- 1Livestock Production and Welfare Group, School of Life and Environmental Sciences, University of Sydney, Camden, NSW, Australia
- 2Department of Dairy Science, Faculty of Animal Science and Veterinary Medicine, Patuakhali Science and Technology University, Patuakhali, Bangladesh
- 3Allflex Australia Pty Ltd., Capalaba, QLD, Australia
- 4Thermal Ergonomics Laboratory, Faculty of Medicine and Health, School of Health Sciences, The University of Sydney, Sydney, NSW, Australia
Climate change related global warming is likely to continue, despite all mitigation measures taken by humans, due to the lag effect of long-term anthropogenic activities. Warming of the atmosphere can impact worldwide cattle production directly by compromising health, welfare and productivity, and indirectly by reducing the quality and quantity of animal feed. Under warm thermal conditions, cattle adjust their physiological and behavioural responses as an integral part of thermoregulation to maintain internal body temperature within a safe range. However, a greater intensity and duration of heat exposure can exceed thermoregulatory capacity leading to an increase in internal body temperature beyond the normal limit that ultimately evokes different animal responses to heat. In cattle, response to heat stress can be visually observed as elevated respiration rate or panting, but continuous visual monitoring is labour intensive, time consuming and subjective. Therefore, different weather-based indices have been developed such as the temperature humidity index (THI) and heat load index (HLI) which are commonly used weather-based indices for monitoring cattle heat stress at commercial level. However, the thermal comfort level of cattle based on weather-based indices has limited use at a microclimatic and individual animal level. Varying sensor-based approaches have shown promise to shift the focus of heat stress management to the individual level. Monitoring individual animal response and mitigation strategies for isolated heat-susceptible cattle could save on heat management costs whilst improving animal welfare and productivity. Here we review the technologies that enable automatic, continuous, and real-time cattle heat stress monitoring and mitigation under commercial conditions. Future platforms for autonomous monitoring and mitigation of heat stress in cattle are likely to be based on minimally-invasive smart technologies either singly, or in an integrated system, enabling real-time solutions to animal responses under various production systems and environmental conditions.
Introduction
The agricultural sector, and more specifically animal agriculture, is increasingly relying on technology to address challenges and improve efficiency under diverse climatic conditions. Technology adoption is increasing to meet the increasing global demand for animal protein with the increasing growing population in the context of climate change. This is becoming more relevant with the widespread recognition of climate change issues and increasing evidence for progressive shift in atmospheric temperature, popularly known by the term “global warming.” Climate change as defined by Zeinhom et al. (2016) is “a large-scale, long-term shift in the planet's weather patterns such as temperature, wind and rainfall characteristics of a specific region.” Climate change can affect livestock both directly and indirectly (McCarthy et al., 2001; Bernabucci et al., 2010), with the marked increase in temperature and frequency of extreme weather events resulting in an increased interest in the effects of heat stress on livestock productivity as it differs across the climatic conditions (Carabaño et al., 2016). Climate change largely affects the sustainability (Das et al., 2016) and viability (Gaughan et al., 2010) of livestock production systems around the world. The fifth assessment report of the Intergovernmental Panel on Climate Change (IPCC): AR5 [IPCC (Intergovernmental Panel on Climate Change), 2014] highlighted that (a) the warming of the climatic system is unequivocal; (b) continued emission of greenhouse gases (GHG) will cause further warming and long-lasting changes in all components of the climate system; and (c) surface air warming by the end of the 21st century based on low- and high-GHG emission scenario projections will range from 0.3 to 1.7°C and of 2.6 to 4.8°C, respectively. However, recently released sixth assessment report [IPCC (Intergovernmental Panel on Climate Change), 2021] highlighted that under all GHG emission scenarios global warming in the current century will exceed 1.5 and 2°C. In addition to increases in temperature and accompanying humidity levels, climate change models suggest that the intensity and duration of heat waves will increase [Rosenzweig et al., 2007; IPCC (Intergovernmental Panel on Climate Change), 2014] and farm animal performance and global livestock productivity may face a strong downward trend due to climate change-related global warming (Nienaber et al., 1999). This trend towards increased global average temperatures is likely to continue, as our climatic shift is the effect of long-term anthropogenic activity (Jay et al., 2021), with no immediate scaled remedy to the issue [IPCC (Intergovernmental Panel on Climate Change), 2014]. As cattle are most vulnerable to compromised production and welfare due to their high metabolic rate and poor water retention capacity under heat stress (Silanikove, 2000), cattle industries are in need of mitigation strategies such that the adverse effect of heat stress can be minimised. Animal response to heat stress become evident with altered physiological responses [increased respiration rate (RR), panting, elevated body temperature etc.] and behaviour (reduced eating, rumination, lying and increased standing, drinking, shade use etc.). The first step towards effective heat stress mitigation is to accurately monitor and quantify animal responses associated with the impact of both intrinsic and extrinsic thermal stressors.
Susceptibility to heat stress depends on an individual's intrinsic factors (such as genotype, coat colour, coat type, sex, body condition, and total mass, surface area to mass ratio, health, physiological, metabolic heat production etc.) and extrinsic factors (such as temperature, humidity, solar radiation, wind speed, cloud cover, rainfall, management etc.) (Gaughan et al., 2002). Animal heat response to heat arises from the cumulative effect of external stressors (Dikmen and Hansen, 2009), the demand made by the environment (Silanikove, 2000) leading to an imbalance between the production and dissipation of body heat (Das et al., 2016) that tends to disrupt the thermal homeostasis of an animal (depending on intrinsic factors). This causes an increase in body temperature that can either reach an elevated steady-state, or continually climb and lead to negative health outcomes. Physiological and behavioural responses are evoked to defend body temperature (Bernabucci et al., 2010) but sustained heat stress has short- and long-term physiological, behavioural, production, reproduction, and welfare impacts on cattle. The reduction of feed intake and milk yield, increase in RR (panting) and water intake, sweating, and changes in hormonal signals are immediate responses (Nardone et al., 2010) that affect responsiveness to environmental stimuli (Collier and Zimbelman, 2007). The expression of stress-related genes on acute heat exposure (like heat shock proteins, HSPs) and metabolic homeostatic regulations (Nardone et al., 2010) may impact an individual's lifetime performance (Mitlohner et al., 2001) and may be passed on to their progeny (Dreiling et al., 1991). However, the more immediate or short-term responses are key to assessing the level of thermal load to an animal. Mitigation measures to heat stress are also applied on the basis of animal response to existing thermal condition since no animal-based indicator can be reliably linked to any imminent heat stress.
Cattle production across the world is characterised by diverse management systems, geographical locations, breeds, feed and marketing systems. Irrespective of this variability, the incidence of heat stress is a common phenomenon that affects most cattle enterprises. Heat stress mitigation is a significant issue in the cattle industry not only impacting production levels for the supply chain but also for minimising animal welfare implications, which is a growing consumer and industry concern. Though the effect of heat stress is more pronounced in the tropics, temperate zones are also experiencing heat waves of short duration during summer, with the duration and frequency of such heat events likely to increase in the future [Vitali et al., 2009; IPCC (Intergovernmental Panel on Climate Change), 2014; CSIRO-BoM, 2020]. Heat stress causes significant economic losses (Busby and Loy, 1996; Mitlohner et al., 2001; St-Pierre et al., 2003; Collier and Zimbelman, 2007; Key et al., 2014) in the cattle industry by reducing production (Hahn, 1999; Kelly et al., 2001; Mitlohner et al., 2001; Seguin, 2008), compromising welfare (Kadim et al., 2004; Polsky and von Keyserlingk, 2017) and in extreme cases, by increasing the incidence of mortality (Collier and Zimbelman, 2007; Vitali et al., 2009). To combat these impacts, heat abatement measures such as provision of shade, water sprinkler and forced ventilation can be applied but these also require a significant investment in infrastructure development, and often require additional labour and energy consumption (Key et al., 2014). Advanced monitoring of the individual animal may help to reduce such costs by enabling strategic mitigation measures during heat stress conditions, and utilising the potential to enable the selection of climate resilient cattle. Animal responses to hot conditions are the most reliable indicators of the intensity and duration of thermal load, however, visual monitoring is impractical for continuous and long-term observation in commercial large-scale operations. Therefore, the cattle industry has adopted index-based heat stress thresholds that account either for weather parameters (e.g., temperature humidity index, THI) or a combination of weather parameters and animal responses (e.g., heat load index, HLI). Index-based monitoring is currently applied at herd level with limited application for individual cattle, for example, individual panting responses varied within the same cattle category with a CV greater than 80 (Islam et al., 2020). However, recent advances in automated animal monitoring technologies have shown promise to monitor heat stress in cattle accounting for individual behavioural and activity profiles (Islam, 2021). As such an understanding of the existing farming systems, climatic conditions, available technologies, current research trends and gaps is required for shifting heat stress management to an individual animal level based on autonomously-collected real-time, or near real-time, data.
The objective of this review is to identify cattle heat stress monitoring options with a particular focus on the potential of automation. Emphasis is also given on the importance of individualised monitoring as a cost-effective mitigation strategy.
Monitoring and Mitigating Heat Stress
Physiological and Behavioural Monitoring
Animals mostly alter their physiological and/or behavioural responses to stress. Therefore, an animal's response to heat stress may come in the form of physiological adaptations leading to altered behavioural organisation (Asher et al., 2009; Ratnakaran et al., 2017; Becker et al., 2020). Behaviour is a powerful tool to evaluate how an animal is coping with stressors in its environment, as it is inter-linked with intrinsic animal factors (Gaughan et al., 2002) and provides a non-invasive visual indirect indicator of wellbeing (Polsky and von Keyserlingk, 2017).
Body Temperature
Like other homeotherms, cattle need to maintain their body temperature within a narrow range to allow body cells and tissues to function optimally. The core body temperature (CBT) of homeotherms is set above the environmental temperature to increase the gradient between CBT and skin surface temperatures (ST), and thereby to increase the gradient between ST and environment such that animal can easily dissipate the extra heat produced from metabolic and physical activities. Besides the heat produced internally, cattle also take in additional heat from direct and indirect reflected radiations in the environment depending on animal's body surface temperature. It has been reported that non-evaporative heat dissipation cease (around ST of 37°C) or become less effective at ambient temperatures above 29–32°C (Allen, 1962; Hansen, 2004). Cattle CBT is normally 38.6°C with a diurnal fluctuation of ±0.5°C depending on environmental temperature, with CBT peaking in the early evening and reaching a minimum in the early morning [Meat and Livestock Australia (MLA), 2006; Burdick et al., 2012; Liu et al., 2019]. The ST is normally slightly lower than CBT which allows body heat to transfer from core to surface that works as heat transfer interface between environment and core of body. If ambient temperature is lower than ST, then excess body heat dissipates and CBT remains within safe range. However, when the difference between ST and surrounding temperature becomes narrowed or environmental temperature is above the set point ST, heat transfer cease or occurs in the opposite direction and CBT rises. The breakdown of the reciprocal cross inhibition between heat production and heat loss effector pathways (Bligh, 2006) may lead to prolonged elevations in CBT above tolerance levels that may cause damage of body tissues and organs and even morbidity (Leon and Helwig, 2010). In this regard, a cattle CBT of greater than 41°C can be lethal (Findlay, 1958; Vermunt and Tranter, 2011).
The body temperature (either core or surface temperature) of cattle can be used as an indicator of heat stress, however, surface temperature may not be a true representation of the core temperature. Measurement of cattle body temperature is impacted by the anatomical location of the site including the tympanic membrane (Bergen and Kennedy, 2000; Davis et al., 2003), rectum (Lea et al., 2008), vagina (Burdick et al., 2012; Lees A. M. et al., 2018), reticulo-rumen (AlZahal et al., 2011), abdomen (Gaughan and Mader, 2014), skin surface (Kou et al., 2017); and method of measurement (Ammer et al., 2016) namely manual thermometer, infrared thermography (Luzi et al., 2013; Paim et al., 2018), radio telemetry (Araki et al., 1984; Lefcourt and Adams, 1996; Brown-Brandl et al., 2003a) or temperature data logger (Lea et al., 2008; Reuter et al., 2010; Burdick et al., 2012; Scanavez et al., 2017; Lees A. M. et al., 2018). Traditionally, rectal temperature (RT) has been considered as a robust indicator of CBT but measuring RT continuously is affected by defecation, and keeping the temperature sensor/probe in place for a considerable duration of time is unfeasible (Lea et al., 2008). Vaginal temperature is highly associated with RT (Cvetkovic et al., 2005; Lees A. M. et al., 2018) and empty controlled internal drug release (CIDR) devices with attached temperature data loggers have been used for short term CBT monitoring (Cvetkovic et al., 2005; Kendall et al., 2008; Vickers et al., 2010; Burdick et al., 2012; Lees A. M. et al., 2018) but is limited to female cattle. Logger failures (Wolaver and Sharp, 2007; Roznik and Alford, 2012; Lees A. M. et al., 2018; Lees et al., 2020) have been reported in experiments with CIDRs incorporating temperature loggers without sealing/waterproofing. In this regard, sealing of temperature loggers can protect against data loss due to prolonged exposure to wet conditions (Roznik and Alford, 2012); however, sealing temperature loggers with different coatings (Burdick et al., 2012; Roznik and Alford, 2012; Scanavez et al., 2017) may pose difficulty of removing the coatings at the end of data collection and may make the CIDR inappropriate for repeated use. As such, a simple and low-cost sealing technique applicable at field level is required.
Indwelling temperature data logging devices have been used relying on the accuracy as specified by the manufacturer in some studies (Verwoerd et al., 2006; Reuter et al., 2010) and in others validated against a certified thermometer (Cvetkovic et al., 2005; Vickers et al., 2010) or data logger placed in the same or alternative anatomical position within an animal (Lea et al., 2008; Lees A. M. et al., 2018). As temperature measurements can vary based on type of logger and its modification (Lea et al., 2008; Roznik and Alford, 2012; Tresoldi et al., 2020), expertise of human handlers, and sites of measurement, modified temperature data loggers should be validated under controlled in-vitro conditions simulating cattle CBT.
The measurement of CBT by an implanted temperature data logger with transmitter in the abdominal cavity of steers (Brown-Brandl et al., 2003a) and CBT data from other research work (Scott et al., 1983; Hahn, 1999; Mader, 2003; Brown-Brandl et al., 2005a) revealed that a change in CBT was dependent on ambient conditions and lagged ambient temperature by 1–5 h. However, mammals can maintain a relatively constant CBT independent of the immediate environment (Blackshaw and Blackshaw, 1994) and such a lag time indicates that elevated CBT at a point of time is indicative of heat stress 1–5 h before that measurement. This previous work did not consider the common diurnal and seasonal pattern in the time series data of ambient thermal conditions and CBT. The analyses of such raw data may result in spurious associations due to cross-correlations between series having a common pattern. The removal of diurnal and long-term patterns from both time series and the use of the residuals of both series could assist to establish a causal relationship (Verwoerd et al., 2006). Also, the variability between individuals in heat-tolerance and heat-susceptibility may play a significant role in this association. The problem with monitoring body core or surface temperature is that current technologies are not practical or suitable for constant monitoring of individual cattle in large herds over a long duration due to the limitations of memory to store the data, battery life, short range of communication and cost. Moreover, most CBT monitoring cannot not be performed in real time and only suitable for research purpose with limited availability for commercial decision making.
Metabolic and Endocrine Profiling
Monitoring metabolic and endocrine changes can help detect stress events, as heat stress responses at the blood level may precede visible behavioural or physiological changes. Plasma cortisol concentration increased significantly from the baseline value in Holstein steers exposed to a temperature of 42°C for 160 min, and the concentration dropped significantly within 5 min after the cessation of heat exposure (Abilay et al., 1975). Significant positive correlations were also reported in the same study for environmental temperature and RT to plasma cortisol concentration. Though it is difficult to differentiate the effect of direct heat stress and the indirect effects of reduced dry matter intake, some other hormonal changes have been attributed to heat stress. A reduction in plasma somatotropin (McGuire et al., 1991), triiodothyronine and thyroxine (Magdub et al., 1982) occurred in cows exposed to high ambient temperatures but triiodothyronine concentration also declines with restricted intake. An alteration in the production of volatile fatty acids (VFAs) has also been reported and heat stress increased the production of propionate and butyrate compared to acetate (Nonaka et al., 2008) in pre-pubertal Holstein heifers. Reduced concentrations of insulin like growth factor-I (IGF-I) (Rhoads et al., 2010), plasma glucose (Baumgard et al., 2007; O'Brien et al., 2010; Wheelock et al., 2010), plasma vitamin C (Padilla et al., 2006) and non-esterified fatty acids (NEFA) (Sano et al., 1983; Ronchi et al., 1999) has been attributed to heat stress. Increase in plasma urea (Bernabucci et al., 2010; Soriani et al., 2013) and basal insulin level, despite reduced feed intake (O'Brien et al., 2010), and greater rate of cellular glucose entry (Baumgard et al., 2007; Wheelock et al., 2010) has been reported during heat stress. There are contrasting opinions as to whether the changes in blood metabolic profile are the direct effect of heat stress or the indirect effect due to reduced dry matter intake. For example, chronic heat stress related reduction in circulating growth hormone (GH) levels has been attributed to a possible effect of plane of nutrition (Mohammed and Johnson, 1985; Igono et al., 1988). Reduced feed intake due to heat stress cannot fully account for reductions in NEFA (Lacetera et al., 1996; Ronchi et al., 1999) and plasma glucose (Itoh et al., 1998) concentration. This is in contrast with the net energy balance: NEFA should increase in the plasma by fat breakdown under negative energy balance due to reduced feed intake. Therefore, these metabolic changes appeared to be the direct impact of heat stress. Further investigations in this field will require targeted work to establish any potential link between metabolic changes and heat stress stimuli. Hormonal (e.g., cortisol) and/or gene expression (e.g., heat shock protein, HSP 70; cell adhesion molecules, CD 25) assays from milk (Alhussien and Dang, 2018), saliva (Lamy et al., 2017; Kovács et al., 2019), hair follicle (Ghassemi Nejad et al., 2020; Kim et al., 2020), faeces (Rees et al., 2016) and urine (Morrow et al., 2000) are used as non-invasive determinants of the degree of heat stress. Individual profiling in base metabolic parameters may add to our current understanding and identification of heat susceptible and heat tolerant animals beforehand. However cattle metabolic response to heat stress varies based on physiological condition (Lamp et al., 2015) and may require cautious utilisation in commercial practise. Practical limitations such as cost and the sophistication of technology may also restrict their use only to experimental conditions unless biosensors are developed for low-cost continuous monitoring.
Respiration Rate and Panting
The RR and panting behaviour of cattle are predominantly associated with ambient conditions and there are genetic-specific temperature thresholds above which RR and panting increase (Gaughan et al., 2008). The RR of cattle is a key indicator of thermal stress as it is impacted by different temperature-humidity categories. Research has demonstrated that RR increases in feedlot cattle with a 1 h lag and is easy to monitor without costly equipment (Brown-Brandl et al., 2005a). However, a 2–3 h lag (Hahn et al., 1997; Gaughan et al., 2008) behind the dry bulb temperature has been reported in climate chamber studies and extended observations from at least 4 h before to 4 h after the hottest part of the day has been recommended for more complete monitoring of cattle heat stress (Gaughan et al., 2002). Eigenberg et al. (2005) considers a dry bulb temperature of between 25 and 30°C as the common threshold range for increased RR. However, a lower threshold of 21°C (Hahn et al., 1997; Brown-Brandl et al., 2006b) and a threshold black globe (BG) temperature of 25°C (Gaughan et al., 2008) are also reported. There are also varying recommendations as to the threshold RR above which heat stress mitigation measures are needed. Mitigation measures are recommended for respiratory frequencies equal to or greater than 70 to 80 breaths per min (BPM) (Berman, 2005), however, mitigation measures may be required at RRs greater than 40 BPM [Brown-Brandl et al., 2006b; Meat and Livestock Australia (MLA), 2006]. Under severe heat stress conditions, the RR of cattle may be greater than 150 BPM and in some circumstances RR may be reduced at severe stress levels due to respiratory phase shifts between “rapid-shallow” and “slow-deep” breathing (Bianca, 1962b). Therefore, RR should be evaluated along with the respiratory dynamics indicative of respiratory phases.
For large numbers of animals, the visual assessment of RR is time consuming and it is challenging to maintain accuracy from the considerable distance needed to minimise animal disturbance. Moreover, RR does not incorporate respiratory dynamics such as drooling and open mouth panting associated with increasing heat stress. RR can also reduce with severe open-mouth panting due to slower and deeper breathing (Bianca, 1962b). The respiratory dynamics of cattle can be assessed as a panting score (PS) (Table 1) which accounts for the visual changes in the respiratory behaviours. Heat stress mitigation measures should be taken when average PS is greater than or equal to 1 for at least 20% of cattle within a herd. The reliability of PS has been assessed under both research and commercial conditions (Davis et al., 2001; Mader et al., 2001; Brown-Brandl et al., 2006a; Gaughan et al., 2008). The PS is applicable over diverse climate and geographical locations, and is a cost-effective robust method for assessing heat tolerance of Bos indicus, Bos taurus, and their crosses (Gaughan et al., 2008). However, PS of cattle varies by genotype and individual circumstances and not all the animals within the same group respond equally for a specific heat load event (Gaughan et al., 2008; Van Iaer et al., 2015). Therefore, decisions based on mean PS may not account for individual variability. Furthermore, a PS represents a point in time and lacks continuity.
Heart Rate
Studies in humans have shown heart rate (HR) together with internal body temperature can indicate heat stress (Buller et al., 2008; Havenith and Fiala, 2016). Holstein-cross dairy cows showed average HR of 81, 95, and 81 beats/min during pre-treatment (under shelter 24 h), heat-treatment (under sunlight, THI >80) and post-treatment periods (under shelter 24 h), respectively (Bun et al., 2018). For automation, elastic HR monitors with transmitters have been adapted from human applications for HR monitoring of different cattle types (Janžekovič et al., 2006). Ingestible boluses or pills incorporating temperature and acoustic sensors with wireless data transmission ability have been validated over short periods of time (Martinez et al., 2006), but need updating for long-term monitoring and to remove the noise associated with rumination and other background sounds. Polar HR monitors [HRMs; S810i; Polar, Kempele, Finland] and Portable Televet Electrocardiogram [Televet 100 version 4.2.3; Kruuse, Marslev, Denmark] showed good agreement [r > 0.99] in monitoring HR and heart rate variability (HRV) (Ille et al., 2014). Periodic human assessment of HR using a stethoscope in combination with RR has been used for identification of heat-susceptible animals (Rout et al., 2018). The HRV as measured by halter-type electrocardiograph with telemetric transmission formed the basis of experimental heat stress assessment in dairy cattle in the tropics and showed that interval between successive heart beats (R-R intervals) reduced from 739 to 635 milliseconds for 12 h exposure to heat treatment from pre- and post-treatment shelter conditions (Bun et al., 2018). Computer vision based estimation of HR has also been validated (Jorquera-Chavez et al., 2019). Though HR and RR are positively correlated, HRV is very sensitive to HR and poorly associated with RR (Gasior et al., 2016). Therefore, HR and HRV can be a potential indicator of heat stress taking into consideration the individual variation in panting ability. Increased heart rate in response to heat stress is near immediate, although heart rates can be adjusted to lower levels afterwards (Bianca, 1959) due to vasodilatory effect. Consequently, HR can be used for the assessment of a short-term heat response, however, additional parameters like RR or CBT may be required for prolonged heat exposure.
Behaviour
Behavioural organisation refers to “the arrangement of behavioural states relative to each other in time and space, and in relation to other individuals' location or behaviour” (Asher et al., 2009). Cattle under heat-stressed conditions have altered behaviour as compared with those in thermoneutral conditions. Basic structural characteristics of behaviour such as bout length, transition frequencies between activities, rest or luxury activities, and variability in behaviour (Asher et al., 2009) can be affected due to heat stress. Heat-stressed cattle drank more to maintain evaporative water loss, and increased standing bouts (Brown-Brandl et al., 2006b; Lee and Hillman, 2007; Allen et al., 2015) which was thought to enhance cooling by exposing more surface area to the environment. Indeed, decreased lying bouts (Brown-Brandl et al., 2006b; Lee and Hillman, 2007; Allen et al., 2015), eating, and agonistic behaviours (Brown-Brandl et al., 2006b) were associated with heat stress. Eating time is shifted with heat stress, where eating frequency per day was shown to reduce from 15 to 3 times with greater meal size (Bernabucci et al., 2010). Animals usually avoid eating during the hotter parts of the day and eat more during the early morning and late afternoon hours including nighttime. In a study with primiparous and multiparous Holstein lactating cows, total daily activity or daytime activity was increased and total daytime and nighttime rumination durations were decreased with increasing THI ranging between 65 and 81 (Abeni and Galli, 2017). Similarly, daily rumination time during summer was negatively associated with daily maximum THI (>72) and there was a clear shift in rumination pattern with more than 60% of total daily rumination occurring at night (Soriani et al., 2013). It has been reported that cattle search for water in the afternoon or at night when shade is available, but cattle without shade went to water early in the morning and stayed near water until it was cooler in the afternoon (Blackshaw and Blackshaw, 1994). However, shade seeking and shade utilisation behaviours can differ between cattle types. Therefore, differences in breed, coat colour and body condition should be considered for behaviour-based cattle heat stress management. Heat stressed cattle cluster probably to seek shade from other animals (Sullivan and Mader, 2018), however, it may reduce convective heat loss due to less air circulation around the body surface (Mader et al., 2002).
Cattle under heat stress reduce feed intake to minimise the heat increment due to feeding. This can lead to an increase in the secretion of ghrelin, the gut hormone associated with the feeling of hunger (Polsky and von Keyserlingk, 2017). Increased peripheral blood circulation in an attempt to increase the temperature gradient between body surface and the surroundings can lead to an increase in total rumen volatile fatty acid (VFA) content due to reduced nutrient absorption efficiency resulting from reduced blood supply to the digestive system (Bernabucci et al., 2010). In addition, reduced rumination and increased panting due to heat stress may result in rumen acidosis and increased vulnerability to endotoxins entering the body (Cronjé, 2005; Bernabucci et al., 2010). Additionally, continuous stress conditions may alter the natural instincts of affected animals: lack of proper cooling facility, social rank in accessing resources and deprivation from natural behaviour (e.g., lying) may lead to frustration (Munksgaard and Simonsen, 1996). This may be more relevant particularly for shy and excitable cattle (Brown-Brandl et al., 2006a; Islam et al., 2021) and some cattle may not use the mitigation measures available to them (Polsky and von Keyserlingk, 2017). Future research should evaluate behaviour-based assessment of thermal stress and the impact of mitigation measures on cattle welfare.
Weather-Based Indices
Weather-based indices are diagnostic quantities of ambient thermal parameters (air temperature, relative humidity, solar radiation, wind speed etc.) to assess animal thermal comfort level in its environment. Though weather-based indices have been in use since the 1960s (Dunshea et al., 2013), these indices have undergone many modifications across the years. Air temperature as a single indicator omits other important ambient factors that influence thermal comfort of animals based on physiological and behavioural observations. Air temperature in combination with other weather factors was used in the development of weather-based indices as a more accurate indicator of heat stress. The requirement to combine multiple climatic (e.g., temperature, humidity, solar radiation, wind speed etc.) and physiological (e.g., body temperature, RR etc.) variables to improve accuracy made more complex indices underutilised (Spiers et al., 2012) or impractical for widespread uptake in livestock industry. Here we will restrict our discussion mainly to within THI and HLI due to their widespread use in the dairy and beef industry, respectively.
Temperature and Humidity-Based Indices
Indices based on air temperature (dry bulb), black globe temperature and relative air humidity (or wet bulb or dew point temperature) can estimate the thermal comfort level of humans and these can be modified for cattle and other animals. The THI is based on the sum of dry and wet bulb temperatures (°C) and is the most widely used index of heat stress in livestock (Ingraham et al., 1974; Hahn and Mader, 1997; Gaughan et al., 1999). The THI was modified from the discomfort index (DI) for humans (Thom, 1959). This index has been widely used as most weather stations readily collect the required climatic input variables. However, there have been many modifications in THI (Table 2) including simple conversions of temperature measurement unit from °C to °F or vice versa and differential relative weightings on temperature and humidity.
A THI value of 72 as the upper threshold of cattle thermal comfort has been suggested by earlier literature (Bohmanova et al., 2007), but recent research suggests a lower threshold of between 60 and 68 for high-producing cattle (Dunshea et al., 2013; Carabaño et al., 2016). However, evaluation of seven different THI methods found that THI thresholds can vary based on geographical location and the formulae used. In this regard, higher weighting on humidity were effective in humid climates whereas higher weighting on dry bulb temperature were more suitable for semiarid/dry climate (Bohmanova et al., 2007).
The THI as an index of thermal comfort has some limitations. The interaction between temperature and humidity are not included as inputs to the index (Berman, 2005) although evaporative heat loss increases with rising air temperature. The important climatic variables impacting heat gain or cooling such as wind speed and solar radiation, management factors including shade or other cooling facility and individual animal factors including health, genotype, coat characteristics were not accounted for in THI methods (St-Pierre et al., 2003; Brown-Brandl et al., 2005b; Mader et al., 2006; Gaughan et al., 2008). As individual animal responses to heat stress vary based on the aforementioned missing factors, THI may under- or over-estimate thermal load. The modifications to THI, for example, black globe humidity index (BGHI), wet bulb globe temperature index (WBGT); and THI adjustments for solar radiation and wind speed, have included some of these factors (Buffington et al., 1981; Eigenberg et al., 2005; Mader et al., 2006). However, THI modifications and thresholds did not consider animal or management factors that can impact on the individual heat-response of cattle under similar thermal conditions.
Heat Load Index
To overcome the limitations of THI, the HLI was developed incorporating the climatic (solar radiation, wind speed in addition to temperature and humidity), animal (genotype, coat characteristics, health, acclimatisation etc.) and management (cooling effect, manure pad status, days on feed, drinking water temperature etc.) factors affecting the heat load of cattle. The HLI model was developed by Gaughan et al. (2008) incorporating black globe (BG) temperature, to account for the effect of temperature and solar radiation, relative humidity and wind speed; and adjustments were suggested to make the model dynamic. However, the degree of adjustment and the input variables required may be a practicality issue in large cattle herds including different cattle types. The research by Gaughan et al. (2008) considered unshaded Angus steers maintained for 100 days on feed as the reference animal. The PS was used to determine the degree of heat stress of the cattle, with the assumption that PS increases from 0 to 1 for at least 20% of cattle in a pen when BG temperature is above 25°C. Gaughan et al. (2008) developed a HLI method where the index changed at a BG temperature threshold of 25°C as follows:
(i) When BG temperature is greater than 25°C
(ii) When BG temperature is less than 25°C
where RH = relative humidity (%); BGT = black globe temperature (°C); WS = wind speed (m/s); and e = the base of the natural logarithm (approximate value of e = 2.71828).
Analysis of the PS and body temperature data enabled the categorisation of HLI values into four thermal conditions (Table 3). A HLI value of <70 was considered thermoneutral and mitigation measures was suggested above 86 for unshaded black Angus steers as reference animals (Gaughan et al., 2008). The dynamic upward (for example, availability of shade might result in an adjustment of +3 to +7) and downward (for example disease condition might result in an adjustment of−5) adjustment to HLI thresholds for animal and management factors made the method suitable to include many factors influencing heat load. However, accumulation of heat from previous exposure and influence of nighttime temperature on upper threshold were not included in HLI. The distance of a pen from the weather station may also affect accuracy of heat assessment due to microclimatic variations between locations. A sudden rise and drop in HLI value can occur when BG temperature transitions between 24.9 and 25.1°C due to the 25°C BG temperature threshold. Population response based HLI threshold may have limited use at individual level as it is indicated that more than 60–90% of cattle from different genotype showed no panting (Figure 1) under different HLI categories (Gaughan et al., 2008, 2010; Van Iaer et al., 2015). This highlights the individual variability that exists in the population. Such variability can form the basis for genetic selection and targeted mitigation against heat stress.
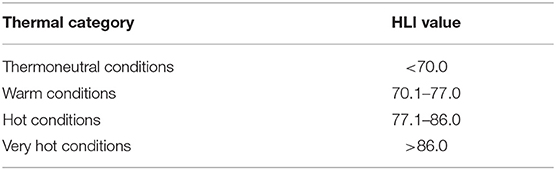
Table 3. Thermal conditions based on heat load index (HLI) values (Gaughan et al., 2008).
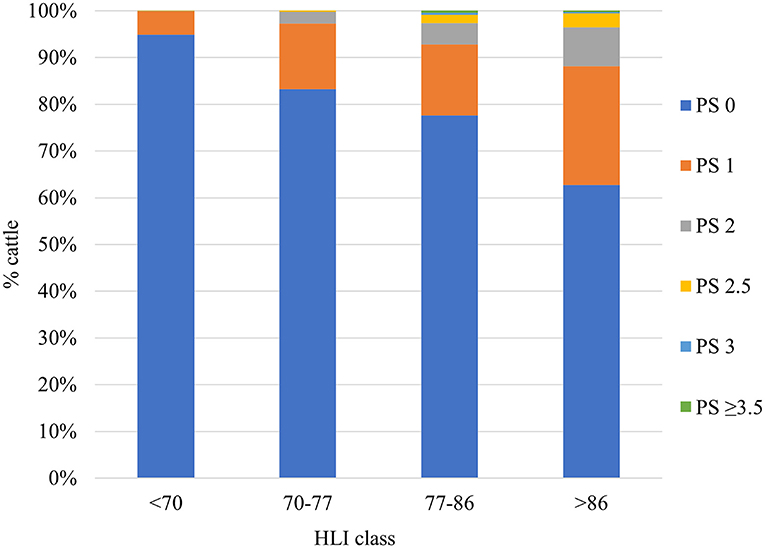
Figure 1. Percentage of cattle showing different panting scores under various heat load index (HLI) categories based on data synthesised from Gaughan et al. (2008). The cattle percentages are average values for six cattle types including Bos taurus, Bos indicus and Bos taurus × Bos indicus genotypes under shaded and unshaded conditions generating four different thermal conditions for all. The HLI classes are thermoneutral (<70), warm (70–77), hot (77–86) and very hot (>86).
Accumulated Heat Load
Hourly temperature, humidity and other thermal events within a day and across locations vary considerably and, as such, cattle may experience differing levels of thermal stress through time. This highlights the importance of the duration of heat exposure above threshold values for assessing the heat stress level of cattle. The diurnal fluctuations of daily thermal events help the dissipation of cattle body heat in the cooler part of the day, with heat typically gained in the hotter daytime periods and dissipated at night. If the nighttime recovery (Hahn and Mader, 1997) or daily cooling periods are insufficient then the animal may enter the next day with a carryover heat load. The accumulated heat load (AHL) unit accounts for this duration of exposure to HLI categories throughout the day. There are upper threshold HLI values above which cattle gain body heat, and lower thresholds below which they lose body heat. So, the heat balance of an animal depends on how long the animal was exposed to upper or lower HLI thresholds.
Gaughan et al. (2008) suggested AHL as an integral part of their HLI model and calculated AHL according to the following formulae:
If (HLIACC < HLILower Threshold), AHL = (HLIACC - HLILower Threshold)/M,
If (HLIACC > HLIUpper Threshold), AHL = (HLIACC - HLIUpper Threshold)/M,
If (HLILower Threshold ≤ HLIACC ≤ HLIUpper Threshold), AHL = 0,
where HLIACC = the actual HLI value at a point in time; HLILower Threshold = the HLI threshold below which cattle in a particular class will dissipate heat; HLIUpper Threshold = the HLI threshold above which cattle in a particular class will gain heat; and M = number of measures taken per hour.
The upper and lower threshold HLI values used to calculate AHL were suggested to be 86 and 77, respectively, for unshaded black Angus steers (Gaughan et al., 2008). A cumulative and instant balance between duration of exposure to upper and lower HLI thresholds is represented by AHL, however for a 24 h period the minimum AHL value should be set to zero indicating thermal balance. Gaughan et al. (2008) suggests five AHL categories should be used to indicate an animal's thermal condition (Table 4). However, AHL is a measure of the climatic conditions and its accuracy is also affected by the limitations of HLI. Therefore, AHL also does not account for an individual animal's response to heat stress.
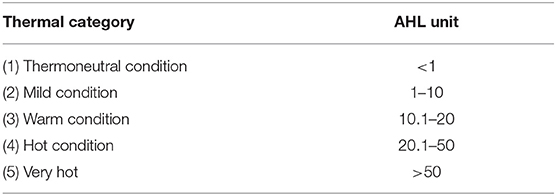
Table 4. Thermal conditions based on accumulated heat load (AHL) values Gaughan et al., 2008.
Other Indices
Numerous other indices have been developed to make them applicable under diverse thermal conditions and to make the indices dynamic and robust. However, comparative evaluation of THI, black globe humidity index (BGHI), HLI, equivalent temperature index (ETI), environmental stress index (ESI) and respiratory rate predictor (PRR) against RR and RT in Holstein and Jersey cattle under tropical conditions showed that THI and BGHI had the lowest correlation with RR and RT, while HLI and ESI were the most highly associated with RR and RT (Silva et al., 2007). An equivalent temperature index for cattle (ETIC) incorporating air temperature, relative air humidity, air velocity, solar radiation and the interactions between temperature and other variables (Wang et al., 2018) showed better predictive performance compared to THI-adjusted, HLI, dairy heat load index (DHLI) (Lees J. C. et al., 2018) and comprehensive climate index (CCI) (Mader et al., 2010). Incorporating factors such as interactions between climatic variables appeared to be more robust in predicting some but not all of the cattle responses to thermal stress. For example, ETIC omits animal factors, and was a better predictor of ST than of CBT and RR (Wang et al., 2018).
Individual Variability and Genetic Selection
Most of the weather-based indices and model-based estimates of thermal comfort are herd-level predictions of the average animal response. These indices present a potential risk to individuals that do not fall within the set threshold for the population (Havenith and Fiala, 2016). Several studies indicate contrasting ability of cattle breeds to cope with heat (Blackshaw and Blackshaw, 1994; Brown-Brandl et al., 2006b; Gaughan et al., 2010). The respiration and sweating responses of Bos indicus cattle commenced at ~8°C higher than other breeds (Worstell and Brody, 1953). With increased air and skin temperature, the sweating response of Jersey heifers increased linearly while Bos indicus heifers responded when air and skin temperature reached 30 and 35°C respectively (Allen, 1962). A comparative performance study of Friesians, Brahman × Friesian F1 crosses, and Brahmans under Australian conditions with exposure to cool, 17.2°C and hot, 37.8°C temperatures, was conducted by Colditz and Kellaway (1972). They found Friesians were more heat-susceptible with the greatest increase in RT and RR, and greatest decrease in intake (%) and weight gains compared with the other two genotypes. The RT and RR of purebred Brahman cattle was significantly lower than Hereford, Brahman and crosses of Hereford with Brahman, Boran, and Tuli breeds in both climate chamber and field studies (Gaughan et al., 1999). Gaughan et al. (2008) found a greater percentage of Brahman with PS 0 (normal breathing no panting) compared to Angus exposed to similar climatic conditions. Charolais-cross heifers had slightly higher RT (39.9 vs. 39.6 ± 0.04°C) than Angus-cross heifers in another study (Mitlohner et al., 2001). Blackshaw and Blackshaw (1994) attributed the greater heat regulatory ability of Bos indicus breeds and their crosses as compared to Bos taurus breeds to differences in metabolic rate, food and water consumption, sweating rate, and coat characteristics and colours. Conversely, Gaughan et al. (1999) pointed to the effect of surface area per unit mass in heat dissipation, and body surface temperature responsible for heat loss in mammals by radiation and convection has been found to be independent of body weight (Mortola, 2013). Differences in metabolic heat production per unit total body mass between groups can be considered in this regard, which somewhat independently of surface area to mass ratio can describe changes in core temperature as indicated for human during exercise in the heat (Cramer and Jay, 2014, 2015; Ravanelli et al., 2017). Therefore, different cattle breeds may require differential mitigation measures under similar thermal conditions.
In general, breeds of tropical origin are more heat tolerant than temperate breeds; however, there are other factors within breed that can affect heat tolerance. Dark-red Bos taurus cattle have greater heat flux at the skin and on average 0.3°C higher RT than their white-haired counterparts (Finch et al., 1984). Black-haired steers had 5.6 and 11.7°C higher body surface temperatures compared to red- and white-haired steers, respectively (Arp et al., 1983). Variation due to coat colour has also been described in other heat event studies that reported greater morbidity, percentage of cattle panting, and tympanic temperature in dark/black cattle (Busby and Loy, 1996; Mader et al., 2001; Davis et al., 2003). Other possible sources of variation may arise from metabolic and physiological differences between heat-tolerant and heat-susceptible animals. Such variability in heat response should be considered for measurement and incorporation into breeding programs. In this regard, Gaughan et al. (2010) speculated that the identification of heat-tolerant individuals within the high producing breeds may be useful in the long run if the selected animals can maintain their productivity and survival under hotter conditions.
Increased demand for meat has led to increased intensification of animal industries around the world and increased crossbreeding to achieve greater levels of productivity (Tisdell, 2003; Hoffmann and Scherf, 2006). However, crossbreeding for improved heat tolerance needs at least one locally proven heat tolerant genotype which is not available across all geographical locations (Voh et al., 2004). The greatest challenge for geneticists is to combine desirable traits that will increase heat tolerance of cattle without affecting productivity. Unfortunately, known heat-tolerant breeds often have lower levels of productivity (Gaughan et al., 2010), and heat tolerant traits are not always positively correlated with production traits. For example, the sweating response and metabolic rate are negatively correlated (Finch et al., 1982). Ortiz-Colón et al. (2018) reviewed research from the Caribbean which provided evidence that the introduction of the “slick hair” gene into Holsteins (by crossing with Senepols) may increase heat tolerance. Recently genomic parameters of heat tolerance and candidate genes along with neuronal pathways have been identified that can be useful in genome-wide and marker-assisted selection (Cheruiyot et al., 2021; Elayadeth-Meethal et al., 2021; Luo et al., 2021a,b). As heat tolerance is one of the most adaptive aspects for cattle to survive and maintain productivity in a changing climate (McManus et al., 2009), selection pressure that was traditionally placed on productive traits should be oriented towards heat tolerance traits and overall robustness (Nardone et al., 2010). In this regard automated monitoring technologies have great potential to redefine heat tolerance and susceptibility of cattle (Clark et al., 2019), and incorporation of individualised models from human studies may improve predictive powers (Havenith and Fiala, 2016).
Automated Monitoring
To maintain the welfare and performance of livestock, monitoring the effects of climatic extremes is important (Nardone et al., 2010). Rapidly evolving technologies have enabled defining a phenotype as “data describing the structure and/or function of a body” and this definition can be applied without the need of direct human observation (Clark et al., 2019). Several remote/automated monitoring techniques are under evaluation, and some have been validated (Bikker et al., 2014; Wolfger et al., 2015; Molfino et al., 2017; Pereira et al., 2018; Bar et al., 2019) to monitor cattle behaviour and health, including heat stress.
On-Animal Sensors
Respiration Rate Monitor
The pressure changes associated with muscle tone, chest movement and exhaled air form the basis of autonomous RR monitoring. Continuously recorded RR using thin-film pressure sensors and a small, battery-powered microcomputer revealed that RR changes at a rate of 6.6 and 1.6 BPM/C for unshaded and shaded steers, respectively (Eigenberg et al., 2000). A similar sensor system was used with the addition of an algorithm and data filter to remove unreliable signals and to process the output as RR (Atkins et al., 2018). The later study found that the recorded RR corresponded to body temperature and ambient thermal conditions (THI). An automated long-term RR monitoring system was developed and validated in dairy cows with high association (correlation ranging from 0.92 to 0.99 for different postures) in response to flank movement (Strutzke et al., 2019). The system was based on a differential pressure sensor and attached to the halter. However, the provision of a continuous power supply was an issue, and the displacement of the device due to animal activity and interactions with others could be a potential source of error. Further, the adjustment of halters or belts for the growth of animals would impose additional handling and welfare issues. Micro-electro-mechanical-system (MEMS) based magnetic sensors developed for humans provided more accurate breathing signals and greater spatial resolutions with lower measurement errors (Oh et al., 2018). This system presents an alternative to existing RR sensors, with some modifications required for cattle in commercial settings.
Radio Telemetric Temperature Sensors
Biosensors have been developed to log cattle body temperature and account for individual variability in thermoregulatory ability. However, temperature-logging sensors without remote transmission of data limits real-time monitoring. Temperature sensing ear-tags, rumen-reticular boluses, intra-rectal and intra-vaginal devices, and wearable and implantable (micro-chips) devices with remote data transmission ability need further development regarding heat stress prediction models, based on real-time temperature data (Koltes et al., 2018). Ingestible bio-sensors (Kim et al., 2019) and radio-frequency identification (RFID) sensors (Opasjumruskit et al., 2006; Ruiz-Garcia and Lunadei, 2011) can monitor the internal temperature of cattle individually. Radio telemetric thermo-logger data suggests that monitoring the thermoregulatory responses of cattle requires near continuous measurement of body temperature (Lefcourt and Adams, 1996). However, telemetric measurements are costly and can only operate over short distances, small numbers of animals and for short time durations (Gaughan et al., 2010). Deflected, absorbed, interfered or distorted radio frequency can provide incorrect data (Ruiz-Garcia and Lunadei, 2011) in real time data transmission. The radio telemetric measurement of CBT (Brown-Brandl et al., 2003b), undertaken by implanting a data logger with transmitter in the abdominal cavity of steers and dairy and beef cattle (Scott et al., 1983; Hahn, 1999; Mader, 2003; Brown-Brandl et al., 2005a), showed the change in CBT to be dependent on ambient conditions, and a lag from ambient temperature of 1 to 5 h. However, cattle can maintain a relatively constant CBT independent of the immediate environment (Blackshaw and Blackshaw, 1994) and such a lag time implies that there are mechanisms preventing rise in CBT and monitoring elevated CBT could be a delayed indicator of heat stress.
Location Trackers With Integrated Temperature and Motion Sensors
The use of global positioning system (GPS) based technology for monitoring animals outdoors is increasing (Bailey et al., 2018). According to Turner et al. (2000), lightweight GPS collar receivers are suitable for monitoring animal position at 5-min intervals, however, a 10-sec position fix may be required for location accuracies greater than 90% (Handcock et al., 2009). Animal behaviour characteristics and pasture utilisation can be assessed by importing the GPS data into a geographic information system (GIS). The use of GPS collars with additional temperature and dual axis (2D) motion sensors in intensively managed beef cattle revealed that cows passed inactive time near a watering point when temperature ranges were from 30–35°C and sequentially started grazing when temperature started to decrease (Turner et al., 2000). There is potential to continue research in this area for time-sequenced studies of behavioural response to heat stress. Ear tags integrating solar-powered GPS trackers are also commercially available (https://www.globalsat.com.tw). However, erroneous GPS coordinates due to poor reception, limited battery life, and the weight of the units could be an additional challenge. Neck-mounted GPS based virtual fencing (VF) technologies for cattle are emerging (Lomax et al., 2019; Colusso et al., 2020) and provide a real-time solution for animal monitoring, control of animal movement and even targeted heat amelioration through isolation of susceptible cattle if integrated with additional temperature and motion sensors. However, VF systems need to address the variability in individual animal responses and associated welfare issues (Lomax et al., 2019; Colusso et al., 2020) before commercial uptake.
Real-time location systems (RTLS) are tracking systems consisting of a fixed receiver or reader that reads location information of an animal wirelessly from small ID tag attached to them, mostly used in indoor conditions or in a specified confined area (Boulos and Berry, 2017). The location and movement of an individual animal in the proximity of feed, water and other resources can be detected and used for developing behavioural indices (Porto et al., 2014). The RTLS based location data can be used to develop algorithms to predict eating, drinking, lying, and grooming behaviours (Porto et al., 2014; Shane et al., 2016; Meunier et al., 2017). This system is effective where GPS-based positioning is interrupted (Boulos and Berry, 2017). Such systems can identify individual animals that are spending more time near water and shade and thereby determine its heat susceptibility.
Accelerometer Based Sensors
Accelerometers are devices that measure the acceleration of a structure or object in 2D or 3D space. They calculate and record the static and dynamic acceleration in x-, y- and z-axes using electromechanical sensors. These acceleration data can be converted through effective algorithms to understand the state of an object. Each behaviour of an animal has characteristic movement of the body or idleness. Animal static or dynamic movements captured in 3D can be used to classify core behaviours through defined algorithmic transformation. For example, eating, drinking, grazing, rumination, lying/resting, standing, and activity of cattle were detected by accelerometers with good correlations and moderate to high sensitivity (Se 0.50–0.98) and specificity (Sp 0.75–0.99) compared with visual observations (Bikker et al., 2014; Wolfger et al., 2015; Benaissa et al., 2017; Molfino et al., 2017; Pereira et al., 2018). Triaxial accelerometers were used by Stewart et al. (2017) to measure the flinch, step, kick (FSK) response to assess stress and discomfort in Friesian and Friesian cross Jersey cows under pasture based system. The FSK responses scored (1 to 4) based on continuous video analysis of leg movement showed high to moderate correlation with acceleration (3D leg orientation including height and direction of leg movements). Cross validating the FSK response with RR, Stewart et al. (2017) suggest that with further development the technology could be incorporated for the remote monitoring of cattle. In addition, forward-backward heaving (panting) has been assessed, along with other respiratory dynamics, as a potential indicator of panting for accelerometer-based monitoring of heat stress response in dairy and feedlot beef cattle (Bar et al., 2019; Islam et al., 2020). Therefore, accelerometer-based cattle monitoring data could enable a multimodal behaviour-based heat stress prediction/alarm model for early strategic mitigation interventions. Ear tag accelerometer sensors are most promising in this regard having been validated under moderate to hot conditions for PS up to 2 (Islam et al., 2020). However, further work is required to validate such systems during heatwave events for higher PS. Validated sensor data should be used for determining panting duration upper thresholds above which mitigation measures for heat stress can be activated. Such monitoring will provide useful actionable insights at the individual animal level allowing potential improvements in cattle welfare in heat stress, health and production issues. In addition to panting, overall behavioural organisation including timing and durations of eating, rumination, lying, standing and activity as determined by accelerometer-based sensors can indicate whether an individual animal is coping with the hot condition or not (Islam et al., 2020, 2021).
Off-Animal Devices
Weather Data Based Smart Phone Applications
Weather data can be continuously obtained from on-site weather stations and processed for automated and remote monitoring of thermal conditions. Weather stations with wireless connectivity can relay data into a network accessed virtually from anywhere. Smartphone-based applications using similar protocols have been developed combining current and projected weather information with individual animal information (Spiers et al., 2012) helping the decision-making process by sending alerts to enable farm managers to undertake remedial action to reduce heat stress. Portable weather data collection devices with Bluetooth connectivity can calculate THI and BGHI in different microclimatic areas across large-scale farming environments (de Oliveira Júnior et al., 2018). However, weather data-based assessments are indirect measures of animal response to heat stress and a fixed threshold of chosen indices in these applications may not be equally applicable for individual cattle.
Depth Imaging, Video Surveillance, and Artificial Intelligence
Computer vision-based video surveillance could be the ultimate off-animal monitoring device in the future. Legrand et al. (2011) used video cameras (red light video at night) to observe physiological and behavioural changes of dairy cattle exposed to summer weather and found that RR, ST and body temperature increased alongside HLI. Artificial neural network, fuzzy logic classifier, and machine learning based approaches using animal physiology and climatic variables have been found promising in monitoring animal core behaviours and thermal status under experimental conditions (Sousa et al., 2016, 2018; Tsai et al., 2020; Becker et al., 2021). Though the use of depth cameras and imaging can provide more accuracy, RGB images and videos of different qualities under all environmental conditions have been analysed with high accuracy (Wu et al., 2021). Considering the speed of technology development, these will very likely be useful under practical conditions in the near future. However, at the current time, identifying individuals (Qiao et al., 2020a,b, 2021) within a large commercial herd may restrict their use for continuous and individualised monitoring. The size of the recorded data may also be an issue for storage and transmission of information. Development of methods of data compression into less memory-consuming images or videos (or transforming into a different signal) and advanced feature extraction methods from transformed data, preferably from cloud-storage, could be potential future improvements in this space. Capacity building for on-site instant analysis of data may minimise data transmission and storage requirements.
Infrared Thermography (IRT)
Infrared thermography (IRT) can estimate the body surface temperature of cattle. The IRT images of different body regions of Jersey heifers were collected by Salles et al. (2016) and analysed to measure body surface temperature patterns. They found very high correlations between THI and right flank, left flank and forehead temperatures. In addition, IRT forehead temperature showed a moderate correlation with RT. Infrared images were used by Stewart et al. (2017) to measure RR to assess stress and discomfort in Friesian and Friesian cross Jersey cows under a pasture based system. The RR measured by continuous IRT imaging of airflow through the nostrils had good agreement with the live and video recording-based measurements. This result suggests that with further development IRT could be incorporated for the remote monitoring of cattle heat response (e.g., RR). However, IRT imaging and videos require a controlled environment involving additional cattle handling for data recording and sophisticated software for analysis. For example, IRT image-based forehead, dewlap and body surface temperature varied under a similar THI value (Paim et al., 2018). Also, raw IRT video data were poorly correlated with cattle internal body temperature and thermal status, and could only be used after extensive manipulations (Cuthbertson et al., 2019). Additionally, IRT in its current form may not identify the cause of change in animals' thermal pattern (McManus et al., 2016). However, recent research show promise of using IRT as a measure of cattle body temperature with associated sensors after calibration and signal processing (Wang et al., 2021); and in combination with computer vision, IRT can be an effective tool to monitor long-term breathing pattern (Kim and Hidaka, 2021).
Heat Stress Mitigation
Real-Time Mitigation
Heat stress mitigation research has gained increased interest due to the concerns of global warming and animal welfare issues associated with reduced heat resilience. Heat susceptibility of cattle has increased due to selection pressure for greater productivity and also for increased feed lotting activities. Physical modification of the environment including improved cooling facilities and nutritional management are immediate measures, where genetic selection for heat tolerance and identification of heat-tolerant genetic traits are the long-term solutions. These are the three basic management schemes that have been suggested for the abatement of heat stress and maintaining cattle performance in current hot and humid climatic conditions and in the future (Beede and Collier, 1986; West, 2003). Considering individual variability in heat stress response, mitigation strategies may not be the same for every animal within the herd [Meat and Livestock Australia (MLA), 2006]. Some factors like genotype, health status, adaptation ability, coat characteristics, body condition, days on feed, timing, and duration of core behaviours (eating, rumination, resting, standing, drinking etc.) and panting severity should be considered at the individual level [Meat and Livestock Australia (MLA), 2006; Islam et al., 2020]. In the absence of cooling facilities, nutritional approaches such as a different heat stress ration (Mader et al., 2002), supplementations (Al-Saiady et al., 2004; Cronjé, 2005; Calamari et al., 2011), and qualitative and quantitative feed restrictions (Mader et al., 2002; Davis et al., 2003) during periods of high heat load have been effective. When only nutritional mitigation is in practise, the impact of dominance (Stricklin and Gonyou, 1981; Haskell et al., 2018; Fiol et al., 2019), limited bunk space (Zobel, 2007) and feed selection (Cozzi and Gottardo, 2005; Miller-Cushon and DeVries, 2017) should be carefully evaluated. Offering feed in multiple deliveries (Schneider et al., 2020) and increasing the feeding space could be effective but may involve additional cost of infrastructure and labour (Watts et al., 2016).
For an imminent heatwave event, climatic forecast based proactive measures with adjustment based on actual environmental demand could be the best possible short-term action strategies. With the advancement of animal monitoring technologies, it is now possible to monitor, identify and isolate individual cattle for heat susceptibility on a real-time basis. It is also possible to automate the mitigation process only for the selected/isolated individuals with the activation of single- or multi-sensor based whole-farming network involving the “internet of things” (IoT) (Michie et al., 2020). A schematic outline of a proposed heat stress monitoring and mitigation protocol is depicted in Figure 2. Briefly, any of the on-animal or off-animal technologies for heat stress response monitoring will send a message to the central data repository that will refer the information alone or in combination with other parallel data (e.g., weather/climate) to the processing unit. The processing unit may work in two possible ways—(i) response for the whole herd by automated activation of heat abatement measures in place such as water spray, air cooling and other environmental modifiers (Gordon et al., 2020) and (ii) individualised response by creating a VF around cooling facilities/zones with the access for only susceptible cattle or by GPS-VF based isolation of susceptible animals to a separate cooling zone. The data repository could also be used for evaluating individual animal responses during heat wave events to identify heat-tolerant individuals and to incorporate the information in the future breeding programs to produce animals with increased heat resilience.
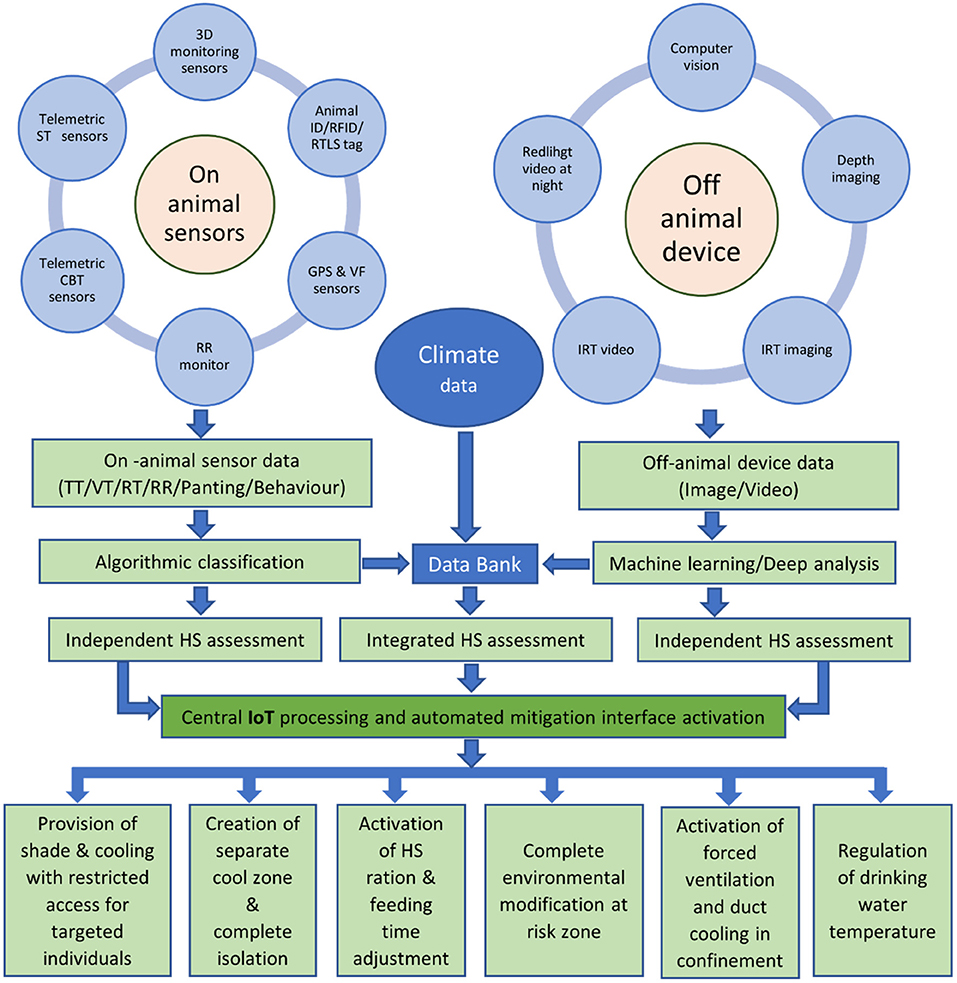
Figure 2. Automated heat stress (HS) monitoring and mitigation protocol. [RFID, radio-frequency identification; RTLS, real-time location sensor; GPS, global positioning system; VF, virtual fencing; RR, respiration rate; CBT, core body temperature; ST, skin surface temperature; IRT, infra-red thermography; TT, tympanic temperature; VT, vaginal temperature; RT, rectal temperature; IoT, internet of things].
Mitigation Cost-Benefit
Heat stress monitoring and mitigation management incurs additional investments for the development and update of facilities, nutritional alterations, and more fuel or electricity consumption for cooling. Therefore, the benefits derived from mitigation measures should outweigh the cost to the producers. Cooling of dry cows was profitable for 89% of the cows in the US (Ferreira et al., 2016), and a gross profit of $18 per heifer has been reported due to shade provisions during the summer (Mitlohner et al., 2001). Key et al. (2014) explained that heat stress mitigation cost rises at an increasing rate as the level of mitigation progresses, but the revenue over the cost gradually plateaued. This indicates a maximum revenue point after which there will be no revenue gain due to additional mitigation measure (Figure 3). Therefore, from a commercial perspective, heat stress mitigation strategies should focus on the optimum point where the benefit over the cost is maximum. However, it is worth noting that to minimise animal welfare issues, mitigation measures beyond the limit of maximum benefit may be required. From Figure 3, heat stress mitigation costs rise exponentially as the level of mitigation increase with an increase in revenue that ultimately reach a plateau near maximum level of mitigation. At middle ranges of mitigation, the benefit (difference between revenue and cost of mitigation) is the maximum over the mitigation process and after this benefit gradually narrows towards no benefit. Further research involving mitigation measures at individual level may redefine the cost-benefit of heat stress management.
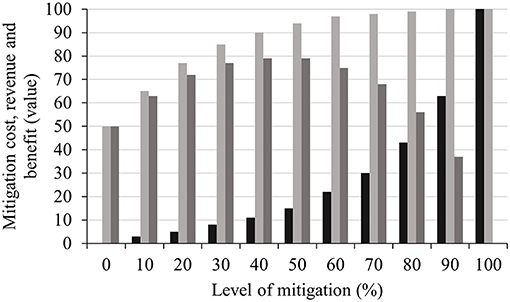
Figure 3. Break-even heat stress mitigation metric based on data synthesised from Key et al. (2014). The level of mitigation and the values ( cost of mitigation
revenue
benefit) involved were set in a hypothetical (relative) range from 0 to 100. Benefits are relative to mitigation level and indicate the revenue value after deducting the cost of mitigation.
Conclusions
Heat stress is well documented as a cause of significant financial loss in cattle production throughout the world. It is likely that the financial losses will be greater if proper monitoring and mitigation strategies are not implemented in line with continued global warming and increased intensity and duration of heat waves. Cattle heat stress monitoring and mitigation decisions have been traditionally based either on visual monitoring of animal response or on weather-based indices incorporating climatic factors and animal factors. As visual monitoring is impractical for large commercial farms and weather-based indices are impacted by individuality and microclimatic variability within farm, there is a need for autonomous monitoring systems to determine the degree of heat stress on a real-time basis. A number of developments have recently occurred in this space including various on-animal and off-animal devices that show potential for non-/least-invasive monitoring of animal responses on a 24-h basis. The development of automated environmental control devices (especially the use of the “internet of things,” IoT) and location and movement regulatory devices like GPS-incorporated virtual fencing together with automated heat stress detection technologies will enable the identification and isolation of heat-susceptible individuals and more direct mitigation measures. It is expected that all these will happen without any human subjective judgement or interactions in the near future. However, the importance of visual observations and weather-based indices cannot be ruled out unless the validity of the new technologies are established under various production systems and incorporating different animal types. Heat stress events are likely to happen more frequently and with longer durations due to the ultimate effect of climate change related to global warming. In the long run we must take measures to minimise emissions causing global warming and cattle farming itself has to find out ways to emit less greenhouse gases (GHG) without compromising the level of production.
Author Contributions
The concept of this review was generated by MAI and CC. MAI conducted the literature search, synthesis, and visualisation of published data and original draft. The structure was refined by CC, SL, and MRI. OJ provided literature support from human field. CC supervised the whole project. The whole work was reviewed and edited by CC, SL, MRI, AD, PT, and OJ. All authors have read and accepted the final version of the manuscript.
Conflict of Interest
AD is employed by Allflex Australia Pty Ltd.
The remaining authors declare that the research was conducted in the absence of any commercial or financial relationships that could be construed as a potential conflict of interest.
Publisher's Note
All claims expressed in this article are solely those of the authors and do not necessarily represent those of their affiliated organizations, or those of the publisher, the editors and the reviewers. Any product that may be evaluated in this article, or claim that may be made by its manufacturer, is not guaranteed or endorsed by the publisher.
Acknowledgments
MAI of this manuscript is an Australia Awards: Endeavour Scholarship recipient and supported by the Supplementary Scholarship for Endeavour recipients from the Faculty of Science of The University of Sydney.
References
Abeni, F., and Galli, A. (2017). Monitoring cow activity and rumination time for an early detection of heat stress in dairy cow. Int. J. Biometeorol. 61, 417–425. doi: 10.1007/s00484-016-1222-z
Abilay, T. A., Mitra, R., and Johnson, H. D. (1975). Plasma cortisol and total progestin levels in Holstein steers during acute exposure to high environmental temperature (42C) conditions. J. Anim. Sci. 41, 113–117. doi: 10.2527/jas1975.411113x
Alhussien, M. N., and Dang, A. K. (2018). Impact of different seasons on the milk somatic and differential cell counts, milk cortisol and neutrophils functionality of three Indian native breeds of cattle. J. Therm. Biol. 78, 27–35. doi: 10.1016/j.jtherbio.2018.08.020
Allen, J. D., Hall, L. W., Collier, R. J., and Smith, J. F. (2015). Effect of core body temperature, time of day, and climate conditions on behavioral patterns of lactating dairy cows experiencing mild to moderate heat stress. J. Dairy Sci. 98, 118–127. doi: 10.3168/jds.2013-7704
Allen, T. E. (1962). Responses of Zebu, Jersey,and Zebu x Jersey crossbred heifers to rising temperature, with particular reference to sweating. Aust. J. Agric. Res. 13, 165–179. doi: 10.1071/AR9620165
Al-Saiady, M. Y., Al-Shaikh, M. A., Al-Mufarrej, S. I., Al-Showeimi, T. A., Mogawer, H. H., and Dirrar, A. (2004). Effect of chelated chromium supplementation on lactation performance and blood parameters of Holstein cows under heat stress. Anim. Feed Sci. Technol. 117, 223–233. doi: 10.1016/j.anifeedsci.2004.07.008
AlZahal, O., AlZahal, H., Steele, M. A., Van Schaik, Kyriazakis, I., Duffield, T. F., et al. (2011). The use of a radiotelemetric ruminal bolus to detect body temperature changes in lactating dairy cattle. J. Dairy Sci. 94, 3568–3574. doi: 10.3168/jds.2010-3944
Ammer, S., Lambertz, C., and Gauly, M. (2016). Comparison of different measuring methods for body temperature in lactating cows under different climatic conditions. J. Dairy Res. 83, 165–172. doi: 10.1017/S0022029916000182
Amundson, J. L., Mader, T. L., Rasby, R. J., and Hu, Q. S. (2005). The effects of temperature and temperature-humidity index on pregnancy rate in beef cattle. Nebraska Beef Cattle Reports 149, 10–12. doi: 10.10/120/digitalcommons.unl.edu/animalscinber/149
Araki, C. T., Nakamura, R. M., Kam, L. W. G., and Clarke, N. (1984). Effect of lactation on diurnal temperature patterns of dairy cattle in hot environments. J. Dairy Sci. 67, 1752–1760. doi: 10.3168/jds.S0022-0302(84)81501-5
Arp, S. C., Owens, F. N., Armbruster, S. L., and Laudert, S. (1983). Relationships of coat color, body surface temperature and respiration rate in feedlot steers. Anim. Sci. Res. Rep. 114, 82–86.
Asher, L., Collins, L. M., Ortiz-Pelaez, A., Drewe, J. A., Nicol, C. J., and Pfeiffer, D. U. (2009). Recent advances in the analysis of behavioural organization and interpretation as indicators of animal welfare. J. R. Soc. Interface 6, 1103–1119. doi: 10.1098/rsif.2009.0221
Atkins, I. K., Cook, N. B., Mondaca, M. R., and Choi, C. Y. (2018). Continuous respiration rate measurement of heat-stressed dairy cows and relation to environment, body temperature, and lying time. Trans. ASABE 61, 1475–1485. doi: 10.13031/trans.12451
Bailey, D. W., Trotter, M. G., Knight, C. W., and Thomas, M. G. (2018). Use of GPS tracking collars and accelerometers for rangeland livestock production research. Transl. Anim. Sci. 2, 81–88. doi: 10.1093/tas/txx006
Bar, D., Kaim, M., Flamenbaum, I., Hanochi, B., and Toaff-Rosenstein, R. L. (2019). Technical note: accelerometer-based recording of heavy breathing in lactating and dry cows as an automated measure of heat load. J. Dairy Sci. 102, 3480–3486. doi: 10.3168/jds.2018-15186
Baumgard, L. H., Wheelock, J. B., O'Brien, M., Shwartz, G., Zimbelman, R. B., Sanders, S. R., et al. (2007). “The differential effects of heat stress vs. underfeeding on production and post-absorptive nutrient partitioning,” in 22nd Annual Southwest Nutrition Manage Conference, 116–124.
Becker, C. A., Aghalari, A., Marufuzzaman, M., and Stone, A. E. (2021). Predicting dairy cattle heat stress using machine learning techniques. J. Dairy Sci. 104, 501–524. doi: 10.3168/jds.2020-18653
Becker, C. A., Collier, R. J., and Stone, A. E. (2020). Invited review: physiological and behavioral effects of heat stress in dairy cows. J. Dairy Sci. 31, 6751–6770. doi: 10.3168/jds.2019-17929
Beede, D. K., and Collier, R. J. (1986). Potential nutritional strategies for intensively managed cattle during thermal stress. J. Anim. Sci. 62, 543–554. doi: 10.2527/jas1986.622543x
Benaissa, S., Tuyttens, F. A. M., Plets, D., De Pessemier, T., Trogh, J., Tanghe, E., et al. (2017). “Behaviours recognition using neck-mounted accelerometers in dairy barns,” in Proceedings of the 8th European Conference on Precision Livestock Farming, 12-14 September 2017 (Nantes, France), 69–76.
Bergen, R. D., and Kennedy, A. D. (2000). Relationship between vaginal and tympanic membrane temperature in beef heifers. Can. J. Anim. Sci. 80, 515–518. doi: 10.4141/A00-033
Berman, A. (2005). Estimates of heat stress relief needs for Holstein dairy cows. J. Anim. Sci. 83, 1377–1384. doi: 10.2527/2005.8361377x
Bernabucci, U., Lacetera, N., Baumgard, L. H., Rhoads, R. P., Ronchi, B., and Nardone, A. (2010). Metabolic and hormonal acclimation to heat stress in domesticated ruminants. Animal 4, 1167–1183. doi: 10.1017/S175173111000090X
Bianca, W. (1959). Acclimatization of calves to a hot humid environment. J. Agric. Sci. 52, 305–312. doi: 10.1017/S0021859600029609
Bianca, W. (1962a). Relative importance of dry- and wet-bulb temperatures in causing heat stress in cattle. Nature 195, 251–252. doi: 10.1038/195251a0
Bianca, W. (1962b). Tolerance to severe heat and the behaviour of respiratory minute volume in cattle. Nature 195, 1208–1209. doi: 10.1038/1951208a0
Bikker, J. P., van Laar, H., Rump, P., Doorenbos, J., van Meurs, K., Griffioen, G. M., et al. (2014). Technical note: evaluation of an ear-attached movement sensor to record cow feeding behavior and activity. J. Dairy Sci. 97, 2974–2979. doi: 10.3168/jds.2013-7560
Blackshaw, J. K., and Blackshaw, A. W. (1994). Heat stress in cattle and the effect of shade on production and behaviour: a review. Aust. J. Exp. Agric. 34, 285–295. doi: 10.1071/EA9940285
Bligh, J. (2006). A theoretical consideration of the means whereby the mammalian core temperature is defended at a null zone. J. Appl. Physiol. 100, 1332–1337. doi: 10.1152/japplphysiol.01068.2005
Bohmanova, J., Misztal, I., and Cole, J. B. (2007). Temperature-humidity indices as indicators of milk production losses due to heat stress. J. Dairy Sci. 90, 1947–1956. doi: 10.3168/jds.2006-513
Boulos, M. N. K., and Berry, G. (2017). Real-time locating systems (RTLS) in healthcare: a condensed primer. Environ. Health Perspect. 117:A56. doi: 10.1186/1476-072X-11-25
Brown-Brandl, T. M., Eigenberg, R. A., and Nienaber, J. A. (2006a). Heat stress risk factors of feedlot heifers. Livest. Sci. 105, 57–68. doi: 10.1016/j.livsci.2006.04.025
Brown-Brandl, T. M., Eigenberg, R. A., Nienaber, J. A., and Hahn, G. L. (2005a). Dynamic response indicators of heat stress in shaded and non-shaded feedlot cattle, part 1: analyses of indicators. Biosyst. Eng. 90, 451–462. doi: 10.1016/j.biosystemseng.2004.12.006
Brown-Brandl, T. M., Jones, D. D., and Woldt, W. E. (2005b). Evaluating modelling techniques for cattle heat stress prediction. Biosyst. Eng. 91, 513–524. doi: 10.1016/j.biosystemseng.2005.04.003
Brown-Brandl, T. M., Nienaber, J. A., Eigenberg, R. A., Hahn, G. L., and Freetly, H. (2003a). Thermoregulatory responses of feeder cattle. J. Therm. Biol. 28, 149–157. doi: 10.1016/S0306-4565(02)00052-9
Brown-Brandl, T. M., Nienaber, J. A., Eigenberg, R. A., Mader, T. L., Morrow, J. L., and Dailey, J. W. (2006b). Comparison of heat tolerance of feedlot heifers of different breeds. Livest. Sci. 105, 19–26. doi: 10.1016/j.livsci.2006.04.012
Brown-Brandl, T. M., Yanagi Jr, T., Xin, H., Gates, R., Bucklin, R. a., and Ross, G. S. (2003b). A new telemetry system for measuring core body temperautre in livestock and poultry. Appl. Eng. Agric. 19, 583–589. doi: 10.13031/2013.15316
Budd, G. M. (2008). Wet-bulb globe temperature (WBGT)-its history and its limitations. J. Sci. Med. Sport 11, 20–32. doi: 10.1016/j.jsams.2007.07.003
Buffington, D. E., Collazo-Arocho, A., Canon, G. H., Pitt, D., Thatcher, W. W., and Collier, R. J. (1981). Black globe-humidity index (BGHI) as a comfort equation for dairy cows. Trans. Am. Soc. Agric. Eng. 24, 711–714. doi: 10.13031/2013.34325
Buller, M. J., Latzka, W. A., Yokota, M., Tharion, W. J., and Moran, D. S. (2008). A real-time heat strain risk classifier using heart rate and skin temperature. Physiol. Meas. 29, 78–85. doi: 10.1088/0967-3334/29/12/N01
Bun, C., Watanabe, Y., Uenoyama, Y., Inoue, N., Ieda, N., Matsuda, F., et al. (2018). Evaluation of heat stress response in crossbred dairy cows under tropical climate by analysis of heart rate variability. J. Vet. Med. Sci. 80, 181–185. doi: 10.1292/jvms.17-0368
Burdick, N. C., Carroll, J. A., Dailey, J. W., Randel, R. D., Falkenberg, S. M., and Schmidt, T. B. (2012). Development of a self-contained, indwelling vaginal temperature probe for use in cattle research. J. Therm. Biol. 37, 339–343. doi: 10.1016/j.jtherbio.2011.10.007
Busby, D., and Loy, D. (1996). Heat stress in feedlot cattle: producer survey results. Beef Research Report. Beef Res. Rep. 26:1996.
Calamari, L., Petrera, F., Abeni, F., and Bertin, G. (2011). Metabolic and hematological profiles in heat stressed lactating dairy cows fed diets supplemented with different selenium sources and doses. Livest. Sci. 142, 128–137. doi: 10.1016/j.livsci.2011.07.005
Carabaño, M. J., Logar, B., Bormann, J., Minet, J., Vanrobays, M.-L., Díaz, C., et al. (2016). Modeling heat stress under different environmental conditions. J. Dairy Sci. 99, 3798–3814. doi: 10.3168/jds.2015-10212
Cheruiyot, E. K., Haile-Mariam, M., Cocks, B. G., MacLeod, I. M., Xiang, R., and Pryce, J. E. (2021). New loci and neuronal pathways for resilience to heat stress in cattle. Sci. Rep. 11, 1–16. doi: 10.1038/s41598-021-95816-8
Clark, C. E. F., Garcia, S. C., Marshall, K., Pryce, J. E., Greenwood, P., and Lomax, S. (2019). The livestock phenotype revolution: enabling a step-change in farm management and scientific discovery. Proc. Assoc. Advmt. Anim. Breed. Genet. 23, 596–599. Available online at: http://www.aaabg.org/aaabghome/AAABG23papers/147Clark23596.pdf.
Colditz, P. J., and Kellaway, R. C. (1972). The effect of diet and heat stress on feed intake, growth, and nitrogen metabolism in friesian, F1 Brahman x Friesian, and Brahman Heifers. Aust. J. Agric. Res. 23, 717–725. doi: 10.1071/AR9720717
Collier, R. J., and Zimbelman, R. B. (2007). “Heat stress effects on cattle : what we know and what we don't know,” in 22nd Annual Southwest Nutrition and Management Conference, February 22-23, 76–83.
Colusso, P. I., Clark, C. E. F., and Lomax, S. (2020). Should dairy cattle be trained to a virtual fence system as individuals or in groups? Animals 10, 1–19. doi: 10.3390/ani10101767
Cozzi, G., and Gottardo, F. (2005). Feeding behaviour and diet selection of finishing Limousin bulls under intensive rearing system. Appl. Anim. Behav. Sci. 91, 181–192. doi: 10.1016/j.applanim.2004.10.004
Cramer, M. N., and Jay, O. (2014). Selecting the correct exercise intensity for unbiased comparisons of thermoregulatory responses between groups of different mass and surface area. J. Appl. Physiol. 116, 1123–1132. doi: 10.1152/japplphysiol.01312.2013
Cramer, M. N., and Jay, O. (2015). Explained variance in the thermoregulatory responses to exercise: the independent roles of biophysical and fitness/fatness-related factors. J. Appl. Physiol. 119, 982–989. doi: 10.1152/japplphysiol.00281.2015
Cronjé, P. B. (2005). Heat stress in livestock—the role of the gut in its aetiology and a potential role for betaine in its alleviation. Recent Adv. Anim. Nutr. Aust. 15, 107–122. Available online at: http://livestocklibrary.com.au/bitstream/handle/1234/20022/12Cronje.pdf?sequence=1
CSIRO-BoM (2020). State of the climate 2020. Commonwealth Scientific and Industrial Research Organization (CSIRO) and The Australian Government Bureau of Meteorology(BoM).
Cuthbertson, H., Tarr, G., and González, L. A. (2019). Methodology for data processing and analysis techniques of infrared video thermography used to measure cattle temperature in real time. Comput. Electron. Agric. 167:5019. doi: 10.1016/j.compag.2019.105019
Cvetkovic, B., Smith, J. F., and Harner, J. P. (2005). Using vaginal temperature to evaluate heat stress in dairy cattle. Kansas Agric. Exp. Stn. Res. Reports 0, 9–11. doi: 10.4148/2378-5977.3203
Das, R., Sailo, L., Verma, N., Bharti, P., Saikia, J., et al. (2016). Impact of heat stress on health and performance of dairy animals: a review. Vet. World 9, 260–268. doi: 10.14202/vetworld.2016.260-268
Davis, M. S., Holt, S. M., Mader, T. L., and Cerkoney, W. (2001). Effects of feeding regimen on performance, behavior and body temperature of feedlot steers. Nebraska Beef Rep. 293, 69–73. Available online at: https://digitalcommons.unl.edu/cgi/viewcontent.cgi?article=1292&context=animalscinbcr
Davis, M. S., Mader, T. L., Holt, S. M., and Parkhurst, A. M. (2003). Strategies to reduce feedlot cattle heat stress: effect on tympanic temperature. J. Anim. Sci. 81, 649–661. doi: 10.2527/2003.813649x
de Oliveira Júnior, A. J., de Souza, S. R. L., da Cruz, V. F., Vicentin, T. A., and Glavina, A. S. G. (2018). Development of an android APP to calculate thermal comfort indexes on animals and people. Comput. Electron. Agric. 151, 175–184. doi: 10.1016/j.compag.2018.05.014
Dikmen, S., and Hansen, P. J. (2009). Is the temperature-humidity index the best indicator of heat stress in lactating dairy cows in a subtropical environment? J. Dairy Sci. 92, 109–116. doi: 10.3168/jds.2008-1370
Dreiling, C. E., Carman, F. S., and Brown, D. E. (1991). Maternal endocrine and fetal metabolic responses to heat stress. J. Dairy Sci. 74, 312–327. doi: 10.3168/jds.S0022-0302(91)78175-7
Dunshea, F. R., Leury, B. J., Fahri, F., Digiacomo, K., Hung, A., Chauhan, S., et al. (2013). Amelioration of thermal stress impacts in dairy cows. Anim. Prod. Sci. 53, 965–975. doi: 10.1071/AN12384
Eigenberg, R. A., Brown-Brandl, T. M., Nienaber, J. A., and Hahn, G. L. (2005). Dynamic response indicators of heat stress in shaded and non-shaded feedlot cattle, part 2: predictive relationships. Biosyst. Eng. 91, 111–118. doi: 10.1016/j.biosystemseng.2005.02.001
Eigenberg, R. A., Hahn, G. L., Brown-Brandl, T. M., and Spiers, D. E. (2000). Development of a new respiration rate monitor for cattle. Trans. Am. Soc. Agric. Eng. 43, 723–728. doi: 10.13031/2013.2755
Elayadeth-Meethal, M., Veettil, A. T., Asaf, M., Pramod, S., Maloney, S. K., Martin, G. B., et al. (2021). Comparative expression profiling and sequence characterization of ATP1A1 gene associated with heat tolerance in tropically adapted cattle. Animals 11:2368. doi: 10.3390/ani11082368
Ferreira, F. C., Gennari, R. S., Dahl, G. E., and De Vries, A. (2016). Economic feasibility of cooling dry cows across the United States. J. Dairy Sci. 99, 9931–9941. doi: 10.3168/jds.2016-11566
Finch, V. A., Bennett, I. L., and Holmes, C. R. (1982). Sweating response in cattle and its relation to rectal temperature, tolerance of sun and metabolic rate. J. Agric. Sci. 99, 479–487. doi: 10.1017/S0021859600031130
Finch, V. A., Bennett, I. L., and Holmes, C. R. (1984). Coat colour in cattle: effect on thermal balance, behaviour and growth, and relationship with coat type. J. Agric. Sci. 102, 141–147. doi: 10.1017/S0021859600041575
Findlay, J. D. (1958). Physiological reactions of cattle to climatic stress. Proc. Nutr. Soc. 17, 186–190. doi: 10.1079/PNS19580037
Fiol, C., Aguerre, M., Carriquiry, M., and Ungerfeld, R. (2019). Social dominance affects intake rate and behavioral time budget in pre-pubertal dairy heifers allocated in continuous competitive situations. Animal 13, 1297–1303. doi: 10.1017/S1751731118002835
Gasior, J. S., Sacha, J., Jelen, P. J., Zielinski, J., and Przybylski, J. (2016). Heart rate and respiratory rate influence on heart rate variability repeatability: effects of the correction for the prevailing heart rate. Front. Physiol. 7:356. doi: 10.3389/fphys.2016.00356
Gaughan, J. B., and Mader, T. L. (2014). Body temperature and respiratory dynamics in un-shaded beef cattle. Int. J. Biometeorol. 58, 1443–1450. doi: 10.1007/s00484-013-0746-8
Gaughan, J. B., Mader, T. L., Holt, S. M., Hahn, G. L. D., and Young, B. A. (2002). Review of current assessment of cattle and microclimate during periods of high heat load. Aust. J. Anim. Prod. 24, 77–80. Available online at: http://livestocklibrary.com.au/bitstream/handle/1234/9116/gaughan1B.pdf?sequence=1
Gaughan, J. B., Mader, T. L., Holt, S. M., Josey, M. J., and Rowan, K. J. (1999). Heat tolerance of Boran and Tuli crossbred steers. J. Anim. Sci. 77, 2398–2405. doi: 10.2527/1999.7792398x
Gaughan, J. B., Mader, T. L., Holt, S. M., and Lisle, A. (2008). A new heat load index for feedlot cattle. J. Anim. Sci. 86, 226–234. doi: 10.2527/jas.2007-0305
Gaughan, J. B., Mader, T. L., Holt, S. M., Sullivan, M. L., and Hahn, G. L. (2010). Assessing the heat tolerance of 17 beef cattle genotypes. Int. J. Biometeorol. 54, 617–627. doi: 10.1007/s00484-009-0233-4
Ghassemi Nejad, J., Park, K. H., Forghani, F., Lee, H. G., Lee, J. S., and Sung, K. (2020). Measuring hair and blood cortisol in sheep and dairy cattle using RIA and ELISA assay: a comparison. Biol. Rhythm Res. 51, 887–897. doi: 10.1080/09291016.2019.1611335
Gordon, M. S., Pickover, C. A., and Vukovic, M. (2020). “Automated deployment of autonomous devices performing localized environment alerting actions,” in International Business Machines Corporation, Armonk, NY (US). United States Patent No. US 10667455 B2. 1–19.
Hahn, G. L. (1999). Dynamic responses of cattle to thermal heat loads. J. Anim. Sci. 77, 10–20. doi: 10.2527/1997.77suppl_210x
Hahn, G. L., and Mader, T. L. (1997). “Heat waves in relation to thermuregulation, feeding behaviour and mortality of feedlot cattle,” in Proceedings of 5th International Livestock Environment Symposium, 563–571.
Hahn, G. L., Parkhurst, A. M., and Gaughan, J. B. (1997). “Cattle respiration rate as a function of ambient temperature,” in ASAE Paper No. MC97-121, 121.
Handcock, R. N., Swain, D. L., Bishop-Hurley, G. J., Patison, K. P., Wark, T., Valencia, P., et al. (2009). Monitoring animal behaviour and environmental interactions using wireless sensor networks, GPS collars and satellite remote sensing. Sensors 9, 3586–3603. doi: 10.3390/s90503586
Hansen, P. J. (2004). Physiological and cellular adaptations of zebu cattle to thermal stress. Anim. Reprod. Sci. 82, 349–360. doi: 10.1016/j.anireprosci.2004.04.011
Haskell, M. J., Rooke, J. A., Roehe, R., Turner, S. P., Hyslop, J. J., Waterhouse, A., et al. (2018). Relationships between feeding behaviour, activity, dominance and feed efficiency in finishing beef steers. Appl. Anim. Behav. Sci. 210, 9–15. doi: 10.1016/j.applanim.2018.10.012
Havenith, G., and Fiala, D. (2016). Thermal indices and thermophysiological modeling for heat stress. Compr. Physiol. 6, 255–302. doi: 10.1002/cphy.c140051
Hoffmann, I., and Scherf, B. (2006). “Animal genetic resources -time to worry?,” in Livestock report. Food and Agriculture Organization of the United Nations, Rome, Italy, 57–74.
Igono, M. O., Johnson, H. D., Steevens, B. J., Hainen, W. A., and Shanklin, M. D. (1988). Effect of season on milk temperature, milk growth hormone, prolactin, and somatic cell count of lactating cattle. Int. J. Biometeorol. 32, 194–200. doi: 10.1007/BF01045279
Ille, N., Aurich, J., Erber, R., and Aurich, C. (2014). Comparison of heart rate and heart rate variability obtained by heart rate monitors and simultaneously recorded electrocardiogram signals in nonexercising horses. J. Vet. Behav. Clin. Appl. Res. 9, 341–346. doi: 10.1016/j.jveb.2014.07.006
Ingraham, R. H., Gillette, D. D., and Wagner, W. D. (1974). Relationship of temperature and humidity to conception rate of holstein cows in subtropical climate. J. Dairy Sci. 57, 476–481. doi: 10.3168/jds.S0022-0302(74)84917-9
IPCC (Intergovernmental Panel on Climate Change) (2014). “Climate Change 2014: synthesis Report,” in Contribution of Working Groups I, II and III to the Fifth Assessment Report of the Intergovernmental Panel on Climate Change, eds. Core Writing Team, R. K. Pachauri, and L. A. Myer, Geneva, Switzerland: IPCC.
IPCC (Intergovernmental Panel on Climate Change) (2021). “Summary for Policymakers,” in Climate Change 2021: The Physical Science Basis. Contribution of Working Group I to the Sixth Assessment Report of the Intergovernmental Panel on Climate Change, eds L. Masson-Delmotte, V., P. Zhai, A. Pirani, S. L. Connors, C. Péan, S. Berger, N. Caud, et al. (Cambridge: Cambridge University Press). Available online at: https://www.ipcc.ch/report/ar6/wg1/downloads/report/IPCC_AR6_WGI_Full_Report.pdf
Islam, M. A. (2021). Montoring and mitigating heat stress in cattle (PhD Thesis). School of Life and Environmental Sciences, The University of Sydney. Available online at: https://ses.library.usyd.edu.au/handle/2123/25989
Islam, M. A., Lomax, S., Doughty, A. K., Islam, M. R., and Clark, C. E. F. (2020). Automated monitoring of panting for feedlot cattle: sensor system accuracy and individual variability. Animals 10:1518. doi: 10.3390/ani10091518
Islam, M. A., Lomax, S., Doughty, A. K., Islam, M. R., and Clark, C. E. F. (2021). Timing of eating during transition impacts feedlot cattle diet and liveweight gain. Animal 15:100137. doi: 10.1016/j.animal.2020.100137
Itoh, F., Obara, Y., Rose, M. T., and Fuse, H. (1998). Heat influences on plasma insulin and glucagon in response to secretogogues in non-lactating dairy cows. Domest. Anim. Endocrinol. 15, 499–510. doi: 10.1016/S0739-7240(98)00038-1
Janžekovič, M., Muršec, B., and Janžekovič, I. (2006). Techniques of measuring heart rate in cattle. Teh. Vjesn. 13, 31–37. Available online at: https://hrcak.srce.hr/8844
Jay, O., Capon, A., Berry, P., Broderick, C., de Dear, R., Havenith, G., et al. (2021). Reducing the health effects of hot weather and heat extremes: from personal cooling strategies to green cities. Lancet 398, 709–724. doi: 10.1016/S0140-6736(21)01209-5
Jorquera-Chavez, M., Fuentes, S., Dunshea, F. R., Warner, R. D., Poblete, T., and Jongman, E. C. (2019). Modelling and validation of computer vision techniques to assess heart rate, eye temperature, ear-base temperature and respiration rate in cattle. Animals 9:1089. doi: 10.3390/ani9121089
Kadim, I. T., Mahgoub, O., Al-Ajmi, D. S., Al-Maqbaly, R. S., Al-Mugheiry, S. M., and Bartolome, D. Y. (2004). The influence of season on quality characteristics of hot-boned beef m. longissimus thoracis. Meat Sci. 66, 831–836. doi: 10.1016/j.meatsci.2003.08.001
Kelly, M. J., Tume, R. K., Newman, S. A., and Thompson, J. M. (2001). Environmental effects on the fatty acid composition of subcutaneous beef fat. Anim. Prod. Sci. 41, 1023–1031. doi: 10.1071/EA00025
Kendall, P. E., Tucker, C. B., Dalley, D. E., Clark, D. A., and Webster, J. R. (2008). Milking frequency affects the circadian body temperature rhythm in dairy cows. Livest. Sci. 117, 130–138. doi: 10.1016/j.livsci.2007.12.009
Key, N., Sneeringer, S., and Marquardt, D. (2014). Climate change, heat stress, and U.S. dairy production. United States Dep. Agric. (USDA). Econ. Res. Serv. 175, 1–39. doi: 10.2139/ssrn.2506668
Kim, H., Min, Y., and Choi, B. (2019). Real-time temperature monitoring for the early detection of mastitis in dairy cattle: methods and case researches. Comput. Electron. Agric. 162, 119–125. doi: 10.1016/j.compag.2019.04.004
Kim, S., and Hidaka, Y. (2021). Breathing pattern analysis in cattle using infrared thermography and computer vision. Animals 11:207. doi: 10.3390/ani11010207
Kim, W. S., Ghassemi Nejad, J., Peng, D. Q., Jung, U. S., Kim, M. J., Jo, Y. H., et al. (2020). Identification of heat shock protein gene expression in hair follicles as a novel indicator of heat stress in beef calves. Animal 14, 1502–1509. doi: 10.1017/S1751731120000075
Koltes, J. E., Koltes, D. A., Mote, B. E., Tucker, J., and Hubbell, D. S. (2018). Automated collection of heat stress data in livestock: new technologies and opportunities. Transl. Anim. Sci. 2, 319–323. doi: 10.1093/tas/txy061
Kou, H. X., Zhao, Y. Q., Ren, K., Chen, X. L., Lu, Y. Q., and Wang, D. (2017). Automated measurement of cattle surface temperature and its correlation with rectal temperature. PLoS ONE 12:175377. doi: 10.1371/journal.pone.0175377
Kovács, L., Kézér, F. L., Ruff, F., Szenci, O., Bakony, M., and Jurkovich, V. (2019). Effect of artificial shade on saliva cortisol concentrations of heat-stressed dairy calves. Domest. Anim. Endocrinol. 66, 43–47. doi: 10.1016/j.domaniend.2018.09.001
Lacetera, N., Bernabucci, U., Ronchi, B., and Nardone, A. (1996). Body condition score, metabolic status and milk production of early lactating dairy cows exposed to warm environment. Riv. di Agric. Subtrop. e Trop. 90, 43–55.
Lamp, O., Derno, M., Otten, W., Mielenz, M., Nürnberg, G., and Kuhla, B. (2015). Metabolic heat stress adaption in transition cows: differences in macronutrient oxidation between late-gestating and early-lactating German Holstein dairy cows. PLoS ONE 10:125264. doi: 10.1371/journal.pone.0125264
Lamy, E., Jurkovich, V., Rodrigues, L., Geraldo, A., Cachucho, L., Silva, F., et al. (2017). Detection of 70 kDa heat shock protein in the saliva of dairy cows. J. Dairy Res. 84, 280–282. doi: 10.1017/S0022029917000280
Lea, J. M., Niemeyer, D. D. O., Reed, M. T., Fisher, A. D., and Ferguson, D. M. (2008). Development and validation of a simple technique for logging body temperature in free-ranging cattle. Aust. J. Exp. Agric. 48, 741–745. doi: 10.1071/EA07422
Lee, C. N., and Hillman, P. E. (2007). “Thermal responses of Holstein dairy cows on pastures with high solar loads and high winds,” in Sixth International Dairy Housing Conference Proceeding, 16-18 June, (Minneapolis, Minnesota) (Michigan: American Society of Agricultural and Biological Engineers), 11.
Lees, A. M., Lea, J. M., Salvin, H. E., Cafe, L. M., Colditz, I. G., and Lee, C. (2018). Relationship between rectal temperature and vaginal temperature in grazing bos taurus heifers. Animals 8, 1–8. doi: 10.3390/ani8090156
Lees, A. M., Salvin, H. E., Colditz, I. G., and Lee, C. (2020). The influence of temperament on body temperature response to handling in angus cattle. Animals 10, 1–16. doi: 10.3390/ani10010172
Lees, J. C., Lees, A. M., and Gaughan, J. B. (2018). Developing a heat load index for lactating dairy cows. Anim. Prod. Sci. 58, 1387–1391. doi: 10.1071/AN17776
Lefcourt, A. M., and Adams, W. R. (1996). Radiotelemetry measurement of body temperatures of feedlot steers during summer. J. Anim. Sci. 74, 2633–2640. doi: 10.2527/1996.74112633x
Legrand, A., Schütz, K. E., and Tucker, C. B. (2011). Using water to cool cattle: Behavioral and physiological changes associated with voluntary use of cow showers. J. Dairy Sci. 94, 3376–3386. doi: 10.3168/jds.2010-3901
Leon, L. R., and Helwig, B. G. (2010). Heat stroke: role of the systemic inflammatory response. J. Appl. Physiol. 109, 1980–1988. doi: 10.1152/japplphysiol.00301.2010
Liu, J., Li, L., Chen, X., Lu, Y., and Wang, D. (2019). Effects of heat stress on body temperature, milk production, and reproduction in dairy cows: a novel idea for monitoring and evaluation of heat stress—a review. Asian-Austral. J. Anim. Sci. 32, 1332–1339. doi: 10.5713/ajas.18.0743
Lomax, S., Colusso, P., and Clark, C. E. F. (2019). Does virtual fencing work for grazing dairy cattle? Animals 9:429. doi: 10.3390/ani9070429
Luo, H., Brito, L. F., Li, X., Su, G., Dou, J., Xu, W., et al. (2021a). Genetic parameters for rectal temperature, respiration rate, and drooling score in Holstein cattle and their relationships with various fertility, production, body conformation, and health traits. J. Dairy Sci. 104, 4390–4403. doi: 10.3168/jds.2020-19192
Luo, H., Li, X., Hu, L., Xu, W., Chu, Q., Liu, A., et al. (2021b). Genomic analyses and biological validation of candidate genes for rectal temperature as an indicator of heat stress in Holstein cattle. J. Dairy Sci. 104, 4441–4451. doi: 10.3168/jds.2020-18725
Luzi, F., Mitchell, M., Nanni Costa, L., and Redaelli, V. (2013). Thermography: current status and advances in livestock animals and in veterinary. Brescia: Fondazione Iniziative Zooprofilattiche e zootecniche.
Mader, T. L. (2003). Environmental stress in confined beef cattle. J. Anim. Sci. 81, E110–E119. Available online at: http://digitalcommons.unl.edu/animalscinbcr/307
Mader, T. L., Davis, M. S., and Brown-Brandl, T. (2006). Environmental factors influencing heat stress in feedlot cattle. J. Anim. Sci. 84, 712–719. doi: 10.2527/2006.843712x
Mader, T. L., Holt, S., Scott, T., and Davis, S. (2001). Restricted feeding strategies for reducing heat load of yearling steers. Nebraska Beef Cattle Rep. 307, 74–77.
Mader, T. L., Holt, S. M., Hahn, G. L., Davis, M. S., and Spers, D. E. (2002). Feeding strategies for managing heat load in feedlot cattle. J. Anim. Sci. 80, 2373–2382. doi: 10.2527/2002.8092373x
Mader, T. L., Johnson, L. J., and Gaughan, J. B. (2010). A comprehensive index for assessing environmental stress in animals. J. Anim. Sci. 88, 2153–2165. doi: 10.2527/jas.2009-2586
Magdub, A., Johnson, H. D., and Belyea, R. L. (1982). Effect of environmental heat and dietary fiber on thyroid physiology of lactating cows. J. Dairy Sci. Dairy Sci. 65, 2323–2331. doi: 10.3168/jds.S0022-0302(82)82504-6
Martinez, A., Schoenig, S., Andresen, D., and Warren, S. (2006). Ingestible pill for heart rate and core temperature measurement in cattle. Annu. Int. Conf. IEEE Eng. Med. Biol. Proc. 25, 3190–3193. doi: 10.1109/IEMBS.2006.259226
McCarthy, J. J., Canziani, O. F., Leary, N. A., Dokken, D. J., and White, K. S. (2001). Climate change 2001 : The scietific basis, contribution of working group II to the third assessment report of the intergovernmental panel on climate change (IPCC). Cambridge: Cambridge University Press.
McGuire, M. A., Beede, D. K., Collier, R. J., Buonomo, F., DeLorenzo, M. A., Wilcox, C. J., et al. (1991). Effects of accute thermal stress and amount of feed intake on concentration of somatotropin, insuline-like growth factor (IGF)-I and IGF-II, and thyroid hormones in plasma of lactating Holstein cows. J. Anim. Sci. 69, 2050–2056. doi: 10.2527/1991.6952050x
McManus, C., Prescott, E., Paludo, G. R., Bianchini, E., Louvandini, H., and Mariante, A. S. (2009). Heat tolerance in naturalized Brazilian cattle breeds. Livest. Sci. 120, 256–264. doi: 10.1016/j.livsci.2008.07.014
McManus, C., Tanure, C. B., Peripolli, V., Seixas, L., Fischer, V., Gabbi, A. M., et al. (2016). Infrared thermography in animal production: an overview. Comput. Electron. Agric. 123, 10–16. doi: 10.1016/j.compag.2016.01.027
Meat Livestock Australia (MLA) (2006). Tips and tools: understanding excessive heat load in feedlot cattle. North Sydney NSW 2060: MLA. Available online at: https://www.mla.com.au/globalassets/mla-corporate/generic/about-mla/heatload-~in-feedlot-cattle.pdf
Meunier, B., Delval, E., Cirié, C., Mialon, M. M., Pradel, P., Gaudron, Y., et al. (2017). “Automated measurement of dairy cow grooming behaviour from real-time location system,” in Proceedings of the 8th European Conference on Precision Livestock Farming, 12-14 September 2017, 77–83.
Michie, C., Andonovic, I., Davison, C., Hamilton, A., Tachtatzis, C., Jonsson, N., et al. (2020). The Internet of Things enhancing animal welfare and farm operational efficiency. J. Dairy Res. 87, 20–27. doi: 10.1017/S0022029920000680
Miller-Cushon, E. K., and DeVries, T. J. (2017). Feed sorting in dairy cattle: causes, consequences, and management. J. Dairy Sci. 100, 4172–4183. doi: 10.3168/jds.2016-11983
Mitlohner, F. M., Morrow, J. L., Dailey, J. W., Wilson, S. C., Galyean, M. L., Miller, M. F., et al. (2001). Shade and water misting effect on behavior, physiology, performance and carcass traits of heat-stressed feedlot cattle. J. Anim. Sci. 79, 2327–2335. doi: 10.2527/2001.7992327x
Mohammed, M. E., and Johnson, H. D. (1985). Effect of growth hormone on milk yields and related physiological functions of holstein cows exposed to heat stress. J. Dairy Sci. 68, 1123–1133. doi: 10.3168/jds.S0022-0302(85)80938-3
Molfino, J., Clark, C. E. F., Kerrisk, K. L., and Garciá, S. C. (2017). Evaluation of an activity and rumination monitor in dairy cattle grazing two types of forages. Anim. Prod. Sci. 57, 1557–1562. doi: 10.1071/AN16514
Morrow, C. J., Kolver, E. S., Verkerk, G. A., and Matthews, L. R. (2000). Urinary corticosteroids: an indicator of stress in dairy. Cattle. 60, 218–221.
Mortola, J. P. (2013). Thermographic analysis of body surface temperature of mammals. Zoolog. Sci. 30, 118–124. doi: 10.2108/zsj.30.118
Munksgaard, L., and Simonsen, H. B. (1996). Behavioral and pituitary adrenal-axis responses of dairy cows to social isolation and deprivation of lying down. J. Anim. Sci. 74, 769–778. doi: 10.2527/1996.744769x
Nardone, A., Ronchi, B., Lacetera, N., Ranieri, M. S., and Bernabucci, U. (2010). Effects of climate changes on animal production and sustainability of livestock systems. Livest. Sci. 130, 57–69. doi: 10.1016/j.livsci.2010.02.011
National Research Council (NRC) (1971). A guide to environmental research on animals. Washington, DC: National Academy of Sciences.
Nienaber, J. A., Hahn, G. L., and Eigenberg, R. A. (1999). Quantifying livestock responses for heat stress management: a review. Int. J. Biometeorol. 42, 183–188. doi: 10.1007/s004840050103
Nonaka, I., Takusari, N., Tajima, K., Suzuki, T., Higuchi, K., and Kurihara, M. (2008). Effects of high environmental temperatures on physiological and nutritional status of prepubertal Holstein heifers. Livest. Sci. 113, 14–23. doi: 10.1016/j.livsci.2007.02.010
O'Brien, M. D., Rhoads, R. P., Sanders, S. R., Duff, G. C., and Baumgard, L. H. (2010). Metabolic adaptations to heat stress in growing cattle. Domest. Anim. Endocrinol. 38, 86–94. doi: 10.1016/j.domaniend.2009.08.005
Oh, Y., Jung, Y. J., Choi, S. H., and Kim, D. W. (2018). Design and evaluation of a MEMS magnetic field sensor-based respiratory monitoring and training system for radiotherapy. Sensors 18:742. doi: 10.3390/s18092742
Opasjumruskit, K., Thanthipwan, T., Sathusen, O., Sirinamarattana, P., Gadmanee, P., Pootarapan, E., et al. (2006). Self-powered wireless temperature sensors exploit RFID technology. IEEE Pervasive Comput. 5, 54–61. doi: 10.1109/MPRV.2006.15
Ortiz-Colón, G., Fain, S. J., Parés, I. K., Curbelo-Rodríguez, J., Jiménez-Cabán, E., Pagán-Morales, M., et al. (2018). Assessing climate vulnerabilities and adaptive strategies for resilient beef and dairy operations in the tropics. Clim. Change 146, 47–58. doi: 10.1007/s10584-017-2110-1
Padilla, L., Matsui, T., Kamiya, Y., Kamiya, M., Tanaka, M., and Yano, H. (2006). Heat stress decreases plasma vitamin C concentration in lactating cows. Livest. Sci. 101, 300–304. doi: 10.1016/j.livprodsci.2005.12.002
Paim, T. P., Goulart, R., de, O., da Silva, D. A. L., Sousa, J. A., Gonçalves, L. F., Claudio, F. L., et al. (2018). Relationship of weight gain with infrared temperatures in Nelore and F1 (Nelore × Angus) heifers reared in two forage production systems. J. Anim. Sci. 96, 4002–4011. doi: 10.1093/jas/sky242
Pereira, G. M., Heins, B. J., and Endres, M. I. (2018). Technical note: validation of an ear-tag accelerometer sensor to determine rumination, eating, and activity behaviors of grazing dairy cattle. J. Dairy Sci. 101, 2492–2495. doi: 10.3168/jds.2016-12534
Polsky, L., and von Keyserlingk, M. A. G. (2017). Invited review: effects of heat stress on dairy cattle welfare. J. Dairy Sci. 100, 8645–8657. doi: 10.3168/jds.2017-12651
Porto, S. M. C., Arcidiacono, C., Giummarra, A., Anguzza, U., and Cascone, G. (2014). Localisation and identification performances of a real-time location system based on ultra wide band technology for monitoring and tracking dairy cow behaviour in a semi-open free-stall barn. Comput. Electron. Agric. 108, 221–229. doi: 10.1016/j.compag.2014.08.001
Qiao, Y., Kong, H., Clark, C., Lomax, S., Su, D., Eiffert, S., et al. (2021). Intelligent perception for cattle monitoring: a review for cattle identification, body condition score evaluation, and weight estimation. Comput. Electron. Agric. 185:106143. doi: 10.1016/j.compag.2021.106143
Qiao, Y., Su, D., Kong, H., Sukkarieh, S., Lomax, S., and Clark, C. (2020a). BiLSTM-based individual cattle identification for automated precision livestock farming. IEEE Int. Conf. Autom. Sci. Eng. 92, 967–972. doi: 10.1109/CASE48305.2020.9217026
Qiao, Y., Su, D., Kong, H., Sukkarieh, S., Lomax, S., and Clark, C. (2020b). Data augmentation for deep learning based cattle segmentation in precision livestock farming. IEEE Int. Conf. Autom. Sci. Eng. 92, 979–984. doi: 10.1109/CASE48305.2020.9216758
Ratnakaran, A. P., Sejian, V., Jose, V. S., Vaswani, S., Bagath, M., Krishnan, G., et al. (2017). Behavioral responses to livestock adaptation to heat stress challenges. Asian J. Anim. Sci. 11, 1–13. doi: 10.3923/ajas.2017.1.13
Ravanelli, N., Cramer, M., Imbeault, P., and Jay, O. (2017). The optimal exercise intensity for the unbiased comparison of thermoregulatory responses between groups unmatched for body size during uncompensable heat stress. Physiol. Rep. 5, 1–14. doi: 10.14814/phy2.13099
Rees, A., Fischer-Tenhagen, C., and Heuwieser, W. (2016). Effect of heat stress on concentrations of faecal cortisol metabolites in dairy cows. Reprod. Domest. Anim. 51, 392–399. doi: 10.1111/rda.12691
Reuter, R. R., Carroll, J. A., Hulbert, L. E., Dailey, J. W., and Galyean, M. L. (2010). Technical note: development of a self-contained, indwelling rectal temperature probe for cattle research. J. Anim. Sci. 88, 3291–3295. doi: 10.2527/jas.2010-3093
Rhoads, M. L., Kim, J. W., Collier, R. J., Crooker, B. A., Boisclair, Y. R., Baumgard, L. H., et al. (2010). Effects of heat stress and nutrition on lactating Holstein cows: II. aspects of hepatic growth hormone responsiveness. J. Dairy Sci. 93, 170–179. doi: 10.3168/jds.2009-2469
Ronchi, B., Lacetera, N., Bernabucci, U., Nardone, A., and Verini Supplizi, A. (1999). Distinct and common effects of heat stress and restricted feeding on metabolic status of Holstein heifers. Zootec. e Nutr. Anim. 25, 11–20.
Rosenzweig, C., Casassa, G., Karoly, D. J., Imeson, A., Liu, C., Menzel, A., et al. (2007). “Assessment of observed changes and responses in natural and managed systems.,” in Climate Change 2007: Impacts, Adaptation and Vulnerability. Contribution of Working Group II to the Fourth Assessment Report of the Intergovernmental Panel on Climate Change (IPCC), eds. M. L. Parry, O. F. Canziani, J. P. Palutikof, P. J. van der Linden, and C. E. Hanson (Cambridge: Cambridge University Press), 79–131.
Rout, P. K., Kaushik, R., Ramachandran, N., and Jindal, S. K. (2018). Identification of heat stress-susceptible and -tolerant phenotypes in goats in semiarid tropics. Anim. Prod. Sci. 58, 1349–1357. doi: 10.1071/AN15818
Roznik, E. A., and Alford, R. A. (2012). Does waterproofing Thermochron iButton dataloggers influence temperature readings? J. Therm. Biol. 37, 260–264. doi: 10.1016/j.jtherbio.2012.02.004
Ruiz-Garcia, L., and Lunadei, L. (2011). The role of RFID in agriculture: applications, limitations and challenges. Comput. Electron. Agric. 79, 42–50. doi: 10.1016/j.compag.2011.08.010
Salles, M. S. V., da Silva, S. C., Salles, F. A., Roma, L. C., El Faro, L., Bustos Mac Lean, P. A., et al. (2016). Mapping the body surface temperature of cattle by infrared thermography. J. Therm. Biol. 62, 63–69. doi: 10.1016/j.jtherbio.2016.10.003
Sano, H., Takahashi, K., Ambo, K., and Tsuda, T. (1983). Turnover and oxidation rates of blood glucose and heat production in sheep exposed to heat. J. Dairy Sci. 66, 856–861. doi: 10.3168/jds.S0022-0302(83)81867-0
Scanavez, A. L. A., Fragomeni, B., Rocha, L., Voelz, B. E., Hulbert, L. E., and Mendonça, L. G. D. (2017). Association between 4-day vaginal temperature assessment during the dry period and performance in the subsequent lactation of dairy cows during the warm season. J. Anim. Sci. 95, 5208–5217. doi: 10.2527/jas2017.1620
Schneider, L., Volkmann, N., Kemper, N., and Spindler, B. (2020). Feeding behavior of fattening bulls fed six times per day using an automatic feeding system. Front. Vet. Sci. 7:43. doi: 10.3389/fvets.2020.00043
Scott, I. M., Johnson, H. D., and Hahn, G. L. (1983). Effect of programmed diurnal temperature cycles on plasma thyroxine level, body temperature, and feed intake of holstein dairy cows. Int. J. Biometeorol. 27, 47–62. doi: 10.1007/BF02186300
Seguin, B. (2008). “The consequences of global warming for agriculture and food production,” in The Proceedings of the Livestock and Global Climate Change, Hammamet, Tunisia, 17-20 May 2008, eds P. Rowlinson, M. Steele, and A. Nefzaoui (Cambridge: Cambridge University Press), 9–11.
Shane, D. D., White, B. J., Larson, R. L., Amrine, D. E., and Kramer, J. L. (2016). Probabilities of cattle participating in eating and drinking behavior when located at feeding and watering locations by a real time location system. Comput. Electron. Agric. 127, 460–466. doi: 10.1016/j.compag.2016.07.005
Silanikove, N. (2000). Effects of heat stress on the welfare of extensively managed domestic ruminants. Livest. Prod. Sci. 67, 1–18. doi: 10.1016/S0301-6226(00)00162-7
Silva, R. G., da Morais, D. A. E. F., and Guilhermino, M. M. (2007). Evaluation of thermal stress indexes for dairy cows in tropical regions. R. Bras. Zootec. 36, 1192–1198. doi: 10.1590/S1516-35982007000500028
Soriani, N., Panella, G., and Calamari, L. (2013). Rumination time during the summer season and its relationships with metabolic conditions and milk production. J. Dairy Sci. 96, 5082–5094. doi: 10.3168/jds.2013-6620
Sousa, R. V., de Canata, T. F., Leme, P. R., and Martello, L. S. (2016). Development and evaluation of a fuzzy logic classifier for assessing beef cattle thermal stress using weather and physiological variables. Comput. Electron. Agric. 127, 176–183. doi: 10.1016/j.compag.2016.06.014
Sousa, R. V., de Rodrigues, A. V., Da, S., Abreu, M. G., de Tabile, R. A., and Martello, L. S. (2018). Predictive model based on artificial neural network for assessing beef cattle thermal stress using weather and physiological variables. Comput. Electron. Agric. 144, 37–43. doi: 10.1016/j.compag.2017.11.033
Spiers, D. E., Scharf, B., and Eichen, P. A. (2012). “Development of a smart phone application for heat stress detection and mitigation in livestock,” in 9th International Livestock Environment Symposium 2012 (St. Joseph, Michigan: American Society of Agricultural and Biological Engineers (ASABE)), 388.
Stewart, M., Wilson, M. T., Schaefer, A. L., Huddart, F., and Sutherland, M. A. (2017). The use of infrared thermography and accelerometers for remote monitoring of dairy cow health and welfare. J. Dairy Sci. 100, 3893–3901. doi: 10.3168/jds.2016-12055
St-Pierre, N. R., Cobanov, B., and Schnitkey, G. (2003). Economic losses from heat stress by US livestock industries. J. Dairy Sci. 86, E52–E77. doi: 10.3168/jds.S0022-0302(03)74040-5
Stricklin, W. R., and Gonyou, H. W. (1981). Dominance and eating behaviour of beef cattle fed from a single stall. Appl. Anim. Ethol. 7, 135–140. doi: 10.1016/0304-3762(81)90091-2
Strutzke, S., Fiske, D., Hoffmann, G., Ammon, C., Heuwieser, W., and Amon, T. (2019). Technical note: Development of a noninvasive respiration rate sensor for cattle. J. Dairy Sci. 102, 690–695. doi: 10.3168/jds.2018-14999
Sullivan, K. F., and Mader, T. L. (2018). Managing heat stress episodes in confined cattle. Vet. Clin. North Am. Food Anim. Pract. 34, 325–339. doi: 10.1016/j.cvfa.2018.05.001
Tisdell, C. (2003). Socioeconomic causes of loss of animal genetic diversity: analysis and assessment. Ecol. Econ. 45, 365–376. doi: 10.1016/S0921-8009(03)00091-0
Tresoldi, G., Schütz, K. E., and Tucker, C. B. (2020). Sampling strategy and measurement device affect vaginal temperature outcomes in lactating dairy cattle. J. Dairy Sci. 103, 5414–5421. doi: 10.3168/jds.2019-16667
Tsai, Y. C., Hsu, J. T., Ding, S. T., Rustia, D. J. A., and Lin, T. (2020). Assessment of dairy cow heat stress by monitoring drinking behaviour using an embedded imaging system. Biosyst. Eng. 199, 97–108. doi: 10.1016/j.biosystemseng.2020.03.013
Turner, L. W., Udal, M. C., Larson, B. T., and Shearer, S. A. (2000). Monitoring cattle behavior and pasture use with GPS and GIS. Can. J. Anim. Sci. 80, 405–413. doi: 10.4141/A99-093
Van Iaer, E., Moons, C. P. H., Ampe, B., Sonck, B., Vandaele, L., De Campeneere, S., et al. (2015). Effect of summer conditions and shade on behavioural indicators of thermal discomfort in Holstein dairy and Belgian Blue beef cattle on pasture. Animal 9, 1536–1546. doi: 10.1017/S1751731115000804
Vermunt, J. J., and Tranter, B. P. (2011). “Heat stress in dairy cattle: a review, and some of the potential risks associated with the nutritional management of this condition,” in Annual Conference of the Australian Veterinary Association—Queensland Division, 212–221.
Verwoerd, W., Wellby, M., and Barrell, G. (2006). Absence of a causal relationship between environmental and body temperature in dairy cows (Bos taurus) under moderate climatic conditions. J. Therm. Biol. 31, 533–540. doi: 10.1016/j.jtherbio.2006.07.001
Vickers, L. A., Burfeind, O., von Keyserlingk, M. A. G., Veira, D. M., Weary, D. M., and Heuwieser, W. (2010). Technical note: comparison of rectal and vaginal temperatures in lactating dairy cows. J. Dairy Sci. 93, 5246–5251. doi: 10.3168/jds.2010-3388
Vitali, A., Segnalini, M., Bertocchi, L., Bernabucci, U., Nardone, A., and Lacetera, N. (2009). Seasonal pattern of mortality and relationships between mortality and temperature-humidity index in dairy cows. J. Dairy Sci. 92, 3781–3790. doi: 10.3168/jds.2009-2127
Voh, A. A., Larbi, A., Olorunju, S. A. S., Agyemang, K., Abiola, B. D., and Williams, T. O. (2004). Fertility of N'dama and Bunaji cattle to artificial insemination following oestrus synchronization with PRID and PGF2 in the hot humid zone of Nigeria. Trop. Anim. Health Prod. 36, 499–511. doi: 10.1023/B:TROP.0000035007.19522.62
Wang, F. K., Shih, J. Y., Juan, P. H., Su, Y. C., and Wang, Y. C. (2021). Non-invasive cattle body temperature measurement using infrared thermography and auxiliary sensors. Sensors 21:72425. doi: 10.3390/s21072425
Wang, X., Gao, H., Gebremedhin, K. G., Bjerg, B. S., Van Os, J., Tucker, C. B., et al. (2018). A predictive model of equivalent temperature index for dairy cattle (ETIC). J. Therm. Biol. 76, 165–170. doi: 10.1016/j.jtherbio.2018.07.013
Watts, P. J., Davis, R. J., Keane, O. B., Luttrell, M. M., Tucker, R. W., Stafford, R., et al. (2016). Beef cattle feedlots : design and construction (Section 2: Feedlot site layout and Section 19: Feeding systems). 40 Mount Street, North Sydney, NSW 2060: Meat and Livestock Australia Ltd. Available online at: www.mla.com.au
West, J. W. (2003). Effects of heat-stress on production in dairy Cattle. J. Dairy Sci. 86, 2131–2144. doi: 10.3168/jds.S0022-0302(03)73803-X
Wheelock, J. B., Rhoads, R. P., VanBaale, M. J., Sanders, S. R., and Baumgard, L. H. (2010). Effects of heat stress on energetic metabolism in lactating Holstein cows. J. Dairy Sci. 93, 644–655. doi: 10.3168/jds.2009-2295
Wolaver, B. D., and Sharp, J. M. (2007). Thermochron iButton: limitation of this inexpensive and small-diameter temperature logger. Gr. Water Monit. Remediat. 27, 127–128. doi: 10.1111/j.1745-6592.2007.00158.x
Wolfger, B., Timsit, E., Pajor, E. A., Cook, N., Barkema, H. W., and Orsel, K. (2015). Technical note: accuracy of an ear tag-attached accelerometer to monitor rumination and feeding behavior in feedlot cattle. J. Anim. Sci. 93, 3164–3168. doi: 10.2527/jas.2014-8802
Worstell, D. M., and Brody, S. (1953). Comparative physiological reactions of European and Indian cattle to changing temperature. Missouri Agric. Exp. Stn. Bull. 515:53.
Wu, D., Wang, Y., Han, M., Song, L., Shang, Y., Zhang, X., et al. (2021). Using a CNN-LSTM for basic behaviors detection of a single dairy cow in a complex environment. Comput. Electron. Agric. 182:106016. doi: 10.1016/j.compag.2021.106016
Keywords: heat stress, sensors, individual monitoring, behavioural profiling, weather-based indices, strategic amelioration, automation
Citation: Islam MA, Lomax S, Doughty A, Islam MR, Jay O, Thomson P and Clark C (2021) Automated Monitoring of Cattle Heat Stress and Its Mitigation. Front. Anim. Sci. 2:737213. doi: 10.3389/fanim.2021.737213
Received: 06 July 2021; Accepted: 30 September 2021;
Published: 25 October 2021.
Edited by:
Surinder Singh Chauhan, The University of Melbourne, AustraliaReviewed by:
Richard Osei-Amponsah, University of Ghana, GhanaAleena Joy, The University of Melbourne, Australia
Copyright © 2021 Islam, Lomax, Doughty, Islam, Jay, Thomson and Clark. This is an open-access article distributed under the terms of the Creative Commons Attribution License (CC BY). The use, distribution or reproduction in other forums is permitted, provided the original author(s) and the copyright owner(s) are credited and that the original publication in this journal is cited, in accordance with accepted academic practice. No use, distribution or reproduction is permitted which does not comply with these terms.
*Correspondence: Md. Ashraful Islam, misl7424@uni.sydney.edu.au