- 1Central Agricultural Research Center, Montana State University, Moccasin, MT, United States
- 2Department of Animal and Range Sciences, Montana State University, Bozeman, MT, United States
Introduction: Limited forage quantity and quality are challenges faced in livestock production systems in semi-arid rangelands of the western United States, particularly when livestock face stressors such as cold weather or have increased nutritional requirements such as during pregnancy and lactation. To meet livestock nutrient requirements, producers frequently provide supplemental nutrition, however there is limited knowledge regarding the effects of these practices on the rumen microbiome in these environments.
Methods: A study was conducted to evaluate changes in the rumen microbiome in response to high- and low- quality forage with sustained release mineral boluses. The study consisted of 16 ruminally-cannulated 2–3-year-old black angus cows fed high quality grass alfalfa hay or low-quality grass hay with a 90 or 180 day sustained release mineral bolus. Rumen samples were collected pre-feeding and 8 hours post feeding and bacterial 16S rRNA gene amplicons were sequenced from the rumen fluid.
Results: Alpha diversity as measured by Shannon’s diversity index decreased significantly over time (p<0.01) and averaged 5.6 pre-feeding and 5.4 post- feeding and was not significantly different between high- and low-quality forages or between mineral bolus types (p>0.05). Principal coordinates analysis (PCoA) of the Bray-Curtis dissimilarity matrix showed distinct grouping by feed quality and time but not by mineral bolus type. Bacteroidetes and Firmicutes were the dominant phyla in all treatments and significant increases (p<0.05) in the relative abundance of the family Lachnospiraceae and the genus Prevotella were observed in high quality forage diets. Rumen VFA and NH3-N concentrations were also strongly associated with the high-quality forage diet. Predictive functional profiling indicated that functions associated with methanogenesis were negatively correlated with feed quality.
Discussion: The results of this study suggest that mineral bolus type is unlikely to affect rumen bacterial community structure or function while forage quality can significantly alter community structure and predicted functions associated with methanogenesis and VFA production.
Introduction
Livestock production systems in semi-arid rangelands in the western United States are challenged by limited forage quantity and quality. Shorter growing seasons in northern latitudes and high-elevation areas require reliance on harvested forage to support livestock during the winter. Forage quality is particularly important when livestock face stressors like cold weather or have increased nutritional requirements such as during pregnancy and lactation. In addition, trace mineral deficiencies are common in ruminants during lactation (Zhao et al., 2022). To meet livestock nutrient requirements, producers supplement low quality (low crude protein) forage with protein and mineral supplements (DelCurto et al., 2000). Producers have access to a variety of mineral delivery systems to meet nutrient requirements but often these systems have limitations. Self-fed systems are often limited by extreme variation in intake, while single dose delivery systems have rapid serum and liver response rates, but a short-term effect requiring multiple treatments to meet the animal’s nutrient requirements (Arthington and Swensont, 2004; Jackson et al., 2020).
Previous work has evaluated the use of sustained release mineral boluses on cattle performance (Sprinkle et al., 2006; Jackson et al., 2020; Sprinkle et al., 2021). Results have shown that supplementation with a long-acting trace mineral bolus decreased calving interval and increased calf-weaning weights from cattle grazed in a rangeland environment (Sprinkle et al., 2021). There are few studies characterizing degradation rates of sustained release mineral boluses and factors that impact degradation rates (Carlisle et al., 2021) and little is known regarding the interaction of mineral boluses and forage quality on the rumen microbial community structure and function.
Dietary nutritional profile, forage quality, and mineral availability are crucial in shaping the structure and function of the rumen microbial community. The rumen contains a complex microbial consortium that colonizes and degrades ingested plant material. End products of the anaerobic degradation of complex cellulolytic and hemicellulolytic feedstocks are primarily volatile fatty acids (VFAs) consisting of predominantly acetate, propionate, and butyrate (Newbold and Ramos-Morales, 2020). Dietary changes in nutrient availability can alter the rumen microbial community composition and associated functions such as fiber degradation and fatty acid hydrogenation (Newbold and Ramos-Morales, 2020). Studies have also shown that cattle fed high-concentrate diets tend to have higher abundances of Prevotella ruminicola, P. brevis, P. bryantii and P. albensis while forage-fed cattle had greater abundances of Fibrobacter and greater microbial diversity based on Simpson’s alpha diversity and beta diversity metrics (Henderson et al., 2015; Tapio et al., 2017). Research comparing different forage sources with supplemental protein and minerals have shown that cattle fed an alfalfa hay-based diets had greater microbial alpha diversity than cattle fed a triticale straw-based diet (Kong et al., 2010; Petri et al., 2018). Mineral availability can impact fermentation. Selenium can alter the rumen microbiome composition and VFA content (Hendawy et al., 2021) and mineral supplementation has been linked to greater abundance of propionate producing bacteria (Liu et al., 2017). In contrast, other studies have shown either no effect or a negative effect on rumen fermentation in response to manganese and zinc supplementation although the response varied based on the mineral source (Liu et al., 2017; Vigh et al., 2023).
While numerous studies have evaluated the effects of diet quality on rumen microbial community structure and function, few have evaluated forage quality in conjunction with sustained release mineral boluses in beef cattle. Previously we found that diet quality affected degradation rates of sustained release mineral boluses (Carlisle et al., 2021). The objective of this present study was to characterize the effect of forage quality in conjunction with two different time release mineral supplement boluses on the structure and function of the rumen microbiome. We hypothesized that; 1) Rumen microbial diversity would be higher in cows fed high quality forage and 2) Mineral bolus prototype would alter rumen microbial community structure or function.
Materials and methods
All protocols and procedures were approved by the Montana State University Agricultural Animal Care and Use Committee (#2019-AA12). All animals used in this study were provided by the Montana Agriculture Experiment Station. This study was conducted at the Bozeman Agriculture Research and Teaching farm at Montana State University in Bozeman, MT, USA.
Samples used in this study were obtained in a previous experiment (Carlisle et al., 2021). Briefly, a diet quality and mineral bolus study was established with 16 ruminally cannulated Angus crossbred cows (age 2 – 3 years, average weight 644 kg). The study was conducted as a completely randomized design with a 2 × 2 factorial arrangement of treatments. Treatments consisted of high- or low-quality forage diets and a 90-day or 180-day sustained release mineral bolus. The low-quality forage had > 600 g/kg neutral detergent fiber and < 80 g/kg crude protein, dry matter basis while high-quality forage had < 500 g/kg neutral detergent fiber and > 150 g/kg crude protein, dry matter basis (Carlisle et al., 2021). High quality forage consisted of alfalfa grass hay while low quality forage was mature wheatgrass hay. The low-quality treatment was protein supplemented with canola meal supplement containing 463 g/kg crude protein (CP) at a daily rate of 0.91 kg/hd to meet the maintenance requirements of mature, non-lactating, beef cows per NRC requirements. Average daily intake in the high-quality treatment was 14.24 kg/d (2.5 kg CP/d) while the cattle in the low quality group consumed an average of 13.86 kg/d (1.4 kg CP/d) (Carlisle et al., 2021). This resulted in an average of 78% greater CP intake by cattle on the higher quality diet. Nutrient analysis of the high quality and low-quality hay are shown in Table 1 and nutrient composition of the sustained release mineral boluses are shown in Table 2. Cattle were adapted to basal diets for 14 days prior to initiating the intake and digestion period. This was followed with a 15-d intake and digestion period to quantify the effects of forage quality on intake, digestion, and rumen fermentation characteristics of individual cows. The period selected for sampling was based on a previous evaluation of the kinetic of bolus degradation (Carlisle et al., 2021). Animals were randomly assigned to individual pens according to diet and bolus treatment (Carlisle et al., 2021). Rumen samples (100 ml) were collected with a suction strainer prior to feeding (0-hr) and 8-hr post feeding for microbial community analysis. Samples collected at 0-hr represented a baseline prior to feeding, and 8-hr post feeding was selected based on rumen content evaluation which showed maximum VFA concentrations were achieved by this timepoint (Carlisle et al., 2021). Samples were stored at -80°C prior to submitting for DNA sequencing.
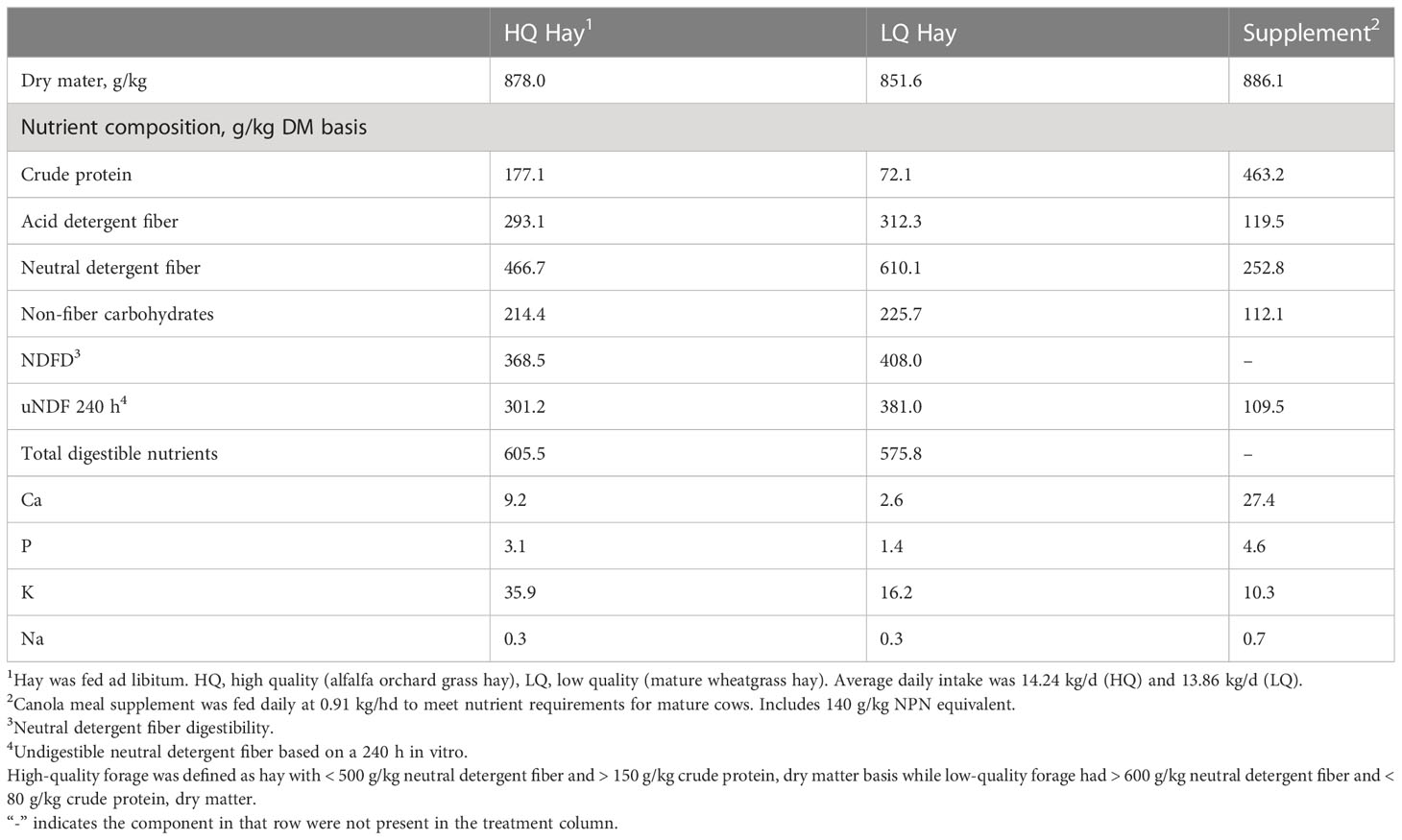
Table 1 Nutrient analysis of high-quality hay, low-quality hay, and supplement offered to cannulated beef cows.
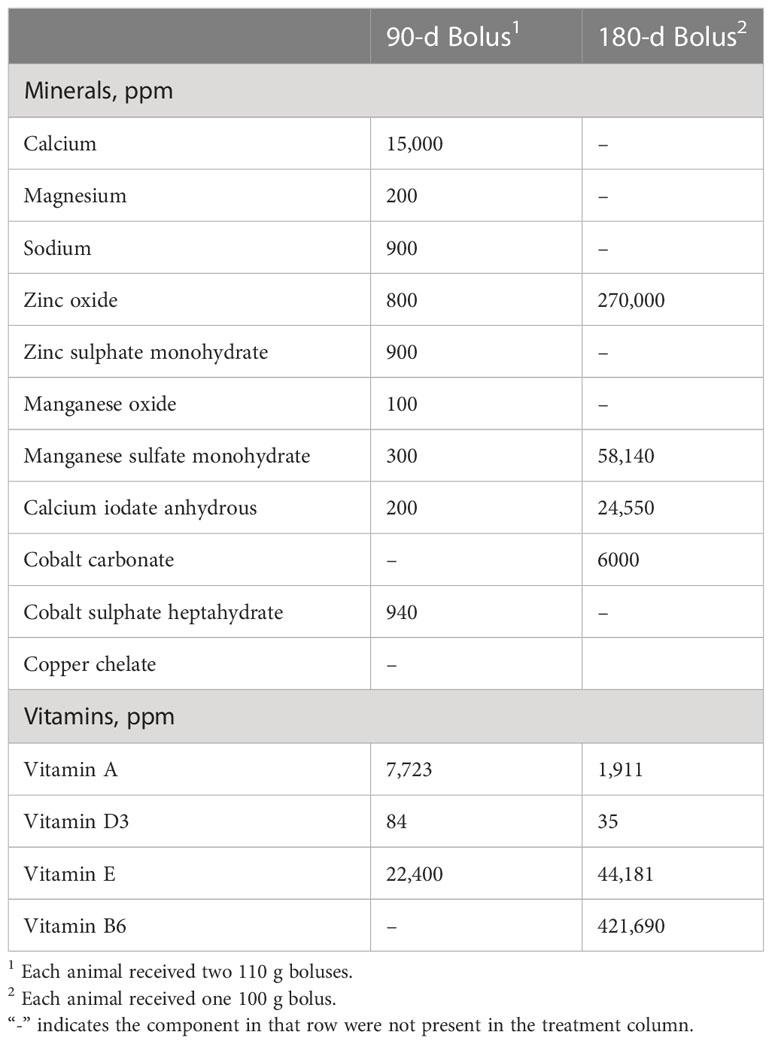
Table 2 Nutrient composition and dosages of sustained release mineral boluses, administered to rumen cannulated Angus crossbred beef cows. .
Bacterial community sequencing
DNA extraction and sequencing were performed by Zymo Research (Irvine, CA, USA) on the Illumina MiSeq platform (Illumina, San Diego, CA). The universal V3V4 region of the 16S gene was targeted for bacterial amplification an Illumina v3 reagent kit (2 x 300 bp). The ZymoBIOMIC Microbial Community DNA Standard (Zymo Research, Irvine, CA) was used as a positive control. Negative controls were included to assess contamination. The final library was sequenced on the Illumina MiSeq® platform (Illumina, San Diego, CA).
Bioinformatics
Quality control and processing of sequence reads was performed using the QIIME2 bioinformatics pipeline (Bolyen et al., 2019). Demultiplexed sequences were trimmed by 5 bases at the start position and truncated using a Q score threshold of 30 which resulted in forward and reverse reads being truncated to 280 bp and 250 bp respectively. Following quality filtering and chimera removal, paired end reads were assembled into error-corrected amplicon sequence variants (ASVs) using DADA2 (Callahan et al., 2016). Taxonomic assignment was performed using a naive Bayes classifier pre-trained on the weighted Silva 138 database with a 99% identity threshold (Quast et al., 2013).
Files generated in QIIME2 were imported into R 4.1.3 (Team, 2021) using the package Qiime2R (Bisanz, 2018) and statistical analyses and data visualization were performed using phyloseq, MicroViz, ggplot2, ggpubr, and vegan packages (McMurdie and Holmes, 2013; Wickham, 2016; Okansen et al., 2019; Barnett et al., 2021). ASV counts were center log ratio (clr) transformed as recommended for compositional data (Gloor et al., 2017) using the R package phyloseq (McMurdie and Holmes, 2013). Alpha diversity analysis was performed using the Shannon diversity index, an indicator of species diversity.
Beta diversity variance was visualized with compositionally transformed abundances using the Bray-Curtis dissimilarity matrix and clustered using principal coordinates analysis (PCoA) with ellipses drawn at the 95% confidence interval. The relationship between rumen bacterial community structure and forage quality and rumen content factors was analyzed by redundancy analysis (RDA) with plots generated in MicroViz (Barnett et al., 2021). After removal of redundant variables, 11 forage and rumen factors were selected for RDA.
Differential abundance testing across forage quality and time pre-, and 8 hours post-feeding was performed with the metacoder R package (Foster et al., 2017) using the Wilcoxon rank-sum test and FDR corrected for multiple comparisons. Heat tree comparisons incorporate phylogenetic trees which provide a more informative comparison of community structure than bar plots alone (Foster et al., 2017). The Log2 ratio of median proportions of taxa were plotted at the family level and taxa names were displayed only for taxa that were significantly different between treatments (p<0.05, FDR corrected).
Predictive functional profiling
To assess treatment induced functional changes in the rumen, potential functions in bacterial communities were predicted using Functional Annotation of Prokaryotic Taxa (FAPROTAX) (Louca et al., 2016a; Louca et al., 2016b) as implemented in the Microeco R package (Liu et al., 2021). A correlation heat map was generated to show Spearman’s correlation between the most abundant functions and forage quality, mineral bolus type, and sampling time. A linear discriminant analysis (LDA) effect size (LEfSe) approach was used to identify functional pathways that were discriminatory between treatments (Segata et al., 2011). LDA was performed using the microeco R package (Liu et al., 2021). Only functions with an LDA score greater than 3 and significant differences (p<0.05, FDR corrected) between treatments were plotted.
Statistical analysis
Linear regression with mixed effect models were used to explore the relationships between alpha diversity and treatments. Forage quality, mineral bolus type, time, and interactions among them were included as fixed effects with replication as a random effect in the mixed effects model. Tukey’s post-hoc comparison was used to evaluate differences in means between treatments. Regression analyses were performed using the lme4 R package (Bates et al., 2015). The betadisper function in the vegan package was used to assess the assumption of homogenous dispersion for performing a permutational analysis of variance (PERMANOVA). PERMANOVA was performed used weighted UniFrac distances in QIIME2 to test for community differences between feed quality, mineral bolus type, and sampling time. This approach uses pseudo-F test statistics, to provide pseudo-F probability values (p-values) and q-values to correct for multiple hypothesis testing, based on false discovery rate (FDR) (Storey, 2003).
Results
Bacterial community composition
Following quality filtering, bimera removal, and feature table construction with DADA2 (Callahan et al., 2016), a total of 405,185 bacterial amplicon sequence variants (ASVs) with a mean frequency of 12,662 per sample were retained for subsequent analysis. Alpha diversity decreased significantly (p < 0.01) from pre-feeding to 8 hours post-feeding (Figure 1). Alpha diversity as measured by Shannon’s diversity index averaged 5.6 pre-feeding and 5.4 post-feeding and was not significantly different between high- and low-quality forages and was also not significantly different between mineral bolus types (p>0.05). Beta diversity analysis showed Bacteroidota and Firmicutes were the predominant phyla across all treatments and represented around 80% relative abundance of the rumen community (Figure S1). PERMANOVA analysis of the bacterial community β-diversity showed significant differences in response to forage quality and sampling time but not in response to mineral bolus type (Table 3). Forage quality x sampling time interactions, corrected for multiple comparisons, were also significant (p=0.027) while forage quality x mineral bolus type interactions were only significant (p<0.05) when differences in forage quality were considered. Forage quality x time x bolus interactions were not significant (p=0.918) and thus were not included in Table 3.
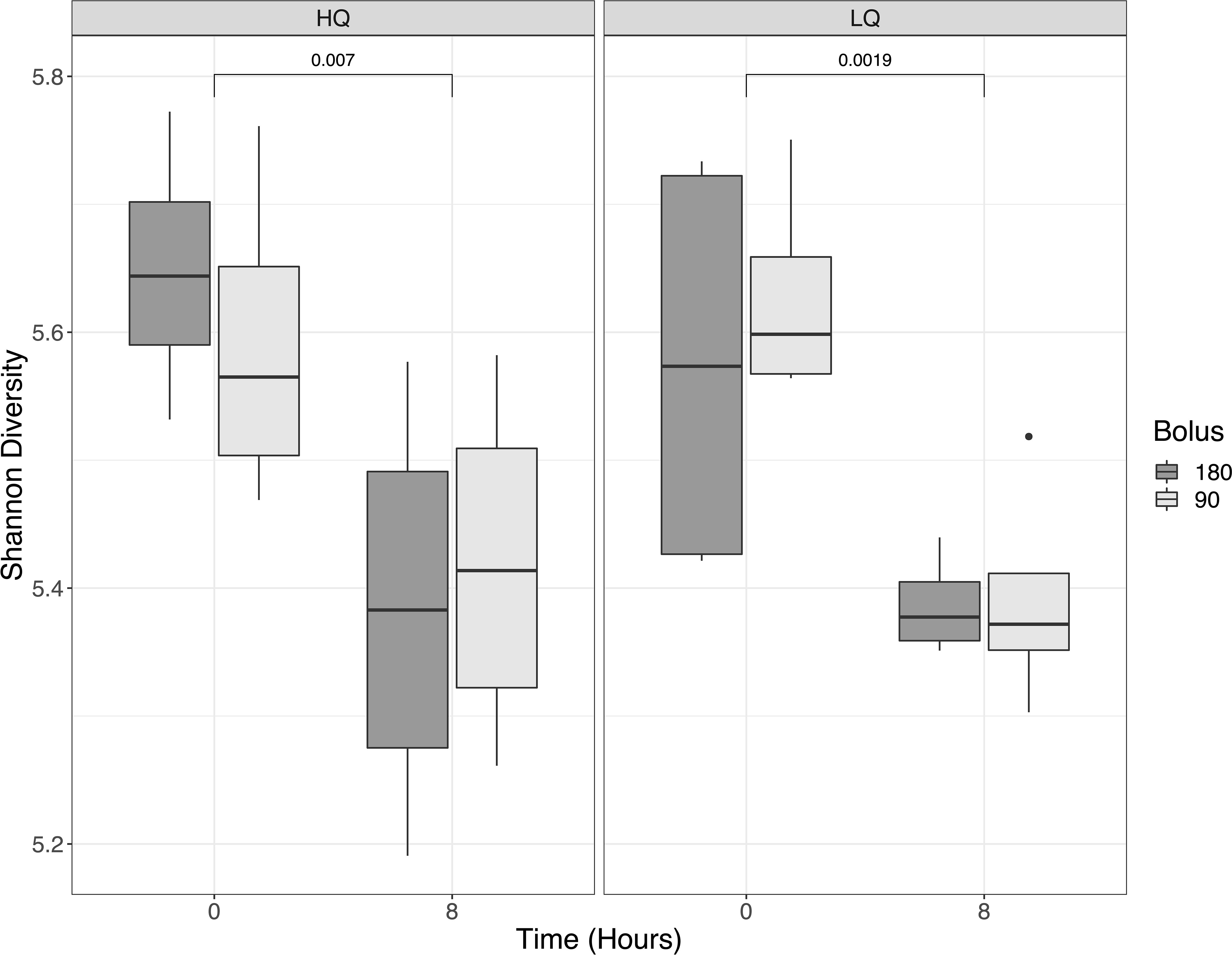
Figure 1 Rumen alpha diversity, as measured by Shannon’s diversity index, comparison of low- and high-quality forages and bolus type (90-day vs 180-day). Samples were collected pre-feeding (0 hours) and 8 hours post-feeding. Differences in Shannon’s diversity were significant pre- and post-feeding but not between feed quality or bolus type.
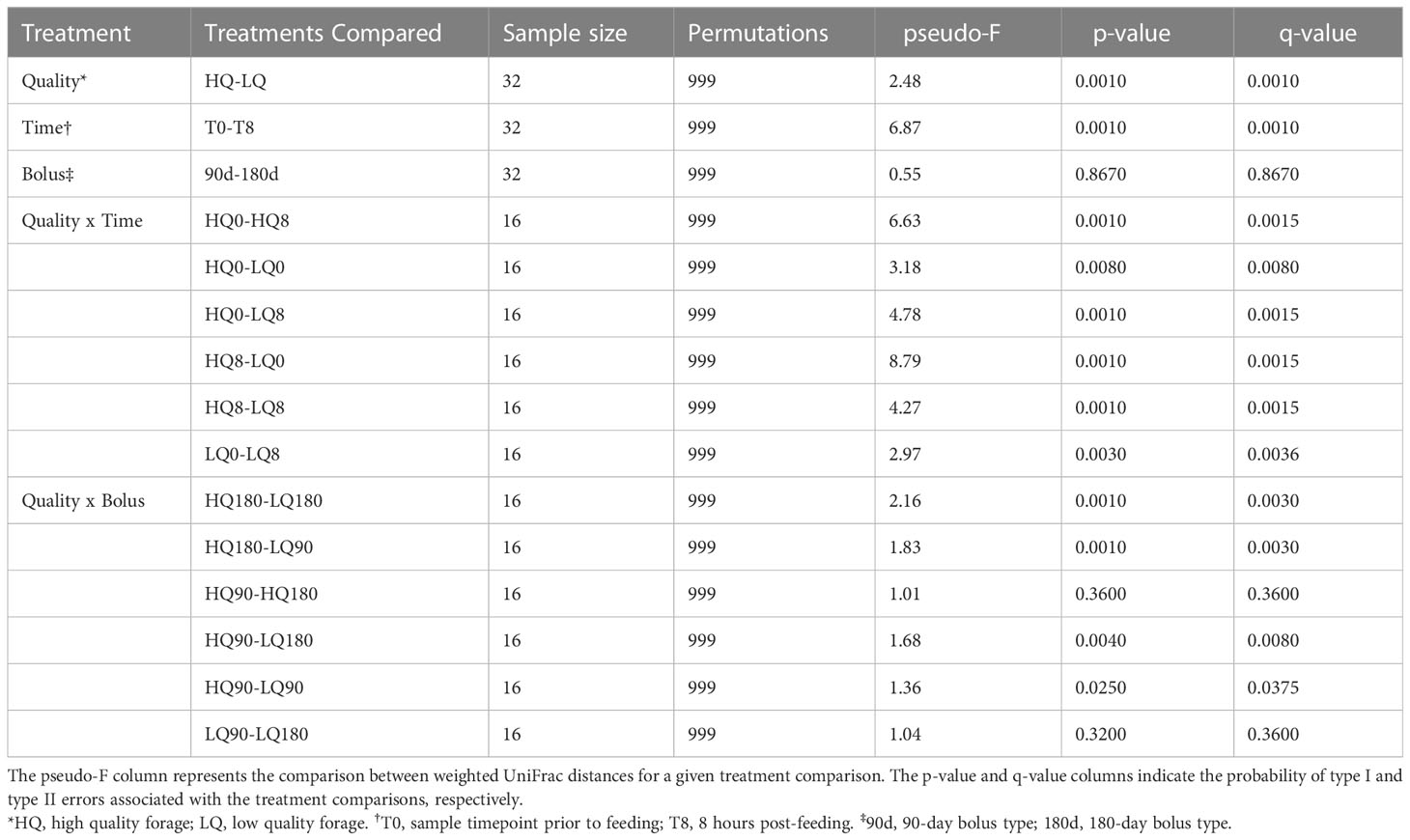
Table 3 Community structure (β-diversity) based on pairwise permanova computation of weighted UniFrac distances.
Principal coordinates analysis (PCoA) based on Bray-Curtis dissimilarity matrix revealed that 45% of the variation between bacterial communities was explained by the first two principal components. Distinct differences were observed between communities based on both feed quality and time pre- and post-feeding (Figure 2). Redundancy Analysis revealed that the bacterial community structure was associated with forage dry matter, rumen volatile fatty acids (VFAs), and rumen pH and NH3-N (Figure 3). Total VFAs, propionic acid, butyric acid, and isovaleric acid were significantly (p<0.05, FDR corrected) correlated to community structure. The relative abundance of Prevotella was positively correlated (p<0.05, FDR corrected) with high quality forage diet 8 hours post-feeding while Ruminococcus, Rickenellaceae_RC9_gut_group, and several poorly defined genera were strongly associated with the low-quality forage diet (p<0.05, FDR corrected) (Figure 3).
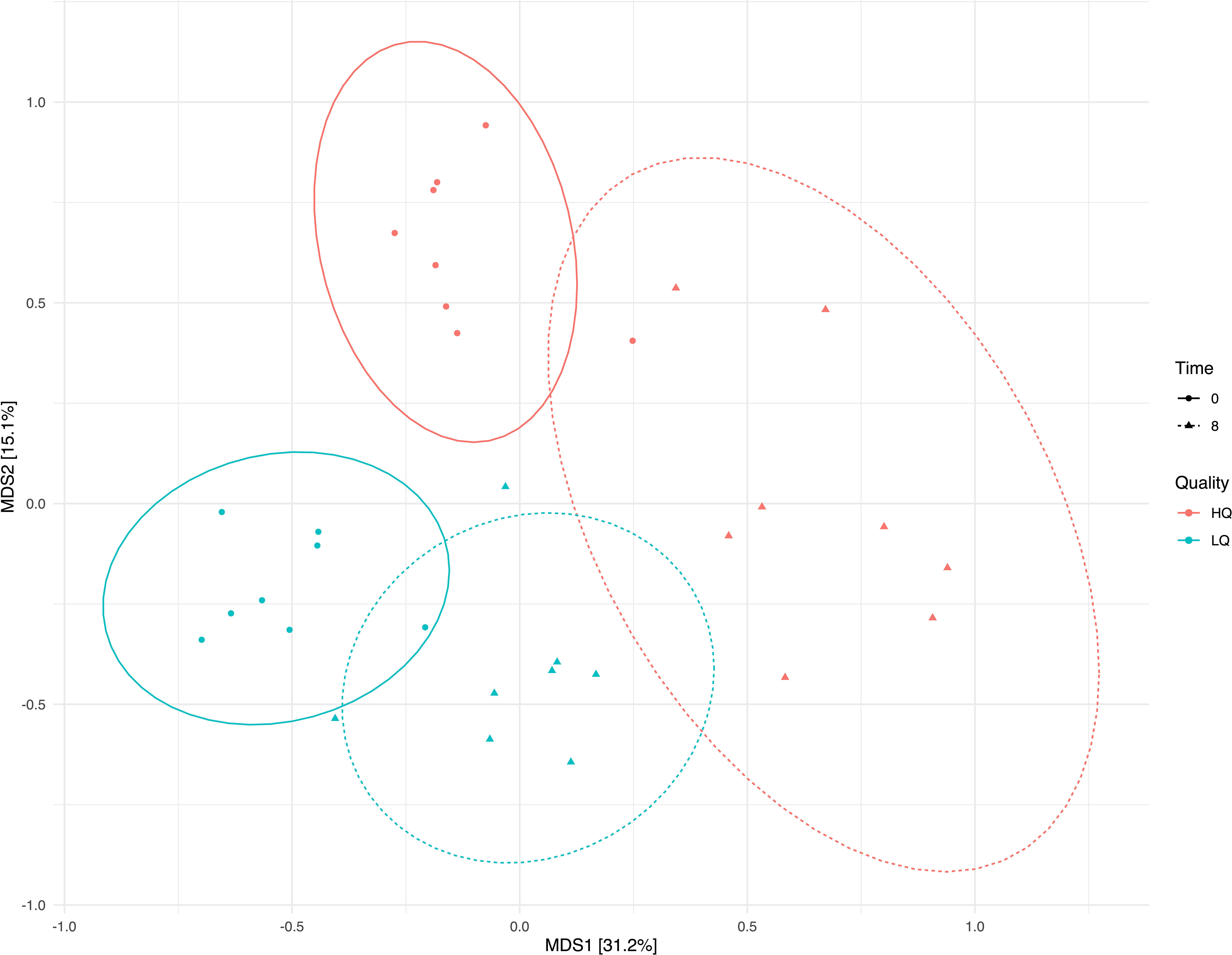
Figure 2 Principal coordinates analysis (PCoA) of the Bray-Curtis dissimilarity matrix showing sample grouping by feed quality and time. Color indicates feed quality and shape indicates sampling time; pre-feeding (0) and 8 hours post-feeding (8). Elipses show 95% confidence intervals.
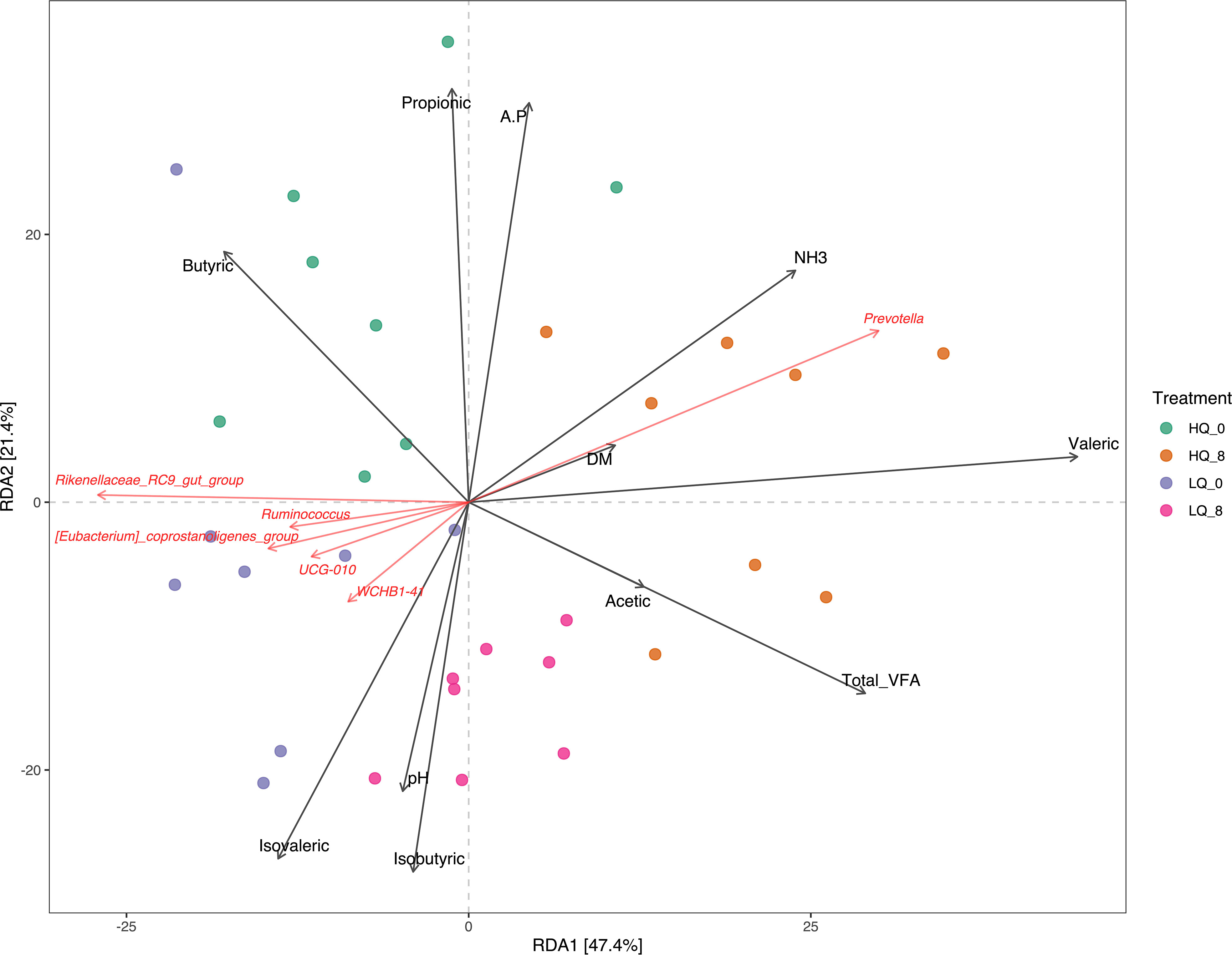
Figure 3 Redundancy analysis (RDA) showing the relationships between rumen taxa and VFAs, forage dry matter, NH3, and pH. A.P, acetate:propionate ratio; DM, forage dry matter. Taxa are shown at the genus level.
Differential abundance analysis at a family level was used to identify taxa with significant differences in relative abundance between treatments (p<0.05, FDR corrected). The analysis showed significantly higher (p<0.05, FDR corrected) relative abundance of Desulfovibrionaceae 8 hours post-feeding compared to pre-feeding (Figure 4). In general, members of the phylum Actinobacteria were more abundant pre-feeding than 8-hours post-feeding. The families Defluviitaleaceae, Saccharimonadaceae, and Selenomonadaceae were significantly (p<0.05, FDR corrected) more abundant in the high-quality forage diet compared to low quality, 8 hours post-feeding while Anaerovoracaceae and Streptococcaceae were significantly lower (p<0.05, FDR corrected). The phylum Desulfobacterota was also more abundant (p<0.05, FDR corrected) in the high-quality forage diet compared to low quality, 8 hours post-feeding.
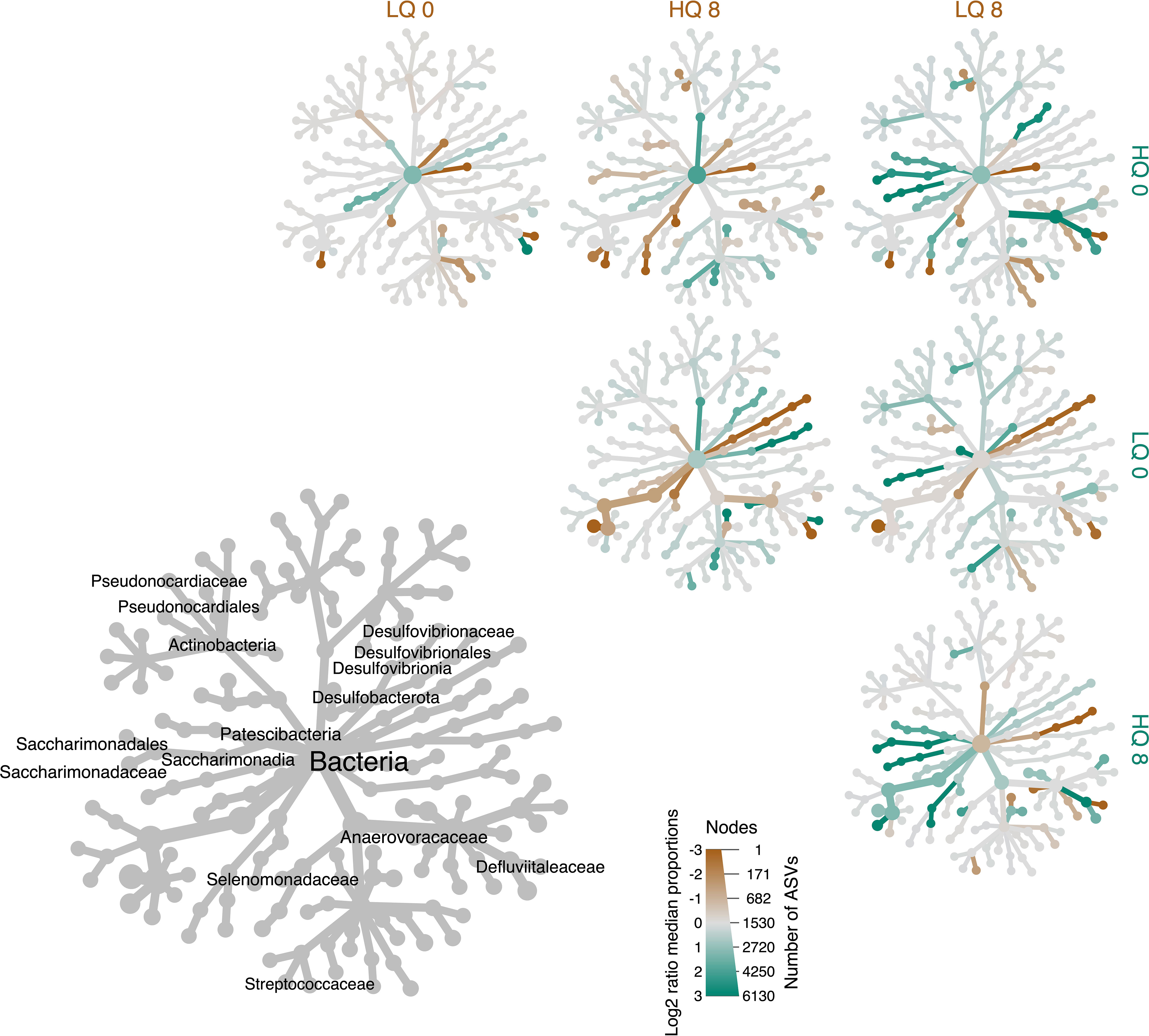
Figure 4 Heat Tree showing differential abundance of taxa at the family level between high quality (HQ) and low quality (LQ) diets and time; pre-feeding (0) and 8 hours post-feeding (8). Diameter of nodes indicates number of ASVs while color represents the Log2 ratio of median proportions. The gray tree on the lower left is a taxonomic reference for the smaller unlabeled trees which compare forage quality and time. Taxa names are shown only for taxa that were significantly different between treatments (p<0.05, FDR corrected).
Linear discriminant analysis identified 31 genera with an LDA score > 3 that were significantly (p < 0.05, FDR corrected) discriminatory among treatments (Figure 5). Eighteen taxa were discriminatory for the low-quality forage treatment while 13 taxa were discriminatory for the high-quality forage treatment. Taxa belonging to the phylum Firmicutes accounted for 50% of the discriminatory taxa for both low- and high-quality forage treatments (Figure 5A). Within this phylum, all but one taxon belonged to the class Clostridia in the high-quality forage treatment. Other taxa that were discriminatory for the high-quality forage treatment included members of the phyla Actinobacteria, Elusimicrobiota, Proteobacteria, and Mycoplasmatota. Methanosphaera, a methanogenic genus of the archaea phylum Euryarchaeota, was also discriminatory for the high-quality forage treatment. Taxa that were discriminatory for the low-quality forage treatment included members of the phyla Actinobacteria, Bacteroidota, Lentisphaerae, and Spirochaetota. The family Methanobacteriaceae of the archaea phylum Euryarchaeota, was also discriminatory for the low-quality forage treatment.
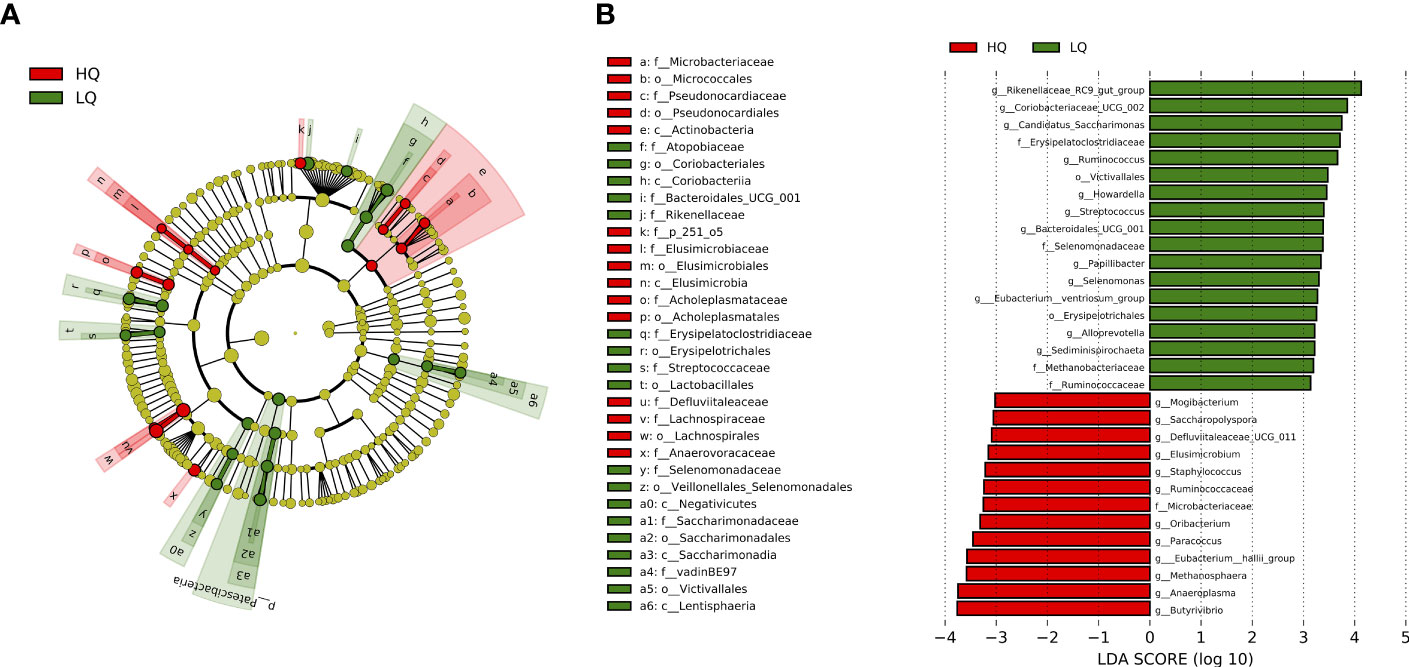
Figure 5 Visualization of unique phyla in each treatment identified using a linear discriminant analysis effect size (LEfSe) analysis. (A) cladeogram of significant (p <0.01) differentially abundant taxa. (B) Histogram of the LDA scores computed for genera differentially abundant between treatment ranked by effect size. Only taxa meeting a LDA score of >3.0 were shown. Sequence variants that were not identified at a genera level are shown at a family or order level.
Predictive functional profiling
Correlation analysis showed a significant negative (p<0.05) Spearman correlation between forage quality and predicted functional pathways associated with methanogenesis (R = -0.5) and a positive correlation with functions associated with anaerobic chemoheterotrophy (R=0.6) and fermentation (R = 0.5) (Figure 6). Mineral bolus type was not significantly correlated to any function. Sampling time was negatively correlated with methylotrophy (R = -0.5) and positively correlated to reductive acetogenesis (R = 0.6). Linear discriminant analysis (LDA) identified 9 predicted functional pathways that were significantly (p < 0.05, FDR corrected) discriminatory between treatments (Figure 7). Metabolic pathways associated with aerobic chemoheterotrophy, methanogenesis, nitrate reduction, and ureolysis were discriminatory for high quality diets. Pathways associated with chemoheterotrophy, anaerobic chemoheterotrophy, and fermentation were discriminatory for low quality diets.
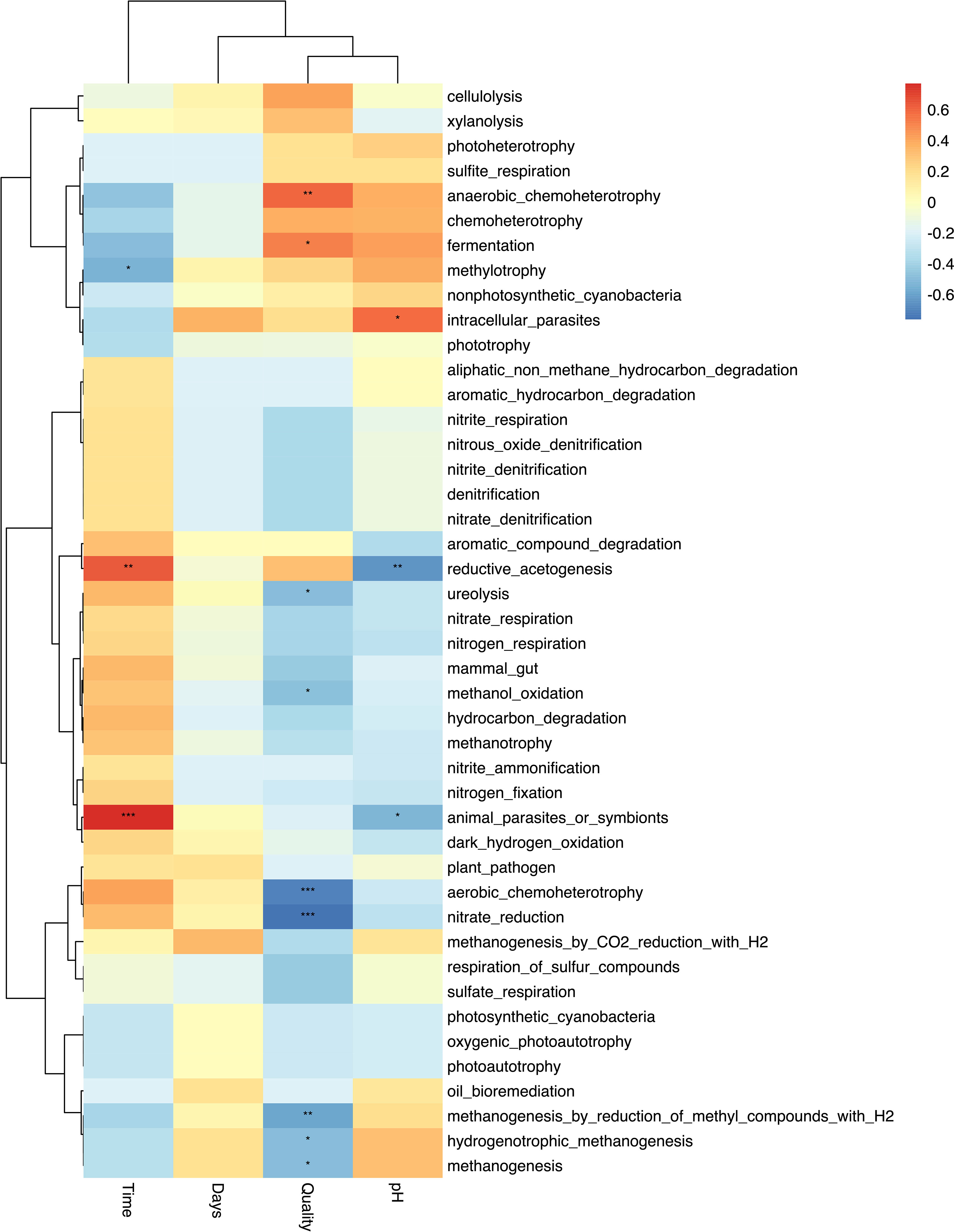
Figure 6 Correlation heat map of predicted functions correlated to diet quality (Quality), sampling time (Time), bolus type (Days), and pH. * indicates differences were significant at p<0.05; ** indicates differences were significant at p<0.01; *** indicates differences were significant at p<0.001.
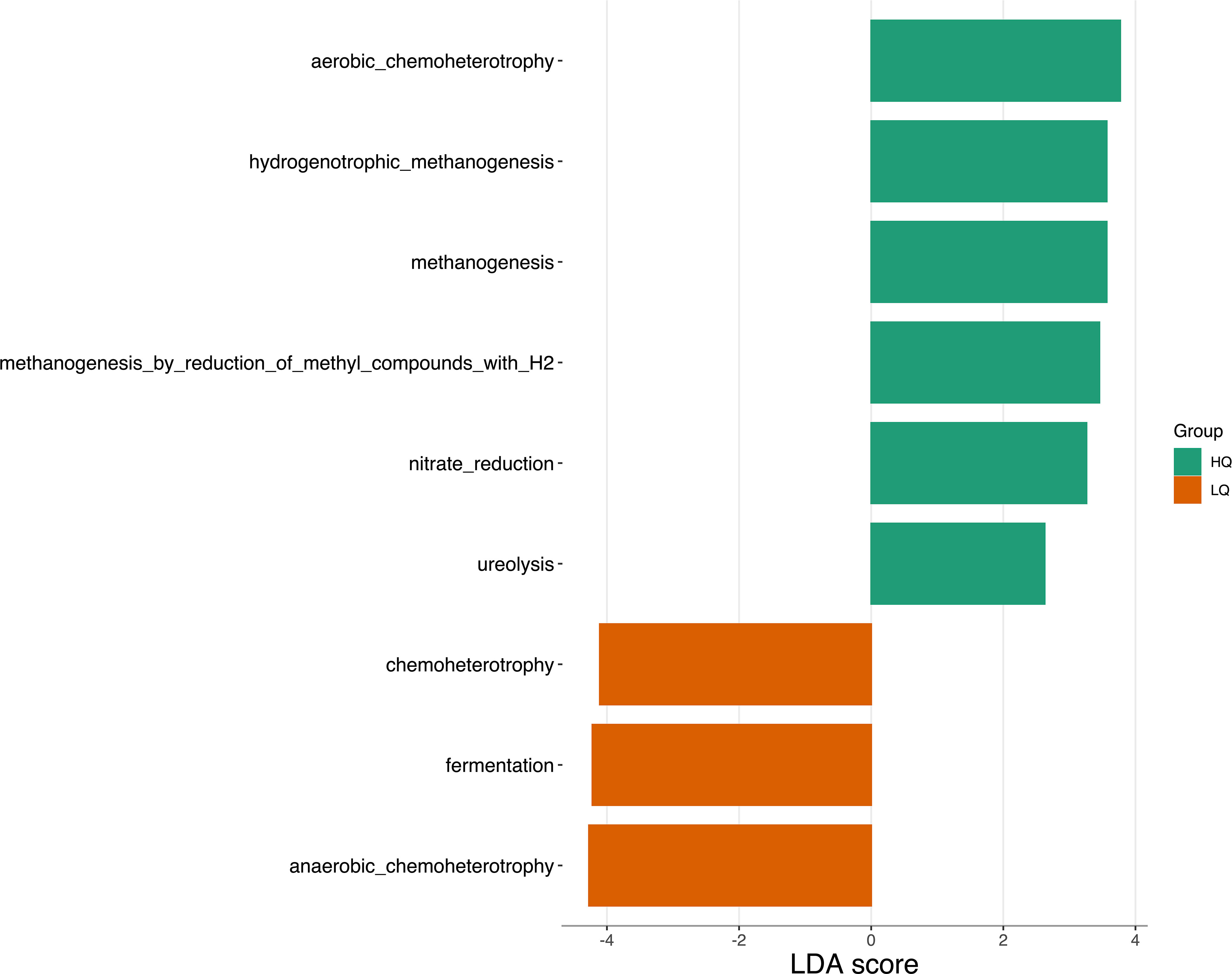
Figure 7 LEfSe analysis of metabolic pathways between high quality (HQ) and low quality (LQ) diets and time; pre-feeding (0) and 8 hours post-feeding (8). Only features with significant (p<0.01, FDR adjusted) differences between treatments and an LDA score > 2.5 are shown.
RDA revealed that the predicted microbial functions were influenced by forage dry matter, rumen volatile fatty acids (VFAs), and rumen pH and NH3-N (Figure 8). After removal of the redundant variables, 11 forage and rumen factors were selected for RDA. Of these factors, Total VFAs, propionic acid, butyric acid, and isovaleric acid were significantly (p<0.05, FDR corrected) correlated to predicted bacterial functions. Functions associated with chemoheterotrophy, anaerobic chemoheterotrophy, and fermentation were highly correlated with butyric acid within the low-quality forage diet treatments. Functions associated with animal parasites or symbionts were also correlated with total VFAs in the high-quality forage diet 8 hours post-feeding.
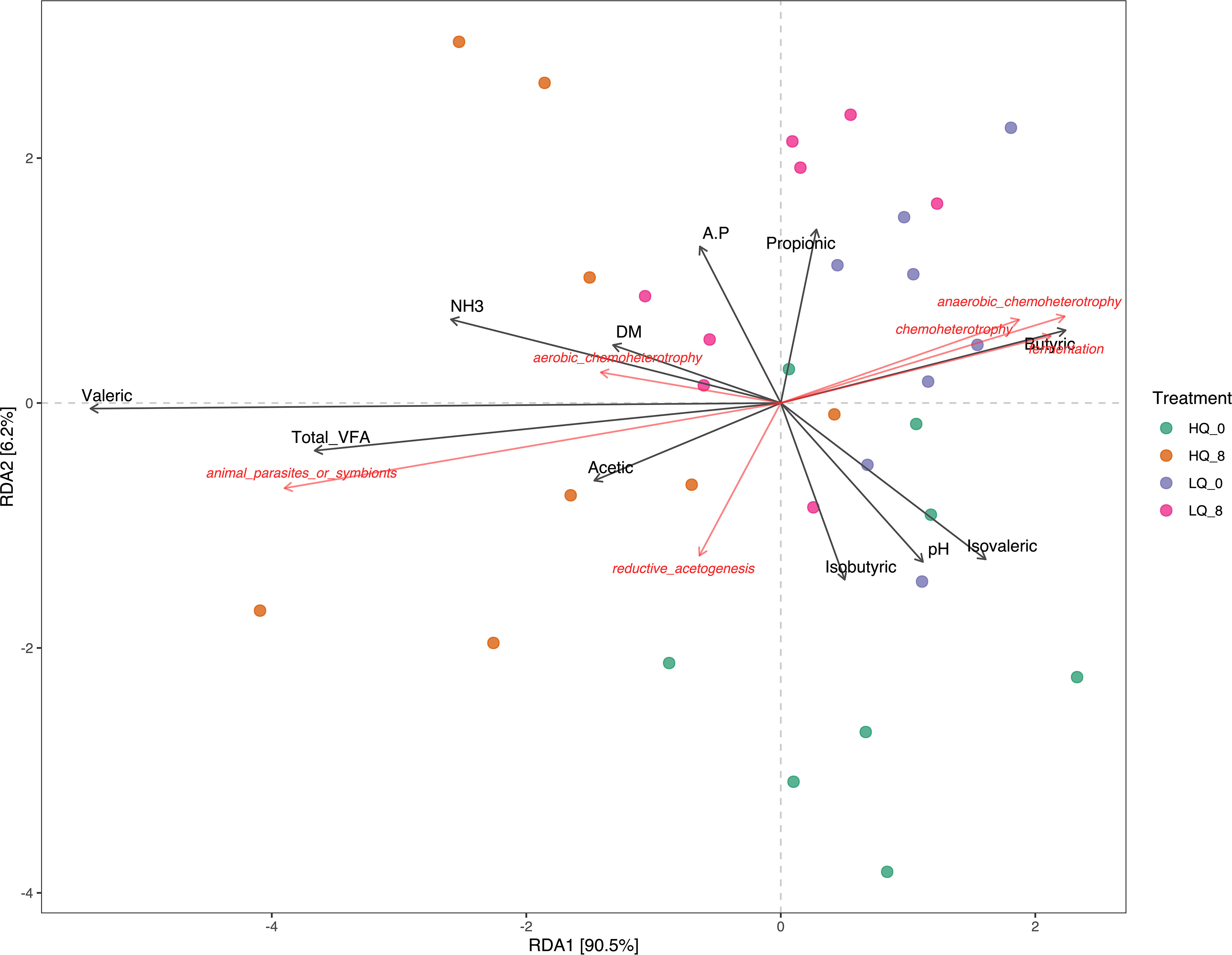
Figure 8 Redundancy analysis (RDA) showing the relationships between predicted rumen functions and VFAs, forage dry matter, NH3, and pH. A.P, acetate:propionate ratio; DM, forage dry matter.
Discussion
In this study we investigated the effects of diets consisting of alfalfa grass hay or mature wheatgrass hay in conjunction with sustained release mineral bolus supplements on the rumen microbiome in beef cattle. Previously we found that forage quality affected degradation rates of sustained release mineral boluses (Carlisle et al., 2021). Although differences were observed, the neutral detergent fiber digestibility (NDFD) for the grass alfalfa mix hay was less than the NDFD of the wheatgrass hay and levels of non-fiber carbohydrates were similar which suggests hemicellulose content was greater in the low quality diet. The differences in ruminal response may relate to the low digestibility of stems in legumes compared to stems in grasses (Soest, 1994) and intake and total tract digestion for both diets may have been limited by fiber digestion rate and ruminal fill limitations (Galyean and Defoor, 2003). Despite the lower NDFD, ruminal volatile fatty acids and ammonia differed among the two diets suggesting differing ruminal environments which was hypothesized to be related to the rumen microbiome (Carlisle et al., 2021). The results of this present work showed that the rumen bacterial community structure and function was significantly altered by forage quality but were not affected by sustained release mineral bolus type. Rumen VFA and NH3-N concentrations are important markers of rumen function (Wei et al., 2019). Redundancy analysis showed total VFAs were associated with high quality forage 8 hours post-feeding which suggests that high quality forage contributed to an increased fermentation rate in the rumen (Wei et al., 2019). VFAs are an important energy source contributing to the production performance of ruminants (Wang et al., 2020) thus, it is important to understand how forage quality and supplements effect microorganisms associated with VFA production.
Mineral bolus type was not associated with changes in the bacterial community composition or any rumen parameter. Our results are consistent with studies which have shown that mineral supplementation did not significantly alter bacterial abundances in dairy steers (Son et al., 2023) and yak (Bos grunniens) (Zhao et al., 2022). In contrast, other studies have shown a response to specific minerals. Copper (Cu), manganese (Mn), and zinc (Zn) have been linked to enhanced microbial enzyme activity (Vigh et al., 2023). Addition of (Cu) to dairy cow diets was associated with increased populations of the genera Rumminococcus and Fibrobacter (Wang et al., 2021). Another study reported no change bacterial abundance in response to Cu supplementation from 0 – 60 mg/kg DM but reduced abundance at higher concentrations (Hernandez-Sanchez et al., 2019). Other studies have shown selenium (Se) supplements lead to a reduction in total bacteria in dairy cows and increased abundance of the genera Rumminococcus, Fibrobacter, and Butyrivibrio in dairy bulls (Du et al., 2019; Liu et al., 2019; Zhang et al., 2020). Our results showed no difference in bacterial community composition between mineral boluses and thus is not likely to impact rumen function or compensate for low quality forage.
Rumen bacterial communities were predominated by members of the phyla Bacteriodota and Fermicutes which is consistent with other studies (Myer et al., 2015; Liu et al., 2016; Gharechahi et al., 2021; Zhou et al., 2021). The genus Prevotella, a member of the phylum Bacteriodota, was strongly associated with the high-quality forage diet 8 hours post feeding (Figure 3). Similar results have been observed in dairy cattle where alfalfa hay increased the abundance of Prevotella and Selenomonas compared to cows fed cornstalks (Zhang et al., 2014). Prevotella is one of the most abundant rumen genera and is crucial for degradation and utilization of polysaccharides and protein (Bohra et al., 2019). The high-quality forage had a higher protein content which may have contributed to the greater abundance of Prevotella. This contrasts with other studies which have found Prevotella is the dominant genus regardless of diet (Myer et al., 2015; Cristobal-Carballo et al., 2021). These previous studies did not consider temporal changes in Prevotella abundance with measurements collected pre-feeding (Cristobal-Carballo et al., 2021) or at the end of the feeding experiment (Myer et al., 2015) which may explain why a diet associated effect was not observed. Previous work has shown Prevotella populations peak on the surface of fiber within six hours of material entering the rumen (Liu et al., 2016). Our results are consistent with this finding and suggest forage quality may have a significant but transitory effect on Prevotella abundance.
Multiple predicted functions were correlated to rumen factors (Figure 8). One limitation of this study is that functional predictions were limited to the genus level resolution of the sequencing data. Microbial function can vary even at the species or strain level and predictive functional profiling cannot resolve strain level variations or identify functions if the pathway annotations are poor (Langille et al., 2013). Predicted functions associated with methanogenesis were negatively correlated with forage quality. This is consistent with previous findings which reported higher methane associated with low quality feed (Johnson and Johnson, 1995).
Precision nutrition and/or the optimization of rumen function depends on increased knowledge of the response of the rumen microbiome to the nutrient composition of the diet. Although previous research has demonstrated methanogenesis is negatively correlated with diet quality (Johnson and Johnson, 1995; Chagunda et al., 2010), most of the research to date is limited to comparisons of concentrate and forage-based diets. Similarly, the present study found that methanogenesis is negatively correlated with forage quality. Additionally, in this study, the rumen microbiome reflected the nutritional environment where crude protein intake was 78% greater in the high-quality diet and Prevotella was strongly associated with the higher protein alfalfa grass hay diet, and Ruminococcus was strongly associated with the lower protein, high fiber mature wheatgrass hay. These results are consistent with the literature since numerous strains of Prevotella are associated with protein and polysaccharide digestion while members of the genus Ruminococcus are associated with cellulose and hemicellulose degradation (Griswold et al., 1999; Zhang et al., 2014; Wei et al., 2021). However, since only genus level taxonomic identification was obtained in our study, these functions cannot be definitively correlated to the presence of these genera. The addition of supplemental protein to protein deficient high fiber diets likely enhances the fiber digesting microbiota in the rumen, such as Ruminococcus (DelCurto et al., 2000) suggesting a need to provide supplemental protein to cattle grazing dormant senesced vegetation or consuming high fiber protein deficient diets (straw or mature hay). There was no response of the bacterial community to the trace mineral boluses, suggesting that trace minerals were not limiting in the rumen reticular environments for both alfalfa grass or mature wheatgrass hay-based diets.
Supplementation of diets for beef cows with different sustained release mineral bolus types did not result in any changes to the rumen microbiome composition or predicted function. In contrast, forage quality altered rumen bacterial community composition and predicted function. These findings suggest that livestock managers who use supplemental minerals to increase beef cattle performance in rangeland systems can do so with minimal impact to rumen bacterial community. However, these mineral supplements cannot substitute for low forage quality, which impact the rumen microbiome composition and function. Results from this research underscore the need to evaluate forage quality for potential impacts on rumen microbial community composition and function. Optimization of the rumen reticular environment can enhance microbial community function, resulting in more efficient use of forages and nutrients available to the host animal.
Data availability statement
The datasets presented in this study can be found in online repositories. The names of the repository/repositories and accession number(s) can be found below: https://www.ncbi.nlm.nih.gov/, PRJNA945870.
Ethics statement
The animal study was reviewed and approved by Montana State University Agricultural Animal Care and Use Committee (#2019-AA12).
Author contributions
JE: Investigation, Data curation, Visualization, Writing- original draft, Writing- reviewing & editing. SW: Conceptualization, Methodology, Data collection, Writing- reviewing & editing. TC: Methodology, Data collection, Writing- reviewing & editing. TD: Conceptualization, Methodology, Supervision, Resources, Writing- reviewing & editing. All authors contributed to the article and approved the submitted version.
Funding
Funding and support were generously provided by Cargill Animal Nutrition, Montana State University Nancy Cameron Endowment, Bair Ranch Foundation, Montana Stockgrowers Association, and the Montana Agriculture Experiment Station.
Conflict of interest
The authors declare that the research was conducted in the absence of any commercial or financial relationships that could be construed as a potential conflict of interest.
Publisher’s note
All claims expressed in this article are solely those of the authors and do not necessarily represent those of their affiliated organizations, or those of the publisher, the editors and the reviewers. Any product that may be evaluated in this article, or claim that may be made by its manufacturer, is not guaranteed or endorsed by the publisher.
Supplementary material
The Supplementary Material for this article can be found online at: https://www.frontiersin.org/articles/10.3389/fanim.2023.1188874/full#supplementary-material
References
Arthington J. D., Swensont C. K. (2004). Effects of trace mineral source and feeding method on the productivity of grazing braford Cows1. Prof. Anim. Scientist 20 (2), 155–161. doi: 10.15232/S1080-7446(15)31290-0
Barnett D., Arts I., Penders J. (2021). microViz: an r package for microbiome data visualization and statistics. J. Open Source Software 6 (63), 3201. doi: 10.21105/joss.03201
Bates D., Mächler M., Bolker B., Walker S. (2015). Fitting linear mixed-effects models using lme4. J. Stat. Software 67 (1), 1–48. doi: 10.18637/jss.v067.i01
Bisanz J. E. (2018) qiime2R: importing QIIME2 artifacts and associated data into r sessions v0.99. Available at: https://github.com/jbisanz/qiime2R.
Bohra V., Dafale N. A., Purohit H. J. (2019). Understanding the alteration in rumen microbiome and CAZymes profile with diet and host through comparative metagenomic approach. Arch. Microbiol. 201 (10), 1385–1397. doi: 10.1007/s00203-019-01706-z
Bolyen E., Rideout J. R., Dillon M. R., Bokulich N. A., Abnet C. C., Al-Ghalith G. A., et al. (2019). Reproducible, interactive, scalable and extensible microbiome data science using QIIME 2. Nat. Biotechnol. 37 (8), 852–857. doi: 10.1038/s41587-019-0209-9
Callahan B. J., McMurdie P. J., Rosen M. J., Han A. W., Johnson A. J., Holmes S. P. (2016). DADA2: high-resolution sample inference from illumina amplicon data. Nat. Methods 13 (7), 581–583. doi: 10.1038/nmeth.3869
Carlisle T. J., Wyffels S. A., Stafford S. D., Taylor A. R., Van Emon M. L., DelCurto T. (2021). Evaluation of sustained release mineral boluses as a long-term nutrient delivery method for beef cattle. Anim. Feed Sci. Technol. 279, 115028. doi: 10.1016/j.anifeedsci.2021.115028
Chagunda M. G. G., Flockhart J. F., Roberts D. J. (2010). The effect of forage quality on predicted enteric methane production from dairy cows. Int. J. Agric. Sustainability 8 (4), 250–256. doi: 10.3763/ijas.2010.0490
Cristobal-Carballo O., McCoard S. A., Cookson A. L., Laven R. A., Ganesh S., Lewis S. J., et al. (2021). Effect of divergent feeding regimes during early life on the rumen microbiota in calves. Front. Microbiol. 12, 711040. doi: 10.3389/fmicb.2021.711040
DelCurto T., Hess B. W., Huston J. E., Olson K. C. (2000). Optimum supplementation strategies for beef cattle consuming low-quality roughages in the western united states. J. Anim. Sci. 77 (E-Suppl), 1–16. doi: 10.2527/jas2000.77E-Suppl1v
Du H. S., Wang C., Wu Z. Z., Zhang G. W., Liu Q., Guo G., et al. (2019). Effects of rumen-protected folic acid and rumen-protected sodium selenite supplementation on lactation performance, nutrient digestion, ruminal fermentation and blood metabolites in dairy cows. J. Sci. Food Agric. 99 (13), 5826–5833. doi: 10.1002/jsfa.9853
Foster Z. S., Sharpton T. J., Grunwald N. J. (2017). Metacoder: an r package for visualization and manipulation of community taxonomic diversity data. PloS Comput. Biol. 13 (2), e1005404. doi: 10.1371/journal.pcbi.1005404
Galyean M. L., Defoor P. J. (2003). Effects of roughage source and level on intake by feedlot cattle1. J. Anim. Sci. 81 (14_suppl_2), E8–E16. doi: 10.2527/2003.8114_suppl_2E8x
Gharechahi J., Vahidi M. F., Bahram M., Han J. L., Ding X. Z., Salekdeh G. H. (2021). Metagenomic analysis reveals a dynamic microbiome with diversified adaptive functions to utilize high lignocellulosic forages in the cattle rumen. ISME J. 15 (4), 1108–1120. doi: 10.1038/s41396-020-00837-2
Gloor G. B., Macklaim J. M., Pawlowsky-Glahn V., Egozcue J. J. (2017). Microbiome datasets are compositional: and this is not optional. Front. Microbiol. 8, 2224. doi: 10.3389/fmicb.2017.02224
Griswold K. E., White B. A., Mackie R. I. (1999). Diversity of extracellular proteolytic activities among prevotella species from the rumen. Curr. Microbiol. 39 (4), 187–194. doi: 10.1007/s002849900443
Hendawy A. O., Sugimura S., Sato K., Mansour M. M., Abd El-Aziz A. H., Samir H., et al. (2021). Effects of selenium supplementation on rumen microbiota, rumen fermentation, and apparent nutrient digestibility of ruminant animals: a review. Fermentation 8 (1). doi: 10.3390/fermentation8010004
Henderson G., Cox F., Ganesh S., Jonker A., Young W., Global Rumen Census C., et al. (2015). Rumen microbial community composition varies with diet and host, but a core microbiome is found across a wide geographical range. Sci. Rep. 5, 14567. doi: 10.1038/srep14567
Hernandez-Sanchez D., Cervantes-Gomez D., Ramirez-Bribiesca J. E., Cobos-Peralta M., Pinto-Ruiz R., Astigarraga L., et al. (2019). The influence of copper levels on in vitro ruminal fermentation, bacterial growth and methane production. J. Sci. Food Agric. 99 (3), 1073–1077. doi: 10.1002/jsfa.9274
Jackson T. D., Carmichael R. N., Deters E. L., Messersmith E. M., VanValin K. R., Loy D. D., et al. (2020). Comparison of multiple single-use, pulse-dose trace mineral products provided as injectable, oral drench, oral paste, or bolus on circulating and liver trace mineral concentrations of beef steers. Appl. Anim. Sci. 36 (1), 26–35. doi: 10.15232/aas.2019-01856
Johnson K. A., Johnson D. E. (1995). Methane emissions from cattle. J. Anim. Sci. 73 (8), 2483–2492. doi: 10.2527/1995.7382483x
Kong Y., Teather R., Forster R. (2010). Composition, spatial distribution, and diversity of the bacterial communities in the rumen of cows fed different forages. FEMS Microbiol. Ecol. 74 (3), 612–622. doi: 10.1111/j.1574-6941.2010.00977.x
Langille M. G. I., Zaneveld J., Caporaso J. G., McDonald D., Knights D., Reyes J. A., et al. (2013). Predictive functional profiling of microbial communities using 16S rRNA marker gene sequences. Nat. Biotech. 31 (9), 814–821. doi: 10.1038/nbt.2676
Liu C., Cui Y., Li X., Yao M. (2021). Microeco: an r package for data mining in microbial community ecology. FEMS Microbiol. Ecol. 97 (2), fiaa255. doi: 10.1093/femsec/fiaa255
Liu C., Li X. H., Chen Y. X., Cheng Z. H., Duan Q. H., Meng Q. H., et al. (2017). Age-related response of rumen microbiota to mineral salt and effects of their interactions on enteric methane emissions in cattle. Microb. Ecol. 73 (3), 590–601. doi: 10.1007/s00248-016-0888-4
Liu Y., Wang C., Liu Q., Guo G., Huo W., Zhang Y., et al. (2019). Effects of sodium selenite addition on ruminal fermentation, microflora and urinary excretion of purine derivatives in Holstein dairy bulls. J. Anim. Physiol. Anim. Nutr. (Berl) 103 (6), 1719–1726. doi: 10.1111/jpn.13193
Liu J., Zhang M., Xue C., Zhu W., Mao S. (2016). Characterization and comparison of the temporal dynamics of ruminal bacterial microbiota colonizing rice straw and alfalfa hay within ruminants. J. Dairy Sci. 99 (12), 9668–9681. doi: 10.3168/jds.2016-11398
Louca S., Jacques S. M. S., Pires A. P. F., Leal J. S., Srivastava D. S., Parfrey L. W., et al. (2016a). High taxonomic variability despite stable functional structure across microbial communities. Nat. Ecol. Evol. 1 (1), 15. doi: 10.1038/s41559-016-0015
Louca S., Parfrey L. W., Doebeli M. (2016b). Decoupling function and taxonomy in the global ocean microbiome. Science 353 (6305), 1272–1277. doi: 10.1126/science.aaf4507
McMurdie P. J., Holmes S. (2013). Phyloseq: an r package for reproducible interactive analysis and graphics of microbiome census data. PloS One 8 (4), e61217. doi: 10.1371/journal.pone.0061217
Myer P. R., Smith T. P., Wells J. E., Kuehn L. A., Freetly H. C. (2015). Rumen microbiome from steers differing in feed efficiency. PloS One 10 (6), e0129174. doi: 10.1371/journal.pone.0129174
Newbold C. J., Ramos-Morales E. (2020). Review: ruminal microbiome and microbial metabolome: effects of diet and ruminant host. Animal 14 (S1), s78–s86. doi: 10.1017/S1751731119003252
Okansen J., Blanchet F. G., Friendly M., Kindt R., Legendre P., McGlinn D., et al. (2019). Vegan: community ecology package (R Package). Available at: https://CRAN.R-project.org/package=vegan.
Petri R. M., Kleefisch M. T., Metzler-Zebeli B. U., Zebeli Q., Klevenhusen F. (2018). Changes in the rumen epithelial microbiota of cattle and host gene expression in response to alterations in dietary carbohydrate composition. Appl. Environ. Microbiol. 84 (12), 105–115. doi: 10.1128/AEM.00384-18
Quast C., Pruesse E., Yilmaz P., Gerken J., Schweer T., Yarza P., et al. (2013). The SILVA ribosomal RNA gene database project: improved data processing and web-based tools. Nucleic Acids Res. 41, D590–D596. doi: 10.1093/nar/gks1219
Segata N., Izard J., Waldron L., Gevers D., Miropolsky L., Garrett W. S., et al. (2011). Metagenomic biomarker discovery and explanation. Genome Biol. 12 (6), R60. doi: 10.1186/gb-2011-12-6-r60
Son A. R., Islam M., Kim S. H., Lee S. S., Lee S. S. (2023). Influence of dietary organic trace minerals on enteric methane emissions and rumen microbiota of heat-stressed dairy steers. J. Anim. Sci. Technol. 65 (1), 132–148. doi: 10.5187/jast.2022.e100
Sprinkle J. E., Cuneo S. P., Frederick H. M., Enns R. M., Schafer D. W., Carstens G. E., et al. (2006). Effects of a long-acting, trace mineral, reticulorumen bolus on range cow productivity and trace mineral profiles1. J. Anim. Sci. 84 (6), 1439–1453. doi: 10.2527/2006.8461439x
Sprinkle J. E., Schafer D. W., Cuneo S. P., Tolleson D. R., Enns R. M. (2021). Effects of a long-acting trace mineral rumen bolus upon range cow productivity. Transl. Anim. Sci. 5 (1), txaa232. doi: 10.1093/tas/txaa232
Storey J. D. (2003). The positive false discovery rate: a Bayesian interpretation and the q-value. Ann. Stat 31 (6), 2013–2035. doi: 10.1214/aos/1074290335
Tapio I., Fischer D., Blasco L., Tapio M., Wallace R. J., Bayat A. R., et al. (2017). Taxon abundance, diversity, co-occurrence and network analysis of the ruminal microbiota in response to dietary changes in dairy cows. PloS One 12 (7), e0180260. doi: 10.1371/journal.pone.0180260
Team, R. C (2021). R: a language and environment for statistical computing. r foundation for statistical computing (Vienna, Austria: R Foundation for Statistical Computing). Available at: https://www.R-project.org/.
Vigh A., Criste A., Gragnic K., Moquet L., Gerard C. (2023). Ruminal solubility and bioavailability of inorganic trace mineral sources and effects on fermentation activity measured in vitro. Agriculture 13 (4), 879. doi: 10.3390/agriculture13040879
Wang C., Han L., Zhang G. W., Du H. S., Wu Z. Z., Liu Q., et al. (2021). Effects of copper sulphate and coated copper sulphate addition on lactation performance, nutrient digestibility, ruminal fermentation and blood metabolites in dairy cows. Br. J. Nutr. 125 (3), 251–259. doi: 10.1017/S0007114520002986
Wang L., Zhang G., Li Y., Zhang Y. (2020). Effects of high Forage/Concentrate diet on volatile fatty acid production and the microorganisms involved in VFA production in cow rumen. Anim. (Basel) 10 (2), 223. doi: 10.3390/ani10020223
Wei J. Y., Wang J., Liu W., Zhang K. Z., Sun P. (2019). Short communication: effects of different selenium supplements on rumen fermentation and apparent nutrient and selenium digestibility of mid-lactation dairy cows. J. Dairy Sci. 102 (4), 3131–3135. doi: 10.3168/jds.2018-15455
Wei Z., Xie X., Xue M., Valencak T. G., Liu J., Sun H. (2021). The effects of non-fiber carbohydrate content and forage type on rumen microbiome of dairy cows. Anim. (Basel) 11 (12). doi: 10.3390/ani11123519
Zhang Z. D., Wang C., Du H. S., Liu Q., Guo G., Huo W. J., et al. (2020). Effects of sodium selenite and coated sodium selenite on lactation performance, total tract nutrient digestion and rumen fermentation in Holstein dairy cows. Animal 14 (10), 2091–2099. doi: 10.1017/S1751731120000804
Zhang R., Zhu W., Zhu W., Liu J., Mao S. (2014). Effect of dietary forage sources on rumen microbiota, rumen fermentation and biogenic amines in dairy cows. J. Sci. Food Agric. 94 (9), 1886–1895. doi: 10.1002/jsfa.6508
Zhao Z. W., Ma Z. Y., Wang H. C., Zhang C. F. (2022). Effects of trace minerals supply from rumen sustained release boluses on milk yields and components, rumen fermentation and the rumen bacteria in lactating yaks (Bos grunniens). Anim. Feed Sci. Technol. 283, 115184. doi: 10.1016/j.anifeedsci.2021.115184
Keywords: microbiome, rumen, mineral bolus, feed quality, forages
Citation: Eberly JO, Wyffels SA, Carlisle TJ and DelCurto T (2023) Rumen microbiome response to sustained release mineral bolus supplement with low- and high-quality forages. Front. Anim. Sci. 4:1188874. doi: 10.3389/fanim.2023.1188874
Received: 17 March 2023; Accepted: 09 June 2023;
Published: 23 June 2023.
Edited by:
Bianca Castiglioni, Consiglio Nazionale delle Ricerche (CNR), ItalyReviewed by:
Tao Ran, Lanzhou University, ChinaBrittany Davis, United States Department of Agriculture (USDA), United States
Copyright © 2023 Eberly, Wyffels, Carlisle and DelCurto. This is an open-access article distributed under the terms of the Creative Commons Attribution License (CC BY). The use, distribution or reproduction in other forums is permitted, provided the original author(s) and the copyright owner(s) are credited and that the original publication in this journal is cited, in accordance with accepted academic practice. No use, distribution or reproduction is permitted which does not comply with these terms.
*Correspondence: Jed O. Eberly, amVkLmViZXJseUBtb250YW5hLmVkdQ==