-1591(95)00668-0
Nowak R., Poindron P. (2006). From birth to colostrum: Early steps leading to lamb survival. Reprod. Nutr. Dev. 46, 431–446. doi: 10.1051/rnd:2006023
Räsänen K., Kruuk L. E. B. (2007). Maternal effects and evolution at ecological time-scales. Funct. Ecol. 21, 408–421. doi: 10.1111/j.1365-2435.2007.01246.x
Thonney M. L., Martínez-Hernández P., Taylor J. B., Murphy T. (2007). Sheep symposium: Biology and management of low-input lambing in easy-care systems. J. Anim. Sci. 86, E244–E245. doi: 10.2527/jas.2006-759
Keywords: maternal quality, machine learning, maternal behavior, livestock management, lamb survival
Citation: Emsen E, Odevci BB and Kutluca Korkmaz M (2025) Using machine learning to identify key predictors of maternal success in sheep for improved lamb survival. Front. Anim. Sci. 6:1543490. doi: 10.3389/fanim.2025.1543490
Received: 11 December 2024; Accepted: 18 March 2025;
Published: 14 April 2025.
Edited by:
Juan Mauricio Alvarez, National Institute of Agricultural Technology (INTA), ArgentinaReviewed by:
Gabriel Ciappesoni, National Institute for Agricultural Research (INIA), UruguayCopyright © 2025 Emsen, Odevci and Kutluca Korkmaz. This is an open-access article distributed under the terms of the Creative Commons Attribution License (CC BY). The use, distribution or reproduction in other forums is permitted, provided the original author(s) and the copyright owner(s) are credited and that the original publication in this journal is cited, in accordance with accepted academic practice. No use, distribution or reproduction is permitted which does not comply with these terms.
*Correspondence: Ebru Emsen, ZWJydWVtc2VuQHVhZXUuYWMuYWU=
Disclaimer: All claims expressed in this article are solely those of the authors and do not necessarily represent those of their affiliated organizations, or those of the publisher, the editors and the reviewers. Any product that may be evaluated in this article or claim that may be made by its manufacturer is not guaranteed or endorsed by the publisher.
Research integrity at Frontiers
Learn more about the work of our research integrity team to safeguard the quality of each article we publish.