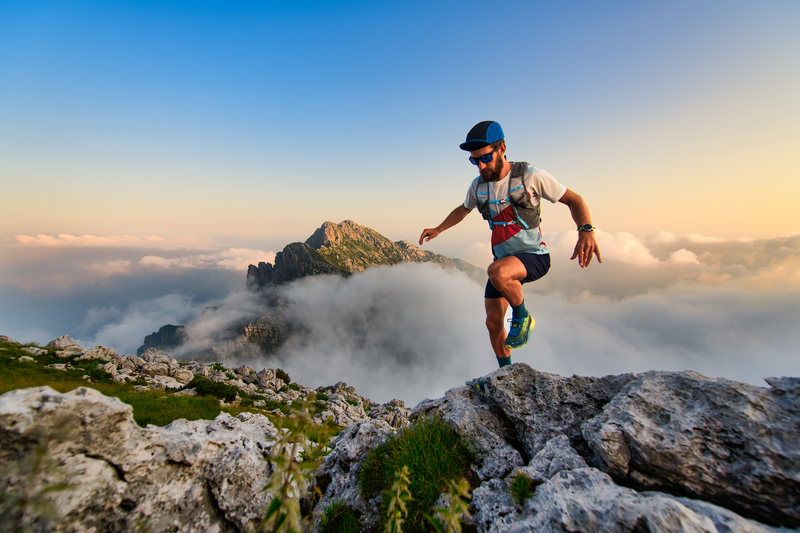
94% of researchers rate our articles as excellent or good
Learn more about the work of our research integrity team to safeguard the quality of each article we publish.
Find out more
METHODS article
Front. Anim. Sci.
Sec. Animal Welfare and Policy
Volume 6 - 2025 | doi: 10.3389/fanim.2025.1532385
The final, formatted version of the article will be published soon.
You have multiple emails registered with Frontiers:
Please enter your email address:
If you already have an account, please login
You don't have a Frontiers account ? You can register here
Dairy cattle's susceptibility to diseases significantly impacts their health, welfare, and longevity.Disability weights reflect the relative severity or impact of important diseases and provide an extension of epidemiological frequency measures. They are central for comparing disease burden across diverse causes when summarizing health status and disease severity. Yet, they often reflect group-level health status and rely on expert judgment, which is subjective. In absence of an objective approach, this study aimed to create disability weight metrics using pathophysiological data with machine learning approach. Four binary classifiers using a generalized linear model with Lasso regularization were developed to identify distinguishing features for healthy and diseased cows affected by hypocalcemia, ketosis, metritis and mastitis. Model performance, assessed via the Area Under the Curve (AUC), reached values of 0.72, 0.66, 0.82, and 0.92 for distinguishing hypocalcemia, ketosis, metritis and mastitis in cows from healthy groups. The selected features were combined into a summary disability weight -cumulative health measure -for each disease computed through weighted sums of feature importance from classification models. Notably, the average cumulative health measure differed significantly between healthy and diseased groups (p < 0.05). The relative ranking of diseases based on the average cumulative health measure was comparable to the expert survey-based approach reported in McConnel et al. (2017). Such features will offer insights into disease impact and will provide a standardized metric for comparing disease severity.
Keywords: Disability weight, pathophysiological data, Behavior data, Gene expression data, Feature Selection, disease burden
Received: 21 Nov 2024; Accepted: 26 Feb 2025.
Copyright: © 2025 Marzougui, McConnel, Adams Progar, Biggs, Ficklin and Sankaran. This is an open-access article distributed under the terms of the Creative Commons Attribution License (CC BY). The use, distribution or reproduction in other forums is permitted, provided the original author(s) or licensor are credited and that the original publication in this journal is cited, in accordance with accepted academic practice. No use, distribution or reproduction is permitted which does not comply with these terms.
* Correspondence:
Sindhuja Sankaran, Washington State University, Pullman, United States
Disclaimer: All claims expressed in this article are solely those of the authors and do not necessarily represent those of their affiliated organizations, or those of the publisher, the editors and the reviewers. Any product that may be evaluated in this article or claim that may be made by its manufacturer is not guaranteed or endorsed by the publisher.
Research integrity at Frontiers
Learn more about the work of our research integrity team to safeguard the quality of each article we publish.