- 1Agriculture Victoria Research, AgriBio, Centre for AgriBioscience, Bundoora, VIC, Australia
- 2School of Applied Systems Biology, La Trobe University, Bundoora, VIC, Australia
- 3Department of Animal, Plant and Soil Sciences, La Trobe University, Bundoora, VIC, Australia
- 4School of Mathematics and Physics, University of Queensland, St. Lucia, QLD, Australia
- 5Centre for Technology Infusion, La Trobe University, Bundoora, VIC, Australia
Introduction: The automated collection of phenotypic measurements in livestock is of interest to both researchers and farmers. Real-time, low-cost, and accurate phenotyping can enhance precision livestock management and could lead to the optimized utilization of pasture and breeding of efficient animals. Wearable sensors provide the tools for researchers to develop novel phenotypes across all production systems, which is especially valuable for grazing conditions. The objectives of this study were to estimate the repeatability and heritability of traits related to grazing and rumination activities and their correlations with other traits.
Methods: This study was conducted on a commercial Merino farm in the west of Victoria, Australia, from 4 May 2020 to 29 May 2020. A total of 160 ActiGraph sensors embedded in halters were attached to the left side of the muzzles of Merino sheep (M = 74, F = 86) aged 10–11 months while the sheep were grazing on pasture. Support vector machine (SVM) algorithms classified the sensor output into the categories of grazing, rumination, walking, idle, and other activities. These activities were further classified into daily grazing time (GT), number of grazing events (NGE), grazing length (GL), rumination time (RT), number of rumination events (NRE), rumination length (RL), walking time (WT), and idle time (IT). The data were analyzed using univariate and bivariate models in ASReml-SA to estimate the repeatability, heritability, and phenotypic correlations among traits.
Results: The heritability of GT was estimated to be 0.44 ± 0.23, whereas the other traits had heritability estimates close to zero. The estimated repeatability for all traits was moderate to high, with the highest estimate being for GT (0.70 ± 0.03) and the lowest for RT (0.44 ± 0.03). The intraclass correlation or repeatability at a 1-day interval (i.e., 2 consecutive days) was high for all traits, and steadily reduced when the interval between measurements was longer than 1 week.
Discussion: The estimated repeatability for the grazing traits showed that wearable sensors and SVM methods are reliable methods for recording sheep activities on pasture, and have a potential application in the ranking of animals for selective breeding.
1 Introduction
Genetic improvement relies on there being sufficient individual phenotypic records on traits associated with breeding objectives (Greenwood et al., 2016; Cole et al., 2020). In fact, recently, the importance of high-throughput phenomics as a means of enhancing precision livestock management and optimizing the utilization of pasture, which requires detailed phenotypic data, has been gaining attention (Greenwood et al., 2016). Economically important traits in grazing livestock, such as pasture intake, feed efficiency at pasture, grazing and rumination behaviors and other key animal activities, disease resistance, and reproductive performance are difficult to measure on a large scale for the genetic improvement of grazing livestock (Greenwood and Bell, 2014; Greenwood et al., 2016). Pasture intake in growing sheep is an important driver of productivity, such as growth rate and body weight, with pasture-based systems dominating Australian sheep production. In addition, feed represents the largest variable cost of sheep production (Cammack et al., 2005); therefore, breeding for more efficient sheep could increase the profitability of farms and reduce the cost of production.
The residual feed intake (RFI) is defined as the difference between actual feed intake and predicted feed requirements for maintenance and production (Koch et al., 1963); it is an estimate used to identify efficient animals, that is, those that eat less than the predicted amount for maintenance and growth. As RFI has a moderate heritability, it is gaining in popularity in dairy cattle breeding objectives (Pryce et al., 2015) and is also of interest in sheep breeding (Johnson et al., 2022; Sepulveda et al., 2022). In these examples, dry matter intake is measured under experimental conditions, during which individual feed intakes are measured using special equipment (e.g., Muir et al., 2018). However, often these experiments are expensive to run and result in low numbers of individuals having the phenotype of interest. Therefore, there is growing interest in low-cost alternatives. This is especially true in grazing situations, where the experimental conditions are often quite different, and it may not capture variation due to feeding behavior at pasture. Knowing feed intake at pasture is therefore important in grazing situations, but it is difficult to measure this trait. Therefore, it is of interest to determine whether or not measurements for other feeding-behavior traits (e.g., grazing time, number, and length of grazing events) and rumination time can be used as indicators of feed intake. For animal breeding purposes, being able to consistently rank animals (repeatability), is as important as heritability.
There are different methods available for estimating feed intake at pasture. One method is to use chemical markers (Lee et al., 1995; Fogarty et al., 2009), which has limitations, such as those associated with dosing animals, fecal collection, laboratory extractions, and the measurement of the chemical markers. Furthermore, the implementation of this method, for a sample size that is sufficient for genomic selection, is expensive, time consuming, and requires specialized labor. The heritability of feed intake at pasture in sheep using this method was 0.32 ± 0.08 (Fogarty et al., 2009). Feed intake has also has been reported to have a positive genetic correlation with growth traits, despite large standard errors (Fogarty et al., 2009). However, sensor technology provides a rapid and accurate estimation of time spent on grazing, ruminating, and other behaviors under commercial conditions when animals graze on pasture (González et al., 2015; Greenwood et al., 2017; Almasi et al., 2022; Sohi et al., 2022). In a study by Greenwood et al. (2017), pasture intake in grazing cattle (n = 10) was estimated using an algorithm based on the time spent grazing. This estimation showed a significant correlation (r2 = 0.53), indicating that there is a moderate to high relationship between grazing time and pasture intake. Grazing time is defined as the time spent prehending, chewing, and swallowing the feed and has a similar definition to eating time in a controlled environment (Beauchemin, 2018). De Mol et al. (2016) reported that the average correlation between eating time and feed intake in dairy cows was 53% and 59% for total-mixed ration and partial-mixed ration, respectively. However, the genetic correlations between these traits may vary with differences in fiber content and sward height. This may explain why there is a large variation in correlations between eating and rumination time in dairy cows (because they often do not eat the same feed) (Zebeli et al., 2006; White et al., 2017), although there are other contributing factors, such as different measurement techniques, physical composition of diet, and inherent variability among animals (Beauchemin, 2018). In addition, the fact that there are still few studies in this area with small sample sizes could be a major driver of the variation.
Accelerometer sensor technology can provide longitudinal measurements of behavioral traits in sheep, such as grazing, ruminating, walking, or being idle. These behavioral traits have the potential to act as proxies for animal breeders, as they can provide objective and non-invasive measurements of various aspects of animal behavior. By incorporating the measurement of these behavioral traits into animal breeding programs, breeders can select individuals with desirable activity patterns, ultimately improving the productivity and profitability of the population. In a recent review, Brito et al. (2020) highlighted the importance of accurately measuring behavior, health, and production performance to improve animal welfare and productivity in commercial production systems. Therefore, the use of accelerometer sensor technology to measure behavioral traits in sheep may have the potential to enhance animal breeding programs and improve the productivity of commercial production systems.
To the best of our knowledge, a study estimating the heritability and repeatability of grazing time (GT), number of grazing events (NGE), grazing length (GL), rumination time (RT), number of rumination events (NRE), rumination length (RL), walking time (WT), and idling time (IT) using sensor technology in sheep grazing on pasture has not yet been conducted. Therefore, the aims of this study were to estimate (i) the heritability and repeatability of GT, NGE, GL, RT, NRE, RL, WT, and IT; (ii) the phenotypic correlation between these traits; and (iii) the repeatability of these traits between days.
2 Materials and methods
2.1 Animal management
All the experimental procedures of this research study were approved by the Animal Ethics Committee, La Trobe University, Victoria, Australia (AEC number 19–037). The study was conducted on a commercial farm in Edenhope, Victoria, Australia.
ActiGraph (wGT3X-BT; ActiGraph, LLC, Pensacola, FL, USA) accelerometer sensors were attached on the left side of the muzzles of Merino sheep aged 10–11 months. The experiment (on the farm) started on 4 May 2020 and was completed on 29 May 2020. Rams and ewes grazed on two different paddocks with areas of 16 and 30 ha, respectively. Both paddocks contained various plant species, with the dominant ones being Phalaris, barley grass, cape weed, and clover. In addition, the farmer assessed the pasture availability 3 weeks prior to the start of the study. The pasture availability was found to be 1,500 kg/ha in the ewes’ paddock and 2,000 kg/ha in the rams’ paddock. The details of the sheep and their feeding management were described in Almasi et al. (2022). In total, there were 160 sheep (female, n = 86; male, n = 74) with an average initial weight (± standard deviation) of 45.8 kg (± 14.0 kg) in our experiment.
2.2 Algorithms
A support vector machine (SVM)-based algorithm was developed by the Center for Technology Infusion at La Trobe University, Victoria, Australia to process the sensor data. For further details on the validation and description of algorithms, refer to Sohi et al. (2022). In brief, an SVM classifier was chosen due to the non-linear nature of the data, which meant that kernel space embeddings were appropriate. The activities of grazing sheep were classified into six categories: grazing, ruminating, walking, being idle, licking, and other behaviors. The SVM algorithms were trained using carefully labeled visual observations based on video data from field trials. The SVM classifications were based on sensor data with 5-second epochs. The algorithms used a range of additional features, where relevant, to improve the accuracy of each of the predicted classifications, such as the mean, standard deviation, kurtosis, power signal, peak-to-peak amplitude, autocorrelation, principal component analysis, and spectral analysis (Sohi et al., 2022). The concordance between the predicted and visually classified grazing, rumination, idle, and walking behaviors was 0.90 ± 0.11, 0.95 ± 0.10, 0.96 ± 0.06, and 0.93 ± 0.08, respectively (Sohi et al., 2022). Activities that did not fall into any of the previously defined categories were labeled as “unclassified” (Table 1).
2.3 Statistical analysis
The data for the predicted traits were checked to ensure that they were in logical ranges. For example, on some days the data collected showed unusually low rumination or grazing times (less than 10 minutes) for a few animals. These kinds of outlier records were scarce and could have been due to sensor malfunctions or the health of the animals. We removed such records, but we kept those for the rumination and grazing activities of the same animals on other days if they were in reasonable ranges. Variations in the behaviors between animals and across days were expected due to genetic and environmental factors. Furthermore, a single 5-second behavior was removed if it was not a continuous behavior. For example, a single 5-second grazing behavior was removed for a sheep when it was either before or after another continuous behavior.
The definition of each trait is given in Table 1. In general, grazing time was defined as the time for which an animal had its head down, and was prehending, chewing, or swallowing feed; rumination time was when an animal regurgitated, remasticated, and swallowed the digesta from the rumen; walking time encompassed the time that animals spent either walking or running; and idle time encompassed the periods when the animal was not grazing, ruminating, walking, or engaged in other activities, whether standing or lying.
The traits were analyzed using linear mixed-animal models in ASReml (Gilmour et al., 2021). The heritability and repeatability of the traits were estimated using univariate and bivariate models. We also estimated the phenotypic correlations between traits in the bivariate models. The univariate model was:
where y is a vector containing the response variable (traits described in Table 1), b is the vector of fixed effects [overall mean, sex, day of experiment (from day 1 to day 26), and initial weight as a covariate]; and u ~N(0, ), c ~N(0, ), and e ~N(0, ) are the vectors of random animal effects, permanent environment effects, and residual effects, respectively. A is the relationship matrix calculated from a pedigree of 403 animals, which was traced back for five generations, and I is the identity matrix. X, Z, and W are the design matrices relating observations to the corresponding fixed or random effects (animal or permanent environment).
The narrow-sense heritability is the ratio of additive genetic variance () to the phenotypic variance (). The repeatability of each trait was calculated by dividing the sum of and permanent environment variance () by the total phenotypic variance. Moreover, to calculate the repeatability of a trait over 2 days, or the interclass correlation between measurements for the same animals on 2 different days, the observations for just 2 days were included in the model as two traits. Repeatabilities below 0.3, between 0.3 and 0.6, and over 0.6 were considered to be low, moderate, and high, respectively.
The bivariate models included two of the traits described in Table 1:
where y1 and y2 are the vectors of phenotypic records for traits 1 and 2, respectively; b1 and b2 are the vectors of fixed effects for both traits; u1 and u2 and c1 and c2 are the vectors of random genetic effects and random permanent environment effects for traits 1 and 2, respectively. X, Z, and W are design matrices for the associated fixed and random effects. The random effects (i.e., animal, permanent environment, and residual effects) had non-zero covariances. For random animal effects:
the estimated additive genetic covariance between two traits (σu1,2) was used to calculate the genetic correlation between traits. In addition, the covariances between permanent environment effects and random residual terms were used to calculate phenotypic covariance, and, consequently, the phenotypic correlation between two traits.
3 Results
The overall mean, heritability, repeatability, and effect of initial weight on GT, NGE, GL, RT, NRE, RL, WT, and IT are presented in Table 2. The repeatabilities of NGE and GL were 0.52 ± 0.03 and 0.63 ± 0.02, respectively. RT, NRE, and RL had a moderate to high repeatability of between 0.40 and 0.50, with that of NRE being the highest (0.50 ± 0.03). The repeatability of WT was 0.49 ± 0.03, and IT had a slightly higher repeatability, of 0.56 ± 0.02 (Table 2). The heritabilities (h2 ± SE) of GT and WT were 0.44 ± 0.23 and 0.23 ± 0.17, respectively, whereas the heritability of the other traits was close to zero. The repeatabilities (r ± SE) of activities related to feeding were over 0.5, and that for GE was the highest (0.7 ± 0.03). There was a significant effect of initial weight on each trait, except for RT and RL. The effect of initial weight on GT was 3.6 minutes/kg body weight (BW), whereas for IT it was −4 minutes/kg BW (p< 0.01). The heavier animals grazed longer and idled for shorter periods of time. Furthermore, the heavier animals had fewer NGE (−1.9 minutes/kg BW), but their GL (0.02 minutes/kg BW) was longer (p< 0.01). In addition, the effect of BW on WT was statistically significant (p< 0.01), with heavier animals walking 1.4 minutes less per extra kg of BW.
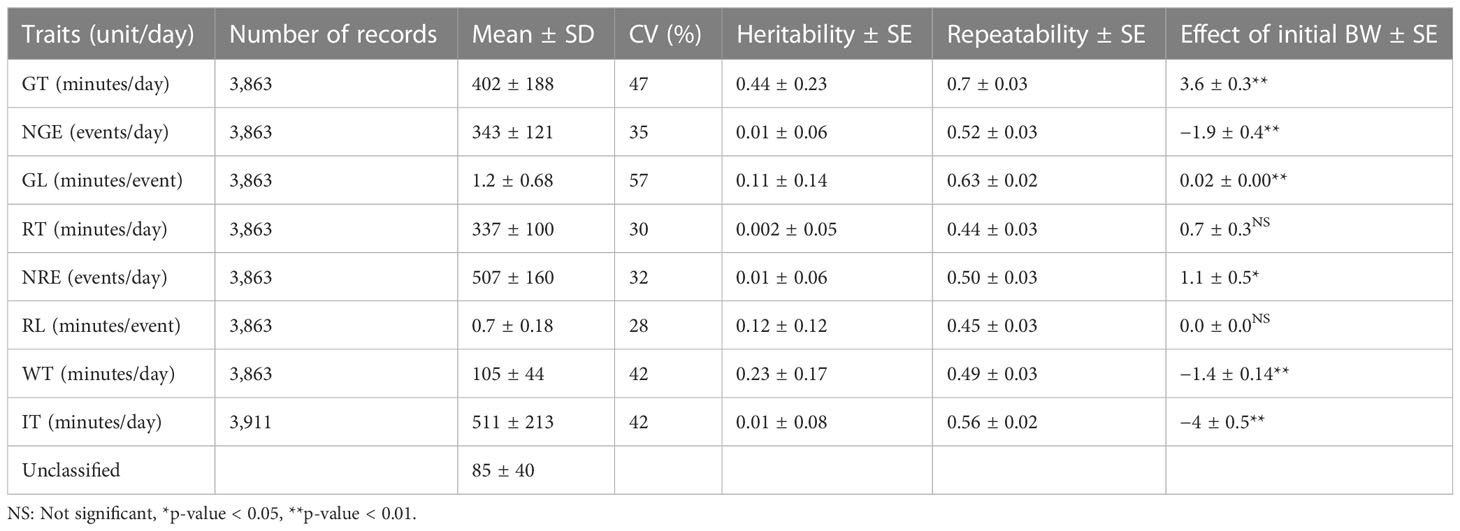
Table 2 The mean ± SD, heritability ± S.E., repeatability ± S.E., and effect of initial weight on grazing time (GT), number of grazing events (NGE), grazing length (GL), rumination time (RT), number of rumination events (NRE), rumination length (RL), walking time (WT), and idling time (IT).
The repeatability of all traits between days are illustrated in Figure 1. All activities had repeatabilities of over 0.7 when the interval between measurements was 1 day, with GT having the highest value, which was 0.83. The repeatabilities of traits related to grazing were between 0.55 and 0.7 when the interval was 1 week, and decreased to approximately 0.2 when the interval was 23 days. The repeatabilities of traits related to rumination, that is, WT and IT, were approximately 0.5 when the interval was 1 week, and decreased slightly to below 0.4 when the interval was more than 2 weeks. Interestingly, the repeatability of RL increased when the interval was over 18 days; however, the SE was large due to there being fewer observations (Figure 1B).
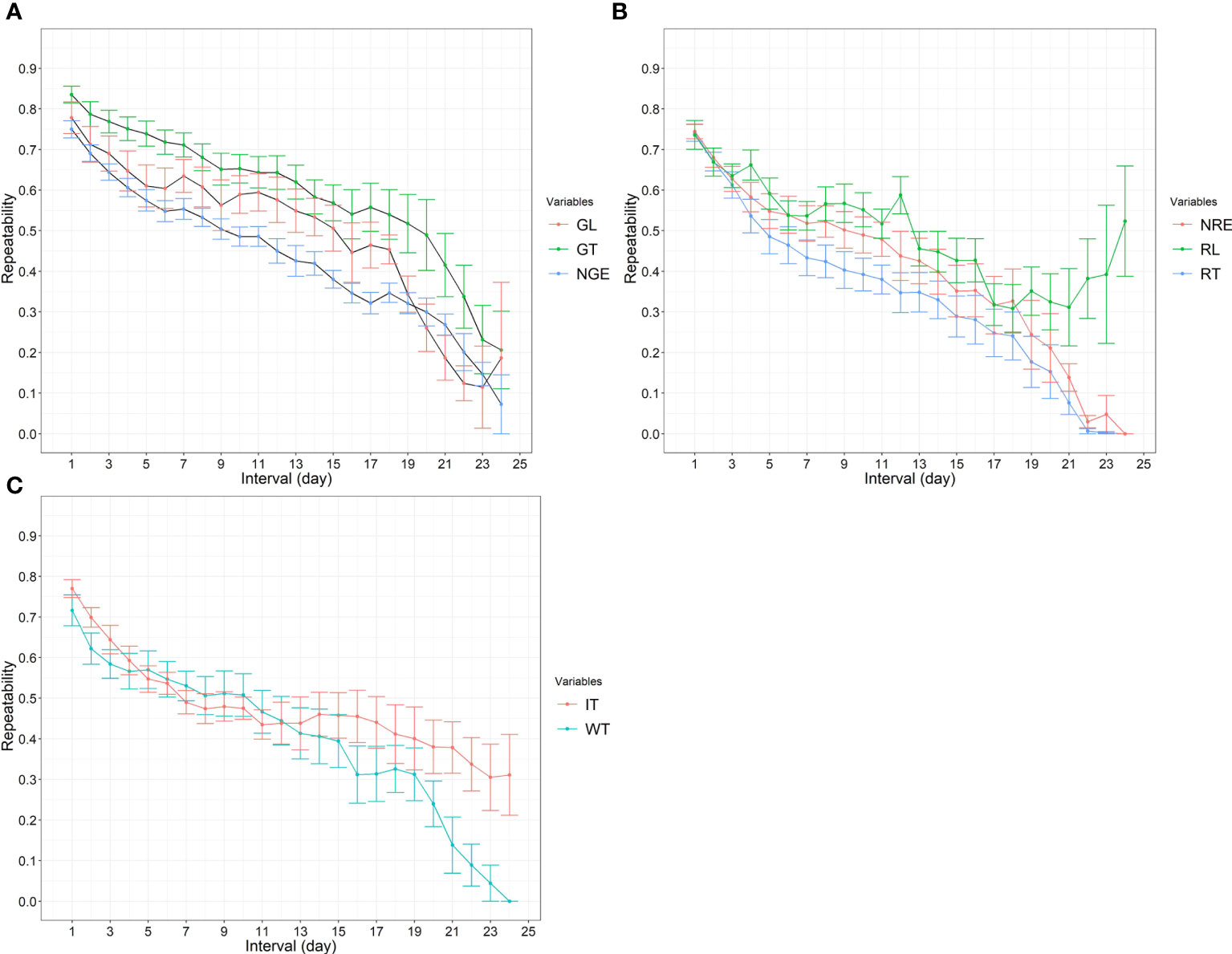
Figure 1 (A) The repeatability of grazing time (GT), number of grazing events (NGE), and grazing length (GL) between days (intervals were from 1 to 24 days). (B) The repeatability of rumination time (RT), number of rumination events (NRE), and rumination length (RL) between days (intervals were from 1 to 24 days). (C) The repeatability of walking time (WT) and idling time (IT) between days (intervals were from 1 to 24 days).
The phenotypic correlations among the traits are shown in Table 3. The phenotypic correlations between GT and NGE and GL were moderate and positive, whereas there was a negative correlation between GL and NGE (−0.53 ± 0.03). The phenotypic correlation between RT and vs. NRE and RL were strong and positive. GT had a positive phenotypic correlation with RL, but it had a moderate and negative correlation with NRE, WT, and IT.
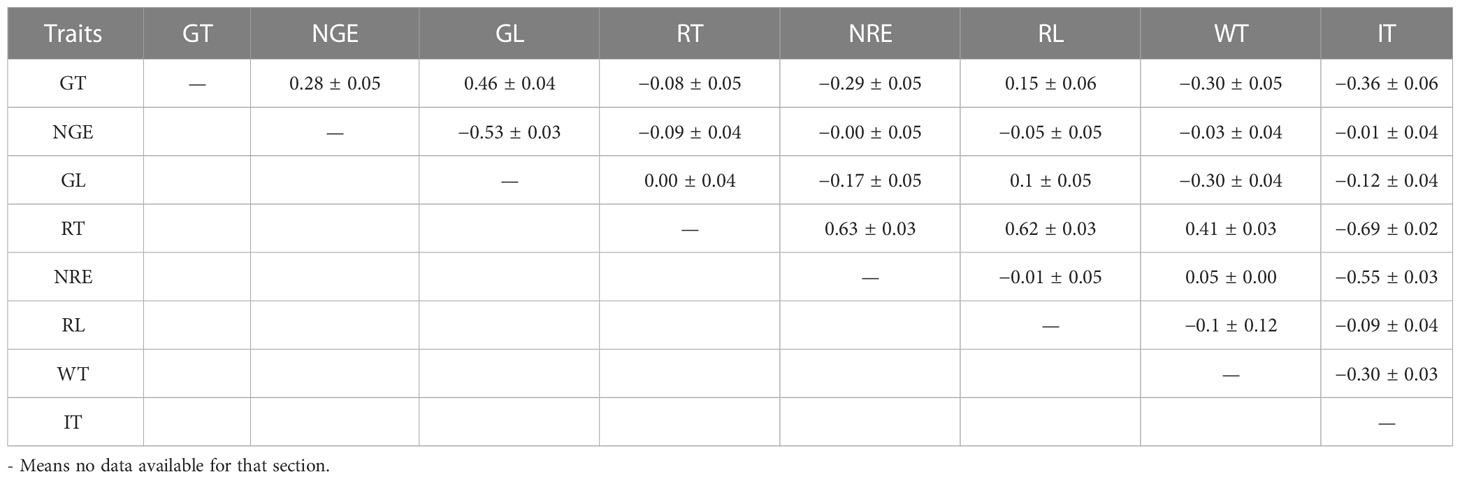
Table 3 Phenotypic correlations among grazing time (GT), number of grazing events (NGE), grazing length (GL), rumination time (RT), number of rumination events (NRE), rumination length (RL), walking time (WT), and idling time (IT).
4 Discussion
This research paper is the first to phenotype some novel traits in sheep with wearable sensors in natural grazing conditions to estimate their interactions with other traits. In this study we used wearable accelerometer devices to examine eight behaviors in 160 grazing Merino sheep from a single flock over a 26-day period. All traits were repeatable over this time period, with higher repeatabilities between consecutive days. The grazing sheep in this study had short and frequent grazing bouts, but spent, on average, 402 minutes per day grazing, 105 minutes per day walking, and 511 minutes per day being idle. In addition to providing a valuable insight into the repeatability of the behaviors of grazing sheep, this is the first research study that reports the heritability of the time spent grazing in sheep.
4.1 Feeding activities
The means of feeding behaviors in Table 2 indicate that sheep in this study had many short grazing events (343 events per day), whereas animals in an intensive farming system have fewer and longer eating events, as the occurrence at feedlot ranged from 2 to 70 events per day depending on the species (Cammack et al., 2005; Kelly et al., 2010b; Muir et al., 2018; Sepulveda et al., 2022). In the current study, animals had on average of 343 grazing events per day which implies that each grazing event would have been of a short duration (i.e., 1.2 minutes per day). Our findings are inconsistent with those of Muir et al. (2018). In our experiment, the sheep in grazing conditions tended to consume small rather than large quantities of pasture at each event. It should be noted that Muir et al. (2018) is an Australian study of sheep kept in confined conditions and fed a pelleted ration. There are no data available in grazing conditions to directly compare with our results. Muir et al. (2018) reported a strong unfavorable correlation between meal numbers and sheep feeding efficiency, meaning for that for every ewe’s additional meal, RFI increased by 0.04 kg DM/day. A similar outcome was reported in cattle, where inefficient animals had 14% to 22% more daily feeding events than efficient animals (Kelly et al., 2010a).
Although the repeatabilities of GL and NGE were estimated to be high and moderate, respectively, their heritabilities were not significantly different from zero, which could have been mainly caused by the small size of the study. However, in general, these estimates can also be affected by the length of the experiment and the interval between measurements. The heritability of GL and NGE were estimated to be approximately 0.3 in an intensive sheep farming system in Australia (Sepulveda et al., 2022). In another study, a low heritability and repeatability was reported for number of meals per day (h2= 0.09, r = 0.23) and duration of each meal (h2 = 0.14, r = 0.31) in dairy cows (Cavani et al., 2022), but when these traits were measured weekly, they increased to moderate. In the current study, when the measurements were restricted to a window of between 4 and 7 days, the results were not significantly different to the daily estimates. Kelly et al. (2010b) estimated a lower repeatability of meal length than the current study (0.37 vs. 0.63), although the estimation of feeding events was slightly higher than this study (0.60 vs. 0.52). Eating time and feeding time are two different terms that need to be carefully interpreted in research studies, as the former includes the time spent prehending, chewing, and swallowing the feed, which is similar to grazing conditions, whereas the latter consists of times spent eating meals and of inactivity (Beauchemin, 2018).
The total time spent grazing provides an indication of the amount of feed consumed daily by individuals in an extensive farming system. The mean GT recorded was 402 minutes per day, and the heritability of GT was estimated to be 0.44 ± 0.23, which is slightly higher than other studies that estimated the total eating time in a controlled environment (Cammack et al., 2005; Sepulveda et al., 2022), acknowledging the large standard error. The repeatability of GL in the current study was substantially higher (0.7 ± 0.03) than that in another study in beef cattle, in which it was reported as being approximately 0.3 (Kelly et al., 2010b; Basarab et al., 2013). Although GT was the activity least impacted by the environment, with very similar GTs being observed in the animals within the next day, the variation across studies could be due to different criteria being used to define eating time and the different techniques of measurement.
4.2 Walking and idle activity
Walking is considered to be an energy-expending activity (Richardson and Herd, 2004), and efficient animals are engaged in fewer daily feeding activities that could be due to developing energy-sparing (Kelly et al., 2010a). In addition, a positive relationship between RFI and daily feeding events was reported (Kelly et al., 2010b), which indicated that efficient animals spent more time idling, and, consequently, conserving more energy. In the current study, GL had a moderate negative phenotypic correlation (−0.3 ± 0.04) with WT, whereas the correlation between NGE and WT was close to zero. Therefore, selecting animals with a higher GL could result in selecting animals that spend less time walking and idling. We speculate that our findings suggest that selecting for GL might reduce the energy required for maintenance and potentially increase animal efficiency. This assumption is based on a higher repeatability of GL (0.63 ± 0.02; Table 2) than WT. Considering the principle of time budgeting, a fundamental aspect in animal behavior studies, this negative correlation between time traits could be attributed to the finite nature of time, 24 h or 1440 min per day. The increase in one trait necessarily implies a reduction in others due to the time constraint. However, in the complex web of animal activities, pinpointing which activity might decrease when GL increases is not straightforward.
4.3 Rumination activities
The act of rumination is well described by Beauchemin (1991). Rumination plays a key role in breaking down roughage particles to facilitate microbial colonization, which ultimately assists the passage of small particles through the lower gastrointestinal tract. In addition, rumination induces the secretion of saliva to lubricate feed particles and maintains optimum rumen pH. Therefore, increased rumination time can minimize the risk of acidosis, enhance fiber digestion, and promote a high level of feed intake. RT can be monitored and used as an indicator of health status (Stangaferro et al., 2016). The physiological and pathological aspects of rumination have been well studied (Beauchemin, 2018), but the genetic parameters have rarely been investigated. The heritability estimates of RT, NRE, and RL were close to zero, whereas the repeatability estimates were between 0.44 and 0.50 (Table 2). Two research studies in Italian and Danish Holstein dairy cows estimated the heritability of rumination time to be 0.32 (Byskov et al., 2017; Moretti et al., 2018) and the repeatability of RT over the total lactation period was estimated to be 0.75 and 0.80 for research and commercial herds, respectively (Byskov et al., 2017). RT had a negative phenotypic correlation with GT (−0.08 ± 0.05) in the current study. This negative correlation was weak and could be due to a compensatory relationship between these two traits. Moretti et al. (2018) reported a negative phenotypic correlation between RT and milk yield, protein yield, and fat yield in Holstein dairy cows. The genetic correlations among RT and protein yield and fat yield were also negative, whereas the genetic correlation of RT with milk production was positive (Moretti et al., 2018). In addition, Byskov et al. (2017) showed that RT is not a useful predictor trait for RFI. The genetic correlations among traits were estimated in the current study, but were not reported due to the large standard error, which could be because of the small sample size.
It is important to note that the high heritability of the indicator trait does not necessarily imply that there will be a highly correlated response. The efficacy of indirect selection or correlated response to selection depends on the combination of heritability of the indicator and key traits and their genetic correlation, that is, co-heritability. This term was first defined by Falconer (1960), and is the product of the genetic correlation and the square root of the heritability of both the indicator and the key traits. Therefore, the efficiency of the selection depends not only on the high heritability, but also high genetic correlation among the indicator and key traits.
4.4 Interday correlation
Strong correlations were estimated between a 1- and 7-day interval for all activity measurements (Figure 1). The results in Figure 1 imply that, although sensor technology is a reliable tool for the measurement of feeding activities, animals have similar behaviors within the same week and then the repeatability dropped to below 0.60 when the interval between measurements was over 1 week. The estimated repeatability of the traits suggests that there is a role for assessing these traits for quantitative genetic analysis. Although we acknowledge the substantial daily variation within each animal in our study, it is necessary to conduct the experiment for an adequate duration to effectively capture this daily variation within animals. In addition, it is important to note that our understanding of these novel phenotypes and their repeatability over time is limited. Nonetheless, a more optimal approach for genetic evaluation would involve a larger number of animals and would be carried out over a shorter period of time. Furthermore, it is important to estimate and understand the genetic correlations between grazing behavior traits and other economically important traits such as feed efficiency and methane emission.
5 Conclusion
In conclusion, apart from GT, the heritability of all traits was extremely low in grazing conditions. The main factors that reduced the estimated additive genetic variance could be the small sample size, and the large effect of permanent environment, short period of measurement (26 days), and low genetic connectedness between animals. However, a moderate to high repeatability implied that the sensor technology and SVM method were able to rank animals reliably in accordance with their feeding activities. However, this research was carried out under specific and limited conditions, including a particular set of livestock, pasture, and weather conditions. Therefore, the potential impact of these findings on the industry should be interpreted cautiously until further evidence is gathered to confirm their general applicability to wider contexts.
Data availability statement
The raw data supporting the conclusions of this article will be made available by the authors, without undue reservation.
Ethics statement
The animal study was reviewed and approved by the La Trobe University Animal Ethics Committee, Victoria, Australia (AEC number 19–037).
Author contributions
FA, MS, and JP contributed to the conception, and design of the study. FA, MK, and HN conducted the statistical analysis. FA wrote the first draft and sections of the manuscript. AD led the development of the ML algorithms. All authors contributed to the manuscript revision and read and approved the submitted version.
Funding
FA was financially supported by La Trobe University. JP and MK acknowledge funding from DairyBio, Dairy Australia, Agriculture Victoria, and the Gardiner Foundation.
Acknowledgments
We would like to thank Australian Wool Innovation (AWI) for lending us ActiGraph sensors. We also thank the Kealy family who allowed us to use the animals on their farm and provided support and assistance.
Conflict of interest
The authors declare that the research was conducted in the absence of any commercial or financial relationships that could be construed as a potential conflict of interest.
Publisher’s note
All claims expressed in this article are solely those of the authors and do not necessarily represent those of their affiliated organizations, or those of the publisher, the editors and the reviewers. Any product that may be evaluated in this article, or claim that may be made by its manufacturer, is not guaranteed or endorsed by the publisher.
References
Almasi F., Nguyen H., Heydarian D., Sohi R., Nikbin S., Jenvey C. J., et al. (2022). Quantification of behavioural variation among sheep grazing on pasture using accelerometer sensors. Anim. Prod. Sci. 62 (15), 1527–1538. doi: 10.1071/AN21464
Basarab J. A., Beauchemin K. A., Baron V. S., Ominski K. H., Guan L. L., Miller S. P., et al. (2013). Reducing GHG emissions through genetic improvement for feed efficiency: effects on economically important traits and enteric methane production. Animal 7, 303–315. doi: 10.1017/S1751731113000888
Beauchemin K. A. (1991). Ingestion and mastication of feed by dairy cattle. Vet. Clin. North Am. Food Anim. Pract. 7, 439–463. doi: 10.1016/S0749-0720(15)30794-5
Beauchemin K. A. (2018). Invited review: current perspectives on eating and rumination activity in dairy cows. J. Dairy Sci. 101, 4762–4784. doi: 10.3168/jds.2017-13706
Brito L. F., Oliveira H. R., McConn B. R., Schinckel A. P., Arrazola A., Marchant-Forde J. N., et al. (2020). Large-Scale phenotyping of livestock welfare in commercial production systems: a new frontier in animal breeding. Front. Genet. 11. doi: 10.3389/fgene.2020.00793
Byskov M. V., Fogh A., Løvendahl P. (2017). Genetic parameters of rumination time and feed efficiency traits in primiparous Holstein cows under research and commercial conditions. J. Dairy Sci. 100, 9635–9642. doi: 10.3168/jds.2016-12511
Cammack K. M., Leymaster K. A., Jenkins T. G., Nielsen M. K. (2005). Estimates of genetic parameters for feed intake, feeding behavior, and daily gain in composite ram lambs1,2. J. Anim. Sci. 83, 777–785. doi: 10.2527/2005.834777x
Cavani L., Brown W. E., Parker Gaddis K. L., Tempelman R. J., VandeHaar M. J., White H. M., et al. (2022). Estimates of genetic parameters for feeding behavior traits and their associations with feed efficiency in Holstein cows. J. Dairy Sci. 105, 7564–7574. doi: 10.3168/jds.2022-22066
Cole J. B., Eaglen S. A. E., Maltecca C., Mulder H. A., Pryce J. E. (2020). The future of phenomics in dairy cattle breeding. Anim. Front. 10, 37–44. doi: 10.1093/af/vfaa007
De Mol R. M., Goselink R. M. A., Van Riel J. W., Knijn H. M., Van Knegsel A. T. M. (2016). The relation between eating time and feed intake of dairy cows. Wagening Acad. Publ. Wagening Precis. Dairy Farm. 2016, 387–392.
Falconer D. S. (1960). Introduction to quantitative genetics, reprint. Eds. Oliver, Boyd (Edinburgh: Oliver and Boyd).
Fogarty N. M., Safari E., Mortimer S. I., Greeff J. C., Hatcher S. (2009). Heritability of feed intake in grazing merino ewes and the genetic relationships with production traits. Anim. Prod. Sci. 49, 1080. doi: 10.1071/AN09075
Gilmour A. R., Gogel B. J., Cullis B. R., Welham S. J., Thompson R. (2021). ASReml user guide release 4.2 functional specification (Hemel Hempstead, HP2 4TP, UK: VSN International Ltd). Available at: www.vsni.co.uk.
González L. A., Bishop-Hurley G. J., Handcock R. N., Crossman C. (2015). Behavioral classification of data from collars containing motion sensors in grazing cattle. Comput. Electron. Agric. 110, 91–102. doi: 10.1016/j.compag.2014.10.018
Greenwood P. L., Bell A. W. (2014). Consequences of nutrition during gestation, and the challenge to better understand and enhance livestock productivity and efficiency in pastoral ecosystems. Anim. Prod. Sci. 54, 1109. doi: 10.1071/AN14480
Greenwood P. L., Bishop-Hurley G. J., González L. A., Ingham A. B. (2016). Development and application of a livestock phenomics platform to enhance productivity and efficiency at pasture. Anim. Prod. Sci. 56, 1299. doi: 10.1071/AN15400
Greenwood P. L., Paull D. R., McNally J., Kalinowski T., Ebert D., Little B., et al. (2017). Use of sensor-determined behaviours to develop algorithms for pasture intake by individual grazing cattle. Crop Pasture Sci. 68, 1091. doi: 10.1071/CP16383
Johnson P. L., Hickey S., Knowler K., Wing J., Bryson B., Hall M., et al. (2022). Genetic parameters for residual feed intake, methane emissions, and body composition in new Zealand maternal sheep. Front. Genet. 13. doi: 10.3389/fgene.2022.911639
Kelly A. K., McGee M., Crews D. H., Fahey A. G., Wylie A. R., Kenny D. A. (2010a). Effect of divergence in residual feed intake on feeding behavior, blood metabolic variables, and body composition traits in growing beef heifers1. J. Anim. Sci. 88, 109–123. doi: 10.2527/jas.2009-2196
Kelly A. K., McGee M., Crews D. H., Sweeney T., Boland T. M., Kenny D. A. (2010b). Repeatability of feed efficiency, carcass ultrasound, feeding behavior, and blood metabolic variables in finishing heifers divergently selected for residual feed intake1. J. Anim. Sci. 88, 3214–3225. doi: 10.2527/jas.2009-2700
Koch R. M., Swiger L. A., Chambers D., Gregory K. E. (1963). Efficiency of feed use in beef cattle. J. Anim. Sci. 22, 486–494. doi: 10.2527/jas1963.222486x
Lee G. J., Atkins K. D., Mortimer S. I. (1995). Variation between merino ewes in pasture intake 1. between flock differences and some environmental sources of variation. Livest. Prod. Sci. 41, 133–142. doi: 10.1016/0301-6226(94)00057-E
Moretti R., de Rezende M. P. G., Biffani S., Bozzi R. (2018). Heritability and genetic correlations between rumination time and production traits in Holstein dairy cows during different lactation phases. J. Anim. Breed. Genet. 135, 293–299. doi: 10.1111/jbg.12346
Muir S. K., Linden N., Knight M., Behrendt R., Kearney G. (2018). Sheep residual feed intake and feeding behaviour: are ‘nibblers’ or ‘binge eaters’ more efficient? Anim. Prod. Sci. 58, 1459. doi: 10.1071/AN17770
Pryce J. E., Gonzalez-Recio O., Nieuwhof G., Wales W. J., Coffey M. P., Hayes B. J., et al. (2015). Hot topic: definition and implementation of a breeding value for feed efficiency in dairy cows. J. Dairy Sci. 98, 7340–7350. doi: 10.3168/jds.2015-9621
Richardson E. C., Herd R. M. (2004). Biological basis for variation in residual feed intake in beef cattle. 2. synthesis of results following divergent selection. Aust. J. Exp. Agric. 44, 431. doi: 10.1071/EA02221
Sepulveda B. J., Muir S. K., Bolormaa S., Knight M. I., Behrendt R., MacLeod I. M., et al. (2022). Eating time as a genetic indicator of methane emissions and feed efficiency in Australian maternal composite sheep. Front. Genet. 13. doi: 10.3389/fgene.2022.883520
Sohi R., Almasi F., Nguyen H., Carroll A., Trompf J., Weerasinghe M., et al. (2022). Determination of ewe behaviour around lambing time and prediction of parturition 7days prior to lambing by tri-axial accelerometer sensors in an extensive farming system. Anim. Prod. Sci. 62 (17), 1729–1738. doi: 10.1071/AN21460
Stangaferro M. L., Wijma R., Caixeta L. S., Al-Abri M. A., Giordano J. O. (2016). Use of rumination and activity monitoring for the identification of dairy cows with health disorders: part II. Mastitis. J. Dairy Sci. 99, 7411–7421. doi: 10.3168/jds.2016-10908
White R. R., Hall M. B., Firkins J. L., Kononoff P. J. (2017). Physically adjusted neutral detergent fiber system for lactating dairy cow rations. I: deriving equations that identify factors that influence effectiveness of fiber. J. Dairy Sci. 100, 9551–9568. doi: 10.3168/jds.2017-12765
Keywords: grazing time, rumination time, repeatability, phenotypic correlation, Merino sheep
Citation: Almasi F, Stear MJ, Khansefid M, Nguyen H, Desai A and Pryce JE (2023) The repeatability and heritability of traits derived from accelerometer sensors associated with grazing and rumination time in an extensive sheep farming system. Front. Anim. Sci. 4:1154797. doi: 10.3389/fanim.2023.1154797
Received: 31 January 2023; Accepted: 16 June 2023;
Published: 05 July 2023.
Edited by:
Peer Berg, Norwegian University of Life Sciences, NorwayReviewed by:
Peter Løvendahl, Aarhus University, DenmarkDean Thomas, Commonwealth Scientific and Industrial Research Organization (CSIRO), Australia
Copyright © 2023 Almasi, Stear, Khansefid, Nguyen, Desai and Pryce. This is an open-access article distributed under the terms of the Creative Commons Attribution License (CC BY). The use, distribution or reproduction in other forums is permitted, provided the original author(s) and the copyright owner(s) are credited and that the original publication in this journal is cited, in accordance with accepted academic practice. No use, distribution or reproduction is permitted which does not comply with these terms.
*Correspondence: Fazel Almasi, fazel.almasi@agriculture.vic.gov.au