- 1Department of Anesthesia, Infanta Leonor University Hospital, Madrid, Spain
- 2Universidad Complutense de Madrid, Madrid, Spain
- 3Fluid Therapy and Hemodynamic Monitoring Group of the Spanish Society of Anesthesiology and Critical Care (SEDAR), Madrid, Spain
- 4Centro de Tecnología Biomédica, Universidad Politécnica de Madrid, Madrid, Spain
- 5Instituto de Investigación e Innovación Parc Taulí (I3PT), Sabadell, Spain
- 6CIBER de Bioingeniería, Biomateriales y Nanomedicina, Instituto de Salud Carlos III, Madrid, Spain
- 7Department of Anesthesia, Virgen de las Nieves University Hospital, Granada, Spain
- 8Department of Anesthesia, San Cecilio University Hospital, Granada, Spain
- 9Department of Anesthesia, Marqués de Valdecilla University Hospital, Santander, Spain
- 10Department of Anesthesia, Althaia University Network, Manresa, Spain
- 11Department of Anesthesia, Juan Ramón Jiménez University Hospital, Huelva, Spain
- 12Department of Anesthesia, Valme University Hospital, Sevilla, Spain
- 13Department of Anesthesia, Donostia University Hospital, San Sebastian, Spain
- 14Department of Anesthesia, Mohammed Bin Khalifa Cardiac Centre, Awali, Bahrain
- 15King Salman Specialist Hospital (KSSH), Hail, Saudi Arabia
- 16Department of Anesthesia, Virgen del Rocío University Hospital, Seville, Spain
- 17Department of Intensive Care, Jerez de la Frontera University Hospital, Jerez de la Frontera, Spain
Background: Intraoperative hypotension (IOH) has been associated with increased postoperative morbidity and mortality, emphasizing the importance of maintaining hemodynamic stability during general anesthesia. The Hypotension Prediction Index (HPI) is a recently developed tool obtained from the arterial pressure waveform for predicting the likelihood of hypotension and reflecting the level of hemodynamic instability (HI).
Methods: In this retrospective observational cohort study, we analyzed anonymized data that were prospectively collected in patients having major abdominal surgery in six Spanish centers during 2022. We analyzed data from patients who underwent general anesthesia and required intraoperative monitoring with the Hemosphere monitoring system and HPI software. We defined five patterns of HI using a decision-making algorithm based on cardiovascular pathophysiology: absolute and relative hypovolemia, vasoplegia, and myocardial depression with and without vasoplegia.
Results: After analyzing 2,038 hours of hemodynamic monitoring from 393 patients, 1,946 episodes of HI were identified. Vasoplegia was the most common type of HI, accounting for 50.1% HI episodes. Absolute and relative hypovolemia were the next most frequent types, contributing 30.7% and 15.5% of the HI episodes.
Conclusions: We identified five distinct patterns of hemodynamic instability based on the evaluation of hemodynamic parameters underlying arterial hypotension during general anesthesia for patients undergoing major abdominal surgery. Vasoplegia was found to be the most frequent type. These novel classifications can help guide targeted treatment strategies for managing hemodynamic instability and reducing the risk of intraoperative hypotension.
Introduction
The association of intraoperative hypotension (IOH) with postoperative morbi-mortality (1–3) suggests that intraoperative hemodynamic stability is a significant determinant of the postoperative outcome and a potential target for hemodynamic management during general anesthesia (4). However, current management strategies for this instability are often reactive, with therapeutical interventions typically initiated only when significant derangements in cardiovascular physiology have already occurred, such as low blood pressure or cardiac output (CO) (5).
There is no consensus on how to define hemodynamic instability (HI) (6–8). However, this term is commonly used to describe an abnormal cardiovascular condition characterized by one or several out-of-range macrohemodynamic variables that, if not addressed, may lead to organ dysfunction and ultimately shock (6). Thus, HI can be a clinical manifestation of shock, but shock may be masked by physiologic compensatory mechanisms or external therapeutic support, such as vasopressors. Indeed, shock is not exclusively defined by the presence of hypotension, as it arises from an imbalance between oxygen supply and demand in the tissues. However, when clinical evidence of HI is apparent, prompt intervention is required to avoid the development or worsening of organ hypoperfusion.
HI often results from three potential and not mutually exclusive pathophysiological mechanisms: hypovolemia, myocardiac contractility impairment, or vasoplegia (6). Similarly, the etiology of IOH is multifactorial and based on patient-specific and procedure-related factors. However, whatever the mechanism causing IOH, they all eventually lead to organ hypoperfusion (8). Therefore, early and adequate hemodynamic support is crucial to prevent and avoid worsening dysfunction and postoperative complications. Besides, determining the root cause(s) of instability according to the underlying pathophysiological mechanism is a prerequisite for appropriate therapy selection, which can be a real challenge without proper hemodynamic monitoring.
The Hypotension Prediction Index (HPI, Edwards Lifesciences in Irvine, CA, USA), is a recently introduced tool that predicts the occurrence of arterial hypotension while the patient is still hemodynamically stable. The HPI algorithm is based on a supervised machine learning algorithm from the arterial pressure waveform trained to predict the likelihood of arterial hypotension, defined as mean arterial pressure (MAP) < 65 mmHg during at least 1 min. HPI values range from 0 to 100, with higher values indicating a greater likelihood of hypotension. An HPI value >80 indicates that the patient will experience a hypotensive episode in the near future with a high sensitivity and specificity, i.e., it warns that the patient is no longer hemodynamically stable (9).
In this study, we tested the hypothesis that intraoperative HI results from different pathophysiological mechanisms in a cohort of patients undergoing elective major abdominal surgery using well-defined hemodynamic patterns based on known parameters of preload-dependency (stroke volume variation, SVV), left ventricular contractility (arterial dP/dtmax) and arterial system (Eadyn, dynamic arterial elastance).
Methods
In this observational study, we retrospectively analyzed prospectively collected anonymized data from six Spanish hospitals in 2022. The study was approved by the Ethics Committee of the Hospital Universitario Infanta Leonor, Madrid, Spain (2022). The requirement for written informed consent was waived. All participating centers signed a data release agreement and received a training program in managing intraoperative arterial hypotension with the HPI. This study adheres to the applicable Strengthening the Reporting of Observational Studies in Epidemiology (STROBE) guidelines (10). A data analysis and statistical plan was written after the data were accessed.
Patients
We included patients >18 years old who underwent scheduled major abdominal surgery with an anticipated surgical duration of > 90 min and required intraoperative monitoring through an invasive arterial line with the Hemosphere monitoring system and the HPI software (Edwards Lifesciences, Irvine, CA, USA). All patients had an American Society of Anesthesiologists (ASA) physical status 2–4.
Data processing
Hemodynamic data were recorded during surgery between induction of anesthesia and tracheal extubation. We followed a four-phase methodology for data analysis divided into extraction, processing, filtering, and analysis. Initially, data were downloaded and extracted in 20-s sample intervals from the Hemosphere monitor, which provided the main hemodynamic parameters. After the extraction phase, the data was processed to obtain additional necessary parameters for analysis. These were considered secondary and necessary mainly for quality assessment (Supplementary Table 1). To ensure the reliability of the data, a filtering process to was used to detect and remove anomalous values (Supplementary Table 1).
Definition of hemodynamic instability episodes and different subtypes
We defined HI as an HPI value >80% based on the results of the hypotension prediction analysis compared to the actual occurrence of hypotensive events in the HPI validation study (9). An episode of HI was defined when the HPI reached > 80% for at least 1 min (3 consecutive measurements) and there was no other episode in the previous 5 min. The hemodynamic instability episode ends when the HPI reached <80% for at least 1 min. If an HI episode is preceded by an interval of <5 min, the latter is considered part and the evolution of the former. HPI values <80 during an episode of a duration <1 min are considered part of the episode, as they may be typical HPI fluctuations. The values of different hemodynamic parameters averaged during the first minute of the HI episode were used to define the characteristics of the HI episode (Figure 1).
We defined a priori different types of HI using clinical decision-making therapeutic protocol based on cardiovascular pathophysiology and the main mechanisms explaining arterial hypotension (Figure 2). Accordingly, absolute hypovolemia was defined as an SVV > 13% with an Eadyn > 1. Relative hypovolemia was defined as an SVV > 13% and Eadyn < 1. Vasoplegia was defined as an SVV < 13% and dP/dt > 400 mmHg/s. Myocardial depression with vasoplegia was defined as an SVV < 13%, dP/dt < 400 mmHg/s, and systemic vascular resistance index (SRVI) < 1,500 dyn s/cm5/m2; whereas myocardial depression was defined as an SVV < 13%, dP/dt < 400 mmHg/s, and RVSI > 1,500 dyn s/cm5/m2. SVRI was calculated by subtracting the central venous pressure (CVP) from the MAP, dividing by the cardiac index (CI) and multiplying by 80. A fixed value of 5 mmHg was used in cases where there was no continuous measurement of CVP.
Our study did not implement a predefined protocol or algorithm for HPI use. Clinicians had the autonomy to utilize the HPI based on their clinical judgment and experience. Moreover, specific criteria for initiating therapeutic interventions based on HPI predictions were not documented, and no clinical outcomes related to its use were recorded. Although HPI integration varied among clinicians and lacked standardization, it is noteworthy that all clinical staff received specific training on HPI use and interpretation, potentially contributing to some level of consistency in its application and therapeutic decisions during surgery.
Statistical analysis
Descriptive results are presented as the median (25th to 75th interquartile range) or as a mean (standard deviation) for continuous data and as the absolute frequency with percentage for categorical data.
All statistics were performed with MATLAB (version R2022b; The Mathworks Inc, Natick, MA).
Results
A total of 393 patients were included, 34% of whom were women. The mean age was 64.7 (13) and the body mass index was 26.3 (4.2) kg/m2. The median monitoring time per patient was 4.78 h (3.07–6.13 h). A total of 2038hours of monitoring time was evaluated, and after the screening and filtering data, 1946hours met the inclusion for the analysis, representing 95.5% of the initial dataset. A total of 2,058 HI episodes were identified, with a total cumulative duration of 148.5 h (7.62% of the analyzed time). 360 (91.6%) patients experienced at least one episode of intraoperative HI. The mean number episodes per patients was 5.23 (5.58) and the mean duration of an HI episode was 4.33 (9.72) minutes (Table 1).
The most common type of HI was vasoplegia, which accounted for 50.1% (1,032 episodes) of all HI episodes. Absolute hypovolemia was the second most frequent type, contributing 30.7% (632) of HI episodes. The least common type was low contractility with vasoplegia (Supplementary Figure 1).
Table 1 shows the hemodynamic characteristics of each predefined type of HI. The deviation of SVV, Eadyn and dP/dtmax concerning the mean values for each type is illustrated in Figure 3 using spider graphs, while box plots show normalized hemodynamic variables for each intraoperative HI type (Figures 3–7).
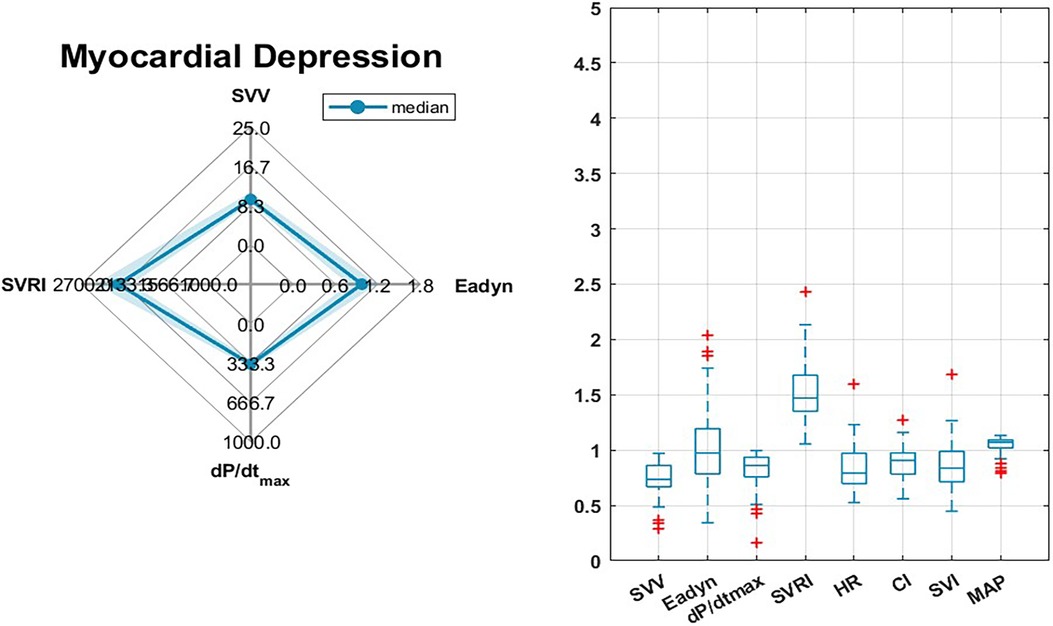
Figure 3 Myocardial depression: Spider plots showing normalized means of stroke volume variation (SVV), Arterial dynamic elastance (Eadyn), arterial Dp/dtmax and systemic vascular resistance index (SRVI) for each intraoperative predefined hemodynamic instability type. Boxplots showing normalized values of stroke volume variation (SVV), dynamic arterial elastance (Eadyn), arterial dP/dtmax, heart rate (HR), cardiac index (CI), stroke volume index (SVI), systemic vascular resistance index (SVRI) and mean arterial pressure (MAP) for each predefined hemodynamic instability type. Data are normalized to: HPI = 80; SVV = 13; Eadyn = 1; dP/dtmax = 400; SVRI = 1500; HR = 80; CI = 2.5; SVI = 40; MAP = 65.
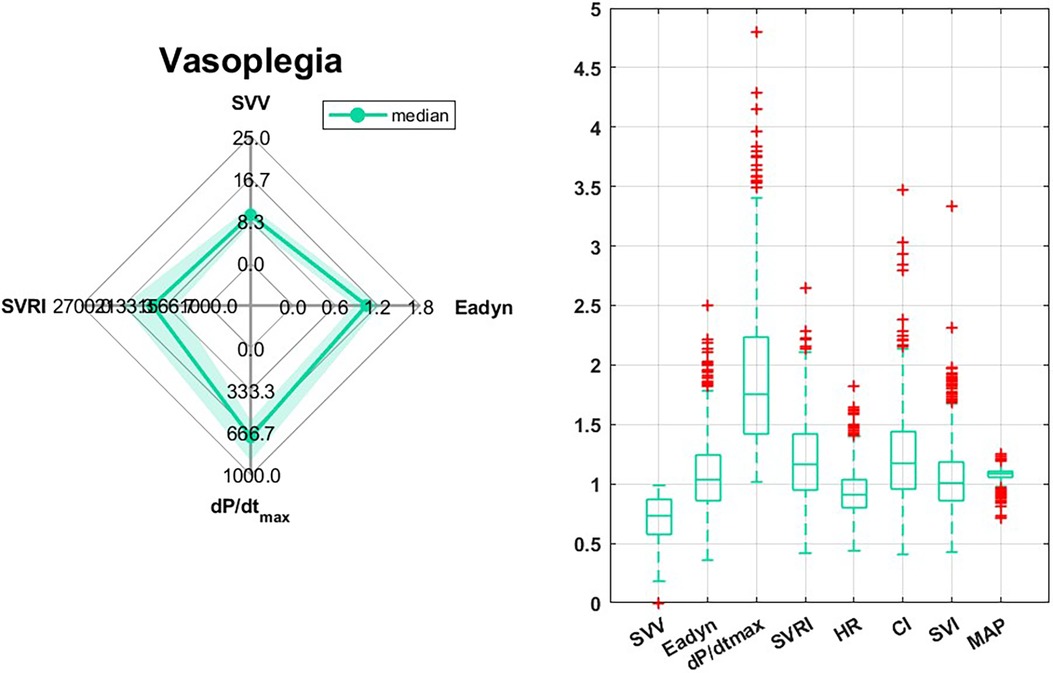
Figure 4 Vasoplegia: Spider plots showing normalized means of stroke volume variation (SVV), Arterial dynamic elastance (Eadyn), arterial Dp/dtmax and systemic vascular resistance index (SRVI) for each intraoperative predefined hemodynamic instability type. Boxplots showing normalized values of stroke volume variation (SVV), dynamic arterial elastance (Eadyn), arterial dP/dtmax, heart rate (HR), cardiac index (CI), stroke volume index (SVI), systemic vascular resistance index (SVRI) and mean arterial pressure (MAP) for each predefined hemodynamic instability type. Data are normalized to: HPI = 80; SVV = 13; Eadyn = 1; dP/dtmax = 400; SVRI = 1500; HR = 80; CI = 2.5; SVI = 40; MAP = 65.
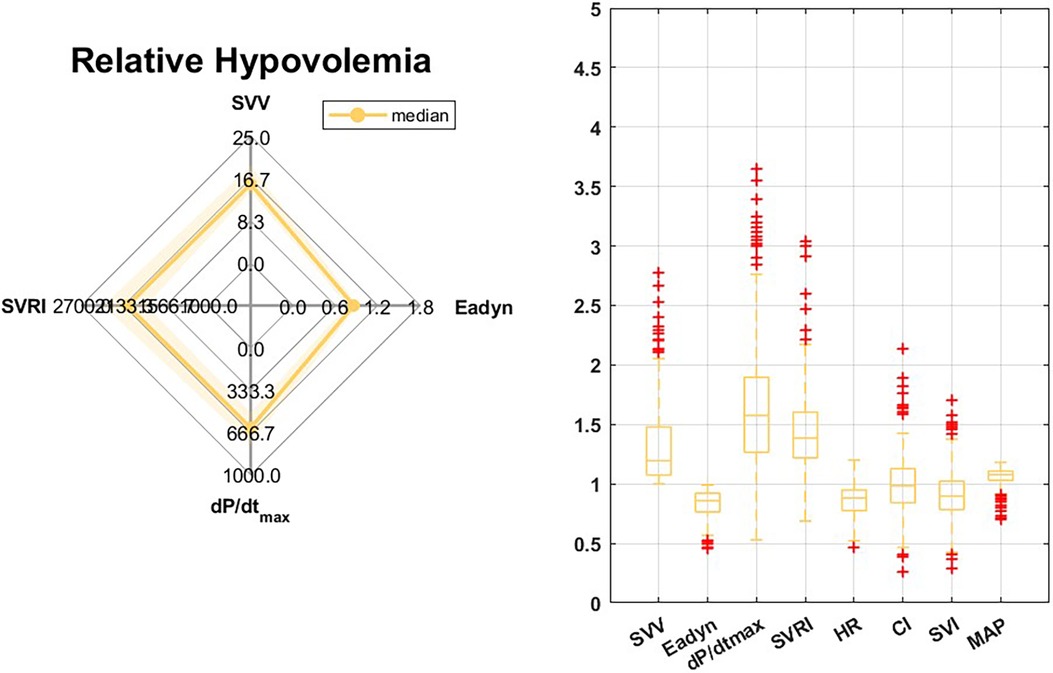
Figure 5 Relative hypovolemia: Spider plots showing normalized means of stroke volume variation (SVV), Arterial dynamic elastance (Eadyn), arterial Dp/dtmax and systemic vascular resistance index (SRVI) for each intraoperative predefined hemodynamic instability type. Boxplots showing normalized values of stroke volume variation (SVV), dynamic arterial elastance (Eadyn), arterial dP/dtmax, heart rate (HR), cardiac index (CI), stroke volume index (SVI), systemic vascular resistance index (SVRI) and mean arterial pressure (MAP) for each predefined hemodynamic instability type. Data are normalized to: HPI = 80; SVV = 13; Eadyn = 1; dP/dtmax = 400; SVRI = 1500; HR = 80; CI = 2.5; SVI = 40; MAP = 65.
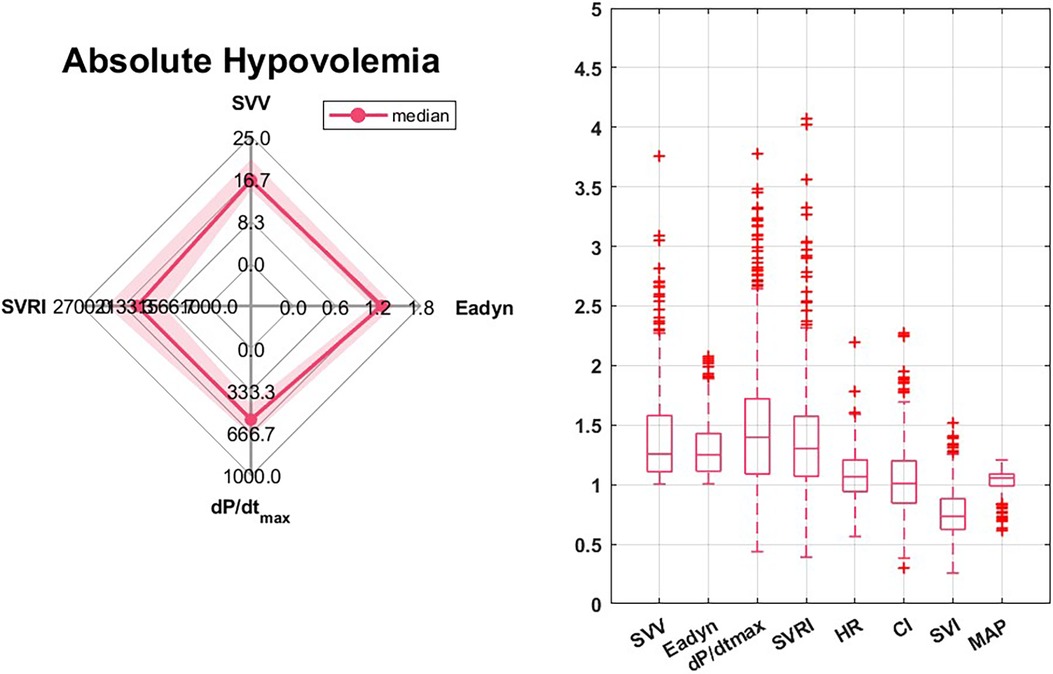
Figure 6 Absolute hypovolemia: Spider plots showing normalized means of stroke volume variation (SVV), Arterial dynamic elastance (Eadyn), arterial Dp/dtmax and systemic vascular resistance index (SRVI) for each intraoperative predefined hemodynamic instability type. Boxplots showing normalized values of stroke volume variation (SVV), dynamic arterial elastance (Eadyn), arterial dP/dtmax, heart rate (HR), cardiac index (CI), stroke volume index (SVI), systemic vascular resistance index (SVRI) and mean arterial pressure (MAP) for each predefined hemodynamic instability type. Data are normalized to: HPI = 80; SVV = 13; Eadyn = 1; dP/dtmax = 400; SVRI = 1500; HR = 80; CI = 2.5; SVI = 40; MAP = 65.
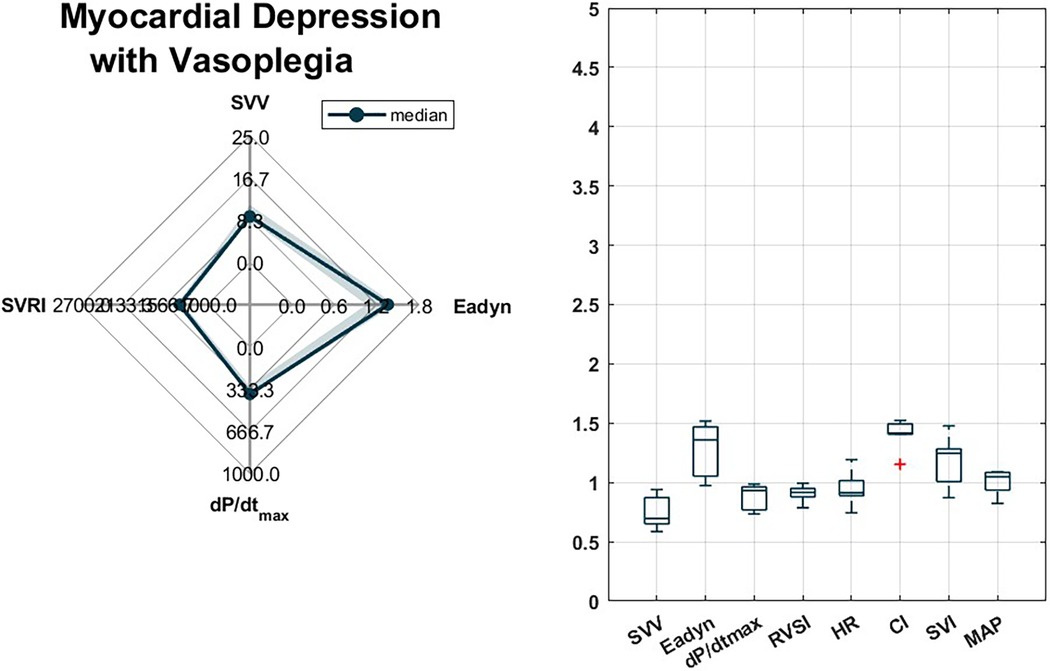
Figure 7 Myocardial depression with vasoplegia: Spider plots showing normalized means of stroke volume variation (SVV), Arterial dynamic elastance (Eadyn), arterial Dp/dtmax and systemic vascular resistance index (SRVI) for each intraoperative predefined hemodynamic instability type. Boxplots showing normalized values of stroke volume variation (SVV), dynamic arterial elastance (Eadyn), arterial dP/dtmax, heart rate (HR), cardiac index (CI), stroke volume index (SVI), systemic vascular resistance index (SVRI) and mean arterial pressure (MAP) for each predefined hemodynamic instability type. Data are normalized to: HPI = 80; SVV = 13; Eadyn = 1; dP/dtmax = 400; SVRI = 1500; HR = 80; CI = 2.5; SVI = 40; MAP = 65.
Discussion
In this study, we defined hemodynamic instability as an HPI value > 80 and identified various causes of HI based on a therapeutical protocol on the physiological mechanisms explaining arterial hypotension. These types of HI are readily identifiable by clinicians at the bedside, potentially allowing for immediate corrective treatment depending on the primary cause of HI. The most common cause of HI was vasoplegia, followed by absolute and relative hypovolemia.
Hemodynamic instability is not a specific diagnosis but a final common pathway resulting from different hemodynamic mechanisms that can coexist or act independently. Vasoplegia was the most prevalent type of HI in our cohort. It is a clinical condition characterized by decreased systemic vascular resistance in the presence of a normal or increased CO, which can lead to low blood pressure and poor tissue perfusion (11). Hypotension due to vasodilation caused by administering general or neuraxial anesthesia, even when transient, has been associated with adverse outcomes (12). Saugel et al. demonstrated that post-induction hypotension results from decreased arterial tone rather than venous vasodilation or diminished myocardial contractility (13). Although vasoplegia is a well-recognized phenomenon, it lacks a standard clinical definition. We characterized HI due to vasoplegia as an SVV value < 13%, indicating non-preload dependency, and a dP/dtmax value > 400 mmHg/s, suggesting no myocardial depression. Consequently, even if SVRI values in this group were near the lower normal range, our definition of vasoplegia was primarily pragmatic and therapeutically targeted.We categorized hypovolemia as either absolute or relative. Absolute hypovolemia refers to the loss of blood, plasma, or water from the vascular compartment, which can be defined as a decrease in intravascular volume relative to a normal-sized vascular compartment. In contrast, relative hypovolemia implies a normal or even increased blood volume that is inadequate to fill the vascular compartment due to increased capacity (14). We define both situations in a clinical and pragmatic manner, as these two situations typically require different treatments. HI due to absolute hypovolemia results from an elevated SVV with an Eadyn > 1, while relative hypovolemia is characterized by an SVV > 13% with an Eadyn < 1. These definitions illustrate two types of hypovolemia, their underlying pathophysiology, and distinct therapeutic approaches. The lack of tachycardia during episodes of absolute hypovolemia raises important questions regarding the sensitivity of autonomic compensatory mechanisms to varying degrees of volume loss. This finding emphasizes the need for a more nuanced understanding of the cardiovascular response to hypovolemia, suggesting potential variability in physiological thresholds for the activation of these autonomic compensatory mechanisms.
Both vasoplegia and hypovolemia are common causes of HI during major abdominal surgery. To address this, various hemodynamic algorithms have been developed, such as goal-directed hemodynamic therapy (15, 16). The IMPRESS study found that optimizing stroke volume (SV), in conjunction with an individualized management strategy aimed at achieving a systolic blood pressure > 10% of the reference value, improved postoperative outcomes (12). However, the use of SV optimization carries a risk of volume overload (17). To mitigate this risk, an algorithm based on HPI could be employed. According to our definitions, fluid administration would be limited to situations of HI (HPI > 80), regardless of SVV or the increase in SV after fluid administration. Moreover, fluid administration would be restricted to pressure-dependent patients, defined as an Eadyn value > 1, since only those patients will experience improved blood pressure, even if CO increases (18).
One potential cause of instability in the operative setting is the negative inotropic effects of anesthetic agents (19). Peripheral arterial dP/dtmax has been shown to correlate with left ventricular end-systolic elastance, a load independent index of ventricular systolic function (20). Since arterial pressure results from the combined interaction of the ventricular ejection and the arterial system properties, other potential factors such as afterload or preload-dependency could also contribute to the peripheral dP/dtmax (21). We therefore defined HI due to low contractility when SVV is below 13%, reflecting a non-preload dependency condition. Nevertheless, contractility changes are the most prominent determinant contributing to the arterial dP/dtmax (20). Defining myocardial depression as reduced SVI and CI, and normal pulse pressure variation (PPV), Kouz et al. found that this was the most frequent cause of IOH during major abdominal surgery (7). In contrast, based on pre-established definitions in our sample, myocardial depression was the least frequent cause of HI that could lead to IOH. This discrepancy can be attributed to various factors: our definitions for different types of HPI were a priori established with a pre-emptive decision in mind and based on a previous therapeutic protocol, while in the study of Kouz et al., endotypes of intraoperative hypotension were arbitrarily labeled after identifying six potential clusters from the studied hemodynamic parameters (SVI, heart rate, CI, SVRI, and PPV). Notably, this hemodynamic assessment did not include dP/dtmax nor Eadyn. Furthermore, the hemodynamic factors determining IOH are likely time-dependent, suggesting that the determinants defining hemodynamic instability, which may eventually lead to arterial hypotension, do not necessarily have to be the same as when hypotension is already evolving. As our identification of different patterns of HI is based on an HPI > 80 value, clinician can potentially intervene earlier to the primary cause leading to IOH.
Limitations
SVV has limitations, including sensitivity to mechanical ventilation and heart rate influence, especially in patients with arrhythmias or autonomic variability. Furthermore, SVV accuracy may be compromised in cases of irregular cardiac rhythms, vascular tone alterations, or significant ventricular dysfunction. Therefore, characterizing HI as we present it may not be useful in patients or situations where SVV validity is compromised.
Second, due to the retrospective nature of our analysis, we did not account stabilizing treatments during episodes of HI, i.e., a given episode of HI could already be adequately or inadequately treated. Although we did not attempt to quantify HI (22) but to categorize and determine the prevalence of the different patterns of HI defined as an HPI > 80. In addition, there are other potential sources of bias. Although all participating centers received prior training on the proper management of a hemodynamic protocol based on the HPI, we cannot determine whether therapeutic interventions to prevent hypotension were administered in all cases or if these interventions were adequate. While the HPI provides a useful tool for predicting hypotension, the effectiveness of targeted interventions to avoid or minimize the occurrence of hypotension may be influenced by different factors, including the experience and judgment of the clinician, patient-specific characteristics, and the procedure being performed. Therefore, despite the standardized training on the use of the HPI, variations in clinical practice may still exist, and the adequacy of interventions may be subject to interpretation.
Third, we did not consider episodes of HI due to external factors or clinical interventions such as laparoscopic insufflation or positional changes. Furthermore, we defined HI as an HPI value > 80. Davies et al. showed that a HPI below our proposed threshold of instability (70–79) was associated to a future IOH rate of 69% (23), so our HPI threshold could be debatable, although it is recommended by the manufacturer to avoid potential overtreatment situations. On the other hand, a low IOH indicates that for most subjects it is unlikely that hypotensive events will occur in the short term, which is why we define hemodynamic stability as an HPI < 30, although below that threshold there is a minimal risk of future hypotension (23).
Finally, despite the lack of consensus for the definition of IOH, a MAP <65 mmHg is most frequently considered nowadays as an appropriate threshold value for defining IOH (24). However, individual patients' factors may dictate the need for higher blood pressure to maintain adequate perfusion pressure, and in these cases, the definition of hypotension according to the HPI algorithm may be questionable. This could result in lower HPI values indicating HI, which could potentially lead to inappropriate interventions. Delving into determining the initial MAP reference value could enhance data comprehension and the effective application of the HPI algorithm, thus reducing the risk of inappropriate interventions.
Conclusions
Our study has developed a classification system with five distinct patterns of HI based on the clinical evaluation of hemodynamic parameters that underlie arterial hypotension. This classification system provides a more nuanced approach to the assessment and management of HI and offers the potential for more personalized care by tailoring interventions to the main cause of HI.
Data availability statement
The raw data supporting the conclusions of this article will be made available by the authors, without undue reservation.
Ethics statement
The studies involving humans were approved by Infanta Leonor Ethics Committe. The studies were conducted in accordance with the local legislation and institutional requirements. The Ethics Committee/institutional review board waived the requirement of written informed consent for participation from the participants or the participants’ legal guardians/next of kin because Anonimized data from hemodynamic monitors.
Author contributions
JR-M: Conceptualization, Data curation, Formal Analysis, Funding acquisition, Investigation, Methodology, Project administration, Resources, Supervision, Validation, Visualization, Writing – original draft, Writing – review & editing. MV-B: Conceptualization, Data curation, Investigation, Methodology, Project administration, Software, Validation, Visualization, Writing – original draft, Writing – review & editing. PF-V-B: Investigation, Supervision, Validation, Visualization, Writing – review & editing. AR-H: Investigation, Methodology, Software, Supervision, Validation, Visualization, Writing – review & editing. JT-R: Investigation, Validation, Visualization, Writing – review & editing. MO-G: Investigation, Supervision, Validation, Visualization, Writing – review & editing. DG-L: Investigation, Supervision, Visualization, Writing – review & editing. AR-E: Investigation, Validation, Visualization, Writing – review & editing. LC-S: Supervision, Validation, Visualization, Writing – review & editing. AA-G: Resources, Supervision, Validation, Writing – review & editing. JL: Investigation, Resources, Supervision, Validation, Visualization, Writing – review & editing. CV-B: Investigation, Supervision, Visualization, Writing – review & editing. AA-P: Investigation, Supervision, Visualization, Writing – review & editing. ÁE: Investigation, Supervision, Visualization, Writing – review & editing. IJ-L: Supervision, Visualization, Writing – review & editing. MM-G: Formal Analysis, Investigation, Methodology, Project administration, Resources, Software, Supervision, Validation, Visualization, Writing – original draft, Writing – review & editing.
Funding
The author(s) declare financial support was received for the research, authorship, and/or publication of this article.
The authors declare that this study received funding from Edwards Lifesciences. The funder was not involved in the study design, collection, analysis, interpretation of data, the writing of this article, or the decision to submit it for publication.
Conflicts of interest
JR-M reports the following: advisory board for Edwards Lifesciences Spain, conference fees from Edwards Lifesciences, Fresenius Kabi and Braun. JT-R conference fees from Edwards Lifesciences and Baxter. LC-S conference fees from Edwards Lifesciences. AA-G reports conference fees from Edwards Lifesciences, MSD and 3M. JL advisory board for Edwards Lifesciences Spain, conference fees from Edwards Lifesciences, Fresenius Kabi, bioMérieux, Vifor Pharma, Grifols. Research support from Edwards Lifesciences and bioMérieux. IJ-L advisory board for Edwards Lifesciences Spain, conference fees from Edwards Lifesciences. MM-G advisory board for Edwards Lifesciences Spain, conference fees from Edwards Lifesciences.
The remaining authors declare that the research was conducted in the absence of any commercial or financial relationships that could be construed as a potential conflict of interest.
Publisher's note
All claims expressed in this article are solely those of the authors and do not necessarily represent those of their affiliated organizations, or those of the publisher, the editors and the reviewers. Any product that may be evaluated in this article, or claim that may be made by its manufacturer, is not guaranteed or endorsed by the publisher.
Supplementary material
The Supplementary Material for this article can be found online at: https://www.frontiersin.org/articles/10.3389/fanes.2024.1405405/full#supplementary-material
References
1. Salmasi V, Maheshwari K, Yang D, Mascha EJ, Singh A, Sessler DI, et al. Relationship between intraoperative hypotension, defined by either reduction from baseline or absolute thresholds, and acute kidney and myocardial injury after noncardiac surgery: a retrospective cohort analysis. Anesthesiology. (2017) 126:47–65. doi: 10.1097/ALN.0000000000001432
2. Walsh M, Devereaux PJ, Garg AX, Kurz A, Turan A, Rodseth RN, et al. Relationship between intraoperative mean arterial pressure and clinical outcomes after noncardiac surgery: toward an empirical definition of hypotension. Anesthesiology. (2013) 119:507–15. doi: 10.1097/ALN.0b013e3182a10e26
3. Wesselink EM, Kappen TH, Torn HM, Slooter AJC, van Klei WA. Intraoperative hypotension and the risk of postoperative adverse outcomes: a systematic review. Br J Anaesth. (2018) 121:706–21. doi: 10.1016/j.bja.2018.04.036
4. Maheshwari K. Blood pressure stability: a road to better outcomes. J Clin Monit Comput. (2021) 35:679–80. doi: 10.1007/s10877-021-00659-3
5. Wickham AJ, Highton DT, Clark S, Fallaha D, Wong DJN, Martin DS. Treatment threshold for intra-operative hypotension in clinical practice-a prospective cohort study in older patients in the UK. Anaesthesia. (2022) 77:153–63. doi: 10.1111/anae.15535
6. Vincent J-L, De Backer D. Circulatory shock. N Engl J Med. (2013) 369:1726–34. doi: 10.1056/NEJMra1208943
7. Kouz K, Brockmann L, Timmermann LM, Bergholz A, Flick M, Maheshwari K, et al. Endotypes of intraoperative hypotension during major abdominal surgery: a retrospective machine learning analysis of an observational cohort study. Br J Anaesth. (2023) 130:253–61. doi: 10.1016/j.bja.2022.07.056
8. Meng L: Heterogeneous impact of hypotension on organ perfusion and outcomes: a narrative review. Br J Anaesth 2021; 127:845–61 doi: 10.1016/j.bja.2021.06.048
9. Hatib F, Jian Z, Buddi S, Lee C, Settels J, Sibert K, et al. Machine-learning algorithm to predict hypotension based on high-fidelity arterial pressure waveform analysis. Anesthesiology. (2018) 129:663–74. doi: 10.1097/ALN.0000000000002300
10. von Elm E, Altman DG, Egger M, Pocock SJ, Gotzsche PC, Vandenbroucke JP. The strengthening the reporting of observational studies in epidemiology (STROBE) statement: guidelines for reporting observational studies. Int J Surg. (2014) 12:1495–9. doi: 10.1016/j.ijsu.2014.07.013
11. Lambden S, Creagh-Brown BC, Hunt J, Summers C, Forni LG. Definitions and pathophysiology of vasoplegic shock. Crit Care. (2018) 22:174. doi: 10.1186/s13054-018-2102-1
12. Futier E, Lefrant J-Y, Guinot P-G, Godet T, Lorne E, Cuvillon P, et al. Effect of individualized vs standard blood pressure management strategies on postoperative organ dysfunction among high-risk patients undergoing Major surgery: a randomized clinical trial. JAMA. (2017) 318:1346–57. doi: 10.1001/jama.2017.14172
13. Saugel B, Bebert E-J, Briesenick L, Hoppe P, Greiwe G, Yang D, et al. Mechanisms contributing to hypotension after anesthetic induction with sufentanil, propofol, and rocuronium: a prospective observational study. J Clin Monit Comput. (2022) 36:341–7. doi: 10.1007/s10877-021-00653-9
14. Kimball JP, Inan OT, Convertino VA, Cardin S, Sawka MN. Wearable sensors and machine learning for hypovolemia problems in occupational, military and sports medicine: physiological basis, hardware and algorithms. Sensors (Basel). (2022) 22:442. doi: 10.3390/s22020442
15. Calvo-Vecino JM, Ripollés-Melchor J, Mythen MG, Casans-Francés R, Balik A, Artacho JP, et al. Effect of goal-directed haemodynamic therapy on postoperative complications in low-moderate risk surgical patients: a multicentre randomised controlled trial (FEDORA trial). Br J Anaesth. (2018) 120:734–44. doi: 10.1016/j.bja.2017.12.018
16. de Waal EEC, Frank M, Scheeren TWL, Kaufmann T, de Korte-de Boer D, Cox B, et al. Perioperative goal-directed therapy in high-risk abdominal surgery. A multicenter randomized controlled superiority trial. J Clin Anesth. (2021) 75:110506. doi: 10.1016/j.jclinane.2021.110506
17. Monnet X, Marik PE, Teboul JL. Prediction of fluid responsiveness: an update. Ann Intensive Care. (2016) 6:111. doi: 10.1186/s13613-016-0216-7
18. Monge García MI, Guijo González P, Gracia Romero M, Gil Cano A, Rhodes A, Grounds RM, et al. Effects of arterial load variations on dynamic arterial elastance: an experimental study. Br J Anaesth. (2017) 118:938–46. doi: 10.1093/bja/aex070
19. Ririe DG, Lundell JC, Neville MJ. Direct effects of propofol on myocardial and vascular tissue from mature and immature rats. J Cardiothorac Vasc Anesth. (2001) 15:745–9. doi: 10.1053/jcan.2001.28321
20. Monge Garcia MI, Jian Z, Settels JJ, Hunley C, Cecconi M, Hatib F, et al. Performance comparison of ventricular and arterial dP/dt(max) for assessing left ventricular systolic function during different experimental loading and contractile conditions. Crit Care. (2018) 22:325. doi: 10.1186/s13054-018-2260-1
21. De Hert SG, Robert D, Cromheecke S, Michard F, Nijs J, Rodrigus IE. Evaluation of left ventricular function in anesthetized patients using femoral artery dP/dt(max). J Cardiothorac Vasc Anesth. (2006) 20:325–30. doi: 10.1053/j.jvca.2005.11.006
22. Buitenwerf E, Boekel MF, van der Velde MI, Voogd MF, Kerstens MN, Wietasch GJKG, et al. The haemodynamic instability score. Eur J Anaesthesiol. (2019) 36:290–6. doi: 10.1097/EJA.0000000000000941
23. Davies SJ, Vistisen ST, Jian Z, Hatib F, Scheeren TWL. Ability of an arterial waveform analysis–derived hypotension prediction index to predict future hypotensive events in surgical patients. Anesth Analg. (2020) 130:352–9. doi: 10.1213/ANE.0000000000004121
Keywords: decision making, hemodynamics/physiology, blood pressure monitor, clinical decision support, hypotension, hypotension prediction
Citation: Ripollés-Melchor J, Valbuena-Bueno MA, Fernández-Valdés-Bango P, Rodríguez-Herrero A, Tomé-Roca JL, Olvera-García M, García-López D, Ruiz-Escobar A, Carrasco-Sánchez L, Abad-Gurumeta A, Lorente JV, Vargas-Berenjeno CA, Adell-Pérez AB, Espinosa ÁV, Jiménez-López I and Monge-García MI (2024) Characterization of intraoperative hemodynamic instability in patients undergoing general anesthesia. Front. Anesthesiol. 3:1405405. doi: 10.3389/fanes.2024.1405405
Received: 22 March 2024; Accepted: 22 May 2024;
Published: 25 June 2024.
Edited by:
Hong Liu, UC Davis Health, United StatesReviewed by:
Anais Caillard, Centre Hospitalier Regional Universitaire (CHU) de Brest, FranceHan Huang, Sichuan University, China
© 2024 Ripollés-Melchor, Valbuena-Bueno, Fernández-Valdés-Bango, Rodríguez-Herrero, Tomé-Roca, Olvera-García, García-López, Ruiz-Escobar, Carrasco-Sánchez, Abad-Gurumeta, Lorente, Vargas-Berenjeno, Adell-Pérez, Espinosa, Jiménez-López and Monge-García. This is an open-access article distributed under the terms of the Creative Commons Attribution License (CC BY). The use, distribution or reproduction in other forums is permitted, provided the original author(s) and the copyright owner(s) are credited and that the original publication in this journal is cited, in accordance with accepted academic practice. No use, distribution or reproduction is permitted which does not comply with these terms.
*Correspondence: Javier Ripollés-Melchor, cmlwbzU0MkBnbWFpbC5jb20=