- 1Department of Animal Science, University of Pretoria, Hatfield, South Africa
- 2Tarleton State University, Texas A&M University System, Stephenville, TX, United States
- 3Department of Paraclinical Sciences, University of Pretoria, Onderstepoort, South Africa
- 4School of Life Sciences, Center for Functional Biodiversity, School of Life Sciences, University of KwaZulu-Natal, Pietermaritzburg, South Africa
Population surveys of crocodilians using uncrewed aerial vehicles (UAV) or drones may become accurate and cost-effective alternatives to more traditional approaches. However, there are currently no quantitative methods for deriving body condition scores of crocodilians through remote sensing. This study presents seven UAV-based morphometric measures collected from rectified aerial imagery of farmed Nile crocodiles. Two hundred and eighty-eight Nile crocodiles, from two commercial crocodile farms in South Africa were included in this study. One farm housed crocodiles which appeared to have wider abdominal girths than those on the second farm, allowing comparisons for a range of sizes and body condition states. An initial disturbance assessment was conducted, and an appropriate flight altitude selected for image acquisition of farmed Nile crocodiles. Altitudes between 40m and 60m above ground level suited the studies requirements and minimized disturbance. A UAV-based body condition index for Nile crocodiles was then developed, offering a non-invasive alternative to traditional condition scoring methods. The body condition index (BCI) was calculated for each crocodile by measuring the relationship between total length and belly width (with the equation: BCI = BW/TL*10) derived from photogrammetrically processed orthophotos in GIS. The BCI values were then normalized to form a body condition score (BCS) with the equation: BCS = (BCI/1.27)*4 + 1. The BCS ranked crocodile body conditions from 1-5, where a score of 1 identified a crocodile that was comparatively thin or emaciated, while a score of 5 identified a crocodile that was relatively fat or obese in contrast to the other crocodiles assessed. A BCS of 3 was the most frequent across all crocodiles in the study, with few animals scoring a 1 or 5. The farm housing crocodiles with narrower abdominal girths had no BCS 5 occurrences, and the farm housing crocodiles with wider abdominal girths had no BCS 1 occurrences. This UAV-based body condition score could be applied to large wild or captive populations for a fast-paced health and welfare evaluation.
1 Introduction
Frequent monitoring of wild Nile crocodile (Crocodylus niloticus) populations to gather demographic and management data can be complicated by their cryptic behavior, occurrence in often inaccessible areas and their semi-aquatic nature (Behangana et al., 2017). In wild crocodile population surveys, it is often easier to observe larger animals, which are more reliably spotted and objectively classed into size categories (Behangana et al., 2017; Aubert et al., 2021; Utete, 2021). Until recently, crocodile population surveys relied exclusively on counts from fixed-wing aircraft and helicopters, or spotlight counts from boats at night; where waterways are accessible (Bayliss, 1987; Combrink, 2004; Ferreira and Pienaar, 2011; Wallace et al., 2013). These methods are potentially disruptive, time consuming, require costly fuel and equipment, and may result in observer-biased size categorization (Elsey and Trosclair, 2016; Ezat et al., 2018).
Uncrewed aerial vehicles (UAVs) have emerged as a valuable tool for conducting crocodile population and habitat surveys due to several advantages over traditional methods, including improved counting accuracy, cost-effectiveness, repeatability, reduced animal stress, and improved researcher safety (Aubert et al., 2021). The efficacy of UAV surveys is constrained by the visibility of animals from above (Aubert et al., 2021). Recent advancements in imaging sensor quality have resulted in higher-quality images, reducing the need for lower flight altitudes (Toffanin, 2019). Given sufficient drone batteries and suitable vehicle access to establish viable drone takeoff and landing points, large areas can now be surveyed whilst producing clear enough images for accurate morphological measurements. Basking behaviour in open systems (e.g., bedrock based, mature river reaches), especially during the winter season, offers the ideal UAV monitoring scenario as many crocodiles leave the water between 10h00 and 17h00 to thermoregulate (Downs et al., 2008). The effects of UAV presence, with a focus on auditory and visual disturbances, observer bias, ethical flight practices, and UAV-related animal behaviours, have been considered (Hodgson and Koh, 2016; Bevan et al., 2018; Appendix 1). The recommended flight altitudes for crocodilians range from 40 to 50 meters, depending on the imaging sensor used, to produce images with sufficient clarity and photogrammetric accuracy for crocodile population monitoring (Bevan et al., 2018; Ezat et al., 2018; Aubert et al., 2021; Myburgh et al., 2021).
Zoometric measures have ecological significance as they provide information about the size, growth, and health of individual crocodiles and populations (Salem, 2011; Warner et al., 2016a). Several zoometric measurements are associated with crocodilians (Edwards et al., 2017), a few of which can be recognized from the perspective of a UAV. Examples of standard morphometric measures for crocodiles are total length (TL: distance from the tip of the snout to the tip of the tail), snout vent length (SVL: distance from the tip of the snout to the third circumcircle scute layer that corresponds to the caudal margin of the cloaca), head length (HL: distance from the tip of the snout to the posterior ridge of the supraoccipital bone) and belly width (BW: transverse measurement across the widest part of a crocodile’s smooth ventral scutes) (Warner et al., 2016a; Edwards et al., 2017; Webb et al., 2021).
Despite advantages of UAV-based crocodile population census are clear, some factors limit the widespread application of the technique. In the case of UAV-based morphometrics, TL as a measure can be problematic due to the loss of tail ends, the difficulty to accurately distinguish the thin tail tip from its surroundings, or due to partial submersion of a crocodile in water (Mazzotti et al., 2012; Myburgh, 2021). Orthophotos with a ground sampling distance (GSD) >3cm/pixel can make sections of the tail difficult to distinguish. Previous studies have derived relationships between TL and SVL or HL of crocodiles as a means of accurately estimating TL from these more reliably captured measures. Morphometric measures hold importance for body condition and therefore welfare assessments, as well as population size estimates (Hutton, 1987; Salem, 2011; Warner et al., 2016a).
A body condition score is a measure of an animal’s relative “fatness”, from which health and well-being is inferred (Fujisaki et al., 2009; Mazzotti et al., 2012; Ojeda-Adame et al., 2020). Body conditions of crocodiles have been assessed in multiple studies using Fultons index (K) which relates length to mass, which is considered useful for comparisons across populations (Zweig, 2003; Fujisaki et al., 2009; Mazzotti et al., 2012; Brandt et al., 2016; Shirley et al., 2017; Webb et al., 2021). Ojeda-Adame et al. (2020) evaluated three condition score indices based on length and mass measurements of 26 American crocodiles (Crocodylus acutus); namely Fultons index, relative condition index and scaled mass index and suggested that the indices were difficult to interpret and potentially misleading. The proposed alternative was an in-depth condition score that assessed multiple morphological zones rather than assigning a relationship between length and volume measures (Ojeda-Adame et al., 2020). For a hands-on assessment this is the recommended condition scoring method as it assesses regions of the body known to be affected by nutritional status.
Hands -on condition scoring techniques may not always be desirable or possible when working with wild populations or particularly large crocodiles. UAV-based body condition assessments have the advantage of being non-invasive and have been applied to Fur seals, Antillean manatees and eastern North Pacific gray whales (Allan et al., 2019; Soledade Lemos et al., 2020; Ramos et al., 2022). In these studies, straight-line body length, belly girth and surface area, among other measures were considered. To our knowledge, no remote sensing body condition assessments have been attempted for crocodilians. A normalized UAV-based body condition score could be a useful health and well-being assessment tool in situations where large-scale assessments are required. These methods could be valuable in instances where rapid assessments of the well-being of a large crocodile population are required, a population is distributed over a large area, where crocodile populations occur in remote locations, or where capture/handling is not possible (i.e., capture difficulties, not wanting to cause the animals undue stress, illness is suspected in a population).
In this study, the relationships between Nile crocodile morphometric measures derived from high resolution rectified UAV imagery were assessed. A logical combination of these measures for the assessment of well-being/health is derived and discussed, in the form of a body condition score. The relationship between several crocodile morphometric parameters is determined for use in future UAV based crocodilian population monitoring.
2 Materials and methods
Two hundred and eighty-eight crocodiles were assessed at two commercial crocodile farms in South Africa. One of the farms crocodiles appeared slimmer at time of the flights, when compared to the other farms crocodiles. The spectrum of thin to fat crocodiles was assessed by the following UAV-captured measures: total length (TL), snout hindlimb length (SHL), snout neck length (SNL), neck width (NW), belly width (BW), total surface area (SA) and perimeter. A drone disturbance assessment occurred prior to morphometrics capture and identified the drone model and flight altitudes that were utilized in the current study, the results of which can be found in Appendix 1.
2.1 Aerial image acquisition and processing
A DJI Mavic Mini UAV with a 12-megapixel CMOS sensor was used to survey 4 pens across two commercial crocodile farms (henceforth referred to as farm A and farm B) in South Africa with an estimated model error of<25mm (Myburgh et al., 2021), i.e., all length measurements accurate to within 25mm. The UAV was programmed to follow a predetermined flight path at an altitude of 40m-60m covering each area in a grid pattern, taking images with approximately 80% frontal and 70% side overlap over the surveyed area. These flight altitudes did produce a noticeable difference in image clarity, however altitudes in this range are suitable for this method and maintain adequate accuracy as per Myburgh et al. (2021) (Appendix 1). The flights were programmed using the automated flight software Dronelink. The flights were conducted between 10h00 and 11h00 on a relatively cold winter morning when the crocodiles were basking and could be photographed out of the water (Downs et al., 2008). Each flight produced images which were processed into a single orthophotograph per flight at 1.42 cm resolution using OpenDroneMap (ODM, version 1.9.3 build 30). An Emlid Reach RS+ differential GPS and 7 ground control points were used to ensure ground truthing of the orthoimages during processing. The resulting orthophotograph from ODM was imported into QGIS (version 3.16-Hannover) (QGIS 2021) for morphometric data capture.
One hundred and forty-five crocodiles from farm A and 143 crocodiles from farm B were included in the analyses. These crocodiles included large growers (between one-year-old and harvest size) up to breeder (mature or adult crocodiles) sized individuals, ranging from >1 meter to 4.8 metres TL. Crocodiles less than 1 meter (m) total length were not included as data capture would have been complicated by orthophoto clarity and the tendency of smaller crocodiles to pile up which concealed body parts needed for the measurements. For consistency, only crocodiles that were lying motionless on the dry emergent area (i.e., basking) with their full bodies captured by the UAV imagery were used in the analyses, while crocodiles in motion or in the water bodies were not measured.
2.2 Deriving morphometric data
Head lengths are traditionally measured using a standard tape measure, but from a UAVs perspective the HL measure can be problematic as the required features of measurement are difficult to detect in the images, especially in low-resolution imagery (Ezat et al., 2018; Jordaan, 2021). HL can be adapted for UAV observations by measuring from the tip of the snout to the posterior margin of the four large dermal scute plates on the neck corresponding to the front limbs of the crocodile, henceforth referred to as snout neck length (SNL). SVL is a measure commonly used when the full length of a crocodile’s tail cannot be accurately measured due to missing tail ends. SVL cannot be precisely measured from UAV imagery as the caudal margin of the cloaca cannot be viewed dorsally on a crocodile (Myburgh, 2021), but this measure can be adapted for UAV observations by measuring from the tip of the snout to the circumcircle scute layer immediately posterior to the back legs, henceforth referred to as snout hindlimb length (SHL). Relationships between TL and SHL and TL and SNL have been proposed (Myburgh, 2021), however correction factors have not been thoroughly tested on a range of crocodilian body sizes and conditions.
All morphometric data were derived with either a line-string layer or vector polygon layer in QGIS. Total length (TL) was measured by estimating the distance between tip of the snout, following the curve of the animals back to the tip of the tail for those individuals where the tip of the tail could be clearly distinguished from the background (line-string layer in QGIS). In images of crocodiles where the tail did not terminate in a clear tip (indicating loss of tail) the individuals were excluded from the assessment. Snout neck length (SNL) was measured by drawing a line (line-string layer in QGIS) from the tip of the crocodile’s snout to the posterior margin of the large dermal scutes on the neck corresponding to the anterior origin of the front limbs. Snout hindlimb length (SHL) was estimated by deriving the distance from the tip of the crocodile’s snout to the last scute layer posterior to the crocodiles’ hind limbs (line-string layer in QGIS). Neck width (NW) was measured by drawing a line across the widest part of a crocodile’s neck, keeping perpendicular to the TL/SHL string layers. The relationships between TL, SNL and SHL were then compared using linear regressions. Figure 1A identifies the morphological features for measuring the UAV-adapted morphometrics that replace SVL and HL (SHL and SNL, respectively) in this study.
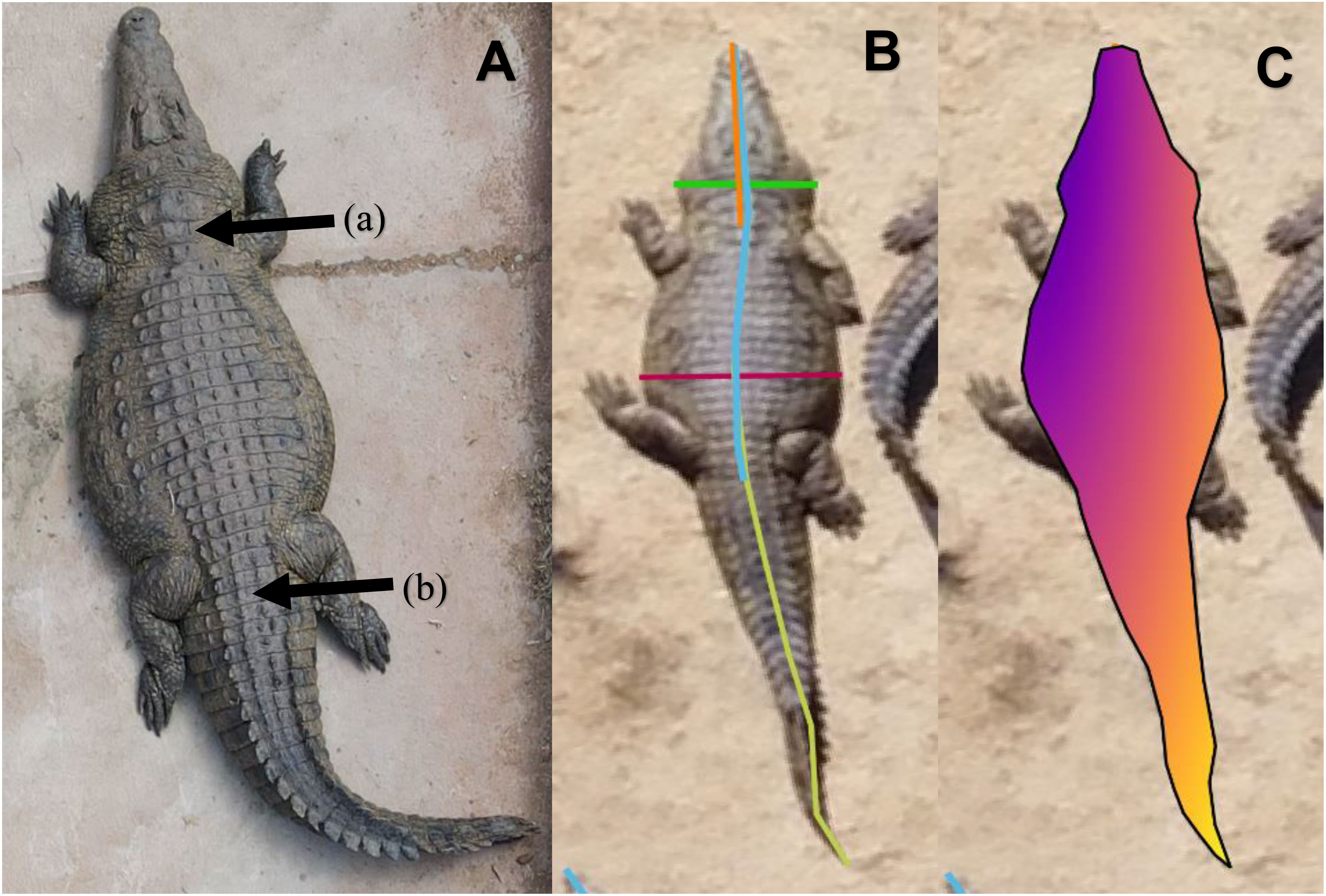
Figure 1 (A) Morphological features recognisable from UAV imagery that are required to capture the morphometric measurements for the current study. (a) Illustrates the conclusion of the dermal neck scutes required for the morphological measure SNL. (b) Illustrates to the circumcircle scute layer behind the hind legs, which is required for the morphological measure SHL. (B) Depicts morphometric measures captured as vector line layers in QGIS; colour codes: total length in yellow, snout hindlimb length in blue, belly width in pink, neck width in green and snout neck length in orange. (C) Depicts morphometric measures captured as vector polygon layers in QGIS: perimeter shown by the black outline of the crocodile, SA shown by the gradient-coloured area that falls within the perimeter line.
Belly width (BW) was measured with a line-string layer in QGIS across the widest part of the crocodile’s stomach, keeping perpendicular to the TL/SHL string layers. Surface area (SA) was measured in QGIS using a vector polygon layer and outlining the crocodile’s body. For the SA morphometric measurement, the tails were included as this is an area of fat deposition (Osthoff et al., 2014), whereas the crocodiles’ legs were not included as their positions were inconsistent from animal to animal. The perimeter was measured with the same vector polygon layer by extracting the length of the outline used to create the SA morphometric. The morphometric measures recorded in QGIS are depicted in Figures 1B, C.
2.3 UAV-based body condition assessment
A body condition index (BCI) was calculated using TL and BW by means of the equation:
The BCI results were used to estimate a body condition score (BCS), by normalizing the BCI values and applying a rank between 1 and 5 (1 being a very low BCS and 5 a very high BCS) to create a score for each crocodile indicating its body condition in relation to all crocodiles measured in this study. This resulted in the following ‘standardized’ equation:
2.4 Statistical analyses
All morphometric data were analyzed in SPSS, version 28.0.1.0 (142), using Generalized Linear Mixed Model analysis and Discriminant Analysis procedures. Pearson correlation coefficients were calculated between variables. All data were analyzed for the determination of significant differences (P< 0.05). Linear regression analyses were performed in Microsoft Excel® to compare the relationships between total length, snout hindlimb length and snout neck length. The relationships between these three measures were considered particularly important because similar measures have been traditionally used for population surveys.
Correlations among the morphometric measures were computed with confidence intervals of 95.0% in SPSS. A stepwise regression was used to assess which of the measures most accurately estimated the body condition of the crocodiles. Total length and belly width were excluded from this analysis because these variables were included in calculating the BCI. Three other morphometric measurements displayed significant (P< 0.05) relationships with body condition: neck width, perimeter, and surface area. The body condition index (BCI) was calculated for each crocodile and then ranked on a scale of 1-5 using a min-max normalisation in Microsoft Excel®.
3 Results
3.1 Deriving morphometric data
Seven UAV-based morphometric measures were captured for 288 farmed Nile crocodiles at two commercial crocodile farms in South Africa. The morphometrics measured were total length (TL), snout hindlimb length (SHL), snout neck length (SNL), neck width (NW), belly width (BW), surface area (SA) and perimeter. Figure 2 depicts various relationships between the UAV-based length morphometrics. Table 1 summarizes the morphometric data collected for all crocodiles in the study. The following morphometric measures varied significantly (P< 0.05) among farms: SHL, BW, NW, SNL, SA and BCI. TL did not vary significantly between farms, possibly because the crocodiles were selected for the study based on TL, ranging from >1m up to breeder sized animals. All morphometric measures were strongly, positively correlated with one another (P< 0.01).
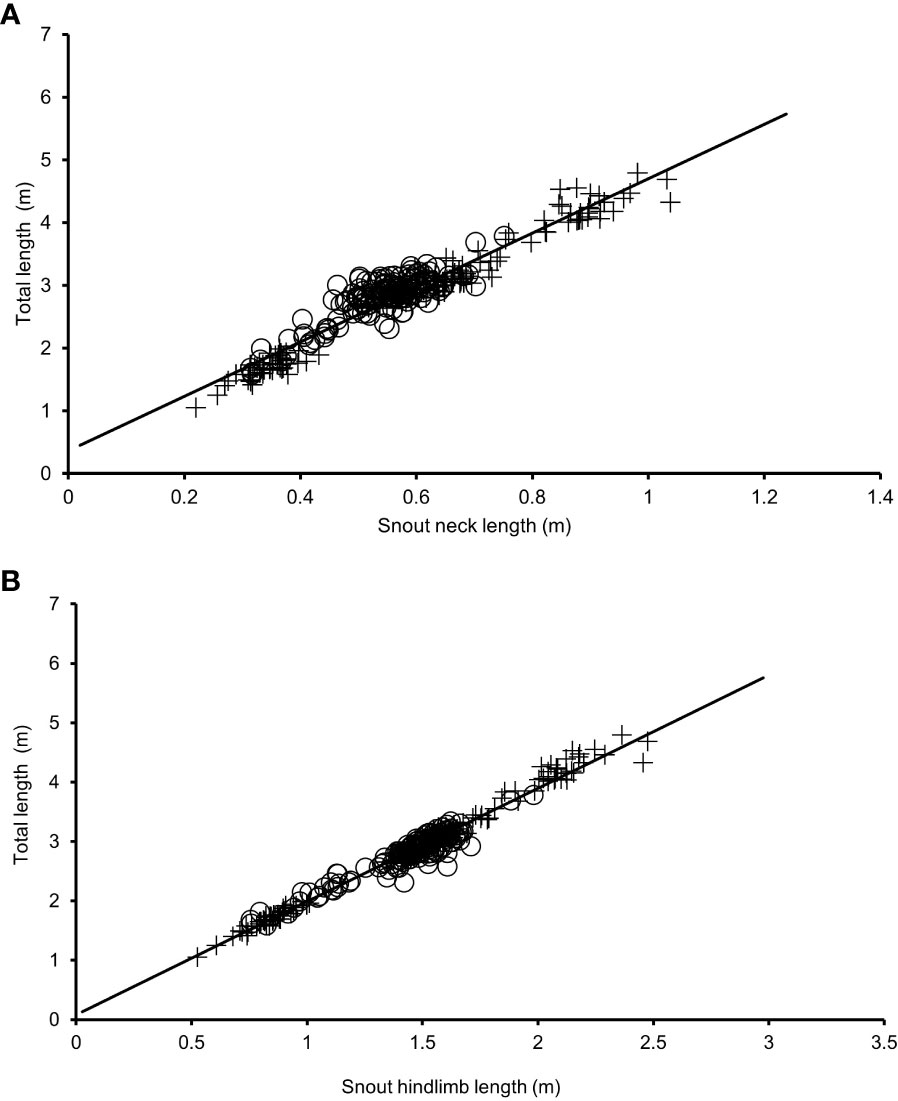
Figure 2 (A) Scatterplot depicting the relationship between total length and snout neck length of 288 farmed Nile crocodiles. (B) Scatterplot depicting total length and snout hindlimb length of 288 farmed Nile crocodiles. Graphs (A, B) present the effects of farm of origin on morphometric measurements, with crocodiles from farm A indicated with “+” and those from farm B with “O”.
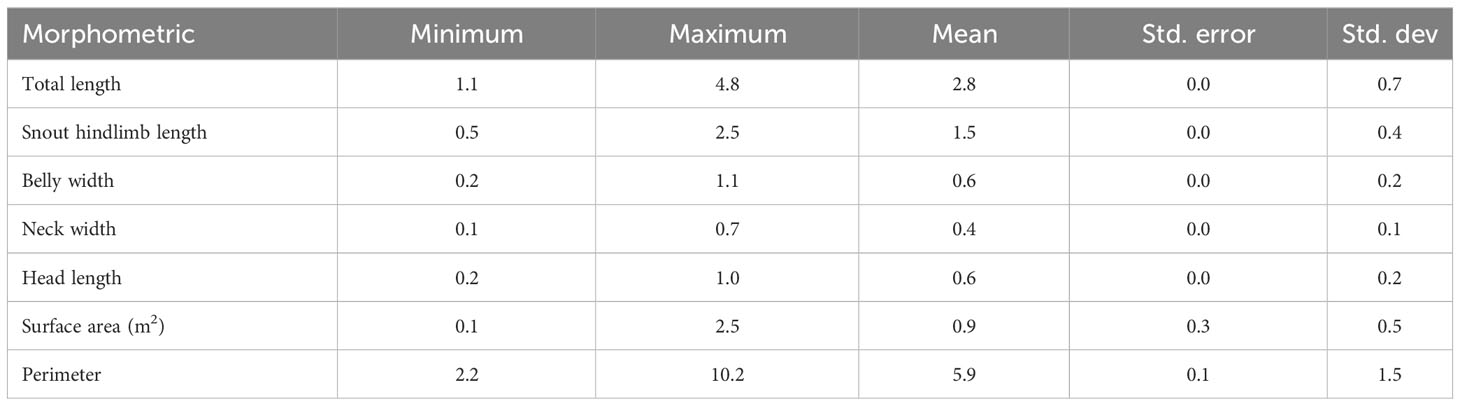
Table 1 Descriptive statistics for the seven UAV-based morphometric measures (n=288), all descriptive statistics were in meters unless otherwise specified.
Figure 2 (A, B) show strong linear relationships between the length based morphometrics in this study, with R2 values of 0.92 (y = 4.33x + 0.37) and 0.97 (y = 1.91x + 0.08), respectively. The R2 values for Farm A were greater than those of Farm B for both morphometric comparisons. The equations describing the regressions between total length and snout neck length are y = 4.51x + 0.17 (R² = 0.97) and y = 4.02x + 0.63 (R² = 0.72), for Farms A and B respectively. The equations describing the regressions between total length and snout hindlimb length are y = 1.95x + 0.05 (R² = 0.99) and y = 1.69x + 0.36 (R² = 0.89), for Farms A and B respectively.
The relationships between TL, SNL, and SHL were assessed using a regression analysis. The resulting equations can be used to infer values that cannot be measured in the field from UAV imagery. The regression analyses between TL, SHL, and SNL were all significant (P< 0.05) linear relationships and resulted in the following equations (rounded to two decimal places):
There was a strong correlation (R2 = 0.97) between the TL and SHL described by the equation:
There was a strong correlation (R2 = 0.92) between TL and SNL described by the equation:
There was a strong correlation (R2 = 0.92) between SNL and SHL described by the equation:
3.2 Body condition scores based on UAV-derived morphometrics
The body condition index (BCI) calculation yielded an index range of 1.344 – 2.618 for both farms, where farm A’s BCIs ranged from 1.62-2.62 (rounded) with an average of 2.06 and farm B’s BCIs ranged from 1.34 - 2.40 (rounded) with an average of 1.90. A min-max normalization allowed the crocodiles to be ranked (BCS) from 1-5 based on their BCIs. Table 2 summarizes the outcome of this normalization and shows the range of body condition indices within each body condition score category.
Figure 3 presents a distribution of crocodiles within the five body condition score categories. Farm A, which was characterized by crocodiles with wider abdominal girths at the time of this assessment, had no crocodiles with BCS 1. Farm B, which was characterized by crocodiles with narrower abdominal girths at the time of this assessment, had no crocodiles with a BCS 5. Scores 1 and 2 are represented by images captured at a flight altitude of 60m and scores 3-5 are represented by images captured at a 40m flight altitude.
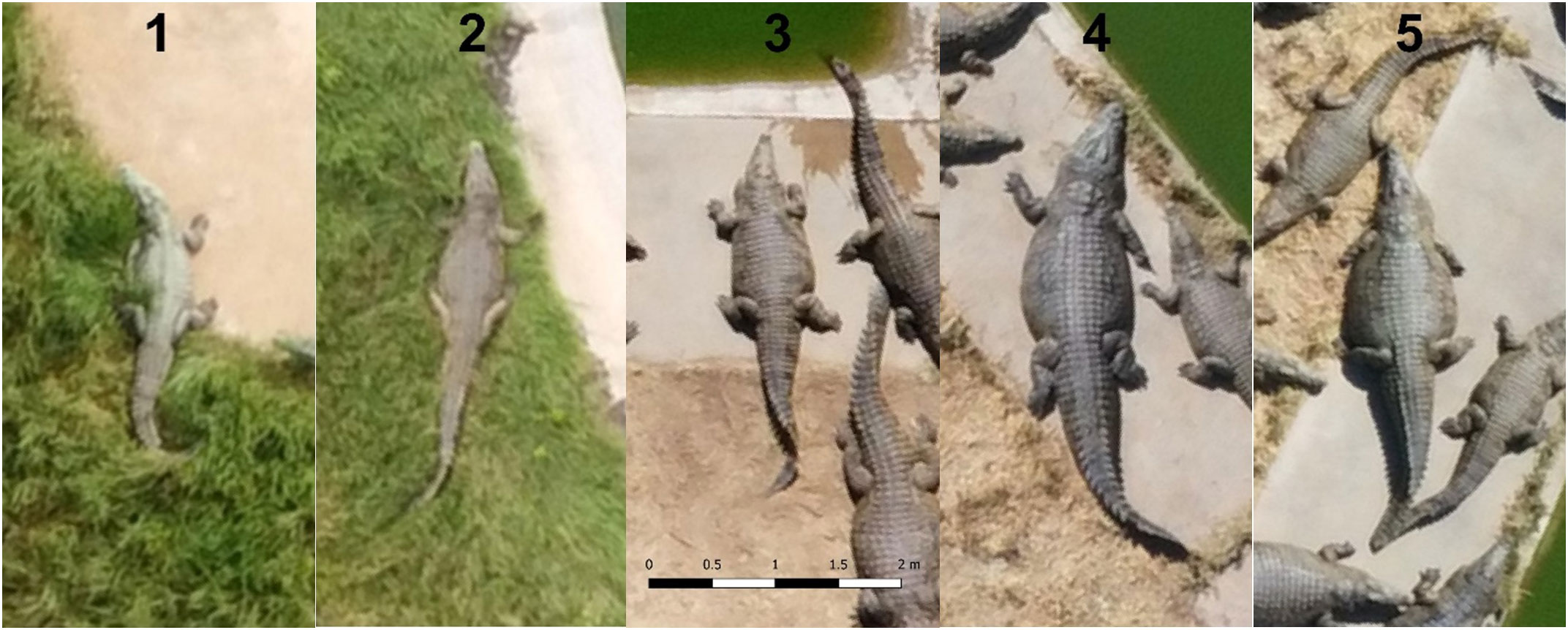
Figure 3 Nile crocodiles within each of the 5 different body condition scoring (BCS) categories. From left to right, a BCS from 1 to 5 as assessed and derived in the current study.
4 Discussion
The current study assessed hundreds of farmed Nile crocodiles in a matter of days, at a relatively low cost. The crocodiles were counted, their morphometrics measured and their health inferred through a body condition score developed with data generated from this work. The variation in body condition scores between the two farms lends credibility to the measures. This method allowed a fast paced and repeatable examination of the sizes and conditions of two populations of captive crocodiles. This method has conservation value and could easily be applied to wild Nile crocodile populations.
When appropriate morphometric measures are used, UAVs enable accurate body condition analyses of crocodiles. The crocodiles assessed in the present study represented a range of body condition states, covering a wide range of body conditions that may be encountered during wild crocodile population surveys. NW was not considered a viable metric for use in wild population surveys due to the difficulty of identifying the neck of crocodiles in lower resolution imagery (Myburgh, unpub. data). BW is considered a more suitable UAV-derived metric for estimating body condition, as it is easier to identify/measure and could be better suited for future applications of emerging technologies. Artificial intelligence, for example, requires distinguishable features for automated detection. Although SHL could be used for estimating body condition relationships, the use of TL was intentional, because previous studies have used TL and its relation to other length-based morphometrics (SVL and HL traditionally, SHL and SNL in this study). Therefore, a UAV-based body condition score was calculated (Equation 2) using TL and BW, which ranked the assessed crocodiles between 1 (comparatively thin) and 5 (comparatively fat). Applying this simple formula to UAV-derived imagery could offer an accurate, fast-paced, and non-invasive health and welfare monitoring tool for large, wild or captive crocodile populations.
Relationships between various zoometric parameters for crocodilians have been investigated through traditional capture and measurement methods (Hutton, 1987; Salem, 2011; Warner et al., 2016a), but none have assessed UAV derived morphometrics. Multiple equations for predicting UAV-based length morphometrics from one another were presented in the current study (equations 3-5), all with R2 values ≥0.92. TL was more reliably estimated from SNL and SHL for Farm A than from farm B, and it is postulated that variations in body condition status may have influenced the observed differences in R-squared values.
These equations can be useful in generating population-wide morphometric statistics, even when not all body parts of a Nile crocodile can be seen in UAV imagery. The strong positive and significant Pearson correlation coefficients between the length-based morphometric measures are likely indicative of allometric growth. Relating these morphometrics to body weight and manual morphometric measurements would add further precision to UAV-based morphometric data collections and body condition scoring in future studies.
Morphometrics measured in this study were only possible for crocodiles >1m TL due to image distortion for smaller crocodiles (measurement landmarks not clearly visible). The tendency for smaller crocodiles to pile (especially in pens on commercial farms) and thereby prevent accurate measurements, and the skittish nature of small (<1m total length) crocodiles impedes image quality. For farmed Nile crocodile populations specifically, the measurement of crocodiles <1m in TL is still a potentially useful endeavor in terms of continuous morphometric and condition assessments if a viable method for surveying them can be developed. Further assessments are ongoing regarding UAV-based morphometrics for farmed grower (<1m total length) Nile crocodiles (D Viljoen, unpub. data).
Possible confounding factors for health predictions conducted through monitoring morphometrics (i.e., a body condition index/score) would be chronic diseases, toxicological problems, pathological changes, being gravid, and food availability. Pansteatitis for example, where crocodiles appear obese due to the swelling of all fat depots (Lane et al., 2013), would likely confound condition scoring within a population. To our knowledge, the effects of egg-bearing on crocodilian morphometric relationships have yet to be explored. Emaciation is suggestive of animals experiencing health or welfare issues; however, sightings of emaciated crocodiles are not uncommon in the wild (Swanepoel et al., 2000; Warner et al., 2016a, b). The low BCS of 1 or 2 for farmed crocodiles may be a more natural state for wild crocodiles where feeding is infrequent and health issues are not as controlled.
Future studies could consider assigning a cut-off value for “healthy” morphometrics per size class, which may differ for wild and farmed crocodiles, allowing the identification of discrepancies/differences between obese, emaciated, and gravid crocodiles. Considering pansteatitis specifically, this might allow particularly obese crocodiles to be identified as early warning signs of the disease. Future studies utilizing UAV-based morphometrics should also consider comparing UAV measures with true measures from captured crocodiles and including weight recordings to expand the UAV body condition methods capabilities and accuracy, as well as its comparison to traditional body metrics.
Data availability statement
The datasets presented in this article are not readily available because of the sensitive nature of the data and confidentiality agreements with the farms involved. Requests to access the datasets should be directed to the corresponding author.
Ethics statement
The animal studies were approved by the Animal Ethics Committee, University of Pretoria. The studies were conducted in accordance with the local legislation and institutional requirements. Written informed consent was obtained from the owners for the participation of their animals in this study.
Author contributions
DV and AM designed the study and conducted the experiments/data collection. DV, EW and AM contributed to the statistical analyses. DV composed the initial and subsequent drafts of the manuscript. All authors reviewed and edited the manuscript, providing critical feedback and approving the final version.
Funding
The authors gratefully acknowledge the financial support provided for this research by the IUCN-SSC Crocodile Specialist Group and the University of Pretoria.
Acknowledgments
The authors thank the crocodile farms for their cooperation in this study.
Conflict of interest
The authors declare that the research was conducted in the absence of any commercial or financial relationships that could be construed as a potential conflict of interest.
The reviewer XC declared a past co-authorship with the author AM to the handling editor.
Publisher’s note
All claims expressed in this article are solely those of the authors and do not necessarily represent those of their affiliated organizations, or those of the publisher, the editors and the reviewers. Any product that may be evaluated in this article, or claim that may be made by its manufacturer, is not guaranteed or endorsed by the publisher.
Supplementary material
The Supplementary Material for this article can be found online at: https://www.frontiersin.org/articles/10.3389/fanim.2023.1225396/full#supplementary-material
References
Allan B. M., Ierodiaconou D., Hoskins A. J., Arnould J. P. (2019). A rapid UAV method for assessing body condition in fur seals. Drones 3 (1), 24. doi: 10.3390/drones3010024
Aubert C., Le Moguédec G., Assio C., Blatrix R., Ahizi M. N. D., Hedegbetan G. C., et al. (2021). Evaluation of the use of drones to monitor a diverse crocodylian assemblage in West Africa. Wildlife Res. 49 (1), 11–23. doi: 10.1071/WR20170
Bayliss P. (1987). Survey methods and monitoring within crocodile management programs. Wildlife management: crocodiles alligators, 157–175.
Behangana M., Lukwago W., Dendi D., Luiselli L., Ochanda D. (2017). Population surveys of Nile crocodiles (Crocodylus niloticus) in the Murchison Falls National Park, Victoria Nile, Uganda. Eur. J. Ecol. 3 (2), 67–76. doi: 10.1515/eje-2017-0015
Bevan E., Whiting S., Tucker T., Guinea M., Raith A., Douglas R. (2018). Measuring behavioral responses of sea turtles, saltwater crocodiles, and crested terns to drone disturbance to define ethical operating thresholds. PloS One 13 (3), e0194460. doi: 10.1371/journal.pone.0194460
Brandt L. A., Nestler J. H., Brunell A. M., Beauchamp J. S., Mazzotti F. J. (2016). Variation in body condition of Alligator mississippiensis in Florida. Bull. Fl Mus Nat. History 54, 1–12. Available at: https://www.flmnh.ufl.edu/bulletin/publications/.
Combrink A. S. (2004). Population Survey of Crocodylus niloticus (Nile crocodile) at Lake Sibaya, Republic of South Africa. Doctoral dissertation (South Africa: University of KwaZulu-Natal). Available at: https://researchspace.ukzn.ac.za/handle/10413/3572.
Downs C. T., Greaver C., Taylor R. (2008). Body temperature and basking behaviour of Nile crocodiles (Crocodylus niloticus) during winter. J. Thermal Biol. 33 (3), 185–192. doi: 10.1016/j.jtherbio.2008.02.001
Edwards G. P., Webb G. J., Manolis S. C., Mazanov A. (2017). Morphometric analysis of the Australian freshwater crocodile (Crocodylus johnstoni). Aust. J. Zool. 65 (2), 97–111. doi: 10.1071/ZO16079
Elsey R. M., Trosclair P. L. (2016). The use of an unmanned aerial vehicle to locate alligator nests. Southeastern Nat. 15 (1), 76–82. doi: 10.1656/058.015.0106
Ezat M. A., Fritsch C. J., Downs C. T. (2018). Use of an unmanned aerial vehicle (drone) to survey Nile crocodile populations: A case study at Lake Nyamithi, Ndumo game reserve, South Africa. Biol. Conserv. 223, 76–81. doi: 10.1016/j.biocon.2018.04.032
Ferreira S. M., Pienaar D. (2011). Degradation of the crocodile population in the Olifants River gorge of Kruger National Park, South Africa. Aquat. Conservation: Mar. Freshw. Ecosyst. 21 (2), 155–164. doi: 10.1002/aqc.1175
Fujisaki I., Rice K. G., Pearlstine L. G., Mazzotti F. J. (2009). Relationship between body condition of American alligators and water depth in the Everglades, Florida. Hydrobiologia 635, 329–338.
Hodgson J. C., Koh L. P. (2016). Best practice for minimizing unmanned aerial vehicle disturbance to wildlife in biological field research. Curr. Biol. 26 (10), R404–R405. doi: 10.1016/j.cub.2016.04.001
Hutton J. M. (1987). Morphometrics and field estimation of the size of the Nile crocodile. Afr. J. Ecol. 25 (4), 225–230. doi: 10.1111/j.1365-2028.1987.tb01113.x
Jordaan P. R. (2021). The establishment of a multifaceted Crocodylus niloticus Laurenti 1768 monitoring program on Maputo Special Reserve (Maputo Province, Mozambique) with preliminary notes on the population (Reptilia: Crocodylidae). Herpetol. Notes 14, 1155–1162.
Lane E. P., Huchzermeyer F. W., Govender D., Bengis R. G., Buss P. E., Hofmeyr M., et al. (2013). Pansteatitis of unknown etiology associated with large-scale nile crocodile (Crocodylus niloticus) mortality in Kruger National Park, South Africa: Pathologic findings. J. Zoo Wildlife Med. 44 (4), 899–910. doi: 10.1638/2012-0264R.1
Mazzotti F. J., Cherkiss M. S., Brandt L. A., Fujisaki I., Hart K., Jeffery B., et al. (2012). Body condition of Morelet's crocodiles (Crocodylus moreletii) from Northern Belizea. J. Herpetol. 46 (3), 356–362. doi: 10.1670/11-188
Myburgh H. A. (2021). Aspects of monitoring wild and captive Nile crocodile (Crocodylus niloticus) populations in southern Africa. Doctoral dissertation (South Africa: University of KwaZulu-Natal). Available at: https://researchspace.ukzn.ac.za/handle/10413/21089.
Myburgh A., Botha H., Downs C. T., Woodborne S. (2021). The application and limitations of a low-cost UAV platform and open-source software combination for ecological mapping and monitoring. Afr. J. Wildlife Res. South Africa: Doctoral dissertation, University of KwaZulu-Natal 51 (1), 166–177. doi: 10.3957/056.051.0166
Ojeda-Adame R. A., Hernández-Hurtado H., Ramírez-Martinez M. M., Iñiguez-Davalos L. I. (2020). A body condition score for crocodilians. South Am. J. Herpetol. 16 (1), 10–15. doi: 10.2994/SAJH-D-18-00074.1
Osthoff G., Hugo A., Govender D., Huchzermeyer F., Bouwman H. (2014). Comparison of the lipid composition of three adipose tissue types of male and female wild Nile crocodiles (Crocodylus niloticus). J. Herpetol. 48 (4), 525–531. doi: 10.1670/13-096
Ramos E. A., Landeo-Yauri S., Castelblanco-Martínez N., Arreola M. R., Quade A. H., Rieucau G. (2022). Drone-based photogrammetry assessments of body size and body condition of Antillean manatees. Mamm. Biol. 102 (3), 765–779. doi: 10.1007/s42991-022-00228-4
Salem A. (2011). Morphometric measurement and field estimation of the size of Crocodylus niloticus in Lake Nasser (Egypt). Transylv. Rev. Syst. Ecol. Res. 12, 53–74.
Shirley M. H., Burtner B., Oslisly R., Sebag D., Testa O. (2017). Diet and body condition of cave-dwelling dwarf crocodiles (Osteolaemus tetraspis, Cope 1861) in Gabon. Afr. J. Ecol. 55 (4), 411–422. doi: 10.1111/aje.12365
Soledade Lemos L., Burnett J. D., Chandler T. E., Sumich J. L., Torres L. G. (2020). Intra-and inter-annual variation in gray whale body condition on a foraging ground. Ecosphere 11 (4), e03094. doi: 10.1002/ecs2.3094
Swanepoel D., Kriek N. P. J., Boomker J. D. F. (2000). Selected chemical parameters in the blood and metals in the organs of the Nile crocodile, Crocodylus niloticus, in the Kruger National Park. Onderstepoort J. Vet. Res. 67, 141–148.
Toffanin P. (2019). OpenDroneMap: the missing guide (UAV4GEO). Available at: https://odmbook.com/.
Utete B. (2021). A review of the conservation status of the Nile crocodile (Crocodylus niloticus Laurenti 1768) in aquatic systems of Zimbabwe. Global Ecol. Conserv. 29, e01743. doi: 10.1016/j.gecco.2021.e01743
Wallace K. M., Leslie A. J., Coulson T., Wallace A. S. (2013). Population size and structure of the Nile crocodile Crocodylus niloticus in the lower Zambezi valley. Oryx 47 (3), 457–465. doi: 10.1017/S0030605311001712
Warner J. K., Combrink X., Calverley P., Champion G., Downs C. T. (2016a). Morphometrics, sex ratio, sexual size dimorphism, biomass, and population size of the Nile crocodile (Crocodylus niloticus) at its southern range limit in KwaZulu-Natal, South Africa. Zoomorphology 135, 511–521. doi: 10.1007/s00435-016-0325-8
Warner J. K., Combrink X., Myburgh J. G., Downs C. T. (2016b). Blood lead concentrations in free-ranging Nile crocodiles (Crocodylus niloticus) from South Africa. Ecotoxicology 25, 950–958. doi: 10.1007/s10646-016-1652-8
Webb E. C., Veldsman D. M., Myburgh J. G., Swan G. E. (2021). Effects of stocking density on growth and skin quality of grower Nile crocodiles (Crocodylus niloticus). South Afr. J. Anim. Sci. 51 (2), 142–150. doi: 10.4314/sajas.v51i2.1
Keywords: UAV, Nile crocodile, welfare, body condition, morphometrics, non-invasive
Citation: Viljoen D, Webb E, Myburgh J, Truter C and Myburgh A (2023) Remote body condition scoring of Nile crocodiles (Crocodylus niloticus) using uncrewed aerial vehicle derived morphometrics. Front. Anim. Sci. 4:1225396. doi: 10.3389/fanim.2023.1225396
Received: 19 May 2023; Accepted: 30 August 2023;
Published: 18 September 2023.
Edited by:
E. Tobias Krause, Friedrich-Loeffler-Institute, GermanyReviewed by:
Xander Combrink, Tshwane University of Technology, South AfricaGraham Alexander, University of the Witwatersrand, South Africa
Copyright © 2023 Viljoen, Webb, Myburgh, Truter and Myburgh. This is an open-access article distributed under the terms of the Creative Commons Attribution License (CC BY). The use, distribution or reproduction in other forums is permitted, provided the original author(s) and the copyright owner(s) are credited and that the original publication in this journal is cited, in accordance with accepted academic practice. No use, distribution or reproduction is permitted which does not comply with these terms.
*Correspondence: Devon Viljoen, dTEwMTIwMjYzQHR1a3MuY28uemE=
†ORCID: Devon Viljoen, orcid.org/0000-0003-3548-9291
Edward Webb, orcid.org/0000-0001-5648-6319
Jan Myburgh, orcid.org/0000-0002-2132-7251
Christoff Truter, orcid.org/0000-0002-2991-6464
Albert Myburgh, orcid.org/0000-0002-6891-1893