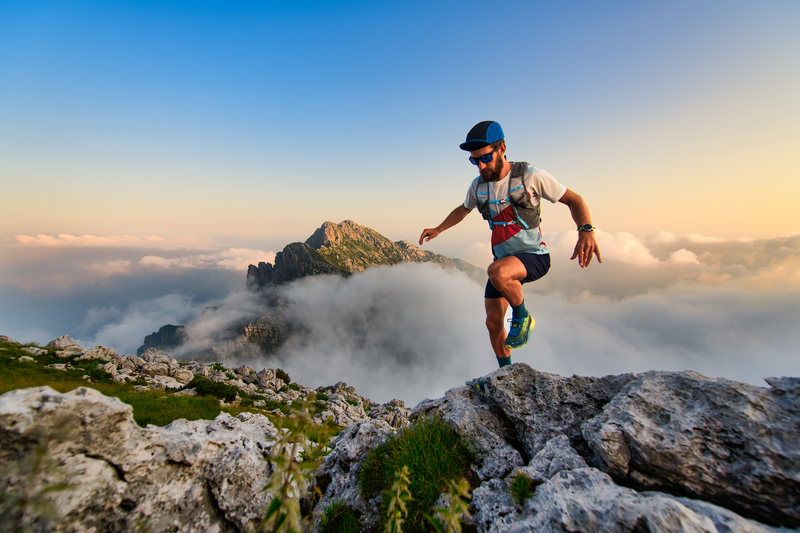
95% of researchers rate our articles as excellent or good
Learn more about the work of our research integrity team to safeguard the quality of each article we publish.
Find out more
ORIGINAL RESEARCH article
Front. Anim. Sci. , 03 January 2025
Sec. Precision Livestock Farming
Volume 5 - 2024 | https://doi.org/10.3389/fanim.2024.1499253
Standing and lying times of animals are often used as an indicator to assess welfare and health status. Changes in standing and lying times due to health problems or discomfort can reduce productivity. Since manual evaluation is time-consuming and cost-intensive, video surveillance offers an opportunity to obtain an unbiased insight. The objective of this study was to identify the individual heifers in group housing and to track their body posture (‘standing’/’lying’) by training a real-time monitoring system based on the convolutional neural network YOLOv4. For this purpose, videos of three groups of five heifers were used and two models were trained. First, a body posture model was trained to localize the heifers and classify their body posture. Therefore, 860 images were extracted from the videos and the heifers were labeled ‘standing’ or ‘lying’ according to their posture. The second model was trained for individual animal identification. Only videos of one group with five heifers were used and 200 images were extracted. Each heifer was assigned its own number and labeled accordingly in the image set. In both cases, the image sets were divided separately into a test set and a training set with the ratio (20%:80%). For each model, the neural network YOLOv4 was adapted as a detector and trained with an own training set (685 images and 160 images, respectively). The accuracy of the detection was validated with an own test set (175 images and 40 images, respectively). The body posture model achieved an accuracy of 99.54%. The individual animal identification model achieved an accuracy of 99.79%. The combination of both models enables an individual evaluation of ‘standing’ and ‘lying’ times for each animal in real time. The use of such a model in practical dairy farming serves the early detection of changes in behavior while simultaneously saving working time.
Health and welfare of animals is a highly discussed topic in public. Various factors, such as rising air temperatures, diseases or unfavorable husbandry conditions, lead to changes in the behavior of cows. The activity level of dairy cows is closely related to their welfare, health status and productivity. Therefore, it is often used for health monitoring (Weary et al., 2009; Dittrich et al., 2019). Changes in the typical behavioral pattern indicate reduced welfare (Sharma and Koundal, 2018; Dittrich et al., 2019). It can also provide information on the health status. According to Johnson (2002) and Tizard (2008) disease-related behavior is an adaptive change in behavior in response to an infection or injury. It is often manifested by reduced activity level due to inflammation, fever, injury, pain or social isolation. As part of ensuring animal welfare, cow behavior can be used for the early detection of diseases (Sharma and Koundal, 2018). This improves the recovery of sick cows, reduces veterinary costs and culling rates, and reduces the negative impact on milk production (Dittrich et al., 2019).
Certain diseases show specific changes in activity level. Activity level distinguishes between physical activity and resting. Resting includes lying and standing still, while physical activity comprises locomotion and restless behavior during milking (Weary et al., 2009; Dittrich et al., 2019). Typically, the average daily lying time is between 12 and 13 hours (Munksgaard et al., 2005), depending on the status of lactation and pregnancy progress (Westin et al., 2016; Maselyne et al., 2017). A decreasing lying time leads to a reduction in productivity and an increase of health problems, such as hypoglycemia and ketosis. In the case of lameness, a cow is lying more due to pain on standing (Munksgaard et al., 2005; Schirmann et al., 2012; Westin et al., 2016; Tucker et al., 2021). In contrast, the standing time increases when a cow suffers from mastitis (Medrano-Galarza et al., 2012). The cow takes more but smaller steps due to pain in the udder also it is restless while milking (Fogsgaard et al., 2015). Therefore, standing and lying are important indicators for evaluating animal welfare (Fregonesi and Leaver, 2001). Early detection of changes in ‘standing’ and ‘lying’ time requires almost 24/7 monitoring. That is not possible to do by staff (Mcdonagh et al., 2021).
With increasing digitalization, various types of wearable sensors have been developed to measure activity as well as standing and lying times. These are pedometers, activity meters or accelerometers (Rutten et al., 2013). Depending on the design, these sensors can be attached to the ear, collar, leg, or even ingested as a rumen bolus (Helwatkar et al., 2014). These sensors can only capture data on an individual animal basis, which means that each animal must be equipped with such a sensor, which can be uncomfortable or disruptive for the cow (Zheng et al., 2023). Moreover, data collection is temporally dependent on battery life, and there is a possibility of loss or damage. Normally, they are then no longer usable in both cases (Andrew et al., 2021).
Video monitoring combined with computer vision does not require the cows to be fitted with additional sensors. It is a stress-free, non-invasive, cost-effective and simple method of monitoring animals without human contact (Nasirahmadi et al., 2017; Li et al., 2021; Zhang et al., 2023). In addition, continuous monitoring is possible. Hofstra et al. (2022) also note that computer vision technology may have the greatest potential for welfare monitoring in an indoor environment. Computer vision and deep learning techniques enable different types of monitoring e.g. identification, localization, behavior (Cangar et al., 2008). In previous studies Shen et al. (2020) used the full side view of cows for identification and trained a YOLO model while. Dac et al. (2022) applied face recognition to cows. Another approach to individual animal recognition was undertaken by Dulal et al. (2022) by using the grain of the muzzle of cows like a fingerprint and trained a YOLO model. Posture tracking has already been studied for different animal species using convolutional neural networks (Cangar et al., 2008; Nasirahmadi et al., 2019; Schütz et al., 2021, 2022). Such techniques have only been used separately for individual identification or for tracking body posture.
The aim of this study was therefore to combine individual animal identification with posture detection. Here, individual heifers were automatically identified and their individual posture (‘standing’/’lying’) was tracked by training a real-time monitoring system based on the convolutional neural network YOLOv4. This monitoring system is intended to enable automated and continuous monitoring to detect changes in the standing and lying times of individual heifers or cows at an early stage.
The video material used in this study was recorded during an animal experiment (authorized by the local authority in Mecklenburg-Western Pomerania (Landesamt für Landwirtschaft, Lebensmittelsicherheit und Fischerei (LALLF) Mecklenburg-Vorpommern), # FLI-7221.3-1-047/17) at the Friedrich-Loeffler-Institut on the isle of Riems – Greifswald (Germany). Heifers were infected with mycoplasma (Mycoplasma mycoides) (Hänske et al., 2023). A total of 20 heifers (Bos Taurus) of the Holstein Friesian breed were divided into four groups of five animals. All heifers were clinically unremarkable during the entire duration of the experiment. Only three pens were equipped with cameras, therefore only three groups could be monitored by cameras 24 hours per day.
The groups were kept in pens with feed fences. The size of the pens was approximately 6 m long and 4 m wide with a solid floor. Separate cubicles were not available. There was no separation of feeding, walking or lying area. The feeding troughs were positioned on the gangway directly behind the feed fence. All animals were fed once a day and given veterinary care. The pens were cleaned by animal caretaker during each feeding process.
In this study, two different types of dome cameras were used to monitor the heifers. In total, three cameras were used, two DIGITUS DN-16081-1 (ASSMANN Electronic GmbH, Lüdenscheid, Germany) and one HiWatch IR Network Camera DS-I111 (Hangzhou Hikvision Digital Technology Co., Ltd., Hangzhou, Zhejiang, China). 3.3 TB of video material was recorded and stored directly on a server at the Friedrich-Loeffler-Institut. The recording durations and recording periods of the cameras are shown in Table 1.
Each pen was equipped with one camera. The cameras were installed directly under the ceiling at a height of approximately 3.5 m. The exact positions of the cameras in each pen can be seen in Figure 1. In the recordings, small parts of the stables are covered by the feed fence or metal rods. The pens were isolated from the outside world without natural daylight. The lighting was provided by artificial light and was regulated according to the light conditions at day and night.
Figure 1. Bird’s eye view of the three pens, with the positions of the cameras. The colored areas are not visible in the respective camera perspective. Pen 1: DIGITUS DN-16081-1 (yellow), pen 2: DIGITUS DN-16081-1 (red), pen 3: HiWatch IR Network Camera DS-I111 (Created in BioRender. Jahn, S. (2024) https://BioRender.com/s01k326).
Video surveillance was carried out continuously throughout the experiment, resulting in recordings day and night. All three cameras had a night vision function so that they recorded at night via infrared and the heifers were not affected. A single video sequence had a length of 117 seconds (DIGITUS) or 45 minutes (HiWatch) (Table 2). The recordings differed in their image sizes depending on the type of camera. The DIGITUS recorded with an image size of 2688 pixels x 1512 pixels (horizontal x vertical), while the image size of the HiWatch was smaller at 1280 pixels x 720 pixels. The frame rate of 25 frames per second was the same for both camera types.
In this study, a subset of the videos was used for training of the models. This corresponds to a total duration of 05:04:21 [hh:mm:ss] (Table 2).
The images of both image sets were extracted from the videos on a Windows 10 Enterprise 64-bit operating system by using the VLC media player (VideoLAN, Paris, France). For this purpose, images with different illumination conditions, like day and night, were selected. The images were also given a high level of variability. The image size of the extracted images corresponded to the image size during the recordings depending on the type of camera (Table 2).
The software LabelImg (Tzutalin, 2015) was used for the labeling of the extracted images. To set a label, the object is framed by a rectangle and assigned to the defined classes. LabelImg saves a txt file for each image. Each line of a file contains the labeled class and the x and y coordinates of the center of the ground truth bounding box, as well as its width and height. All values relating to the bounding box are normalized and range between 0 and 1. The labeling was done by only one person in order to minimize the bias due to different perceptions.
The body posture image set contained 860 images of heifers in ‘standing’ or ‘lying’ posture. Due to the cameras in the pens filming from different perspectives, the extracted images had a high degree of variability in the posture characteristics. The heifers in each image were manually labeled and attributed to one of the two classes, ‘lying’ (1962 labels) or ‘standing’ (2160 labels). Therefore, ‘standing’ was defined as the body posture as long as the chest did not touch the floor. It begins with the change from the ‘lying’ to the ‘standing’ body posture and ends when the body is ‘lying’ again. The body posture was defined as ‘lying’ when the chest touched the floor. No distinction was made between prone and lateral posture. Occasionally, body parts were covered by pen equipment or other heifers. In these cases, these coverings are included in the labeled image section. Since several animals were present in the images, we were able to assign more than one label per image. The body posture image set was then used to train and validate the model to detect the ‘standing’ and ‘lying’ body posture of a heifer. Table 3 shows in detail the split of body posture image set into a test set (175 images) and a training set (685 images) in the ratio 20% to 80%.
Table 3. Body posture image set: Splitting of the image set with 860 images into a test set and a training set in the ratio of 20% to 80%.
The individual animal identification image set consisted of 200 extracted images and was used for individual animal identification based on the coat pattern (Figure 2; Supplementary Figure S1). For this purpose, images were selected from three hours of video material in which the individual heifers could be seen in different postures and positions for the camera. These were used to individualize the heifers and were taken only from pen 2. The classification of individual animal identification image set was also done manually. Each individual heifer was given its own class. This resulted in five classes (‘heifer_1’, ‘heifer_2’, ‘heifer_3’, ‘heifer_4’, ‘heifer_5’) for this image set. Due to the position of the cameras, not all animals were visible at all times. They were in the blind spot of the camera or were covered by another heifer. As long as the individual coat pattern of the heifers was identifiable, the visible section was labeled and classified. Individual animal identification image set was used to train and validate a model to detect the individual heifer. The number of images and labels per class of individual animal identification image set and the split into the test set and the training set are given in Table 4.
Figure 2. Heifers were detected and located by coat pattern using the individual detector. (A) All heifers were detected with a precision of 100%. (B) Heifer_1 is covered by heifer_4.
Table 4. Individual animal identification image set: Splitting of the image set with 200 images into a test set and a training set in the ratio of 20’% to 80%.
To apply the body posture model to new data material, the video material from four further days (day 1, day 2, day 3, day 4) was used. It was only from pen 2. The videos of day 2 and day 3 were used firstly for manual analysis and secondly to evaluate the automated method. All four days were considered in the automated analysis with the validated model.
In this study, the deep learning algorithm YOLOv4 (Bochkovskiy et al., 2020) was used. YOLO (‘you only look once’) is a one-stage object detection algorithm, i.e. a single convolutional neural network (CNN) is used to process images, and is able to directly determine the localization and classification of the detected objects (Redmon et al., 2016).
The image sets with their parameters of YOLOv4 for training of both models are shown in Table 5. The setup and procedure of these training sessions were the same as those already used for foxes (Schütz et al., 2021, 2022).
Table 5. Parameters of the training of the body posture model and the individual animal identification model.
To evaluate the model performance the following parameters were calculated.
The Intersection over Union (IoU) describes the overlap of the bounding box detected by the model and the manual-created bounding box. It is the quotient of their overlapping area and the union area of both boxes (Equation 1) (Everingham et al., 2010).
The resulting ratio indicates whether an object was detected as a true positive (TP) or false positive (FP) by the model. In this case, the threshold value for the IoU was set at 0.5. If the IoU was 0.5 or higher, the detection was considered a TP. For lower values, the detection was a FP. The result was false negative (FN), if a manual-created bounding box was not detected by the model.
The precision indicates how often the model has correctly recognized an object. The quotient is calculated from the number of TP and the sum of TP and FP identified objects (Equation 2) (Chen et al., 2022).
Another parameter is the recall, which indicates whether the model has identified every object to be detected. It is described by the ratio of TP and the sum of TP and FN, this corresponds to the number of labels () (Equation 3) (Chen et al., 2022).
In this case, the interpolated average precision (AP) was used. The precision-recall curve is calculated for the task and class. It is calculated from eleven equidistant recall levels [0,0.1,…,1] (Equation 4) (Everingham et al., 2010).
with
is the precision at recall (Equation 5).
The AP includes only one class, therefore the mean average precision (mAP) is used to evaluate models with more than one class. The average value of the APs of all classes (N) is calculated for this (Equation 6) (Chen et al., 2022).
These parameters are needed to select the best model.
The operating system used in this study was Linux CentOS 7. The processor was an Intel Xenon E5-2667 v4 with 3.20 GHz, 377 GB RAM and a Graphic card NVIDIA K80 with 2 GPUs and 24 GB video RAM (Nvidia, Santa Clara, CA USA). The algorithm was developed by using the Jupyter notebook (Kluyver et al., 2016) and Python 3.6.8 (van Rossum and Drake, 2014).
The body posture model located and classified the ‘standing’ and ‘lying’ body posture while the individual animal identification model located and classified the individual heifer. Per image, the detected body postures were assigned to the corresponding heifer using the center coordinates of the bounding boxes of both models. For each bounding box of the individual animal identification model, the Euclidean distances from its center point to all center points of the bounding boxes of the body posture model were calculated. The bounding box of the body posture model with the smallest distance to the bounding box of the individual animal identification model was combined (Equation 7).
Occasionally, duplications occurred in the detected individuals. In these cases, the detection with the highest accuracy was selected. The model only considered detections that were determined in the individual animal identification model and the body posture model. Detections that were only determined in one of the two models are not included in the individual posture model. By combining both models, the individual posture model was developed.
Three short videos per hour from day 2 and day 3, with an offset of 20 minutes, at approximately hh:00, hh:20 and hh:40, were used for the manual analysis. Three short videos with a length of 117 seconds resulted in an observation time of 00:05:51 per hour and pen 2. This corresponds to a total video duration of 04:40:48 (02:20:24 per day) for these two observation days. Consequently, 144 videos were analyzed, and the ‘standing’ and ‘lying’ times of each heifer were noted for each short video. 288 values (144 ‘standing’ values, 144 ‘lying’ values) per heifer were determined in the 48 hours under consideration. During the manual analysis, it was possible to consistently identify all five heifers and their body posture in the short videos. The determined ‘standing’ and ‘lying’ times for day 2 and day 3 were cumulated separately per hour and heifer. The determined times set the gold standard and are used as a basis for the following validation of the individual posture model.
The individual posture model, was applied to the same 144 short videos. The algorithm extracted one frame every full second from the short videos, so that 117 images were analyzed per short video. The individual posture model located and identified the heifers and classified their ‘standing’ and ‘lying’ posture individually for each image. The standing and lying times determined with the individual posture model were cumulated separately for day 2 and day 3 per hour and heifer, too.
To validate the individual posture model the ‘standing’ and ‘lying’ times of these both determination methods were compared for the two days.
For the automatic determination of ‘standing’ and ‘lying’ times, the validated individual posture model was applied to all videos of the further four days (day 1, day 2, day 3, day 4). The algorithm was applied in the same way as for validation. The values determined were cumulated per hour and heifer.
After training the YOLOv4 neural network and subsequent validation, the performance for the ‘standing’ and ‘lying’ classes of the posture model was as follows. Of the 409 ‘standing’ labels in the test set, 401 TP and 8 FN were detected. Two labels of ‘standing’ heifers were detected FP. This resulted in a precision of 99.50% and a recall of 98.04%. The AP for ‘standing’ was 99.03%, and the average IoU was 0.90. For the class ‘lying’, the precision of 97.54%, recall of 98.61%, and average IoU of 0.86 were slightly lower. The AP of 99.26%, on the other hand, was slightly higher. A total of 426 TP, 10 FP and 6 FN were detected from the 432 ‘lying’ labels.
The overall performance of the posture model was characterized by a precision of 98.54%, a recall of 98.34%, and a mAP of 99.21%. The average IoU was 0.88. The detection speed was 71 ms per image.
YOLOv4 was also trained and validated as an individual model. After validation, the classes ‘heifer_1’ and ‘heifer_3’ achieved the highest precision with 99.56%, while the class ‘heifer_5’ had the lowest with 88.89%. The precision for heifer_2 with 95.12% and for heifer_4 with 94.44% were in between. A recall of 100% was achieved by ‘heifer_1’, ‘heifer_3’ and ‘heifer_5’, while for the other two classes it was 97.50% (‘heifer_2’) and 97.14% (‘heifer_4’). There was a total of 11 FP detections: once for ‘heifer_1’ and ‘heifer_3’, twice for ‘heifer_2’ and ‘heifer_4’, and five times for ‘heifer_5’. Two detections were FN, one for ‘heifer_2’ and one for ‘heifer_4’ The remaining labels were all detected (Figure 2).
The overall performance of the individual animal identification model achieved a precision of 94.61%, a recall of 98.97%, and a mAP of 99.79%. The average IoU was 0.83 and the detection speed was 71 ms per image.
The body posture model detected 1,593,070 postures in the four observation days in all videos. The individual animal identification model detected in the same period 1,692,867 individuals. When merging the two models, only the detections that were detected by both models were considered. In each case, the individual heifer and its posture had to be detected. If only one of the two models returned a result, this detection was not considered in the individual posture model. So, the number of detections was reduced. In total, the body posture was assigned to the corresponding heifer 1,480,478 times during the observation period of four days. This corresponds to an average deviation of 7.07% for the body posture model and 12.55% for the individual animal identification model.
The results of the manual determination and the determination using the individual posture model recognized the increase in ‘standing’ time in the morning hours as well as continuous ‘standing’ in the period from 8 a.m. on day 2 or 9 a.m. on day 3 to 12 p.m. (noon). Both methods also recognized that the heifers lay more in the morning hours and also in the evening from 7 p.m. to 11 p.m. with the peak at night. Comparing the two days, the increased ‘standing’ time on day 3 was detected by both methods. When comparing both methods, it is noticeable that detections are missing from time to time in the individual posture model. Since not all animals on every image were recognized by the individual posture model, there are small time differences in the automated method. On day 2 it was particularly noticeable in ‘heifer_2’ at 3 a.m. and in ‘heifer_5’ between 8 a.m. and 11 a.m. and between 4 p.m. and 9 p.m. (Supplementary Figure S2). This resulted in a time difference between the two methods. ‘Heifer_2’ was 00:14:15 less in the ‘lying’ posture and ‘heifer_5’ was 00:20:16 less in the ‘standing’ posture (Table 6). On day 3, there were more missing detections in ‘heifer_1’ between 0 a.m. (midnight) and 2 a.m., from 11 a.m. to 12 p.m. (noon) and from 8 p.m. to 11 p.m. In the period from 8 a.m. to 10 a.m. there were many missing detections for ‘heifer_2’ and ‘heifer_3’ (Supplementary Figure S3). Missing detections also occurred sporadically in the other heifers. ‘Heifer_1’ was detected 00:27:32 too less in the ‘lying’ position. In the ‘standing’ position, 00:17:35 was missing for ‘heifer_3’ and 00:22:16 for ‘heifer_4’ (Table 6). On average, the time difference for the observation period was 00:16:49 per day, which corresponds to about 11.98% of the daily observation time.
Table 6. ‘Standing’ and ‘lying’ times of each heifer and the herd-based average for day 2 and day 3. Determined by the manual and the automated method.
The model was applied to the videos of four consecutive days. It gave an overview of the ‘standing’ and ‘lying’ time per hour and heifer for each day (Supplementary Figures S4–S7). The average ‘standing’ time per day was approximately between 10.5 and 13 hours (Table 7). This corresponds to about 50% to 60% of the automated determined time per day using the individual posture model.
Table 7. Automatically determined daily “standing” and “lying” times per heifer and herd-based average of all four observation days using the individual posture model.
With the exception of day 2, ‘heifer_4’ stood for more than 88% of the time. The ‘lying’ times were generally short and spread over the day. In rare cases, ‘heifer_4’ lay for a full hour, for example on day 1 from 17:00:00 to 17:59:59.
Two models, the individual animal identification model and the body posture model, were trained in this study with YOLOv4. Bochkovskiy et al. (2020) showed that YOLOv4 is a fast and accurate object detection model. Similarly, in this study both models were characterized by high accuracy. The recall and IoU values were also high. False classifications rarely occurred. With a detection speed of 71 ms per frame, the models can be used for real-time detection in video streams with a frame rate of up to 15 frames per second.
Our individual animal identification model differentiated the heifers by their coat pattern. Heifers could be recognized if they were partially covered by other heifers, as long as typical coat patterns of the corresponding heifer were still visible. The mAP was 99.79%. Compared to other studies, the detection rate of the individual animal identification model is high in this study. Yilmaz et al. (2021) were able to detect and classify cattle and their breed with a precision of 92.85% using YOLOv4. In another study, an accuracy of 93.66% was achieved for automatic cattle identification (Dulal et al., 2022). Zin et al. (2020) used the numbers of the ear tags for the individual detection by camera and object detection in his study and reached a mAP of 92.5%. Instead of ear tags, Dac et al. (2022) used facial recognition to identify individual heifers and achieved a precision of 84%. Shen et al. (2020) achieved 96.65% accuracy by recognizing the side view of the cows based on fine-tuning by segmenting the image into head, trunk and legs. The comparative studies show different approaches to individual animal identification. The different accuracies can therefore be due to various reasons, such as covering, different lighting conditions, soiled animals or ear tags. Dac et al. (2022), for example, did not take coverings into account in its training material, so that animals whose faces were partially or completely covered were not detected at all. This may explain the lower accuracy. Therefore, the image material for model training should contain different types of coverings. For a model that is to identify under different lighting conditions, these should also be present in the image material, for example day and night shots. In general, when compiling the image material for the training, care should be taken to ensure that all possibilities (coverings, day and night shots, soiling, etc.) are included. This is because the more variable the image material is compiled for training, the more robust and accurate the trained model will be.
In this study, only five heifers were identified on the basis of their coat pattern. Identifying all heifers or cows in a larger herd in the same way requires a high labeling effort. If several cows or heifers are also similar (several black cows or similar coat patterns, etc.), the accuracy of the individual animal identification model may be reduced as false identifications may occur more frequently.
The body posture model works on a herd-basis with a mAP of 99.21% (‘lying’ AP = 98.86%, ‘standing’ AP = 99.65%). Compared to other studies, the newly trained model performs very well. Nasirahmadi et al. (2019) were able to achieve ‘lying’ and ‘standing’ postures in pigs with accuracies of 93% on average for ‘lying’ and 95% for ‘standing’ pigs, whereby this was the best result from three different detection methods. ‘Standing’ laying hens could be detected with an accuracy of 94.57% using YOLOv3 (Wang et al., 2020). In an experiment with foxes, Schütz et al. (2022) chose the three object properties ‘sitting’, ‘lying’ and ‘standing’. They achieved a high AP of 99.97% for sitting, 99.79% for lying and 99.96% for standing. While in the model used here the object properties of five animals were recorded simultaneously within a stable box, the study on foxes only had one fox per cage. It was possible to exclude blind spots when observing the foxes individually, as they were observed simultaneously with two cameras instead of one camera as in the experiment in the present study. Furthermore, the heifers in this experiment could partially or completely cover each other, making detection difficult or even impossible. Nevertheless, the model was able to correctly recognize only partially visible animals and their object characteristics.
By combining the individual animal identification model with the body posture model, it was possible to determine the ‘standing’ and ‘lying’ time of the heifers individually for each animal. The assignment of individual heifers and their body posture led to a reduction in the joint detections of both detectors. If only the heifer was detected and not its body posture or vice versa, the information was lost in this case. However, these cases were rare.
Watching the videos, 3 per hour at 20-minute intervals, established a basis for comparison. By analyzing the short clips, animal observation by human staff was simulated in this way. In the manual determination, a total of 02:20:24, or about 10% of a day, was covered by manual animal observation. ‘Standing’ and ‘lying’ times outside the observation periods were not included. There are also no rules on the duration and number of individual observation sequences for conducting visual animal observations. For example, Borchers et al. (2016) carried out their observations for two hours in the morning and two hours in the evening after milking. Mayo et al. (2019) did things differently. They observed their animals four times, for 30 minutes each, throughout the day. In contrast, the individual posture model is able to continuously observe the animals in real-time and can determine ‘standing’ and ‘lying’ times 24 hours a day. Even if the individual posture model has gaps, it can capture more ‘standing’ and ‘lying’ times over the course of the entire day than visual observation of animals by staff in the practice could. The possibility of continuous monitoring means that heifers with unusually long standing or lying times can be better identified. Deviations from the usual standing and lying times can also be detected more quickly and action can be taken if necessary.
With the individual animal identification model, it is possible to identify differences in ‘standing’ and ‘lying’ times between individual animals. Changes in individual animals can also be recognized.
The differences in the ‘standing’ and ‘lying’ times when comparing the two methods can be explained by the type of time recording. Although there were deviations from the manual method, these were small with an average of 25 seconds for ‘standing’ and 17 seconds for ‘lying’ per heifer and three videos per hour (00:05:51) compared to the manual method. There are various reasons for this. Firstly, there are differences in the image classification. As a result, the change from the ‘standing’ to the ‘lying’ position and vice versa may not be detected consistently This is a moving posture that cannot be clearly categorized despite its definition. It can therefore lead to a bias and missing detections. Small differences in classification are possible, especially when changing posture. Secondly, a heifer, especially in the ‘lying’ position, can be covered by another and is therefore not detected by the model. Thirdly, monitoring the heifers with only one camera creates blind spots that cannot be detected. It can also be possible for one heifer to be covered by another. In these two cases too, the problem can be solved by installing additional cameras with a different angle of view. Compared to the time needed to view each individual video, the deviation is tolerable. The resulting differences in ‘standing’ and ‘lying’ times can be attributed to the short sequences of observation periods of the manual method, in which the change between ‘standing’ and ‘lying’ positions could not be determined.
The individual animal identification model is able to monitor ‘standing’ and ‘lying’ posture in real time. With continuous 24-hour monitoring, it is possible to recognize deviations in the ‘standing’ and ‘lying’ times of the animals that occur on different days. The combination of both trained YOLOv4 networks can be used to identify the ‘standing’ and ‘lying’ posture of individual heifers.
In this study, the focus was on determining the individual standing and lying times of heifers. Possible additions to the model would be the counting of standing and lying periods or the inclusion of the activity of the individual animals. For this, the videos would have to be viewed again and the changes between postures as well as the activity behavior would have to be documented manually in order to obtain a gold standard for the respective validation.
The model can also be extended by adding further behaviors. To do this, relevant classes must be defined and additional images must be labeled and classified. These properties can be integrated into the model through further training with new image material. Drinking and eating would be conceivable. The automated recording of eating and drinking behavior, including the duration and number of periods, can provide additional information on the health and welfare of the heifers. However, it is also conceivable that heifers could be mounted. The model could then also be used for heat detection. An extension of the model offers the possibility of obtaining even more information about the heifers and improving the early detection of changes. Decisions to intervene in the event of changes could be made more quickly as a result.
In this study, a model for the automated detection of Holstein Frisian cattle and their individual body posture (‘standing’, ‘lying’) was developed. After image extraction from video data, two models, the posture model and the individual animal identification model, were trained and evaluated with the object detection algorithm YOLOv4. With a mAP of 99.21% for the posture model and a mAP of 99.79% for the individual model, both models achieved a high level of accuracy. Both models were combined and can be used on individual base.
The ‘standing’ and ‘lying’ times of two days were measured manually and automatically in one group of five heifers individually. The automatic method was evaluated by comparing the collected times. The time differences between the two methods were small. They averaged 00:16:49 per day during the daily observation period. This corresponds to 11.98%. Thus, the automated method realizes real-time monitoring of the ‘standing’ and ‘lying’ times of heifers or heifers at individual level in groups. By using such a model in practice, the working time for visual animal observations by staff can be reduced.
The data analyzed in this study is subject to the following licenses/restrictions: As people can be seen in the videos, the videos cannot be made publicly available due to data protection regulations. Requests to access these datasets should be directed to U2FyYWguSmFobkBmbGkuZGU= or VGltby5Ib21laWVyQGZsaS5kZQ==.
The animal study was approved by Landesamt für Landwirtschaft, Lebensmittelsicherheit und Fischerei (LALLF) Mecklenburg-Vorpommern. The study was conducted in accordance with the local legislation and institutional requirements.
SJ: Data curation, Formal analysis, Methodology, Software, Validation, Visualization, Writing – original draft, Writing – review & editing. GS: Formal analysis, Validation, Writing – review & editing. LB: Project administration, Writing – review & editing. HL: Supervision, Writing – review & editing. TH-B: Conceptualization, Methodology, Project administration, Resources, Supervision, Writing – review & editing. AS: Data curation, Formal analysis, Investigation, Methodology, Software, Supervision, Validation, Writing – review & editing.
The author(s) declare financial support was received for the research, authorship, and/or publication of this article. This study is part of the project “Innovations for healthy and ‘happy’ cows” (IGG). The project is funded by the German Federal Ministry of Food and Agriculture (BMEL) based on a decision by the Parliament of the Federal Republic of Germany, granted by the Federal Office for Agriculture and Food (BLE; grant number: 28N303901).
We thank the animal attendants of the Friedrich-Loeffler-Institut for taking care of the heifers and providing further help in organizing the experiments. We would also like to thank Hülya Öz for her helpful comments during the final writing process.
The authors declare that the research was conducted in the absence of any commercial or financial relationships that could be construed as a potential conflict of interest.
All claims expressed in this article are solely those of the authors and do not necessarily represent those of their affiliated organizations, or those of the publisher, the editors and the reviewers. Any product that may be evaluated in this article, or claim that may be made by its manufacturer, is not guaranteed or endorsed by the publisher.
The Supplementary Material for this article can be found online at: https://www.frontiersin.org/articles/10.3389/fanim.2024.1499253/full#supplementary-material
Supplementary Figure 1 | Example of the coat pattern of the five heifer individuals.
Supplementary Figure 2 | Day 2 - Comparison of the ‘standing’ and ‘lying’ times of each heifer determined with the manual and automated method. Three videos result in an observation time of 00:05:51 per hour and heifer.
Supplementary Figure 3 | Day 3 - Comparison of the ‘standing’ and ‘lying’ times of each heifer determined with the manual and automated method. Three videos result in an observation time of 00:05:51 per hour and heifer.
Supplementary Figure 4 | Determined ‘staying’ and ‘lying’ times per hour with the individual posture model on day 1.
Supplementary Figure 5 | Determined ‘staying’ and ‘lying’ times per hour with the individual posture model on day 2.
Supplementary Figure 6 | Determined ‘staying’ and ‘lying’ times per hour with the individual posture model on day 3.
Supplementary Figure 7 | Determined ‘staying’ and ‘lying’ times per hour with the individual posture model on day 4.
AP, average precision; FN, false negative; FP, false positive; IoU, Intersection over Union; mAP, mean average precision; TP, true positive.
Andrew W., Gao J., Mullan S., Campbell N., Dowsey A. W., Burghardt T. (2021). Visual identification of individual holstein-friesian cattle via deep metric learning. Comput. And Electron. In Agric. 185, 106133. doi: 10.1016/J.Compag.2021.106133
Bochkovskiy A., Wang C.-Y., Liao H.-Y. M. (2020). Yolov4: optimal speed and accuracy of object detection. Arxiv. doi: 10.48550/arXiv.2004.10934
Borchers M. R., Chang Y. M., Tsai I. C., Wadsworth B. A., Bewley J. M. (2016). A validation of technologies monitoring dairy cow feeding, ruminating, and lying behaviors. J. Dairy Sci. 99, 7458–7466. doi: 10.3168/Jds.2015-10843
Cangar Ö., Leroy T., Guarino M., Vranken E., Fallon R., Lenehan J., et al. (2008). Automatic real-time monitoring of locomotion and posture behaviour of pregnant cows prior to calving using online image analysis. Comput. And Electron. In Agric. 64, 53–60. doi: 10.1016/J.Compag.2008.05.014
Chen Z., Wu R., Lin Y., Li C., Chen S., Yuan Z., et al. (2022). Plant disease recognition model based on improved yolov5. Agronomy 12, 365. doi: 10.3390/Agronomy12020365
Dac H. H., Gonzalez Viejo C., Lipovetzky N., Tongson E., Dunshea F. R., Fuentes S. (2022). Livestock identification using deep learning for traceability. Sensors (Basel) 22. doi: 10.3390/S22218256
Dittrich I., Gertz M., Krieter J. (2019). Alterations in sick dairy cows’ Daily behavioural patterns. Heliyon 5, E02902. doi: 10.1016/J.Heliyon.2019.E02902
Dulal R., Zheng L., Kabir M. A., Mcgrath S., Medway J., Swain D., et al. (2022). “Automatic cattle identification using yolov5 and mosaic augmentation: A comparative analysis,” in 2022 International Conference On Digital Image Computing: Techniques And Applications (Dicta). 1–8 (Sydney, Australia: IEEE).
Everingham M., Van Gool L., Williams C. K. I., Winn J., Zisserman A. (2010). The pascal visual object classes (Voc) challenge. Int. J. Comput. Vis. 88, 303–338. doi: 10.1007/S11263-009-0275-4
Fogsgaard K. K., Bennedsgaard T. W., Herskin M. S. (2015). Behavioral changes in freestall-housed dairy cows with naturally occurring clinical mastitis. J. Dairy Sci. 98, 1730–1738. doi: 10.3168/Jds.2014-8347
Fregonesi J. A., Leaver J. (2001). Behaviour, performance and health indicators of welfare for dairy cows housed in strawyard or cubicle systems. Livestock Production Sci. 68, 205–216. doi: 10.1016/S0301-6226(00)00234-7
Hänske J., Heller M., Schnee C., Weldearegay Y. B., Franzke K., Jores J., et al. (2023). A new duplex qpcr-based method to quantify mycoplasma mycoides in complex cell culture systems and host tissues. J. Microbiol. Methods 211, 106765. doi: 10.1016/J.Mimet.2023.106765
Helwatkar A., Riordan D., Walsh J. (2014). Sensor technology for animal health monitoring. Int. J. On Smart Sens. And Intelligent Syst. 7, 1–6. doi: 10.21307/Ijssis-2019-057
Hofstra G., Roelofs J., Rutter S. M., Van Erp-Van Der Kooij E., And Vlieg J. (2022). Mapping welfare: location determining techniques and their potential for managing cattle welfare—A review. Dairy 3, 776–788. doi: 10.3390/Dairy3040053
Johnson R. W. (2002). The concept of sickness behavior: A brief chronological account of four key discoveries. Vet. Immunol. Immunopathol. 87, 443–450. doi: 10.1016/S0165-2427(02)00069-7
Kluyver T., Ragan-Kelley B., Pérez F., Granger B., Bussonnier M., Frederic J., et al. (2016). Jupyter notebooks - A publishing format for reproducible computational workflows (Positioning and Power in Academic Publishing: Players, Agents and Agendas: Stand Alone), 87–90.
Li G., Huang Y., Chen Z., Chesser G. D., Purswell J. L., Linhoss J., et al. (2021). Practices and applications of convolutional neural network-based computer vision systems in animal farming: A review. Sensors (Basel) 21. doi: 10.3390/S21041492
Maselyne J., Pastell M., Thomsen P. T., Thorup V. M., Hänninen L., Vangeyte J., et al. (2017). Daily lying time, motion index and step frequency in dairy cows change throughout lactation. Res. Vet. Sci. 110, 1–3. doi: 10.1016/J.Rvsc.2016.10.003
Mayo L. M., Silvia W. J., Ray D. L., Jones B. W., Stone A. E., Tsai I. C., et al. (2019). Automated estrous detection using multiple commercial precision dairy monitoring technologies in synchronized dairy cows. J. Dairy Sci. 102, 2645–2656. doi: 10.3168/Jds.2018-14738
Mcdonagh J., Tzimiropoulos G., Slinger K. R., Huggett Z. J., Down P. M., Bell M. J. (2021). Detecting dairy cow behavior using vision technology. Agriculture 11, 675. doi: 10.3390/Agriculture11070675
Medrano-Galarza C., Gibbons J., Wagner S., De Passillé A. M., Rushen J. (2012). Behavioral changes in dairy cows with mastitis. J. Dairy Sci. 95, 6994–7002. doi: 10.3168/Jds.2011-5247
Munksgaard L., Jensen M. B., Pedersen L. J., Hansen S. W., And Matthews L. (2005). Quantifying behavioural priorities—Effects of time constraints on behaviour of dairy cows, bos taurus. Appl. Anim. Behav. Sci. 92, 3–14. doi: 10.1016/J.Applanim.2004.11.005
Nasirahmadi A., Edwards S. A., And Sturm B. (2017). Implementation of machine vision for detecting behaviour of cattle and pigs. Livestock Sci. 202, 25–38. doi: 10.1016/J.Livsci.2017.05.014
Nasirahmadi A., Sturm B., Edwards S., Jeppsson K.-H., Olsson A.-C., Müller S., et al. (2019). Deep learning and machine vision approaches for posture detection of individual pigs. Sensors (Basel) 19. doi: 10.3390/S19173738
Redmon J., Divvala S., Girshick R., And Farhadi A. (2016). “You only look once: unified, real-time object detection,” in 2016 Ieee Conference On Computer Vision And Pattern Recognition (Cvpr). 779–788 (IEEE).
Rutten C. J., Velthuis A. G. J., Steeneveld W., Hogeveen H. (2013). Invited review: sensors to support health management on dairy farms. J. Dairy Sci. 96, 1928–1952. doi: 10.3168/Jds.2012-6107
Schirmann K., Chapinal N., Weary D. M., Heuwieser W., Von Keyserlingk M. A. G. (2012). Rumination and its relationship to feeding and lying behavior in holstein dairy cows. J. Dairy Sci. 95, 3212–3217. doi: 10.3168/Jds.2011-4741
Schütz A. K., Krause E. T., Fischer M., Müller T., Freuling C. M., Conraths F. J., et al. (2022). Computer vision for detection of body posture and behavior of red foxes. Anim. (Basel) 12. doi: 10.3390/Ani12030233
Schütz A. K., Schöler V., Krause E. T., Fischer M., Müller T., Freuling C. M., et al. (2021). Application of yolov4 for detection and motion monitoring of red foxes. Anim. (Basel) 11. doi: 10.3390/Ani11061723
Sharma B., Koundal D. (2018). Cattle health monitoring system using wireless sensor network: A survey from innovation perspective. Iet Wirel. Sens. Syst. 8, 143–151. doi: 10.1049/Iet-Wss.2017.0060
Shen W., Hu H., Dai B., Wei X., Sun J., Jiang L., et al. (2020). Individual identification of dairy cows based on convolutional neural networks. Multimed Tools Appl. 79, 14711–14724. doi: 10.1007/S11042-019-7344-7
Tizard I. (2008). Sickness behavior, its mechanisms and significance. Anim. Health Res. Rev. 9, 87–99. doi: 10.1017/S1466252308001448
Tucker C. B., Jensen M. B., Passillé A. M., Hänninen L., And Rushen J. (2021). Invited review: lying time and the welfare of dairy cows. J. Dairy Sci. 104, 20–46. doi: 10.3168/Jds.2019-18074
Tzutalin (2015). Labelimg: git code. Available online at: https://Github.Com/Tzutalin/Labelimg (Accessed September 29, 2023).
van Rossum G., Drake F. L. Jr. (2014). The python language reference (Wilmington, De, Usa: Python Software Foundation).
Wang J., Wang N., Li L., Ren Z. (2020). Real-time behavior detection and judgment of egg breeders based on yolo V3. Neural Comput. Applic 32, 5471–5481. doi: 10.1007/S00521-019-04645-4
Weary D. M., Huzzey J. M., Von Keyserlingk M. A. G. (2009). Board-invited review: using behavior to predict and identify ill health in animals. J. Anim. Sci. 87, 770–777. doi: 10.2527/Jas.2008-1297
Westin R., Vaughan A., De Passillé A. M., Devries T. J., Pajor E. A., Pellerin D., et al. (2016). Lying times of lactating cows on dairy farms with automatic milking systems and the relation to lameness, leg lesions, and body condition score. J. Dairy Sci. 99, 551–561. doi: 10.3168/Jds.2015-9737
Yilmaz A., Nur Uzun G., Zahid Gurbuz M., Kivrak O. (2021). “Detection and breed classification of cattle using yolo V4 algorithm,” in 2021 International Conference On Innovations In Intelligent Systems And Applications (Inista). 1–4 (IEEE).
Zhang Y., Zhang Q., Zhang L., Li J., Li M., Liu Y., et al. (2023). Progress of machine vision technologies in intelligent dairy farming. Appl. Sci. 13, 7052. doi: 10.3390/App13127052
Zheng Z., Li J., Qin L. (2023). Yolo-byte: an efficient multi-object tracking algorithm for automatic monitoring of dairy cows. Comput. And Electron. In Agric. 209, 107857. doi: 10.1016/J.Compag.2023.107857
Keywords: animal behavior, computer vision, YOLO(v4), heifer, individual detection, Holstein Friesian
Citation: Jahn S, Schmidt G, Bachmann L, Louton H, Homeier-Bachmann T and Schütz AK (2025) Individual behavior tracking of heifers by using object detection algorithm YOLOv4. Front. Anim. Sci. 5:1499253. doi: 10.3389/fanim.2024.1499253
Received: 20 September 2024; Accepted: 10 December 2024;
Published: 03 January 2025.
Edited by:
Oleksiy Guzhva, Swedish University of Agricultural Sciences, SwedenReviewed by:
Daniella Moura, State University of Campinas, BrazilCopyright © 2025 Jahn, Schmidt, Bachmann, Louton, Homeier-Bachmann and Schütz. This is an open-access article distributed under the terms of the Creative Commons Attribution License (CC BY). The use, distribution or reproduction in other forums is permitted, provided the original author(s) and the copyright owner(s) are credited and that the original publication in this journal is cited, in accordance with accepted academic practice. No use, distribution or reproduction is permitted which does not comply with these terms.
*Correspondence: Sarah Jahn, U2FyYWguSmFobkBmbGkuZGU=; Timo Homeier-Bachmann, VGltby5Ib21laWVyQGZsaS5kZQ==
†These authors share senior authorship
Disclaimer: All claims expressed in this article are solely those of the authors and do not necessarily represent those of their affiliated organizations, or those of the publisher, the editors and the reviewers. Any product that may be evaluated in this article or claim that may be made by its manufacturer is not guaranteed or endorsed by the publisher.
Research integrity at Frontiers
Learn more about the work of our research integrity team to safeguard the quality of each article we publish.