- 1Dept. Biosystems and Technology, Swedish University of Agricultural Sciences (SLU), Alnarp, Sweden
- 2Dept. Animal Environment and Health, Swedish University of Agricultural Sciences (SLU), Skara, Sweden
- 3Dept. Clinical Sciences, Swedish University of Agricultural Sciences (SLU), Uppsala, Sweden
- 4Dept. Biomedical Science and Veterinary Public Health, Swedish University of Agricultural Sciences (SLU), Uppsala, Sweden
The aim of this study was to investigate how the activity of cattle under natural grazing conditions is related to their individual parasite contribution (IPC). Potentially, the individuals contributing the most to the contamination of the pasture with gastrointestinal nematodes (GIN) could then be identified and selectively treated based on sensor data thresholds. A total of 58 steers of the dairy breeds Swedish Red (SR, n = 19) and Swedish Holstein (SH, n = 39) were used for sensor-based data collection that lasted for a total of 10 weeks from 4 May to 13 July 2022. All steers were inoculated with a priming dose of nematode larvae and weighed in conjunction with the pasture release. The animals were then divided into four experimental groups and treated with ivermectin (IVM PO, Boehringer Ingelheim, 0.5 mg/kg BW) at different intervals to obtain an exposure contrast (Group A was left untreated, Group B/IVM PO-4w was treated after 4 weeks, Group C/IVM PO-8w was treated after 8 weeks, and Group D/IVM PO-4&8w was treated after 4 weeks and then after 8 weeks). The steers were weighed on four further occasions, during which faecal samples were also taken for parasite testing. Activity data were collected using leg-mounted IceQube sensors and body weight data and faecal samples were collected bi-weekly. The new threshold metric (IPC) was proposed for individuals with different faecal egg count (FEC) levels [NO (no contribution) <20 EPG, LO (low contribution) = 20–150 EPG, ME (medium contribution) = 150–250 EPG, and HI (high contribution) ≥250 EPG] The effects of IPC on activity patterns were analysed stepwise using a Generalised Estimating Equations model implemented in Python programming language. Results showed significant effects of different IPC values on motion index and number of steps taken (adjusted p-value of 0.008, 0.018, 0.041, and 0.001 for individuals with NO, LO, ME, and HI IPC values, respectively). There were some breed effects on the average number of steps and minutes spent lying. The results also provide alternative threshold methods aimed at finding more sustainable ways of using anthelmintics and integrating individual data into future parasite control strategies.
1 Introduction
Infections with gastrointestinal nematodes (GIN) are among the most common and most debilitating production-limiting diseases in grazing animals worldwide (Vande Velde et al., 2018; Charlier et al., 2020a). In temperate climates, Ostertagia ostertagi and Cooperia oncophora are the main causes of subclinical production losses in cattle (Charlier et al., 2020a). However, the overall intensity and thus the impact of GIN infections vary depending on the climate, management, and host age (Sabatini et al., 2023). It is therefore of great importance to predict the extent of pasture contamination and take this into account in parasite control strategies (Vineer et al., 2020). As GIN infections are recurrent in pastures, a comprehensive understanding of disease progression is required to design effective treatment plans that ideally address the needs of individual animals rather than working at a group level (McFarland et al., 2022).
To promote sustainable pasture-based production and reduce the risk of anthelmintic resistance (AR), it has been suggested that farmers should minimise the use of anthelmintics and instead implement targeted selective treatments (TSTs), where only individual animals within a group are treated based on indicators such as faecal egg count (FEC) or weight gain (Charlier et al., 2014a; Fan et al., 2022). An essential prerequisite for an effective TST and the timely use of anthelmintics is a deeper understanding of the epidemiology of GIN infections in the individual climate zones and reliable methods for their modelling are an essential prerequisite for an effective TST and the timely use of anthelmintics. However, the complexity of natural pasture management systems makes it difficult to simulate the development and progression of GIN infections on farms in different regions (McFarland et al., 2022; Filipe et al., 2023). More data are also needed on disease dynamics and progression in different genotypes when naturally exposed to localised GIN infections (Högberg et al., 2019).
Reliable modelling and diagnosis of GIN infections during the grazing season requires comparable measurements of GIN exposure levels. According to Michel (1969), FEC values are not very reliable as a single threshold for measuring infection levels as they do not reflect the individual clinical condition of the animal (Charlier et al., 2023). This is because animals develop immunity over the course of the grazing season, meaning that the correlation between FEC values and actual infection rates becomes weaker the longer animals remain on pasture (Forbes et al., 2017). However, before immunity gradually sets in, animals shedding large numbers of nematode eggs are likely to be susceptible and contribute more to pasture contamination. The development of effective prevention and TST strategies that could mitigate the potential risks of GIN infections therefore depends on the early detection of these animals (Berk et al., 2016; Sabatini et al., 2023). This emphasises the need to develop new diagnostic tools to identify such individuals and track changes in their clinical condition.
Recent advances in machine learning (ML) have led to numerous developments suitable for sensor-based diagnosis of GIN infections (Ikurior et al., 2020; Högberg et al., 2021). The continuity of data streams and the level of detail offered by modern sensors can provide meaningful insights into changes between individuals and groups that go beyond pure pathophysiology (Fan et al., 2022). Such models could contribute to a more thorough analysis of the seasonal patterns of GIN infections and simulate the effects and relationships between different levels of GIN exposure and the required treatments. However, to date, most ML models do not address the general population dynamics associated with GIN infections. Such models also rarely consider pasture management and the effects associated with host–parasite interactions (Sauermann and Leathwick, 2018). Although studies have shown variability in overall GIN susceptibility and performance levels based on genetic traits (Höglund et al., 2018), there is still a gap in scientific knowledge about the effects on host grazing behaviour. As animals with different parasite burdens differ in metabolism, this is expected to have measurable effects on overall activity and time budget (Charlier et al., 2009; Charlier et al., 2020b). Högberg et al. (2021) showed that growing cattle that were moderately exposed to GIN had an increased lying time in the first part of the grazing season, while their activity increased in the later parts compared to treated animals.
To this day, the use of longitudinal studies is a cornerstone of both clinical trials and observational research projects in various disciplines. These study designs are uniquely poised for inter-individual variance and intra-subject temporal fluctuations, and facilitate the investigation of complex biological, psychological, and behavioural dynamics (Crouchley and Davies, 1999). It should be noted, however, that the repeated measures characteristic of these studies result in temporally correlated responses. Consequently, standard statistical models normally used to interpret cross-sectional data interpretation may be inappropriate for analysing longitudinal data (Zhang et al., 2012).
The aim of this study was to investigate how sensor-based host activity data relate to the contribution to pasture parasite contamination by different individuals during the course of GIN infection in cattle under natural grazing conditions. By establishing reliable thresholds, the individuals that contribute the most can be targeted and selectively treated with an effective anthelmintic.
2 Materials and methods
The study was conducted at SLU Götala Beef and Lamb Research Centre, Skara, Sweden, in the summer of 2022 with sensor-based data collection corresponding to a total of 10 weeks from 4 May until 13 July.
2.1 Animals
Bull calves of the dairy breeds Swedish Red (SR) and Swedish Holstein (SH) were purchased from commercial herds and delivered to the SLU Götala Beef and Lamb Research Centre in autumn 2021. The bull calves were between 2.5 and 4.5 months old at the time of delivery and were castrated shortly afterwards (from here on called steers). The animals were housed in group pens and fed a mixed total ration consisting of grass/clover silage, rolled barley, and cold-pressed rapeseed cake. In addition, they received 100 g per animal and day of a vitaminised mineral supplement. The mixed feed was continuously adjusted at intervals of 76–125 kg, 126–175 kg, 176–225 kg, 225–275 kg, and 275–375 kg body weight. A total of 59 animals were selected for the study. One of the steers died suddenly on pasture within 3 days of the start of the trial; thus, 58 animals were included in the final analysis. The animals had free access to fresh water, salt, and minerals throughout the grazing period. During the first 2 weeks on pasture, they were also offered additional grass/clover silage as the availability of pasture herbage was scarce. Daily focal observations were carried out throughout the study period to ensure that all sensors were switched on and that no animal showed signs of disease or visual trauma.
2.2 Experimental groups
The study included four groups of grazing steers. Before being released to pasture, the animals were randomly assigned to specific treatment groups that were balanced for age, breed, and weight by a one-way ANOVA, with a p-value of 0.95. Each group was released into one of four enclosures representing parts of a larger grazing area.
To investigate the dynamics of GIN infections at the population level during the normal grazing season, steers were treated with ivermectin (IVM PO, Boehringer Ingelheim, 0.5 mg/kg body weight) at different intervals to obtain an exposure contrast. A detailed description of the experimental groups can be found in Table 1.
2.3 Pasture conditions
The pasture consisted of permanent semi-natural grassland, with each of the four enclosures measuring 7–10 hectares. The grassland was naturally contaminated with nematodes from the previous grazing season. The four enclosures were relatively similar to each other and consisted of approximately 20% dry, 60% mesic, and 20% wet areas. They were predominately open, but also contained small areas with deciduous trees. The predominant sward consisted mainly of Deschampsia cespitosa (tufted hairgrass), but Festuca rubra (red fescue) was also strongly represented. Pasture herbage availability was similar in the four enclosures, averaging 5.6–7.3 cm across the experimental period and 5.5–6.9 cm across the entire grazing period. The concentration of crude protein in grass samples was 115–132 g, neutral detergent fibre was 533–580 g, and metabolisable energy was 8.6–9.3 megajoule per kg of dry matter across the grazing period.
2.4 Individual activity data
One day before release to pasture, the steers were fitted with 3D accelerometers (IceQube, IceRobotics Ltd, Edinburgh, UK), which were attached to the left hind leg and secured with Velcro strap. The IceQube device had a sampling frequency of 4 Hz and a sample resolution of 15 min, providing data on the following activity states: number of steps taken, minutes standing/lying, number of lying bouts, and motion index (aggregated value including number of steps taken, lying and standing times, and transition between these two states). The internal memory of the IceQube devices allowed continuous data storage for up to 9 days, after which the data were overwritten. Data collection took place at regular weekly intervals, with the animals being moved in groups to a secluded area for visual inspection, weighing, and additional sampling if required. During the first week of the trial, the data-collection system malfunctioned, resulting in only 9 weeks of reliable data being included in the final analysis.
2.5 Parasitology and weighing
All animals were weighed twice on a digital scale before turnout to pasture and then every second week during the trial period ending with the double weighing at the end of the trial. A further double weighing was carried out for all animals at the end of the grazing season. At each weighing, rectal faecal samples were collected and analysed for FEC using a modified McMaster method with a diagnostic sensitivity of ≥20 eggs per gram. In addition, pooled larval cultures were prepared for each experimental group based on 5 g of faeces per animal at the same intervals as the individual samples. After harvesting using the Petri dish method (Elmahalawy et al., 2018), larvae were concentrated and stored in separate Eppendorf tubes. Total DNA was extracted using the Nucleospin DNA tissue kit (Macherey-Nagel) according to the manufacturer’s protocol. Finally, the proportions of Ostertagia and Cooperia DNA copies located in the internal transcribed spacer region 2 (ITS2) of the ribosomal RNA gene array were determined using a digital droplet PCR (Bio-Rad) in a discrimination assay based on a single universal primer pair and two competing hydrolysis probes. Full details can be found in Baltrušis et al. (2019).
2.6 Data curation
2.6.1 Initial data pre-processing
All data from the IceQube sensors were collected using IceReader software and then exported to csv format. Initial data cleaning and exploratory data analysis (EDA) was performed using the following libraries: pandas, seaborn, researchpy, statsmodels, and pingouin, written in the Python programming language. The data were checked and corrected for missing values and inconsistencies and aligned using timestamps so that each activity data entry had the same start/end time. The raw IceReader data were aggregated for each individual animal to achieve a weekly resolution. One of the steps in the aggregation process involved calculating additional features/characteristics such as sum, mean, min, max, median, standard deviation, variance, and mean absolute deviation for the following variables: Motion Index, Steps, Minutes standing, Minutes lying, Lying bouts, Weight, and FEC.
2.6.2 Definition of individual parasite contribution levels
The dynamic nature and complexity of GIN infections and the physiological response of individual animals to parasite infections make disease modelling difficult (Forbes, 2017). Firstly, different animals within a grazing group have different susceptibility and resilience to GIN infections. Secondly, the same animal may change its health status several times during the grazing season, and such a transition could be influenced by several independent factors. To allow an in-depth analysis and comparison of the different animals contributing to GIN pasture contamination, the animals were divided into four arbitrary categories: (1) animals with an FEC value of <20 were considered to contribute little to pasture contamination (NO), (2) animals with FEC values of 20–150 were classified as low contributors (LO), (3) animals with FEC values of 150–250 were classified as medium contributors (ME), and (4) those with FEC values >250 were classified as high contributors (HI).
2.7 Statistical analysis
The assumptions of variance homoscedasticity of normality of the data were tested by Shapiro–Wilk Bartlett/Levene tests from the pingouin package in Python and by inspection of the residual plots. Differences in activity and whether they were influenced by IPC levels and breed were analysed using the Generalised Estimating Equations (GEE) model within the statsmodels package in Python. Pairwise comparisons were examined using the Games Howell post-hoc procedure for non-normally distributed data with unequal sample sizes.
Common methods that have been adapted for longitudinal data include the GEE and the Generalised Linear Mixed-Effects Model (GLMM). While the GLMM directly models the intra-subject correlation by including random effects, the GEE developed by Liang and Zeger (1986) offers an indirect consideration of these correlations via sandwich-type variance estimates. Furthermore, the GLMM modality prescribes specific distributions for both the random effect (commonly assumed to be normal) and the sampling variability, making the inferences susceptible to deviations from these stipulated assumptions. Theoretically and practically, GEE is an attractive option for evaluating adherence data from GIN studies where varying sample size and greater individual variability could affect the robustness of potential model results/outcome. At a theoretical level, classical longitudinal regression maximises statistical power by adjusting standard errors and p-values for correlated outcomes, while additional robust estimation accounts for the features of fractional data that lead to inefficient estimates of the standard error.
To account for all potential effects of different correlation matrices and distribution parameters on the outcome of the statsmodels implementation of GEE, multigrid testing was performed using scikit-learn Python library. The model results (fitted p-values and confidence intervals) and the skewness and kurtosis of the data were examined and compared. The final GEE model had the following parameters: Poisson family to account for distribution within clusters and independent covariate structure for correlation between and within subjects.
3 Results
3.1 Faecal egg counts and intra-individual differences
The measurements from the first weeks of the experimental period (up until week 4) were excluded since the FEC values were below the egg detection limit. The FEC values (weeks 4–10) were as follows for groups A, B, C and D, respectively: 232 ± 359, 226 ± 230, 95 ± 156, and 136 ± 165. The maximum number of eggs in each group was 1,600, 1,040, 940, and 600, respectively.
There were no significant differences in FEC values between groups throughout the experimental period. As can be seen in Figure 1, large intra-group differences and low treatment efficacy were observed in the Cooperia population, which affected the effects of the intended treatments. The further individual differences between the experimental groups across all weeks on pasture using the IPC thresholds are shown in Figure 2. The differences between the two breeds in terms of susceptibility to GIN are illustrated in Figure 3 by plotting the proportion of animals at each IPC level per breed per week, starting from week 4 when the first positive FEC results were obtained.
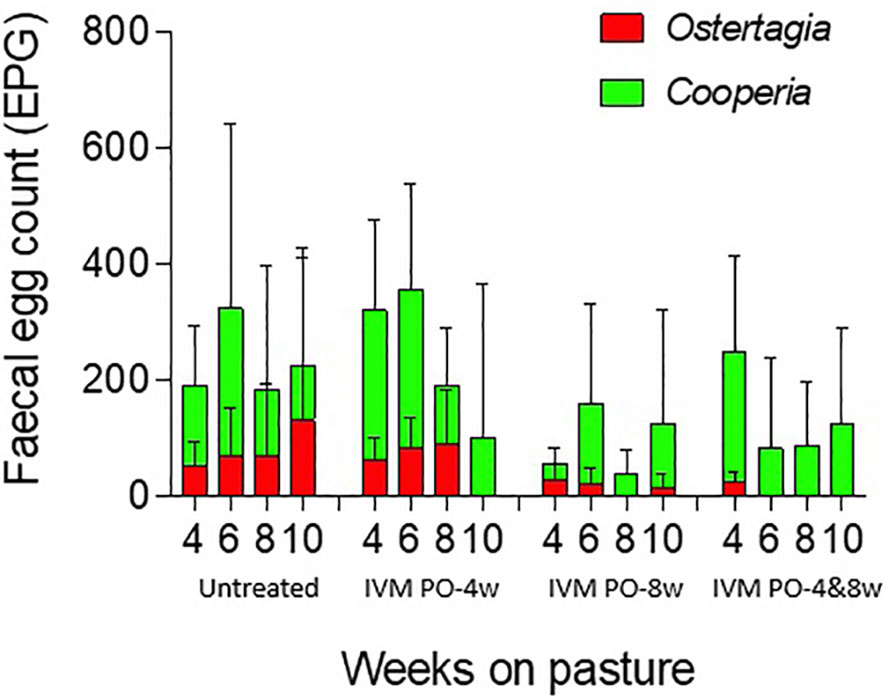
Figure 1 Weekly results of laboratory analysis of faecal samples from four experimental groups of grazing dairy steers; untreated with anthelmintics (Untreated) and treated after 4 weeks (IVM PO-4w), 8 weeks (IVM PO-8w), or both 4 and 8 weeks (IVM PO-4&8w) on pasture. Values displayed are mean eggs per gram (EPG) counts with standard deviation for Ostertagia and Cooperia populations.
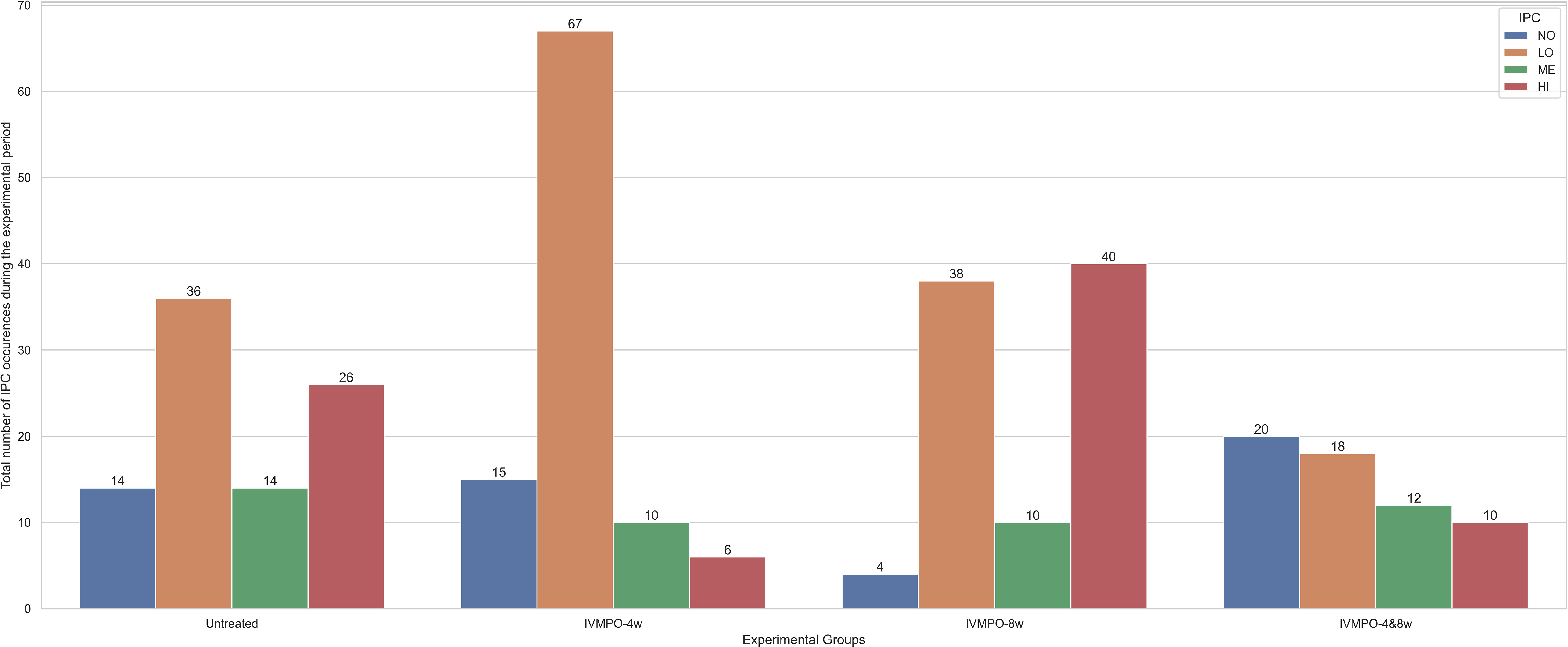
Figure 2 Total number of occurrences for different IPC levels (NO is no, LO is low, ME is medium, and HI is high contribution) across four experimental groups (Untreated, IVMPO-4w, IVMPO-8w, and IVMPO-4&8w) of grazing dairy steers through a 10-week experimental period.
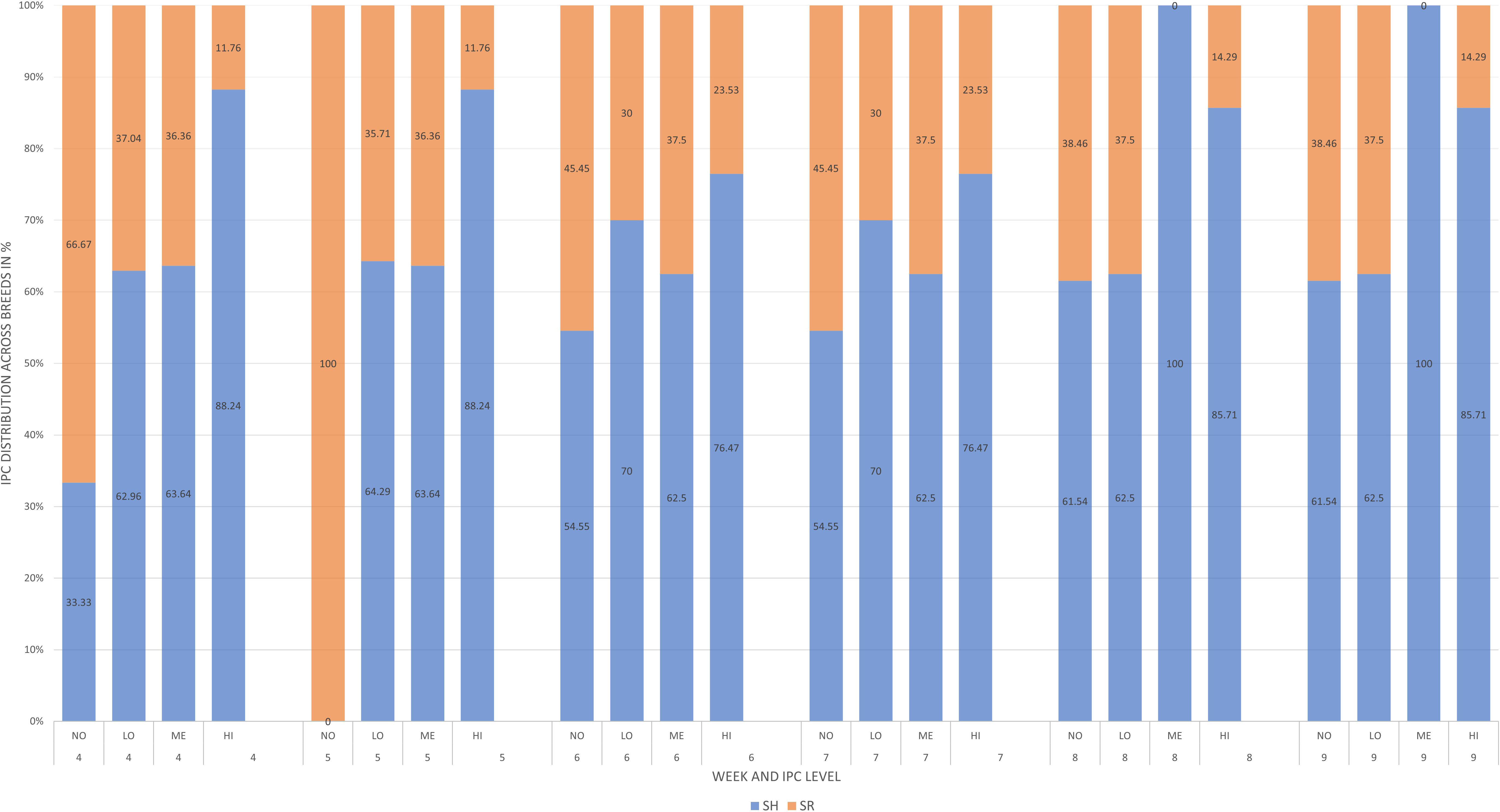
Figure 3 Weekly individual parasite contribution (IPC) distribution in % between two breeds (Swedish Holstein and Swedish Red) of grazing dairy steers through a 10-week experimental period.
3.2 Body weight changes at different individual parasite contribution levels
The IPC levels had no significant influence on the average body weight during the experimental period. However, in a pairwise weekly comparison between animals with different IPC levels, there were statistically significant differences in weeks 8 and 9 of the trial (Figure 4). The average body weight at week 8 differed between individuals with NO and LO (p = 0.006): 351 kg vs. 305 kg, and between NO and ME (p = 0.004) 351 kg vs. 286 kg. The average body weight at week 9 differed between individuals with NO and LO (p = 0.008): 365 kg vs. 321 kg, and between NO and ME (p = 0.009): 365 kg vs. 303 kg.
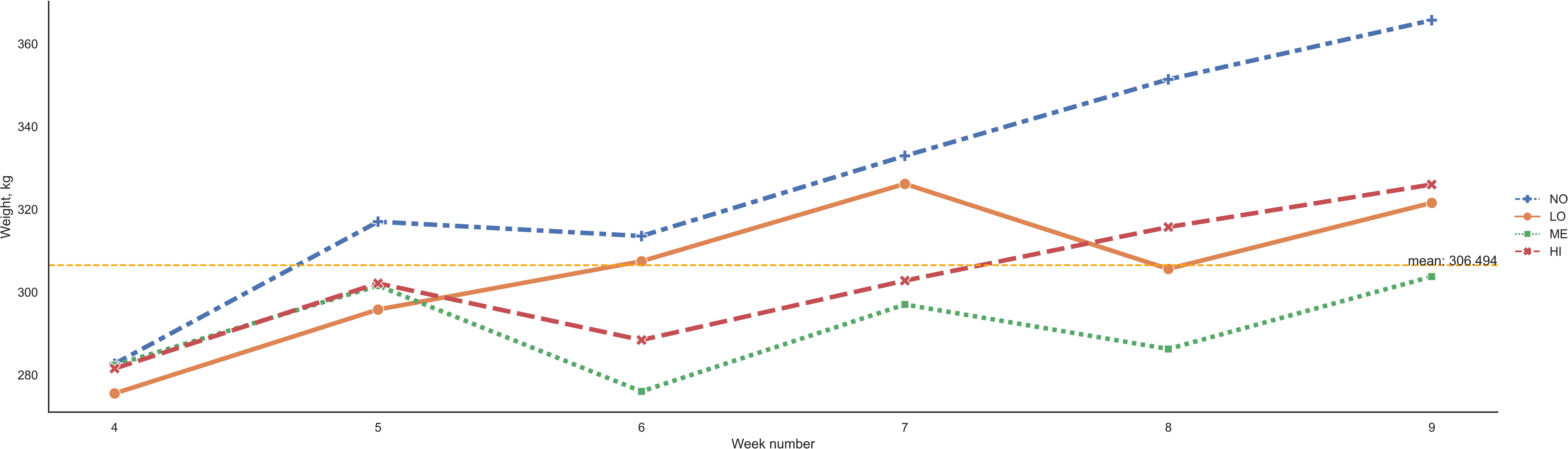
Figure 4 Weekly average body weight in kg for grazing dairy steers with different IPC levels (NO is no, LO is low, ME is medium, and HI is high contribution). The dashed line indicates the average body weight in kg for the entire 10-week experimental period.
3.3 Activity patterns in steers with different individual parasite contribution levels
The general effect of individual parasite contribution (IPC) levels on activity patterns was significant regarding motion index for the NO and LO steers and regarding number of steps for LO and ME steers (Table 2). Duration of lying and standing was not affected by the IPC levels. The total number of occurrences for each IPC level throughout the entire experimental period was as follows (note that individual steers could transition between different IPC levels as GIN infection progresses): NO = 53, LO = 159, ME = 46, and HI = 82.
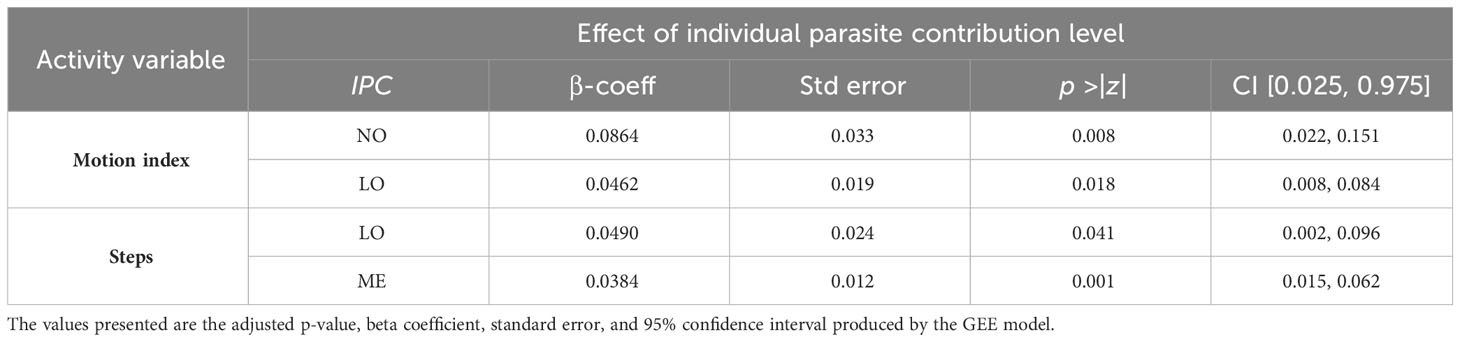
Table 2 The effect of individual parasite contribution (IPC) levels on activity patterns in grazing dairy steers (NO is no, LO is low, ME is medium, and HI is high IPC) throughout the entire experimental period.
The pairwise motion index comparison between animals at different IPC levels showed that NO animals moved significantly more (p = 0.000001) through the entire experimental period, especially in comparison to individuals at the HI IPC level: 15,845 vs. 14,160. The weekly variation in average motion index is shown in Figure 5 with the most pronounced IPC/GIN influence during weeks 4 (p = 0.001) and 5 (p = 0.01).
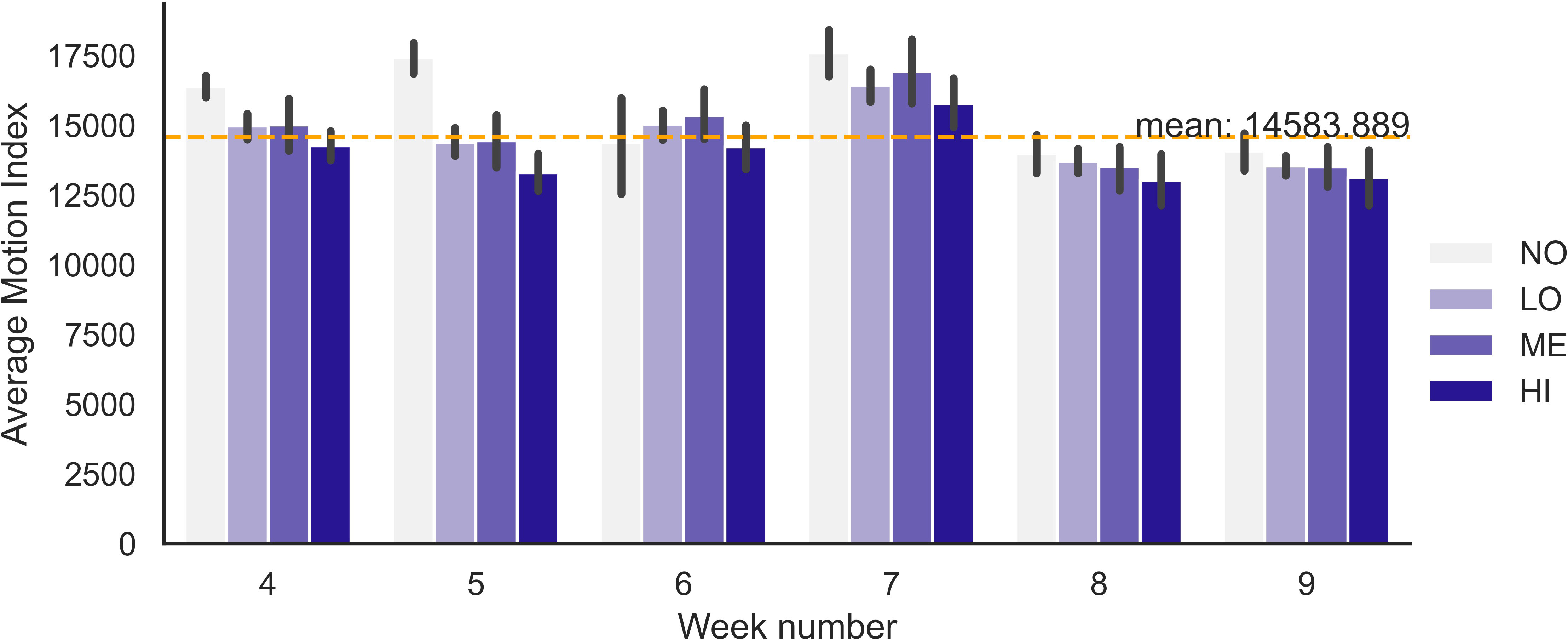
Figure 5 Weekly average motion index for grazing dairy steers with different IPC levels (NO is no, LO is low, ME is medium, and HI is high contribution). The dashed line indicates the average Motion Index across the entire 10-week experimental period.
Regarding the number of steps, the pairwise comparison between animals at different IPC levels showed that individuals at the NO IPC level walked slightly more than those at the LO level (p = 0.03, 4,116 steps vs. 3,965 steps). Weekly differences in number of steps walked were most pronounced at week 7 (p = 0.03). There was a breed effect on the number of steps (p = 0.03) with Swedish Red (SR) steers taking more steps (4,148 steps) than SH steers (3,937 steps) (Figure 6).
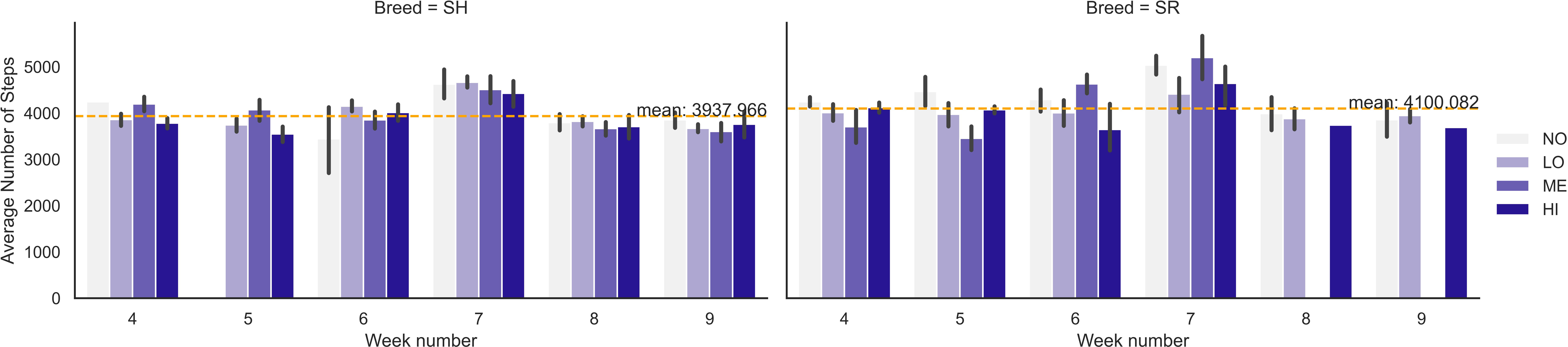
Figure 6 Weekly average number of steps taken by grazing steers of different breeds (Swedish Holstein, SH, and Swedish Red, SR) with different IPC levels (NO is no, LO is low, ME is medium, and HI is high contribution). The dashed line indicates the average number of steps taken by each breed subgroup of the total 10-week experimental period.
While there were no significant differences in terms of minutes spent lying down, a significant effect of breed (p = 0.006) was found on average and maximum weekly lying time, with individuals of SH breed averaging 683 min and a maximum of 771 min compared to individuals of the SR breed averaging 663 min and a maximum of 752 min.
4 Discussion
In this study, we embarked on a novel investigation aimed at enhancing the monitoring of GIN infections in cattle during their first grazing season. This was achieved by using activity sensors to estimate the IPC levels of each animal to pasture contamination. This method diverges from traditional static assessments of GIN infections, which typically compare individuals from distinct exposure groups. Our approach acknowledges the dynamic nature of behavioural responses to GIN exposure, a significant departure from conventional methods that often rely on FECs, which, as highlighted by Sonstegard and Gasbarre (2001) and Forbes, (2017), become overly complex and costly in commercial settings (Charlier et al., 2014b). A critical aspect of addressing the issue of early intervention involves identifying and targeting the individuals within a herd that contribute disproportionately to pasture contamination, often referred to as “supershedders” or “high shedders”. These individuals, although a minority within the population, are responsible for a significant portion of the environmental egg load, driving the transmission dynamics of GIN infections. Identifying these high-shedding individuals is pivotal in implementing TST protocols, thereby reducing the selection pressure for AR. Despite the efficacy of strategic repeated deworming, this approach bears the risk of fostering AR, a concern corroborated by findings of resistance in parasites like Cooperia oncophora to ivermectin in Sweden (Areskog et al., 2013; Areskog et al., 2014). The emerging issue of AR across Europe (Rose Vineer et al., 2020) necessitates innovative measures to mitigate these risks, prompting the consideration of targeted TST strategies. TST, which involves treating animals based on their contribution to pasture contamination and the individual risk for affected performance/health, offers a sustainable alternative to preserve the efficacy of anthelmintics while minimizing the risk of resistance development (Kenyon and Jackson, 2012; Charlier et al., 2014a). In this way, the TST helps to reduce the overall need and cost of anthelmintics, as treatment is evidence-based and needs-orientated.
The importance of devising effective strategies to protect cattle from GIN infections during their first grazing season is underscored by several Swedish trials (Dimander et al., 2003; Larsson et al., 2007; Höglund et al., 2013), which demonstrated the potential for increased production indicated by higher weight gains. While the findings from our study indicated that IPC levels did not significantly influence average body weight overall, significant weight differences were observed in weeks 8 and 9 among animals with different IPC levels. The significant weight differences based on IPC levels in later weeks of the grazing season could indicate a delayed impact of GIN infection on animal growth, warranting further investigation into the timing and duration of treatment interventions. Furthermore, it is crucial to consider the different genetic backgrounds of cattle on pasture, with breed differences potentially influencing the results of studies such as ours. Studies have shown that certain breeds, such as the African N’Dama and the European Holstein, exhibit markedly different responses to GIN challenges, attributed to genetic differences that affect their immune system’s ability to combat parasitic infections. For instance, the N’Dama cattle, adapted to harsh environments with high parasite loads, have developed a degree of natural resistance to certain GIN species, likely due to a combination of genetic traits that enhance their immune response (Baker et al., 1999). Conversely, highly productive breeds like the Holstein may be more susceptible due to selective breeding for traits unrelated to parasite resistance. Understanding these genetic underpinnings is essential for developing breeding programs aimed at enhancing GIN resistance, thereby reducing reliance on anthelmintics and mitigating the risk of drug resistance. Genomic studies offer promising insights into the specific genes and pathways involved in mediating resistance or susceptibility, paving the way for targeted genetic selection and the development of more resilient cattle populations (Stear and Murray, 1994). There are studies that further point to changes in sickness behaviour and how different breeds of cattle perform in terms of production performance, fertility, and resilience to diseases (Clasen et al., 2019; Pipino et al., 2023). Whilst we could not identify a clear pattern comparing the breeds present in our trial in terms of prominent behavioural changes under GIN stress, there were some interesting trends that are worth investigating further. For example, we found that there was a clear difference between the SH and SR breeds when looking at the number of individuals at each IPC level and the possible transition between different clinical states during the trial. We also found when comparing SH and SR steers in our trial that some potential indicators of sickness behavior differed from week to week throughout the grazing season (e.g., activity index, number of steps, and even body weight gain). We should, however, mention that we did not include external factors such as precipitation, temperature fluctuations, and relative humidity in this particular study, which could have provided deeper insight into potential differences.
Recent advancements in technology, particularly the development of sensors and wearable devices, offer promising avenues for early diagnostics of GIN infections. Furthermore, the integration of sensor data with predictive modeling offers a novel approach to understanding and forecasting the progression of GIN infections throughout the grazing season. Such models can incorporate environmental variables, host immunity, and grazing management practices to predict infection dynamics, enabling timely and precise interventions. The study by Filipe et al. (2023) investigating the new modelling approach for nematode infections could potentially support the IPC threshold spread observed in our experiment. Their model assumes that an intermediate AH-treatment interval after the start of grazing (either 0, 4, or 8 weeks after turnout on pasture) is optimal and should lead to increased cumulative body weight gain while reducing the cumulative parasite load in the host. This could subsequently reduce the potential risk of production deficits and clinical pathologies. Conversely, immediate treatment (coinciding with turnout) delays the onset of infection and the buildup of immunity. This could lead to a higher overall GIN load compared to later treatment and thus to significant contamination with larvae at the end of the grazing season. The IPC thresholds proposed in our experiment, supported by changes in activity data, could complement the idea proposed by Filipe et al. (2023) and address the idea of tracking the individual animals that potentially contribute the most to pasture contamination. Although it is problematic to know how the clinical impact of GIN infection will evolve in each individual animal with our current approach, it is crucial to find new indicators for TST strategies. The thresholds in the study were arbitrarily chosen and could be explored further. Although optimal values for FEC thresholds remain unclear and few are quantitatively validated to be used for treatment purposes, we recognise that the approach used in this study is aimed at assessing grazing risk rather than reducing animal performance. Nonetheless, an important aspect of data-driven decision-making regarding GIN infections is that the new thresholds could potentially allow the integration of near-individualised data into standard operating procedures for pasture management and treatment and control strategies.
Our study was not without limitations. First and foremost, the duration of the trial period during which activity-based data were collected was insufficient to model the long-term effects of GIN infections in cattle during their first grazing season. One of the logical next steps in such experiments is to place the activity and treatment data in a broader temporal context that allows the investigation of additional features that can be used to develop better classification models for early diagnosis. This would also include a larger number of individuals with different genetic backgrounds to further investigate the influence of breed on the progression of GIN infection and its clinical impact.
Secondly, with regard to pasture contamination, it is crucial to consider the composition of nematode species in relation to the pathogenicity of the host. The species composition in this study was based on back calculations from pooled group samples. This limits the ability to include individual variation in the analysis. Future studies should consider the inclusion of individual composition data and evaluate possible differences in the contribution of parasite transmission in relation to host pathogenicity.
In conclusion, this study pioneers a novel method for monitoring the contribution of individual cattle to pasture contamination, in contrast to host performance, through the use of on-animal sensors, revealing variability in activity patterns among steers in their first grazing season. The findings propose innovative threshold methods for more sustainable anthelmintic usage, integrating individual activity data into future parasite management strategies. This approach not only paves the way for TST strategies but also emphasises the need for further investigation into individual variation in GIN infections. The study underscores the importance of incorporating individual-level data into broader pasture management and anthelmintic treatment plans, marking a significant step towards more precise and sustainable parasite control in cattle.
Data availability statement
The raw data supporting the conclusions of this article will be made available by the authors, without undue reservation.
Ethics statement
The animal study was approved by the study was approved by the Ethics Committee on Animal Experiments in Gothenburg, Sweden (ID number 002530). The study was conducted in accordance with the local legislation and institutional requirements.
Author contributions
OG: Conceptualization, Data curation, Formal analysis, Investigation, Methodology, Project administration, Software, Validation, Visualization, Writing – original draft, Writing – review & editing. AH: Conceptualization, Funding acquisition, Supervision, Writing – review & editing. NH: Writing – review & editing. LL: Writing – review & editing. JH: Conceptualization, Data curation, Formal analysis, Methodology, Visualization, Writing – review & editing.
Funding
The author(s) declare that financial support was received for the research, authorship, and/or publication of this article. SustAinimal, Swedish National Research Council FORMAS grant no: 2020-02977. Västra Götalandsregionen grants no: MRU 2023-00117. European Innovation Partnership grants no: 2019-1744. SLU base research support.
Acknowledgments
We thank Jonas Dahl and David Johansson for their practical work with the animals at SLU Götala Beef and Lamb Research Centre. We would also like to thank Jan-Erik Englund and Adam Flöhr, statisticians at the Department of Biosystems and Technology, SLU for consultancy and discussions during data analysis and final model implementation.
Conflict of interest
The authors declare that the research was conducted in the absence of any commercial or financial relationships that could be construed as a potential conflict of interest.
The author(s) declared that they were an editorial board member of Frontiers, at the time of submission. This had no impact on the peer review process and the final decision.
Publisher’s note
All claims expressed in this article are solely those of the authors and do not necessarily represent those of their affiliated organizations, or those of the publisher, the editors and the reviewers. Any product that may be evaluated in this article, or claim that may be made by its manufacturer, is not guaranteed or endorsed by the publisher.
References
Areskog M., Ljungström B., Höglund J. (2013). Limited efficacy of pour-on anthelmintic treatment of cattle under Swedish field conditions. Int. J. Parasitol. Drugs Drug Resist. 3, 129–134. doi: 10.1016/j.ijpddr.2013.06.002
Areskog M., Sollenberg S., Engström A., Von Samson-Himmelstjerna G., Höglund J. (2014). A controlled study on gastrointestinal nematodes from two Swedish cattle farms showing field evidence of ivermectin resistance. Parasites Vectors 7, 13. doi: 10.1186/1756-3305-7-13
Baker R. L., Mwamachi D. M., Audho J. O., Aduda E. O., Thorpe W. (1999). Genetic resistance to gastro-intestinal nematode parasites in Red Maasai, Dorper and Red Maasai x Dorper ewes in the sub-humid tropics. Animal Science 690 (2), 335–344. doi: 10.1017/S1357729800050906
Baltrušis P., Halvarsson P., Höglund J. (2019). Molecular detection of two major gastrointestinal parasite genera in cattle using a novel droplet digital PCR approach. Parasitol. Res. 118, 2901–2907. doi: 10.1007/s00436-019-06414-7
Berk Z., Bishop S. C., Forbes A. B., Kyriazakis I. (2016). A simulation model to investigate interactions between first season grazing calves and Ostertagia ostertagi. Veterinary Parasitol. 226, 198–209. doi: 10.1016/j.vetpar.2016.05.001
Charlier J., Höglund J., Morgan E. R., Geldhof P., Vercruysse J., Claerebout E. (2020a). Biology and epidemiology of gastrointestinal nematodes in cattle. Veterinary Clinics: Food Anim. Pract. 36, 1–15. doi: 10.1016/j.cvfa.2019.11.001
Charlier J., Höglund J., von Samson-Himmelstjerna G., Dorny P., Vercruysse J. (2009). Gastrointestinal nematode infections in adult dairy cattle: impact on production, diagnosis and control. Veterinary Parasitol. 164, 70–79. doi: 10.1016/j.vetpar.2009.04.012
Charlier J., Morgan E. R., Rinaldi L., Van Dijk J., Demeler J., Höglund J., et al. (2014a). Practices to optimise gastrointestinal nematode control on sheep, goat and cattle farms in Europe using targeted (selective) treatments. Vet. Rec. 175, 250–255. doi: 10.1136/vr.102512
Charlier J., Rinaldi L., Musella V., Ploeger H. W., Chartier C., Vineer H. R., et al. (2020b). Initial assessment of the economic burden of major parasitic helminth infections to the ruminant livestock industry in Europe. Prev. Veterinary Med. 182, 105103. doi: 10.1016/j.prevetmed.2020.10510
Charlier J., van der Voort M., Kenyon F., Skuce P., Vercruysse J. (2014b). Chasing helminths and their economic impact on farmed ruminants. Trends Parasitol. 30, 361–367. doi: 10.1016/j.pt.2014.04.009
Charlier J., Williams D. J., Ravinet N., Claerebout E. (2023). To treat or not to treat: diagnostic thresholds in subclinical helminth infections of cattle. Trends Parasitol. doi: 10.1016/j.pt.2022.11.014
Clasen J. B., Fogh A., Kargo M. (2019). Differences between performance of F1 crossbreds and Holsteins at different production levels. J. Dairy Sci. 102, 436–441. doi: 10.3168/jds.2018-14975
Crouchley R., Davies R. B. (1999). A comparison of population average and random-effect models for the analysis of longitudinal count data with base-line information. J. R. Stat. Society: Ser. A (Statistics Society) 162, 331–347. doi: 10.4135/9781473957992
Dimander S.-O., Höglund J., Uggla A., Spörndly E., Waller P. J. (2003). Evaluation of gastro-intestinal nematode parasite control strategies for first-season grazing cattle in Sweden. Vet. Parasitol. 111, 193–209. doi: 10.1016/S0304-4017(02)00380-1
Elmahalawy S. T., Halvarsson P., Skarin M., Höglund J. (2018). Droplet digital polymerase chain reaction (ddPCR) as a novel method for absolute quantification of major gastrointestinal nematodes in sheep. Veterinary Parasitol. 261, 1–8. doi: 10.1016/j.vetpar.2018.07.008
Fan B., Bryant R., Greer A. (2022). Behavioral fingerprinting: acceleration sensors for identifying changes in livestock health. J. 5, 435–454. doi: 10.3390/j5040030. J.
Filipe J. A. N., Kyriazakis I., McFarland C., Morgan E. R. (2023). Novel epidemiological model of gastrointestinal nematode infection to assess grazing cattle resilience by integrating host growth, parasite, grass and environmental dynamics. Int. J. Parasitol. 53, 133–155. doi: 10.1016/j.ijpara.2022.11.009
Forbes A. (2017). Faecal egg counts in cattle: how do they stack up? Livestock 22, 124–127. doi: 10.12968/live.2017.22.3.124
Forbes M. R., Morrill A., Schellinck J. (2017). Host species exploitation and discrimination by animal parasites. Philos. Trans. R. Soc. B: Biol. Sci. 372, 20160090. doi: 10.1098/rstb.2016.0090
Högberg N., Hessle A., Lidfors L., Enweji N., Höglund J. (2021). Nematode parasitism affects lying time and overall activity patterns in lambs following pasture exposure around weaning. Veterinary Parasitol. 296, 109500. doi: 10.1016/j.vetpar.2021.109500
Högberg N., Lidfors L., Hessle A., Segerkvist K. A., Herlin A., Höglund J. (2019). Effects of nematode parasitism on activity patterns in first-season grazing cattle. Veterinary Parasitol. 276, 100011. doi: 10.1016/j.vpoa.2019.100011
Höglund J., Dahlström F., Sollenberg S., Hessle A. (2013). Weight gain-based targeted selective treatments (TST) of gastrointestinal nematodes in first-season grazing cattle. Vet. Parasitol. 196, 358–365. doi: 10.1016/j.vetpar.2013.03.028
Höglund J., Hessle A., Zaralis K., Arvidsson-Segerkvist K., Athanasiadou S. (2018). Weight gain and resistance to gastrointestinal nematode infections in two genetically diverse groups of cattle. Veterinary Parasitol. 249, 88–91. doi: 10.1016/j.vetpar.2017.11.011
Ikurior S. J., Pomroy W. E., Scott I., Corner-Thomas R., Marquetoux N., Leu S. T. (2020). Gastrointestinal nematode infection affects overall activity in young sheep monitored with tri-axial accelerometers. Veterinary Parasitol. 283, 109188. doi: 10.1016/j.vetpar.2020.109188
Kenyon F., Jackson F. (2012). Targeted flock/herd and individual ruminant treatment approaches. Vet. Parasitol. 186, 10–17. doi: 10.1016/j.vetpar.2011.11.041
Larsson A., Dimander S. O., Rydzik A., Uggla A., Waller P. J., Höglund J. (2007). A 3-year field evaluation of pasture rotation and supplementary feeding to control parasite infection in first-season grazing cattle-Dynamics of pasture infectivity. Vet. Parasitol. 145, 129–137. doi: 10.1016/j.vetpar.2006.12.005
Liang K. Y., Zeger S. L. (1986). Longitudinal data analysis using generalized linear models. Biometrika 73, 13–22. doi: 10.2307/2531248
McFarland C., Vineer H. R., Chesney L., Henry N., Brown C., Airs P., et al. (2022). Tracking gastrointestinal nematode risk on cattle farms through pasture contamination mapping. Int. J. Parasitol. 52, 691–703. doi: 10.1016/j.ijpara.2022.07.003
Michel J. F. (1969). The epidemiology and control of some nematode infections of grazing animals. Adv. Parasitol. 7, 211–282. doi: 10.1016/S0065-308X(08)60436-4
Pipino D. F., Piccardi M., Lopez-Villalobos N., Hickson R. E., Vázquez M. I. (2023). Fertility and survival of Swedish Red and White× Holstein crossbred cows and purebred Holstein cows. J. Dairy Sci. 106, 2475–2486. doi: 10.3168/jds.2022-22403
Rose Vineer H., Morgan E. R., Hertzberg H., Bartley D. J., Bosco A., Charlier J., et al. (2020). Increasing importance of anthelmintic resistance in European livestock: creation and meta-analysis of an open database. Parasite 27, 69. doi: 10.1051/parasite/2020062
Sabatini G. A., de Almeida Borges F., Claerebout E., Gianechini L. S., Höglund J., Kaplan R. M., et al. (2023). Practical guide to the diagnostics of ruminant gastrointestinal nematodes, liver fluke and lungworm infection: interpretation and usability of results. Parasites Vectors 16, 58. doi: 10.1186/s13071-023-05680-w
Sauermann C. W., Leathwick D. M. (2018). A climate-driven model for the dynamics of the free-living stages of Cooperia oncophora. Veterinary Parasitol. 255, 83–90. doi: 10.1016/j.vetpar.2018.03.026
Sonstegard T. S., Gasbarre L. C. (2001). Genomic tools to improve parasite resistance. Veterinary Parasitol. 101, 387–403. doi: 10.1016/S0304-4017(01)00563-5
Stear M. J., Murray M. (1994). Genetic resistance to parasitic disease: particularly of resistance in ruminants to gastrointestinal nematodes. Veterinary parasitology 54 (1-3), 161-176. doi: 10.1016/0304-4017(94)90089-2
Vande Velde F., Charlier J., Claerebout E. (2018). Farmer behavior and gastrointestinal nematodes in ruminant livestock—uptake of sustainable control approaches. Front. Veterinary Sci. 5. doi: 10.3389/fvets.2018.00255
Vineer H. R., Morgan E. R., Hertzberg H., Bartley D. J., Bosco A., Charlier J., et al. (2020). Increasing importance of anthelmintic resistance in European livestock: creation and meta-analysis of an open database. Parasite 27. doi: 10.1051/parasite/2020062
Keywords: GIN, grazing, cattle, PLF, parasitology, modelling, sensors, early diagnostics
Citation: Guzhva O, Hessle A, Högberg N, Lidfors L and Höglund J (2024) Hide ‘n seek: individual behavioural responses of cattle excreting different amounts of nematode eggs—potential threshold for pasture contamination assessment. Front. Anim. Sci. 5:1369677. doi: 10.3389/fanim.2024.1369677
Received: 12 January 2024; Accepted: 20 February 2024;
Published: 11 March 2024.
Edited by:
Joao HC Costa, University of Vermont, United StatesReviewed by:
Ian Sutherland, AgResearch Ltd., New ZealandIoanna Poulopoulou, Free University of Bozen-Bolzano, Italy
Copyright © 2024 Guzhva, Hessle, Högberg, Lidfors and Höglund. This is an open-access article distributed under the terms of the Creative Commons Attribution License (CC BY). The use, distribution or reproduction in other forums is permitted, provided the original author(s) and the copyright owner(s) are credited and that the original publication in this journal is cited, in accordance with accepted academic practice. No use, distribution or reproduction is permitted which does not comply with these terms.
*Correspondence: Oleksiy Guzhva, b2xla3NpeS5ndXpodmFAc2x1LnNl