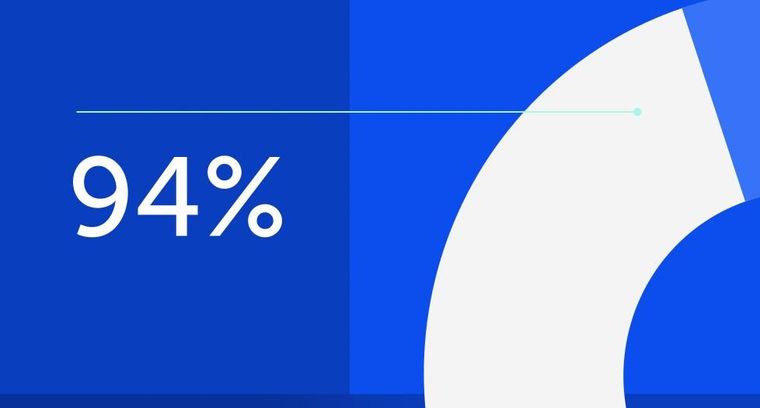
94% of researchers rate our articles as excellent or good
Learn more about the work of our research integrity team to safeguard the quality of each article we publish.
Find out more
EDITORIAL article
Front. Anim. Sci., 04 November 2022
Sec. Precision Livestock Farming
Volume 3 - 2022 | https://doi.org/10.3389/fanim.2022.1069387
This article is part of the Research TopicUnderstanding Animals' Phenotype through Automatic Behavior AssessmentView all 6 articles
Editorial on the Research Topic
Understanding animals’ phenotype through automatic behavior assessment
Animal behaviour is an elastic trait that changes according to environmental circumstances and intrinsic properties, such as health or genetic background (i.e., breed). The term behaviour elasticity refers to the range of behaviour that an animal is capable to express. The evaluation of behaviour may be used to estimate either the adaptation capacity to a changing environment or the intrinsic properties, such as health status, temperament, etc. However, in order to identify changes in behaviour, it needs to be systematically and objectively assessed. This is because some changes in behaviour are only perceptible if it is monitored continuously, which was not feasible in traditional behaviour assessment using subjective human observations.
Precision livestock farming (PLF) has emerged as a revolutionary tool to monitor different outcomes of farm animals including behaviour, physiology, functional capacity, welfare and/or performance measures. Importantly, PLF technologies have now made continuous and systematic behaviour assessment possible. Sensor technologies offer an enormous potential to monitor individual animal behaviour and to understand how and why animals react to their environment continuously. PLF sensors can be used to assess feeding, social and play behaviour and activity, from which many other phenotypical traits can be predicted and thereby create a linkage to genotypic parameters. Therefore, behavioural data can be used for many purposes including evaluation of animal’s resilience to the environment, monitoring their performance and welfare and assessing the genetic merit of breeding animals.
This Research Topic includes five papers that address the assessment of animal behaviour using methodologies capable to perform it in an automatic and systematic manner, mainly (but not exclusively) through precision livestock farming tools. As a proof of the relevance of systematic behaviour assessment in farm animals, this Research Topic includes manuscripts studying behaviour of the more relevant farm animals, including pigs (2), dairy cows (1), poultry (1) and small ruminants (1).
Also, the published 5 papers under this editorial special topic include an amalgam of behaviour assessment techniques revealing its potential. The most preeminent methodology is image analysis, including three references. Image analysis is likely one of the PLF methods that are experiencing the highest expansions, as they offer the possibility to monitor animal’s behaviour in a systematic manner, and importantly being non-intrusive.
Bonneau et al. (2022) demonstrated the application of computerised image analysis to assess changes in behaviour during a heat stress challenge in two contrasted pig breeds. Image analysis was performed using Convolutional Neural Network (CNN), a type of artificial intelligence, which serves as an example of appropriate mathematical models in analysing data from systematic evaluation, as the large data sets that may be obtained from PLF sensors.
Computer vision was also used by van der Zande et al. (2022) to estimate the resilience towards an immune challenge induced by an injection of lipopolysaccharide (LPS) in pigs. CNN were used to analyse images from 144 pigs during approximately 4600 hours, something that would not be feasible using traditional behaviour observation (i.e., human analysis).
The third study on computer vision aimed to automatically estimate goats’ location within pasture and see the possible relationship with the risk of enteric parasitic infection. As well as van der Zande et al. (2022), authors used a form of CNN model called You Only Look Once (YOLO), known to run fast, with high accuracy and high learning capacities. The use of systematic and continuous behaviour assessment allowed the authors to assess thousands of images as results were based on pictures taken every 20 seconds, nearly 12 hours a day for two weeks. However, once the machine learning model is trained, it facilitates enlarging sample size if necessary.
Besides computer vision, two studies used other techniques for behaviour assessment. The work from Fuchs et al. (2022) used accelerometers to monitor the activity of 40 dairy cows. Accelerometers are very popular in dairy cattle farms as they can estimate heat, feeding behaviour and resting, among others, which assist farmers in their daily management of the herd. A significant body of literature have already used accelerometers to monitor cattle behaviour, but their potential is still enormous, given their implementation in many commercial farms, providing huge amount of data for companies and researchers. Accelerometers in the Fuchs et al. (2022) study provided a set of 28-day length activity data that was used to evaluate changes in the circadian rhythm. Changes in circadian rhythm are a promising approach to evaluate animal behaviour as some behaviours do not change in expression “quantity” but on their pattern, which is only possible if continuous and systematic data is recorded.
Still, sensor technologies make behaviour recording easier, but systematisation may also be achieved using traditional human observations. The study from Averós et al (2022) used an app (WatchBroiler app) to systematise behaviour observations within a welfare assessment protocol. The tool was used to monitor large number of birds within a flock to monitor behaviour such as immobility, play, fighting, wing flapping and running, among others. Authors used data collected by the app to confront it against variables such as housing facilities, breeds, etc. This study demonstrates that despite behaviour not being automatically monitored, its systematic recording using software tools may facilitate data homogeneity and ultimately data accuracy.
In conclusion, this Research Topic provides some good examples on how systematic and/or automatic behaviour assessment can be used to monitor the phenotype of different species of farm animals, which is undoubtably dynamic, so this can be used to understand how animals cope with their environment. Nevertheless, none of the methods can provide a holistic assessment of the behaviour. Integration of the methodologies, a multimodality approach in combining the computer vision-based techniques along with sensors-based data, would enlarge the possibilities for automatic behaviour assessment of the farm animals for digital phenotyping.
PL, SN and CM-D conceptualized the Research Topic and collaborated for the coordination of this Research Topic. All authors agree to be accountable for the content of the work. All authors contributed to the article and approved the submitted version.
PL holds a post-doctoral fellowshisp “Ramón y Cajal” (RYC2020-029067-I) supported by the “Ministerio de Ciencia e Innovación”, Spain.
Author SN was employed by the company Farmworx Consultancy.
The remaining authors declare that the research was conducted in the absence of any commercial or financial relationships that could be construed as a potential conflict of interest.
All claims expressed in this article are solely those of the authors and do not necessarily represent those of their affiliated organizations, or those of the publisher, the editors and the reviewers. Any product that may be evaluated in this article, or claim that may be made by its manufacturer, is not guaranteed or endorsed by the publisher.
Keywords: animal behaviour, phenotype, precision livestock farming, computer vision, animal welfare, Sensors
Citation: Llonch P, Neethirajan S and Morgan-Davies C (2022) Editorial: Understanding animals’ phenotype through automatic behavior assessment. Front. Anim. Sci. 3:1069387. doi: 10.3389/fanim.2022.1069387
Received: 13 October 2022; Accepted: 26 October 2022;
Published: 04 November 2022.
Edited and Reviewed by:
Juan Steibel, Iowa State University, United StatesCopyright © 2022 Llonch, Neethirajan and Morgan-Davies. This is an open-access article distributed under the terms of the Creative Commons Attribution License (CC BY). The use, distribution or reproduction in other forums is permitted, provided the original author(s) and the copyright owner(s) are credited and that the original publication in this journal is cited, in accordance with accepted academic practice. No use, distribution or reproduction is permitted which does not comply with these terms.
*Correspondence: Pol Llonch, cG9sLmxsb25jaEB1YWIuY2F0
Disclaimer: All claims expressed in this article are solely those of the authors and do not necessarily represent those of their affiliated organizations, or those of the publisher, the editors and the reviewers. Any product that may be evaluated in this article or claim that may be made by its manufacturer is not guaranteed or endorsed by the publisher.
Research integrity at Frontiers
Learn more about the work of our research integrity team to safeguard the quality of each article we publish.