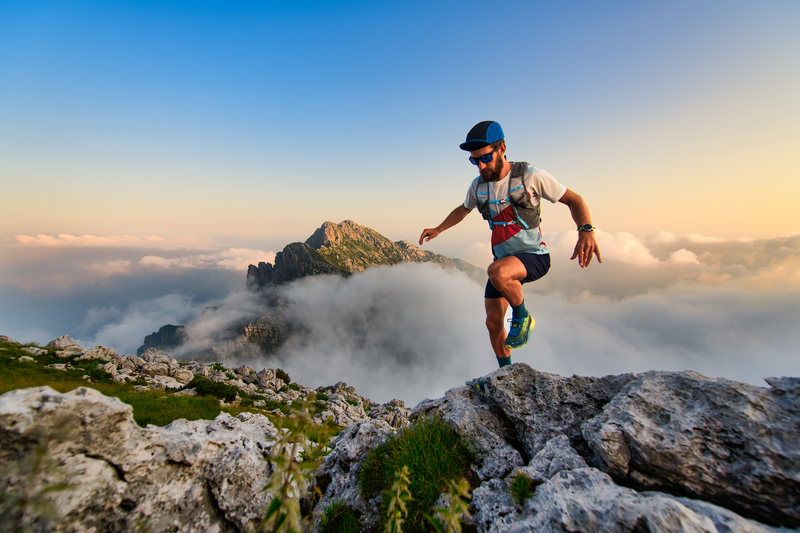
95% of researchers rate our articles as excellent or good
Learn more about the work of our research integrity team to safeguard the quality of each article we publish.
Find out more
BRIEF RESEARCH REPORT article
Front. Anim. Sci. , 07 November 2022
Sec. Precision Livestock Farming
Volume 3 - 2022 | https://doi.org/10.3389/fanim.2022.1046557
This article is part of the Research Topic Recent Advancements in Precision Livestock Farming and the Early Career Scientists Behind Them View all 4 articles
The objective of this study was to investigate the accuracy of a wearable photoplethysmography (PPG) sensor in monitoring heart rate (HR) of sheep housed in high-temperature environments. We hypothesized that the PPG sensor would be capable of differentiating low, normal, and high HR, but would struggle to produce exact HR estimates. The sensor was open source and comprised of a microprocessor (SparkFun® ThingPlus), a photoplethysmography sensor (SparkFun® MAX30101 & MAX32664), and a data storage module (SD Card 16GB), all sewn into a nylon collar with hook-and-loop closure. Sheep (n=4) were divided into 2 groups and exposed to different thermal environments in a cross-over design. The collar was placed around the neck of the sheep during the data collection phase and the manual HR were collected twice a day using a stethoscope. Precision and accuracy of numeric heart rate estimates were analyzed in R software using Pearson correlation and root mean squared prediction errors. Random forest regression was used to classify HR based on low, medium, and high to determine opportunities to leverage the PPG sensors for HR classification. Sensitivity, specificity, and accuracy were measured to evaluate the classification approach. Our results indicated that the PPG-based sensor measured sheep HR with poor accuracy and with higher average estimates in comparison with manually measured with a stethoscope. Categorical classification of HR was also poor, with accuracies ranging from 32% to 49%. Additional work is needed focusing on data analytics, and signal optimization to further rely on PPG sensors for accurately measuring HR in sheep.
Animal welfare and productivity are highly affected by heat stress (HS). Therefore, non-invasive methods of assessing animal responses to HS that can help improve livestock management and especially ensure high standards for animals’ welfare that could possibly minimize the duration of compromised welfare. Heart rate (HR) has been used as a physiological indicator of heat stress in ruminants (Marai et al., 2007) and the rapid changes in HR provide a more immediate indicator of stress (von Borell et al., 2007). However, monitoring HR precisely in livestock remains a challenge because it is not possible to visually observe HR and the most accurate measurement requires animal restraint and the usage of a stethoscope. Both restraint and physical contact with the animal may cause stress, which may, in turn, alter HR. Alternatively, the use of wearable technologies seems to be a promising strategy to provide continuous monitoring and timely diagnosis of diseases and physiological conditions of an individual animal (Neethirajan, 2017).
Photoplethysmography (PPG) sensors have been proposed as a feasible, continuous, small, low-cost, and wearable technology for HR monitoring. Unlike the electrocardiograms that rely on an electrode belt mounted around the animal’s thorax, which is not feasible under field conditions, PPG is a non-invasive method used to detect HR through detecting pulsatile shifts in volumetric blood flow by illuminating the skin using infrared light. A coupled photodetector within the sensor is then used to determine the intensity of light reflecting from the tissue. This reflectance is associated with the variations in blood perfusion of the tissue and has been used to great success in human applications for providing information on HR (Tamura et al., 2014; Nie et al., 2020). However, PPG technology has limited animal applications except for a few, highly controlled studies in pigs (Youssef et al., 2020) and cattle (Jun, Jun et al., 2013). In these studies, animals were anesthetized and shaved to create skin conditions most ideal for PPG sensing. Although promising results were obtained in this highly controlled environment, there is a need to explore how complicating factors such as the presence of hair or wool, animal motion, and housing systems influence the feasibility of use of PPG technology for monitoring animal HR.
The objective of this study was to evaluate the precision and accuracy of a PPG sensor in monitoring HR in sheep exposed to a variety of thermal conditions when HR was treated either as a continuous response (i.e., beats per minute) or a categorical response (i.e., elevated, normal, low). Although we anticipated that the PPG sensor would have poor precision and accuracy in monitoring HR of sheep due to the complicating challenges of wool and animal motion, we expected that the technology would have improved performance when classifying HR (i.e., “high”, “low”, “normal”) and might provide a tool for observing elevated HR in animals.
The sensor comprised of an Arduino-compatible microprocessor, a generic HiLetgo® MPU-9250 motion sensor which included a 3-axis accelerometer, gyroscope, and magnetometer, a PPG sensor (SparkFun® MAX30101 & MAX32664) to measure heart rate, and a data storage module (SD Card 16GB). An interchangeable battery (6700 mAh) was connected to the microcontroller by a micro-USB cord to power the sensor. The microprocessor was programmed using the open-source Arduino Development Environment (IDE) software (https://www.arduino.cc/en/Main/Software) and configured to log the data to the SD card at 100 Hz. All these components were connected and affixed to a nylon collar secured with a hook-and-loop closure. The neck region of all animals was shaved before the sensor deployment in an attempt to enhance the sensor and skin contact.
The animals and procedures in this study were approved by the Virginia Polytechnic Institute and State University Institutional Animal Care and Use Committee (Protocol #20-200). Four commercial wethers (Suffolk, Dorset or Suffolk x Dorset) approximately 5.5 years of age were used for the experiment. Animals were housed in 2 separate, thermally controlled rooms. Animals were divided into 2 groups and were housed for 20 days in these rooms. To induce heat stress the thermal environment in each room was changed five times per period with each temperature range lasting four days.
The animal’s thermoneutral zone is defined as the range in ambient temperature in which regulatory changes in metabolic heat production or evaporative heat loss are absent and considering the thermoneutral zone for sheep is within the range of 21°C to 30°C (Hemingway and Hemingway, 1966), the ambient temperature was adjusted based on that. In one room, the temperature started at the thermoneutral zone, increased to high temperature, and then was returned to the thermoneutral zone (20°C, 27°C, 35°C, 27°C, 20°C). In the other room, the animals were first exposed to the highest temperature with no gradual adaptation, the temperature was gradually reduced to the thermoneutral zone and then elevated again (35°C, 27°C, 20°C, 27°C, 35°C). After completing the 20-d progression of thermal changes in the first room, animals were moved between rooms and were exposed to the alternative set of thermal changes for the second period. The resulting cross-over design allowed for control over thermal environment patterns, room, and animal factors. Animals were adapted to each room for 7 days at a thermoneutral temperature (20°C) before beginning the experimental temperature change protocols.
Throughout the experiment, animals were fed Timothy (Phleum pratense L.) hay ad libitum. Hay allocations were replenished twice daily at 08:00 and 17:30 h and managed to ensure animals had continuous access to forage in order to mimic a typical extensive production system diet. Twice daily for the duration of the experiment, at 7:00 and 17:00, HR (beats/minute [BPM]) was measured manually by a trained technician, using a stethoscope. The HR was counted for fifteen seconds and then multiplied by four to calculate the BPM.
The sensors were configured to collect data at a 100 Hz frequency whereas manually measured HR data were collected twice daily. This resulted in considerable differences in the amount of sensed data compared with measured data. To address these differences in data quantity, only the sensed data obtained within a range of one hour before and one hour after the vital were measured remained used for analysis. This strategy was necessary to appropriately reflect the time where the HR was measured in order to make inferences about the PPG readings.
Normality was tested for the model derivation process through evaluation of residual plots, QQ-plot, and index plot. To evaluate the performance of the sensor in measuring HR as a continuous variable, precision and accuracy of the measurements compared with the sensor data were evaluated by calculating the Root Mean Square Error (RMSE) and Pearson correlation coefficient. For the correlation coefficient, a higher value (closer to 1) reflected improved precision, and for the RMSE a lower value (closer to 0) reflected improved accuracy.
Because we expected the numerical HR measurements to have poor precision and accuracy, the HR were classified as low, normal, and high based on the normal range for sheep (60 to 80 beats per minute) to evaluate whether the sensor system was appropriate for classifying abnormal HR in sheep. A random forest (RF) algorithm from the package randomForest (Liaw and Wiener, 2002) in R version 4.1.2 (R Core Team, 2021) was used to derive a random forest regression to classify animal HR.
To evaluate the system in terms of accuracy, sensitivity, and specificity, a confusion matrix was leveraged using the package caret (R Core team, 2020). The evaluation metrics were computed as follows:
Where TP Where TP (true positives) is the number of occurrences where HR was appropriately classified by the model as normal, low, or high as measured manually by the technician using a stethoscope. TN (true negatives) is the number of occurrences where HR was correctly classified as not being classified as low, normal, or high. FP (false positives) is the number of occurrences where the model incorrectly classified the HR that was not measured. FN (false negatives) is the number of occurrences where the algorithm classified a specific low, normal, or high HR as other classification
The primary purpose of this work was to evaluate the capacity of a wearable PPG sensor to detect heat-stress-induced HR changes in sheep. This technology was theorized as a low-cost and non-invasive tool to help better understand HR dynamics of animals, particularly in response to negative or stressful stimuli (Waiblinger et al., 2006). The PPG technology has been a widely used tool for monitoring human vital signs (Elgendi, 2016) due to its reliability, affordable cost, and small-scale size which facilitates wearable application. Despite these advantages being equally interesting in livestock applications, the testing of PPG-based HR sensing in animals has been limited so far.
Recently conducted PPG tests on pigs (Youssef et al., 2020), cattle (Jun et al., 2013) and dogs (Cugmas et al., 2019) showed promise for PPG use in detecting animal HR in controlled settings; however, more traditional production systems are substantially less controlled. Additionally, limitations related to the applicability of the sensing approach in these systems must be reported for future improvement. As shown in Table 1, the PPG sensor using existing HR detection algorithms had a low, negative correlation with measured HR in sheep, suggesting poor coherence between sensed and measured readings. The low correlation suggests that the sensor lacks precision, on account of the sensed values explaining minimal variation in the measured values. The poor precision could be attributable to the data density differences between the sensed and the measured data; however, more likely it reflects the sensitivity of PPG measurements to environmental interference (i.e., motion, hair). Specifically, the signal quality of PPG sensing (Orphanidou, 2018) is influenced by ambient light, movements, and peripheral perfusion which collectively can result in poor pulse prediction in general (Krishnan et al., 2010; Cugmas et al., 2019). Additionally, the low correlation between the sensed and measured HR could be attributed to a low contact pressure between the sensor and skin, which is necessary for the sensor to accurately detect HR based on changes in the volumetric blood flow (Youssef et al., 2020).
Table 1 Overall descriptive statistics when evaluating measured heart rates versus those obtained from a heart rate sensor.
The sensor measurements, when evaluated as continuous HR responses, also showed substantial mean bias, with the sensed data returning higher HR values (~88 bpm) when compared to measured HR (~ 56 bpm). However, the RMSE (36.47%) obtained suggested that the sensor has moderate error when compared with measured values. Although 36% error might be manageable in some contexts, it is outside the desirable range for accurate tools for monitoring animal wellbeing and identification of strategies to improve accuracy will be important moving forward. For example, PPG readings might be altered according to the location where the pulses are taken from. Future work should focus on exploring how site of placement can be altered to improve accuracy. Furthermore, considering that this technology has been implemented and validated in humans, the degree of skin similarity between humans and animals is likely to contribute to measurements accuracies. This skin similarity is likely why good accuracy estimates were obtained from pigs (Nie et al., 2020) in previous studies. Sheep have slightly thicker skin, averaging 2.89 mm (Alzhaxina et al., 2014), and that skin is typically covered by several mm to cm of wool. Even though the animals in this study were shaved to improve the sensor readings, the absorbance of light and capillary bed could have been impaired by the differences in thickness between sheep and humans, contributing to the notable mean bias and moderate accuracy. Although the challenges with mean bias in sensor estimation of HR must be addressed, the moderate prediction error suggests that optimization of signal processing for livestock applications, as well as optimization of sensor configuration and attachment, when combined with more intensive animal preparation approaches may result in reasonably accurate HR monitoring using PPG sensing.
To better conceptualize the application of PPG sensing to detect abnormal HR in sheep, the classification algorithm were generated using random forest regression, which is typically characterized by its accuracy and robust performance in classification tasks (Cutler et al., 2007). For example, the approach has been widely used to classify livestock behaviors with high accuracy (Barwick et al., 2018a; Barwick et al., 2018b; Mansbridge et al., 2018). In this work, the HR classification algorithm returned poor performance. The sensor could distinguish normal HR with the highest accuracy (49%; Table 2). Low and high HR were classified with 40% and 32.6% accuracy, respectively (Table 2). This classification analysis further supported the need for optimization of data interpretation algorithms, sensor design, and animal preparation before PPG sensing will be effective as a tool for monitoring livestock HR in real-time. We expect that the major challenge to address is that of sensor motion, which has been demonstrated to easily affect the quality of PPG signals (Yunjoo et al., 2008). Additionally, other studies evaluating the use of PPG sensors for HR measurements, have tested the technology on anesthetized animals, where the effects of movement and respiration of a conscious animal did not complicate readings (Cugmas et al., 2019; Youssef et al., 2020). Therefore, improved design of sensor attachment and testing of different sensing locations, which allow security to achieve a consistent contact, would be a logical first step in optimizing PPG use for real-time monitoring of livestock HR.
Considering that heat stress is a serious issue that affects animal production and wellbeing, technologies that provide continuous and real-time animal monitoring would help producers or farmers to manage and address this challenge. The purpose of our study was to evaluate whether a low-cost and open-source PPG sensor was capable of detecting HR of adult sheep, when HR changes were induced through varying the thermal environment. Despite the advantages of the sensor in terms of practicality and affordability, it demonstrated poor precision and limited capacity to detect abnormal HR in sheep, the sensor demonstrated poor precision and limited capacity to detect abnormal HR. Challenges associated with the implementation of the PPG that should be addressed in future research include sensor placement, signal optimization, and data analytics on different and larger population.
The raw data supporting the conclusions of this article will be made available by the authors, without undue reservation.
The animal study was reviewed and approved by Virginia Polytechnic Institute and State University Institutional Animal Care and Use Committee (Protocol #20-200).
All authors listed have made a substantial, direct, and intellectual contribution to the work and approved it for publication.
The authors declare that the research was conducted in the absence of any commercial or financial relationships that could be construed as a potential conflict of interest.
All claims expressed in this article are solely those of the authors and do not necessarily represent those of their affiliated organizations, or those of the publisher, the editors and the reviewers. Any product that may be evaluated in this article, or claim that may be made by its manufacturer, is not guaranteed or endorsed by the publisher.
Alzhaxina N., Begembekov K., Kulmanova G. (2014). Thickness of the skin and its layers at Degeress sheep of various stripes. In Research for Rural Development. International Scientific Conference Proceedings (Latvia University of Agriculture, Jelgava) 118–123. ISBN: 1691-4031
Barwick J., Lamb D., Dobos R., Schneider D., Welch M., Trotter M. (2018a). Predicting lameness in sheep activity using tri-axial acceleration signals. Animals 8, 12. doi: 10.3390/ani8010012
Barwick J., Lamb D. W., Dobos R., Welch M., Trotter M. (2018b). Categorising sheep activity using a tri-axial accelerometer. Comput. Electron. Agriculture 145, 289–297. doi: 10.1016/j.compag.2018.01.007
Cugmas B., Štruc E., Spigulis J. (2019). Photoplethysmography in dogs and cats: A selection of alternative measurement sites for a pet monitor. Physiol. Meas 40(1), 01NT02. doi: 10.1088/1361-6579/aaf433
Cutler D. R., Edwards T. C., Beard K. H., Cutler A., Hess K. T., Gibson J., et al. (2007). Random forests for classification in ecology. Ecology 88, 2783–2792. doi: 10.1890/07-0539.1
Elgendi M. (2016). Optimal signal quality index for photoplethysmogram signals. Bioengineering 3, 21. doi: 10.3390/bioengineering3040021
Hemingway A., Hemingway C. (1966). Respiration of sheep at thermoneutral temperature. Respiration Physiol. 1, 30–37. doi: 10.1016/0034-5687(66)90026-0
Jun H. B., Kim H. J., Kim J. O. (2013). Development of pulse measurement method for health monitoring of dairy cows. J. Korea Contents Assoc. 13, 27–37. doi: 10.5392/JKCA.2013.13.12.027
Krishnan R., Natarajan B., Warren S. (2010). Two-stage approach for detection and reduction of motion artifacts in photoplethysmographic data. IEEE Trans. Biomed. Eng 57, 1867–1876. doi: 10.1109/TBME.2009.2039568
Mansbridge N., Mitsch J., Bollard N., Ellis K., Miguel-Pacheco G., Dottorini T., et al. (2018). Feature selection and comparison of machine learning algorithms in classification of grazing and rumination behaviour in sheep. Sensors 18, 3532. doi: 10.3390/s18103532
Marai I. F. M., El-Darawany A. A., Fadiel A., Abdel-Hafez M. A. M. (2007). Physiological traits as affected by heat stress in sheep–a review. Small Ruminant Res. 71, 1–12. doi: 10.1016/j.smallrumres.2006.10.003
Neethirajan S. (2017). Recent advances in wearable sensors for animal health management. Sens. Bio-Sensing Res. 12, 15–29. doi: 10.1016/j.sbsr.2016.11.004
Nie L., Berckmans D., Wang C., Li B. (2020). Is continuous heart rate monitoring of livestock a dream or is it realistic? A Review Sensors 20, 2291. doi: 10.3390/s20082291
Orphanidou C. (2018). Signal quality assessment in physiological monitoring (Cham: Springer International Publishing). Available at: http://link.springer.com/10.1007/978-3-319-68415-4.
Tamura T., Maeda Y., Sekine M., Yoshida M. (2014). Wearable photoplethysmographic sensors–past and present. Electronics 3, 282–302. doi: 10.3390/electronics3020282
von Borell E., Langbein J., Després G., Hansen S., Leterrier C., Marchant-Forde J., et al. (2007). Heart rate variability as a measure of autonomic regulation of cardiac activity for assessing stress and welfare in farm animals — a review. Physiol. Behavior 92, 293–316. doi: 10.1016/j.physbeh.2007.01.007
Waiblinger S., Boivin X., Pedersen V., Tosi M.-V., Janczak A. M., Visser E. K., et al. (2006). Assessing the human–animal relationship in farmed species: A critical review. Appl. Anim. Behav. Science 101, 185–242. doi: 10.1016/j.applanim.2006.02.001
Youssef A., Peña Fernández A., Wassermann L., Biernot S., Wittauer E.-M., Bleich A., et al. (2020). An approach towards motion-tolerant PPG-based algorithm for real-time heart rate monitoring of moving pigs. Sensors 20, 4251. doi: 10.3390/s20154251
Keywords: sensors, heart rate, heat stress, livestock, technology
Citation: dos Reis BR and White RR (2022) Brief research report: Evaluation of photoplethysmographic heart rate monitoring for sheep under heat-stressed conditions. Front. Anim. Sci. 3:1046557. doi: 10.3389/fanim.2022.1046557
Received: 16 September 2022; Accepted: 18 October 2022;
Published: 07 November 2022.
Edited by:
Yang Zhao, The University of Tennessee, Knoxville, United StatesReviewed by:
Severiano Silva, Universidade de Trás-os-Montes e Alto, PortugalCopyright © 2022 dos Reis and White. This is an open-access article distributed under the terms of the Creative Commons Attribution License (CC BY). The use, distribution or reproduction in other forums is permitted, provided the original author(s) and the copyright owner(s) are credited and that the original publication in this journal is cited, in accordance with accepted academic practice. No use, distribution or reproduction is permitted which does not comply with these terms.
*Correspondence: Robin R. White, cnJ3aGl0ZUB2dC5lZHU=
Disclaimer: All claims expressed in this article are solely those of the authors and do not necessarily represent those of their affiliated organizations, or those of the publisher, the editors and the reviewers. Any product that may be evaluated in this article or claim that may be made by its manufacturer is not guaranteed or endorsed by the publisher.
Research integrity at Frontiers
Learn more about the work of our research integrity team to safeguard the quality of each article we publish.