- 1Department of Anesthesia, Infanta Leonor University Hospital, Madrid, Spain
- 2Universidad Complutense de Madrid, Madrid, Spain
- 3Fluid Therapy and Hemodynamic Monitoring Group of the Spanish Society of Anesthesiology and Critical Care, Madrid, Spain
- 4Department of Anesthesia, Juan Ramón Jiménez University Hospital, Huelva, Spain
- 5Department of Anesthesia, Virgen del Rocío University Hospital, Seville, Spain
- 6Department of Anesthesia, Althaia, Xarxa Assistencial Universitaria de Manresa, Manresa, Jerez de la Frontera, Spain
- 7Department of Critical Care, Jerez de la Frontera University Hospital, Spain
Intraoperative hypotension is common and has been associated with adverse events, including acute kidney failure, myocardial infarction, and stroke. Since blood pressure is a multidimensional and measurable variable, artificial intelligence and machine learning have been used to predict it. To date, studies have shown that the prediction and prevention of hypotension can reduce the incidence of hypotension. This review describes the development and evaluation of an artificial intelligence predictive algorithm called Hypotension Prediction (HPI), which can predict hypotension up to 15 min before it occurs.
Introduction
More than 300 million major surgical procedures are performed worldwide each year (1), most of which require general anesthesia. It is estimated that surgical-related deaths account for 7.7% of global mortality, accounting for the third largest reason for death just after ischemic heart disease and stroke (1). A better understanding and mitigation of this risk requires considering not only the condition or the surgical procedure but also the phenotypic response and the ability to cope with the surgical insult.
Fluid therapy is a crucial element of the hemodynamic optimization of surgical patients (2). The goal of intravenous fluid administration is to restore and maintain tissue fluid and electrolyte homeostasis and central euvolemia (3), avoiding excess salt and water (4). This facilitates tissue oxygenation without causing harm. Optimal intravenous fluid therapy should improve perioperative outcomes and is crucial in many perioperative guidelines (5) and pathways in cardiac (6) and non-cardiac surgery (7). Intravenous fluids should be administered in well-defined protocols according to the patient's needs (8).
Blood pressure (BP) is the primary determinant of organ perfusion (9). Normal BP allows autoregulation and adequate flow distribution according to the tissue needs (10). However, severe hypotension is common in patients undergoing surgery (11). So far, hypotension has been treated reactively after hypotensive values have already occurred. Clinicians can treat hypotension pre-emptively to reduce the occurrence or duration of episodes of hypotension. Clinical decision support systems designed to continuously monitor and identify patients at risk of developing hemodynamic instability can improve early recognition of the need for immediate support (12). In this review, we discuss a novel machine learning (ML) algorithm called “Hemodynamic Prediction Index” (HPI) (13), developed for predicting arterial hypotension and identifying the root source of the upcoming hypotensive event.
Goal-directed hemodynamic therapy, from reactive to proactive hemodynamic management
Treatment of low cardiac output (CO) and/or hypotension situations is usually reactive, which means treatment is generally initiated when the episode of hypotension, low CO, or the combination of both has already occurred. The emergence and integration of the so-called goal-directed hemodynamic therapy (GDHT) algorithms into clinical practice have allowed for more proactive management of patient hemodynamics (14). GDHT represents the standardization of the hemodynamic goals through a therapeutic protocol (15). However, treatment strategies differ substantially in terms of underlying target hemodynamic variables and target values, presumably having substantially different effects on the outcome. Therefore, pooling complex and substantially different hemodynamic therapeutical approaches under the term GDHT has recently been questioned, as it may constitute an oversimplification (16). GDHT usually refers to the optimization of flow-related parameters such as CO or stroke volume (SV) (17), and this optimization generally includes fluid therapy (18). GDHT assumes that optimal vascular volume improves cardiac performance, being “optimal” as the volume necessary to achieve the flat part of the Frank-Starling curve, which should be reflected in an improved microcirculation without reducing oxygen diffusion to tissues (15). However, only some studies have examined the behavior of microcirculation during major surgery. A study by Bouattour et al. concluded that preload dependence (defined as pulse pressure variation, PPV > 13%) was associated with reduced sublingual microcirculation, as assessed by sublingual microcirculatory variables such as microvascular flow index and density of perfused vessels, during major abdominal surgery. Fluid administration restored microvascular perfusion, while mean arterial pressure (MAP) and heart rate remained unchanged (19). The fact that microvascular changes were associated with episodes of preload dependence provides a valuable argument to guide fluid administration through dynamic indexes and correct preload dependence during surgery. Although perioperative hemodynamic optimization is based on cardiovascular monitoring, the ultimate goal of hemodynamic optimization is achieving adequate tissue perfusion (20). Regardless of their complexity, hemodynamic monitoring systems do not constitute medical treatment per se. They only provide clinical data that can aid decision-making and allow therapies to be individualized (21).
Early reports on critically ill patients showed substantial benefits from GDHT (22). Maximizing oxygen delivery to tissues in critically ill patients with a high metabolic rate or prior oxygen debt was useful (22). However, in surgical patients, the potential benefit of GDHT has been inconsistent (23, 24). As preoperative treatment of high-risk patients has been progressively improved (25), the latest studies have failed to show significant reductions in complications with the introduction of Enhanced Recovery After Surgery (ERAS) pathways (26–28). Nevertheless, systematic reviews have generally found that GDHT reduces the length of hospital stay (LOS) and the overall rate of postoperative complications (29). In contrast, estimates of mortality and organ-specific complications tend to favor GDHT with variable degrees of accuracy (30–32). For example, a recent meta-analysis including 76 studies showed that GDHT might reduce mortality {[odds ratio = 0.84; 95% confidence interval (CI), 0.64–1.09]} and shorten LOS (mean difference = −0.72 days; 95% CI: −1.10 to −0.35), but with low certainty in the evidence (30). Based on the encouraging results of clinical trials of GDHT, there has been a growing interest in the more proactive use of monitoring technologies to define the patient's physiologic state and manage it more proactively (15). Although the GDHT concept is primarily based on flow optimization, more recent hemodynamic algorithms add a MAP target ≥65 mm Hg or individually determined from preoperative baseline MAP to be achieved using vasopressors (32, 33). Accordingly, in general terms, the strategy is to optimize flow by fluid administration and then correct hypotension if present (34). The INPRESS trial showed that, among patients undergoing major abdominal surgery, treatment targeting an individualized systolic blood pressure (SBP) with SV optimization reduced the risk of postoperative organ dysfunction when compared to standard therapy (RR, 0.73; 95% CI: 0.56–0.94; p = 0.02; absolute risk difference, −14%, 95% CI: −25% to −2%) (35). On the other hand, some hemodynamic algorithms include target cardiac index (CI) values, which implies the administration of inotropes in non-preload dependent patients (36). In contrast to the “one-size-fits-all” approach, a personalized CI strategy could be more beneficial (37). In high-risk patients undergoing major abdominal surgery, a personalized hemodynamic management comprising strategies to maintain baseline CI using a GDHT algorithm decreased a composite outcome of major postoperative complications or death within 30 days after surgery compared with usual care [relative risk (RR): 0.54, 95% CI: 0.38–0.77; absolute risk reduction: −25.5%, 95% CI: −39.2% to −11.9%; p < 0.001] (38). Von Groote et al. combined data from the two PrevAKI trials involving 554 cardiac surgery patients at high risk of acute kidney injury (AKI), demonstrating the importance of achieving adequate systemic BP and CO to avoid postoperative AKI (39). The selection of hemodynamic targets remains controversial, but there is consensus that both BP and flow are essential determinants (40) of an adequate microcirculatory blood flow: a MAP value able to drive blood flow and a systemic blood flow that can meet the body's total oxygen requirements (41, 42). Therefore, monitoring BP and systemic blood flow on a beat-by-beat basis will ideally help optimize tissue perfusion. GDHT should always be tailored to individual needs (37). This assessment of the dynamic interactions of hemodynamic variables in response to a defined perturbation is referred to as functional hemodynamic monitoring (43). One of the challenges with GDHT algorithms that use fixed target values of a single variable or a combination of variables is that they do not consider individual needs (44). These should be further contextualized and individualized for a particular patient at a particular time by using variables that provide information on tissue oxygenation to provide a more comprehensive clinical picture (45). Unfortunately, how to monitor regional microcirculatory perfusion and tissue oxygenation directly at the bedside has not yet been solved. Whatever GDHT algorithm is used, physician compliance with protocols is imperative and must be closely monitored to improve postoperative outcomes (46).
Recognize hypotension as circulatory insufficiency
Although not mandatory for the definition of shock, hypotension is known to be the most consistent manifestation of decompensated shock leading to severe organ failure and death due to an inadequate tissular blood flow in relation to metabolic demands (47). As pointed out by Molnar et al. intraoperative hypotension (IOH) may be only the tip of the iceberg because although BP is ubiquitously measured during surgery, other signs are rarely recognized because they are not usually monitored (48). There is no universally agreed definition of intraoperative hypotension (IOH) (49), and there is even controversy as to whether IOH should be defined in terms of absolute or relative BP thresholds (50). Bijker et al. identified more than 140 different definitions of IOH in 130 studies. These definitions were either based on SBP or MAP values, absolute or relative changes, or a combination of the above (11). Both absolute and relative thresholds predict myocardial and AKI (51). However, absolute pressures appear to be as predictive as relative reductions over a wide range of clinically obtained basal pressures. For an absolute MAP threshold <65 mmHg, Bijker et al. observed an incidence of IOH ranging from 31% (duration of hypotension ≥10 min) to 65% (duration of hypotension ≥1 min) in a heterogeneous population of patients (11). Sha et al. showed that 88% of cases had at least one IOH event of MAP <65 for at least 1 min. with a mean cumulative hypotension duration ranging from 22.1 to 31.8 min after examining more than 20,000 adult patients ASA 3 and 4 undergoing major surgeries, with arterial line monitoring (52). The relationship between IOH and AKI varied depending on the underlying patient and the risk of the procedure. Low-risk patients did not show an increased associated risk of postoperative AKI across all BP ranges. In contrast, patients with the highest baseline risk demonstrated an association between IOH (defined as MAP between 55 and 59 mmHg at less than 10 min) and AKI (53). Regardless of the cause of the IOH, the risk of adverse events does not seem to be influenced by the moment in which the IOH occurs but by its severity and duration (54). Walsh et al. analyzed data from 33,330 patients undergoing noncardiac surgery. They evaluated the association between MAP below 55–75 mmHg and AKI or postoperative myocardial infarct, finding an incremental exposure-risk relationship in which a longer duration of MAP less than 55 mmHg increased the risk of AKI and myocardial infarct (55). In addition, 30-day mortality was significantly associated with more than 20 min of MAP of less than 55 mmHg (55). Although causality between perioperative hypotension and poor postoperative outcomes has not been established, a strong association between IOH, even if short, and increased postoperative morbidity.
Most studies, except a few exceptional ones (56–59), demonstrate that IOH during several noncardiac surgical procedures is associated with a variety of poor postoperative outcomes, including mortality (60–65), prolonged hospitalization (66, 67), AKI (54, 55, 68–71), myocardial injury (54, 55, 61, 64, 72–74), stroke (74–76) and postoperative delirium (77). A systematic review of 42 studies summarized reported risks of myocardial injury, acute kidney injury, and death depending on the severity and duration of IOH (78).
To determine IOH exposure, the duration and severity are usually expressed using the time-weighted average (TWA) under a MAP value below 65 mm Hg (TWA-MAP < 65). This TWAP-MAP < 65 incorporates the total incidence of IOH and is expressed relative to the entire duration of the monitoring time. The disjunction between hypotension and CI refers to the disconnect between low BP (hypotension) and a low CI. Kouz et al. collected data on MAP and CI in patients undergoing major abdominal surgery, and analyzed the relationship between these two variables. As expected, no clinically meaningful correlation between MAP and CI was found (79).
Arterial pressure is often used as a surrogate for blood flow (80). Arterial pressure and CI are both necessary measures of cardiovascular function, but they are not interchangeable. The changes in BP are not reliable surrogates for concomitant changes in CO (81). Thus, a comprehensive assessment of cardiovascular function should include both arterial pressure and CI measurements. Consequently, monitoring and managing both BP and systemic blood flow would help avoid organ hypoperfusion. Hypotension can arise both in low-flow states due to reduced circulating volume, in high-flow states due to vasodilatation and reduced afterload with preserved contractility, and in vasoplegic states with or without compromised contractility. The underlying hemodynamic monitoring variables that herald impending hypotension need to be clearly defined (82). To test the hypothesis that several hemodynamic alterations are underlying IOH, Kouz et al. used unsupervised ML, specifically clustering, in patients undergoing major abdominal surgery. They found six hemodynamic endotypes indicating that IOH is a reflection of altered intraoperative cardiovascular dynamics, being myocardial depression (characterized by reduced SV and CI and normal pulse pressure), the most commonly observed endotypes (83). The variability in responses to IOH is influenced by several factors, including age, health status, preoperative medications, type of surgery, and type of anesthesia. On the other hand, BP autoregulation is the cardiovascular system's ability to maintain BP within a narrow range, despite changes in blood flow or blood volume, through complex physiological mechanisms involving the cardiovascular, nervous, and endocrine systems. BP autoregulation can differ between patients or even in the same patient under different circumstances, which complicates the definition of a universal MAP threshold that ensures adequate perfusion pressure to the tissues for all patients under all conditions.
Hypotension prediction index: from proactive to predictive hemodynamic management
Hypotension prediction index: internal and external validation
The frequency, depth, and duration reduction of IOH likely decrease organ damage. Although simple, noninvasive continuous blood pressure monitoring in moderate- to high-risk surgical patients has been shown to reduce exposure to IOH compared to intermittent blood pressure monitoring (84, 85). Although observational studies have shown an association between IOH and poor postoperative outcomes, they were unable to clarify the impact of BP control on clinical outcomes. So far, few RCTs have specifically compared outcomes in noncardiac surgery (35, 86–88). These RCTs demonstrated that a higher BP target, compared to a lower one, does not lead to a worse outcome in patients undergoing noncardiac surgery and may lead to a superior outcome when intraoperative BP remains very closely matched to the patient's baseline value.
Among patients undergoing cardiac surgery, however, one RCT showed that the combined incidence of cardiac and neurological complications was significantly lower in the high MAP target group (80–100 mmHg) than in the low MAP group (50–60 mmHg) (89). Another RCT showed that a higher perfusion pressure (80–90 mmHg), compared with a lower target (60–70 mmHg), was associated with significantly lower postoperative cognitive dysfunction and delirium (90). The target for BP during surgery is not fixed and can vary based on several factors, including the patient's age, underlying health conditions, type of surgery, and the use of anesthesia. In general, the main goal is to maintain a BP that is sufficient to deliver oxygen and nutrients to the vital organs and tissues while minimizing the risk of bleeding and other complications (91). The current available evidence suggests that the duration and magnitude of SBP below 100 mm Hg and mean arterial pressures below 60–70 mm Hg during noncardiac surgery in adults are associated with organ injury.
The INPRESS trial conducted in patients undergoing major abdominal surgery demonstrated the benefit of maintaining perioperative BP close to the patient's baseline measurement (±10%) (35). Renal injury is understandable as the threshold for AKI appears to be higher than for myocardial injury, about 75 mm Hg rather than 65 mm Hg. It is also consistent with previous trials evidence for an association between BP control and AKI (92). Data on BP targets before and after cardiopulmonary bypass (CPB) during cardiac surgery are lacking. At this time, it appears prudent to use the criteria for non-cardiac surgery as a reference. For BP targets during CPB, it seems to be prudent to maintain a MAP value within 70–100 mmHg based on the aggregation of the RCTs published to date (89, 90, 93, 94).
Given that even brief episodes of IOH can be deleterious to the patient, the resulting need for a predictive model for IOH is evident. Early recognition of the progression of a hypotensive episode in a patient could lead to effective attenuation of hypotension and potentially better outcomes. In addition, current treatment protocols for hypotension may have unintended consequences, such as excessive fluid administration (95, 96).
Due to the current availability of big data and improved computing power, ML, a subset of Artificial intelligence, is developing rapidly with promising insights in medicine. The Hypotension Prediction Index is a monitoring tool based on ML and one of the first ML-derived predictive algorithms used in the perioperative period (13). The HPI algorithm was based on a supervised ML algorithm with the arterial pressure waveform as input, and the occurrence of hypotension (defined as a MAP <65 mmHg) and non-hypotension (MAP >75 mmHg) for at least 1 min, as the output variables. ML was used to refine further an algorithm based on the complex analysis of the characteristics of high-fidelity BP waveform recordings, resulting in a regression model that can predict a hypotensive episode (MAP < 65 mmHg for at least 1 min), regardless of the actual arterial pressure, up to 15 min in anticipation, with a sensitivity and specificity of 88% [95% CI: 85, 90%] and 87% [85, 90%] (13). The algorithm was developed (model training and cross-validation to fit the model) using waveforms recorded from EV100 (Edwards Lifesciences, Irvine, CA, United States) and Flotrac (Edwards lifesciences, Irvine, CA, United States) monitors from 35 sites worldwide from a cohort of 1,684 patients treated in the operating room and intensive care. The waveforms were then analyzed to extract waveform features by dividing the arterial pressure waveform into single beats and separating them into five sequential phases (systolic phase, diastolic phase, systolic rising phase, systolic falling phase, and overall falling phase). For each stage, several features were analyzed and calculated: duration, amplitude, area, and slope of the arterial pressure waveform; FloTrac algorithm-derived features; CO-Trek features; features related with the signal complexity, baroreflex, variability, and spectral features; “delta shift” features; and finally, combinatorial features. In this way, 3,022 individual characteristics were obtained. By performing a receiver operating characteristic (ROC) analysis for each feature, 51 unique arterial pressure characteristics, referred to as base features, were extracted. Only 23 features of the arterial waveform with the best predictive values were incorporated out of a possible combination of more than 2.6 million features.
The HPI algorithm was subjected to internal validation on 350 randomly selected cases from the dataset initially used to generate the algorithm. Afterward, it was externally validated on 204 cases of intensive care patients, which resulted in an algorithm of high accuracy with an area under the receiver operating characteristic curve (AUC) ranging from 0.95, 0.95, and 0.97 for 15, 10, and 5 min before a hypotensive event, respectively (13). Davies et al. performed a nonfunded HPI validation study to investigate the diagnostic ability of the HPI algorithm to predict IOH compared to other routinely collected hemodynamic variables such as MAP, SV, stroke volume variation (SVV), MAP, CO, PPV, systemic vascular resistance (SVR) and derivatives of these, such as the shock index or dynamic arterial elastance (Eadyn), during the perioperative period through monitoring with EV1000 containing the HPI software. In a retrospective study, they analyzed 292,025 perioperative data points in 255 patients undergoing major cardiac and noncardiac surgery. In this way, they evaluated the performance of the change in MAP in predicting hypotension and the absolute values of MAP, ΔMAP, CO, VS, pulse pressure, SVV, PPV, and SVR. The HPI algorithm reliably predicted a hypotensive episode up to 15 min before it occurred, and the predictive performance of the HPI was superior (AUC 0.879; 95% CI: 0.879–0.880) (97).
Development and testing of this initial HPI algorithm were based on invasive arterial line waveform data. Recently, Maheshwari et al. performed an external validation of the HPI algorithm using the non-invasive arterial waveform from a finger cuff and the volume clamp method (ClearSight, Edwards Lifesciences, Irvine, CA, United States). The HPI algorithm predicted IOH 5 min in advance, with a sensitivity of 0.86 [95% CI: 0.82, 0.89] and a specificity of 0.86 [95% CI: 0.82, 0.89]. At 10 min, the sensitivity was 0.83 [95% CI: 0.79, 0.86] and the specificity 0.83 [95% CI: 0.79, 0.86]. And at 15 min, the sensitivity was 0.75 [95% CI: 0.71, 0.80] and the specificity 0.75 [95% CI: 0.71, 0.80]. The positive predictive value of the algorithm's prediction at a threshold index of 85 was 0.83 [95% CI: 0.79, 0.87] (98). The predictive value of HPI in patients undergoing major vascular or cardiac surgery remains controversial when 85 was used as the cutoff value. Ranucci et al. presented a “symmetric” receiver operating characteristics curve with an AUC of 0.768 for the HPI ability to predict IOH (99). At the same time, Shin et al. reported an AUC for the HPI of 0.90 to predict IOH 5 min before the hypotensive event with high sensitivity and specificity in patients undergoing elective cardiac surgery requiring extracorporeal circulation (99).
The fact that the initial internal and external validation cohorts performed by Hatib et al. arbitrarily used a binary definition of hypotension (hypotensive events defined as MAP < 65 mmHg and non-hypotensive events described as MAP > 75 mmHg) with an intermediate gray zone of MAP 65–75 mmHg (13) has recently been criticized, suggesting that the AUC was biased toward a high specificity that could result in an overestimation of hypotension risk, with consequent overtreatment, leading the authors to suggest new validations (100).
Hypotension prediction index and the hemosphere software, more than hypotension prediction
HPI is a probability score and, a priori, not a physiological parameter, such as MAP, CO, or heart rate. However, the features defining HPI, even under disclosure, are intrinsically related to the physiological mechanisms that regulate and maintain constant BP for normal homeostasis. These mechanisms are mainly associated with the baroreflex control mediated by autonomic control (9). An HPI value ranging from 0 to 100 is calculated every 20 s and displayed on the monitor. It represents the probability that a patient will develop a hypotensive event, defined as a MAP less than 65 mmHg for at least 1 min (Figure 1). An audible alarm warns when the HPI exceeds 85. If the HPI value exceeds the upper limit of two consecutive measurements, a pop-up window invites the clinician to review the hemodynamic information about the patient's hemodynamic status, mainly concerning the preload dependency, afterload, and contractility. Thus, the potential value of HPI in practice is to provide real-time information to the clinician by allowing both proactive and predictive treatment of upcoming hypotensive events (101).
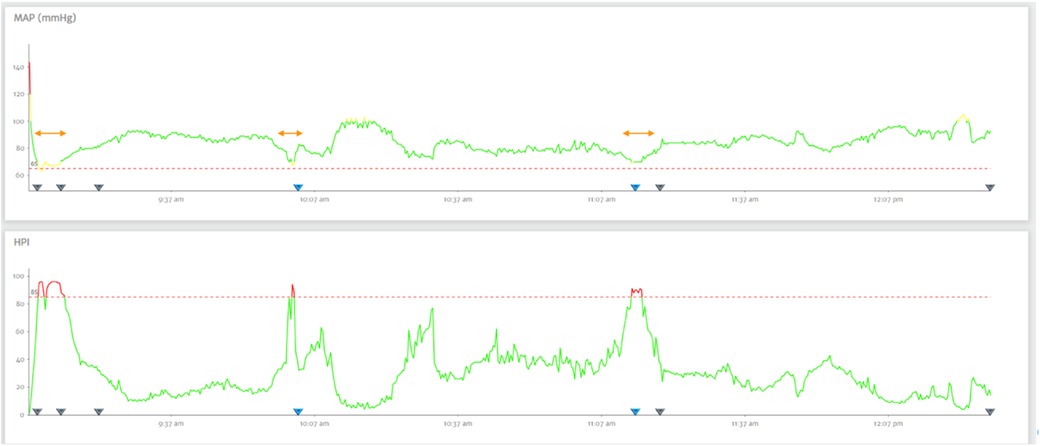
Figure 1. Example of the temporal evolution of HPI and mean arterial pressure in a surgical case. Legends: MAP, mean arterial pressure; HPI, hemodynamic prediction index.
The HPI algorithm developed by Hatib et al. was released as a feature of the Hemosphere clinical monitoring platforms (Edwards Lifesciences, Irvine, CA, United States) (13). Currently, it is possible to obtain it both through non-invasive monitoring (Acumen cuff, Edwards Lifesciences, Irvine, CA, United States) and minimally invasive through an arterial catheter (Acumen IQ, Edwards Lifesciences, Irvine, CA, United States). Significantly, this algorithm is based on detecting physiological signatures in arterial pressure waveforms caused by impaired cardiovascular compensatory mechanisms that occur before hypotension and involve all hemodynamic characteristics: cardiac preload, afterload, and contractility. The HPI generates a value ranging from 0 (i.e., no hypotension expected) to 100 (i.e., hypotensive event), where 85 is recommended as the threshold to initiate treatment. Using a lower HPI threshold provides a longer prediction time, but increases the false positive rate for the dichotomous variable hypotension, although it may evidence some offset hemodynamic instability. Higher HPI thresholds are associated with higher event rates, but with a shorter time to event, whereas lower thresholds have a lower event rate but a longer mean time to event. The optimal time for a ML-based hypotensive predictor to predict IOH events would depend on several factors, including the specific characteristics of the predictor and the clinical context in which it is being used. In general, the predictor should aim to predict hypotensive events as early as possible, in order to give healthcare providers sufficient time to intervene and prevent adverse outcomes. In the case of IOH, the ideal time for prediction would likely depend on the type of surgery being performed, the patient's comorbidities, and the specific definition of hypotension being used.
The approach of the HPI to predicting hypotensive events during surgery is to use real-time monitoring of patient vital signs and other physiological data, combined with machine learning algorithms that can detect patterns and predict the likelihood of hypotension occurring in the near future. In this case, the predictor may aim to provide alerts or warnings several minutes before a hypotensive event is expected to occur, in order to give healthcare providers time to adjust fluid or medication administration or take other corrective measures.
BP results from the interaction of blood flow and arterial load (102). Therefore, BP depends not only on flow rate but also on the modulation of the arterial system. Intravenous fluids, inotropes, or vasopressors can be administered in response to an elevated HPI, reflecting significant changes in SVV, Eadyn, or dP/dtmax. Therefore, the HPI is also an indicator of hemodynamic instability. A high HPI value requires specific treatment according to the hemodynamic variables. There is no single treatment that fits all patients.
Fluid administration is the first line of treatment for most patients with cardiovascular insufficiency (15), and is usually indicated in the presence of both of the following conditions: the patient requires an increase in perfusion (103); and the patient will increase his or her CO in response to fluid administration (104). However, volume expansion raises two main problems (105). SV increases by 10%–15% in only half of patients with cardiovascular insufficiency because the relationship between SV and cardiac preload is inconsistent. Second, positive fluid balance worsens the patient's outcome (3, 106). In addition, patients are assumed to become fluid deficient before surgery, due to the classic and common practice of nil by mouth after midnight, despite strong recommendations (107). Therefore, fluid responsiveness should be assessed before initiating volume expansion in patients with hemodynamic instability (108). The SVV [SVV = (SVmax − SVmin)/SVmean], which reflects the effects of the cyclic respiratory changes on venous return, was one of the first methods to be developed for the dynamic assessment of preload responsiveness (108). SVV is limited by the fact that they cannot be used in many clinical conditions, which can be easily remembered as the acronym LIMITS: Low heart rate- respiratory rate ratio (extremely high respiratory rate), leading to some false-negative results; irregular heartbeat (false positive); mechanical ventilation with low tidal volume (false-negative); increased abdominal pressure (false-positive), thorax open (false-negative); and spontaneous breathing (false-positive) (109). The cut-off values of SVV determining fluid responsiveness vary between 8% and 15% in different studies (110). Thus, a gray-zone range in which the predictive value is suboptimal should be anticipated. The “gray zone approach” proposed by Min et al. consists of increasing tidal volume to 8–12 ml/kg in individuals with SVV into the gray zone and showed that SVV changed to a more informative value (111). Hemodynamic parameters alter appreciably during pneumoperitoneum because of decreased thoracic compliance and increased transmission of airway pressure to the pleural space. So, the reliability of SVV as a predictor of fluid responsiveness in patients undergoing laparoscopic surgery remains controversial (112, 113). Nevertheless, a recent meta-analysis demonstrated that SVV could predict fluid responsiveness with a pooled AUROC for SVV > 0.8, suggesting that it is sufficiently reliable for predicting fluid responsiveness during laparoscopic surgery (114). Similarly, Han et al. found that SVV had good predictive performance in monitoring fluid responsiveness in patients undergoing cardiac surgery (AUC = 0.80) and intensive care after cardiac surgery (AUC = 0.89), while moderate predictive performance in patients during thoracic surgery (AUC = 0.73) (115).
The only reason to administer fluids to a patient is to increase SV. If this does not occur, fluid administration is useless and is likely deleterious (2). Moreover, fluid responsiveness is a normal physiologic condition not equivalent to hypovolemic. For this reason, GDHT algorithms have included so-called SV optimization as a fundamental part of the protocols, either by administering fluids until SV cannot increase or by an SVV value that suggests the patient is not a fluid responder (24). The FEDORA trial included low-moderate risk patients undergoing elective major noncardiac surgery by randomizing to a GDHT algorithm aimed primarily at maximizing SV but also guided to MAP and cardiac index (CI), with a significant reduction in postoperative complications (8.6% vs. 16.6%, p = 0.018) (36).
Similarly, one of the first studies in which the HPI was integrated into a hemodynamic algorithm used the presence of SVV > 12% as a cutoff for initiating fluid treatment in those cases in which HPI was >80%. FC was only repeated when HPI remained over 80% until SVV < 12% or HPI < 80% (116). Although the hemodynamic algorithm used by Schneck et al. is quite similar to that used in the FEDORA trial (36), it presents a clear difference: the use of the HPI to limit intraoperative fluid administration regardless of the presence of elevated SVV, which illustrates that many patients are physiologically volume responsive but are not unstable and that the mere presence of volume responsiveness does not imply circulatory failure (117), as indicated by a low HPI.
Moreover, a pathologic decrease in vasomotor tone (vasoplegia) is a common cause of IOH. Vasoplegia will limit the increase in MAP after fluid administration even though a patient responds to volume by increasing flow (118, 119). While fluid tolerance was recently suggested as the level at which the patient can tolerate fluid administration without causing organ dysfunction (117), the BP response cannot be easily predicted, even if a patient can increase CO with fluids (118). Consequently, it is necessary to assess preload dependence and arterial load to determine whether fluid administration will improve BP (120). Dynamic arterial elastance (Eadyn), computed as the ratio PPV/SVV, was initially conceived to determine whether a volume-responsive patient will also improve BP with volume administration (43). Monge et al. further confirmed the ability of Eadyn to predict arterial pressure response to volume expansion in preload-dependent patients with acute circulatory failure, and further propose that Eadyn may represent an index of coupling between the heart and the systemic circulation (121, 122). Various studies in mechanically ventilated (123) and spontaneously breathing patients (124) reported that an Eadyn <1.0 in a volume-responsive hypotensive patient predicts whose MAP will not increase with the increase in CO (125). A recent meta-analysis showed that Eadyn was a good predictor of MAP increases in response to fluid expansion, with an AUHSROC of 0.92 [95% CI: 0.89–0.94] (125).
Eadyn has also more recently been included as part of hemodynamic optimization protocols to reduce IOH. Therefore, in a hypotensive patient -or one with circulatory instability reflected by an elevated HPI- preload-dependent, i.e., with an elevated SVV, an Eadyn value >1 would reflect a cardiovascular system capable of increasing pressure with changes in flow (Figure 2), whereas an Eadyn <1 a system unable to increase MAP in response to an increase in SV following fluid administration (Figure 3). In this way, Wijnberge et al., using a hemodynamic algorithm adapted from Pinsky (43) included the changes in Eadyn with other variables for defining the hemodynamic condition and determining the adequate treatment when the high HPI describes the circulatory instability (12). In cases where an HPI > 80 was alerted, volume responders (ΔSVV) with a high Eadyn were defined as hypovolemic and treated with fluids. In contrast, patients with HPI > 80, with high ΔSVV and low Eadyn, were described as vasoplegic and received vasopressor treatment until HPI < 80% was achieved (12). This approach was also used recently by Murabito et al. (126). However, Tsoumpa et al., although using a similar GDHT algorithm with the HPI, they did not use the Eadyn to discriminate responding patients to the volume that would increase MAP after FC but to identify if a patient had vasoplegia or decreased contractility (127). This underscores that the information provided by the Hemosphere monitor must be adequately recognized and integrated. If Eadyn is high and the patient is preload-dependent, MAP will improve along with CO after FC. On the contrary, if Eadyn is low, even if the patient is fluid-responsive, CO increase will not improve MAP, and vasopressors should be considered to prevent IOH. Fluid administration will not increase MAP in non-preload dependent patients, regardless of their Eadyn (Figure 4). Furthermore, Eadyn does not represent a physical property of arterial load, such as arterial compliance or resistance, nor define an absolute measure of cardiac afterload, but a variable describing the dynamic interaction between pressure and flow (102).
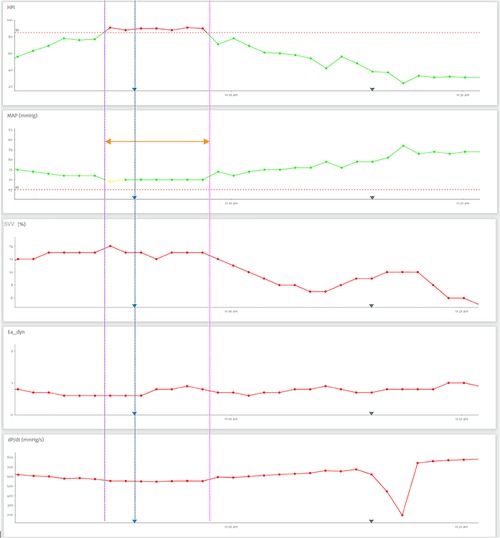
Figure 2. This figure shows a predicted hypotensive event (initiated in purple dashed line) with an elevated hypotension prediction index (HPI) in a preload-dependent patient (elevated stroke volume variation) with Eadyn >1. After fluid administration (blue dashed line) normalizing HPI and mean arterial pressure. (pink dashed line). Legends: MAP, mean arterial pressure; HPI, hemodynamic prediction index; SVV, stroke volume variation.
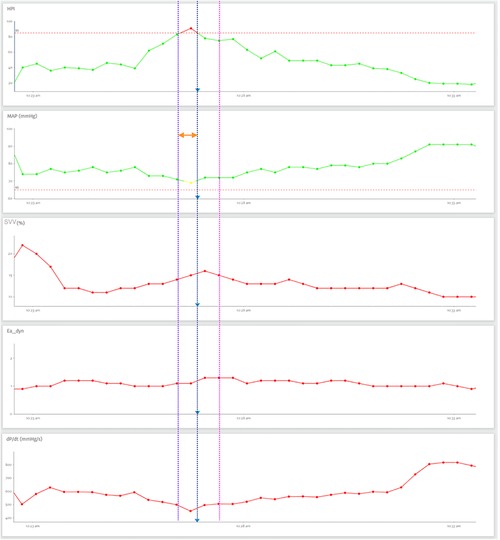
Figure 3. This case shows an occurrence of a hypotensive event prediction (initiated in the purple dashed line) with a high hypotension prediction index (HPI) in a preload dependent patient (high stroke volume variation) with Eadyn <1. After the administration of vasopressors (blue dashed line) HPI and mean arterial pressure normalizes (pink dashed line). Legends: MAP, mean arterial pressure; HPI, Hemodynamic prediction index; SVV, stroke volume variation.
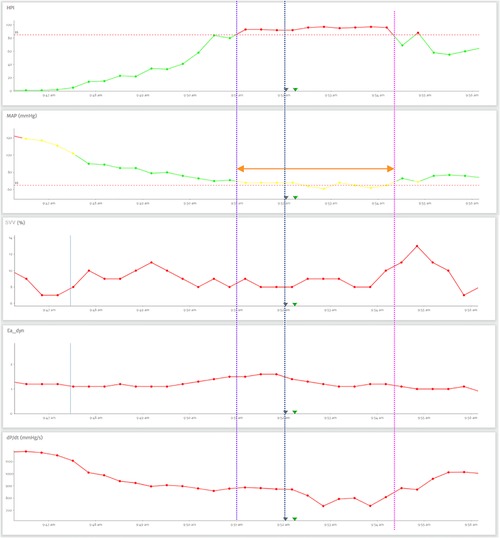
Figure 4. This case shows the occurrence of a hypotensive event prediction (initiated in the purple dashed line) with a high hypotension prediction index (HPI) in a non-dependent preload patient (low stroke volume variation) with normal dP/dtmax, after the administration of vasopressors (blue dashed line) HPI and mean arterial pressure normalizes (pink dashed line). Legends: MAP, mean arterial pressure; HPI, hemodynamic prediction index; SVV, stroke volume variation.
Unlike older algorithms, where dobutamine was administered to achieve supraphysiologic oxygen delivery targets (22), some recent GDHT algorithms have incorporated arbitrary CI targets (usually 2.5 L/min/m2 or basal CI) (36, 38). Optimal CI and related cardiovascular variables may differ according to age and the patient's hemodynamic status. Moreover, there is no normal or abnormal CO, but rather adequate or inadequate to the metabolic needs of a particular patient at a specific time. So it is not surprising that targeting a CI goal has resulted in inconsistent results. While one trial suggested that personalized CI-guided treatment maintaining baseline CI reduced postoperative complications in elective major abdominal surgery patients (38), in a more recent trial, this perioperative treatment did not improve patient outcomes (128). On the other hand, the CO value alone does not determine left ventricular (LV) performance and cardiac function (129). Current options for LV contractility assessment are considerably limited. The gold standard method for measurement of LV contractility (i.e., LV end-systolic elastance) (130) cannot be used in routine clinical practice because of its invasiveness. The maximum rate of LV pressure rise during ventricular contraction (LV dP/dtmax) has been extensively used as a surrogate marker of LV inotropic state and contractility (131). Although clinically feasible, it requires direct measurement of LV pressure, which is still highly invasive. Although LV dP/dtmax can also be estimated noninvasively by echocardiographic techniques (132), its measurement is not practical for continuous monitoring. Instead, arterial dP/dtmax can be calculated beat-to-beat from the invasive or noninvasive arterial pressure waveform (133). Monge et al. reported that arterial dP/dtmax was significantly correlated with LV contractility measured by Ees or LV dP/dtmax (134). On the other hand, it is essential to acknowledge that, unlike LV dP/dtmax, arterial dP/dtmax is measured during ventricular ejection and then it may also be influenced by peripheral arterial factors (135). Moreover, arterial dP/dtmax is more accurate for measuring LV contractility when adequate vascular filling has been achieved (136). Despite these limitations, the introduction of this parameter in hemodynamic algorithms allows precise identification of patients with impaired contractility causing circulatory failure treatable by inotropes (Figure 5). The threshold value of dP/dtmax at which circulatory failure will be manifested as hypotension and predicted as an HPI > 85% remains controversial, with some authors recommending a threshold of 400 mmHg/s (137) and others at 600 mmHg/s (127). In practice, the dP/dtmax trend allows knowing if the patient has decreased contractility. As indicated by Solares et al., in patients with HPI >85% and decreasing dP/dtmax, the administration of dobutamine not only improved dP/dtmax but also normalized HPI and prevented IOH (138). Interestingly, the use of dP/dtmax in randomized clinical trials (RCTs) conducted to date has not been used consistently. In the HYPE study, dP/dtmax was not used to warn of impaired contractility, rather it was a decrease in SV without changes in SVV and/or RVS that was considered suggestive of impaired LV contractility. Prevention of IOH may be detrimental to overtreatment, as indicated by Tsoumpa et al., in which in the HPI-guided group the time spent on hypertension increased (127). The possible overtreatment may result in clinicians not following the action suggested by an HPI-guided algorithm, as occurred in the Maheshwari et al. RCT, in which in cases where the suggested action was to administer dobutamine, this suggestion was in poor compliance. In fact, clinicians followed their own judgment about HPI alerts and intervened in only 45% of alerts, the point that clinicians followed their judgment explains why no differences in outcomes were observed (139). Using predictive technology requires proper use. It is unlikely that, if not used correctly, any predictive technology will lead to better results. So training in the technology, clear treatment guidance, and compliance-focused education should be an integral part of future RCT using HPI- guided algorithms.
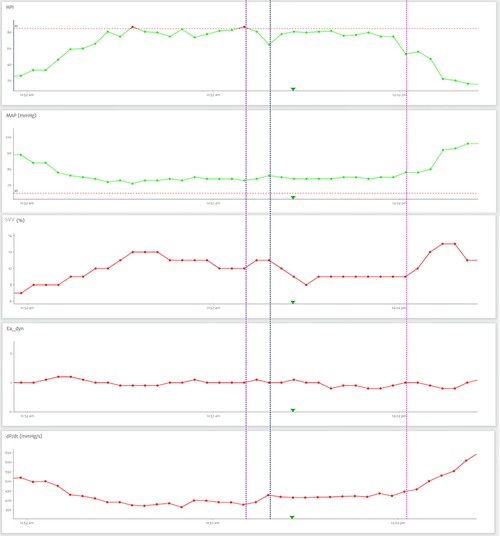
Figure 5. This example shows a prediction of a hypotensive event (initiated in the purple dashed line) with an elevated hypotension prediction index (HPI) in a preload in dependent patient (low stroke volume variation) with decreasing dP/dtmax. After the administration of inotrope (blue dashed line) HPI and mean arterial pressure normalizes (pink dashed line). Legends: MAP, mean arterial pressure; HPI, hemodynamic prediction index; SVV, stroke volume variation.
HPI-algorithm guided reduced hypotension exposition
A recent meta-analysis including five studies and 461 patients suggested that intraoperative HPI-guided hemodynamic management in adult patients undergoing noncardiac surgery led to a decrease in the TWA MAP < 65 mmHg (median difference 0.27 mmHg; 95% CI: −0.38, −0.01); compared with intraoperative hemodynamic management with or without GDHT that did not include HPI (140).
The first published RCT with HPI-guided GDHT showed a significant reduction in the incidence and the absolute and relative duration of hypotensive episodes in the HPI group compared to both control cohorts in patients undergoing hip replacement surgery (116). The HYPE study demonstrated that the application of a protocol of HPI-guided diagnostic guidance and hemodynamic treatment significantly reduced the IOH in adult patients undergoing major abdominal surgery compared to hemodynamic management based on CI, SVV, MAP, and SVR but not protocolized (TWA-MAP < 65 mmHg: 0.10 mm Hg (IQR, 0.01–0.43 mm Hg) vs. 0.44 mm Hg (IQR, 0.23–0.72 mm Hg) for a median difference of 0.38 mm Hg (95% CI: 0.14–0.43 mm Hg; p = 0.001) (12). In addition, the HPI-guided arm had a lower median hypotension time per patient of 8.0 min (IQR, 1.33–26.00 min) vs. 32.7 min (IQR, 11.5–59.7 min), with a median difference of 16.7 min (95% CI: 7.7–31.0 min; p < 0.001). Interestingly, there was no difference in the amount of vasoactive administered between the groups, along with no difference in intraoperative hypertension (TWA mean hypertension (defined as a MAP >100 mm Hg for at least 1 min) 0.09 mm Hg (IQR, 0.00–0.21 mm Hg) vs. 0.05 mm Hg (IQR, 0.00–0.13 mm Hg), suggests that HPI-guided hemodynamic management was not associated with overtreatment (12). Despite the promising results shown in the HYPE study, Maheshwari et al. demonstrated that patients who underwent moderate- to high-risk noncardiac surgery and were monitored with a noninvasive continuous BP (Clearsight) but with any predefined GDHT protocol had a TWA MAP < 65 mm Hg of 0.05 [0.00, 0.22] mm Hg (84). These results may suggest that the algorithm proposed by Wijnberge et al. (12) does not exploit the full potential of HPI-based monitoring. In contrast to previous studies, the largest RCT in patients undergoing major or moderate noncardiac surgery published to date found similar TWA-MAP <65 mmHg between the HPI-guided intervention group and standard care group receiving GDHT [0.14 vs. 0.14 mmHg, with a mean difference of 0 (95%, CI: −0.03 to 0.04), p = 0.757] (139). As previously discussed, these results have been attributed to the responsible clinicians' lack of compliance with the treatment protocol. In a post hoc analysis, HPI-guided treatment was associated with a lower incidence of IOH when the analysis was restricted to those episodes where clinicians intervened according to the HPI protocol (12).
A before-and-after study comparing a GDHT protocol with an HPI-guided GDHT protocol in patients undergoing major abdominal surgery showed that TWA was significantly decreased from 0.27 (0.42) mmHg to 0.10 (0.19) mmHg in the HPI group (p = 0.001) (141). Similar results in a similar group of patients were shown by Tsoumpa et al. (127). Two recent RCTs, in addition to demonstrating a reduction in intraoperative exposure to HPI, sought different outcomes. In the first one, an HPI-guided hemodynamic algorithm showed lower Neuronal Specific Enolase and higher reduced glutathione compared to the control group, while several other biomarkers including neutrophil gelatinase-associated lipocalin and S100B were correlated with TWA of IOH (126). Finally, Solares et al. were the first to report that an HPI-guided algorithm was significantly associated with reduced postoperative complications (0.46 ± 0.98 vs. 0.88 ± 1.20, p = 0.035) and LOS (median difference of 2 days, p = 0.019) compared to a GDHT algorithm in adult patients undergoing major abdominal surgery (142).
Evidence is currently lacking to determine whether the use of an HPI-guided hemodynamic algorithm leads to better postoperative outcomes, which limits the acquisition and implementation of the expensive monitors and consumables required to use these algorithms. However, it is important to note that the HPI is still a relatively new tool, so more research is required to determine if a low intraoperative HPI leads to a significant reduction in postoperative morbidity without increasing significantly costs. Evaluating the cost-effectiveness of ML-based prevention IOH, as the HPI, requires consideration of both the costs and benefits of the intervention. On the cost side, ML-based prevention IOH may require investments in technology infrastructure, data collection and management, and training for healthcare providers. These investments may be substantial, depending on the complexity of the intervention and the resources available for implementation. On the benefits side, ML-based prevention IOH has the potential to reduce the incidence of complications associated with IOH. Preventing these complications could lead to reduced healthcare costs, as patients require fewer resources and interventions to manage these complications. In addition, preventing complications could lead to improved patient outcomes, such as shorter LOS, improved quality of life, and reduced morbidity and mortality. To evaluate the cost-effectiveness ML-based prevention IOH, a framework such as cost-benefit analysis or cost-effectiveness analysis should be used. Cost-benefit analysis compares the total costs of the intervention to the total monetary value of its benefits, while cost-effectiveness analysis compares the costs of the intervention to its health outcomes or benefits, typically measured in quality-adjusted life-years (QALYs). In terms of cost-benefit analysis, the benefits of ML-based prevention IOH may be difficult to quantify in monetary terms, as they may include improved patient outcomes, reduced healthcare costs, and potential productivity gains. While, the cost-effectiveness of ML-based prevention IOH may vary depending on a range of factors, such as the prevalence of hypotensive events, the severity of associated complications, the effectiveness of the intervention in preventing complications, and the cost of implementing the intervention. However, in general, if the benefits of the intervention outweigh its costs, it may be considered cost-effective from an economic perspective. The cost and resources required may not be feasible for all institutions, particularly those with limited budgets or resources.
Evaluating the cost-effectiveness of ML-based prevention IOH, as the HPI, requires consideration of both the costs and benefits of the intervention. On the cost side, ML-based prevention IOH may require investments in technology infrastructure, data collection and management, and training for healthcare providers. These investments may be substantial, depending on the complexity of the intervention and the resources available for implementation. On the benefits side, ML-based prevention IOH has the potential to reduce the incidence of complications associated with IOH. Preventing these complications could lead to reduced healthcare costs, as patients require fewer resources and interventions to manage these complications. In addition, preventing complications could lead to improved patient outcomes, such as shorter LOS, improved quality of life, and reduced morbidity and mortality. To evaluate the cost-effectiveness ML-based prevention IOH, a framework such as cost-benefit analysis or cost-effectiveness analysis should be used. Cost-benefit analysis compares the total costs of the intervention to the total monetary value of its benefits, while cost-effectiveness analysis compares the costs of the intervention to its health outcomes or benefits, typically measured in quality-adjusted life-years (QALYs). In terms of cost-benefit analysis, the benefits of ML-based prevention IOH may be difficult to quantify in monetary terms, as they may include improved patient outcomes, reduced healthcare costs, and potential productivity gains. While, the cost-effectiveness of ML-based prevention IOH may vary depending on a range of factors, such as the prevalence of hypotensive events, the severity of associated complications, the effectiveness of the intervention in preventing complications, and the cost of implementing the intervention. However, in general, if the benefits of the intervention outweigh its costs, it may be considered cost-effective from an economic perspective. The cost and resources required may not be feasible for all institutions, particularly those with limited budgets or resources.
Relationship between MAP and HPI, future directions
Jacquet-Lagreze et al. investigated the value of linear extrapolation of MAP (LepMAP) for predicting IOH and found that the diagnostic performance in predicting IOH of LepMAP was significantly superior compared to MAP increase over time (143). Thus, some authors have suggested that the HPI curve is the mirror of the MAP curve, and a magnifier of MAP changes, alerting clinicians when MAP is approaching 65 mmHg (144). Monitoring a single parameter, however, does not fully describe the patient's complete status and can potentially lead to misinterpretations and underestimation of instability (145). As mentioned above, although HPI has not direct physiological meaning since it does not reflect any specific characteristic of the cardiovascular system, the features that define HPI are intrinsically related to the physiological factors that regulate BP for ensuring an adequate perfusion. A high HPI then implies that those compensatory mechanisms are being exhausted due to an increasing hemodynamic instability. The extenuation of these compensatory mechanisms eventually would lead to the occurrence of arterial hypotension. As expected, the evolution of the trends of HPI and MAP are closely related. So the lower the MAP, the higher the HPI. However, this relationship is not linear and may vary from one patient to another or even within the same patient (Figure 6). Moreover, the inverse sigmoidal function described by HPI and MAP closely resembles the inverse relationship between arterial pressure and the sympathetic activity (146). The scatter around this relationship (Figure 7) should therefore represent the level of different hemodynamic conditions associated with an HPI value in a single patient or the differences in the hemodynamic status when evaluating different patients. Therefore, the HPI would allow characterizing a certain hemodynamic state.
Another important feature of the HPI-MAP relationship is the usual identification of an inflection point in which the HPI increases more significantly, that may determine a point where the homeostatic mechanism accelerates until its extenuation when MAP is <65 mmHg (Figure 8). Interestingly, this critical threshold is usually identified when MAP is >65 mmHg and an the HPI lower than that defined by the manufacturer to predict arterial hypotension (<85). The clinical value of these observations are still pending but may represent an interesting and exciting field for research.
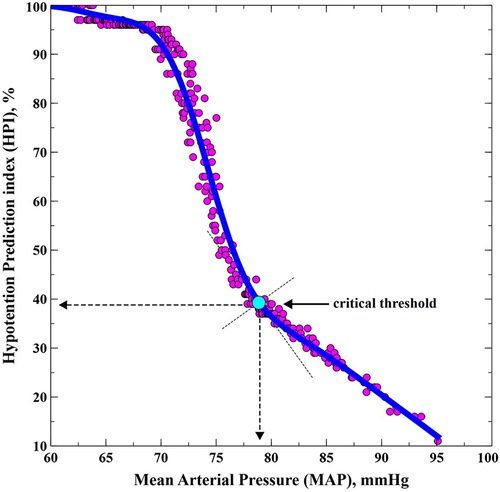
Figure 8. Example of the suggested critical point of physiological compensation of mean arterial blood pressure.
The HPI has the potential to significantly change the management of perioperative hemodynamics by enabling earlier detection of impending hypotensive events, leading to more timely interventions and potentially better patient outcomes. Traditionally, perioperative hemodynamics management has relied on reactive monitoring of patient vital signs, such as blood pressure and heart rate, with healthcare providers intervening only after hypotension has occurred. However, the HPI can analyze real-time patient data and predict the likelihood of hypotension occurring in the near future, allowing healthcare providers to intervene earlier and prevent hypotension from occurring. One potential benefit of the HPI is the ability to personalize hemodynamic management based on patient-specific factors. As mentioned, another potential benefit of the HPI is the ability to reduce the risk of adverse events associated with hypotension, such as myocardial injury, AKI, and prolonged LOS. By detecting impending hypotensive events earlier and intervening more quickly, healthcare providers can potentially prevent these adverse events from occurring or minimize their severity.
However, there are also challenges associated with implementing an HPI-based predictive hypotension in clinical practice, including issues related to data quality, model interpretability, and integration with clinical workflows. There is a risk of over-reliance on technology, where clinicians may become complacent and trust the algorithm to make all the decisions for them. This can lead to a lack of critical thinking and decision-making, which is a critical skill in perioperative medicine. On the other hand, there is currently no standardized approach to perioperative hemodynamics management, and different institutions may use different interventions for hypotension. ML algorithms may be limited in their ability to predict hypotension if the interventions used are not consistent across different institutions.
Ongoing research and development in this area will be needed to address these challenges and to fully realize the potential benefits of predictive hypotension in perioperative hemodynamics management.
Alternatives to prevent hypotension
There is ongoing research on the use of machine ML to predict hypotension and prevent its occurrence in critically ill patients. These ML-based models, which can help identify patients who are at high risk of developing hypotension and allow for earlier intervention to prevent its occurrence, could constitute an alternative to the HPI. There are several different approaches to predict IOH.
ML-approaches include supervised vs. unsupervised learning, feature engineering, algorithm selection using logistic regression, decision trees, random forests, support vector machines, neural networks and evaluation metrics which can be evaluated using various metrics such as accuracy, precision, recall, F1 score and area under the receiver operating characteristic (ROC) curve (147–156). Overall, the choice of machine learning approach for preventing IOH will depend on a variety of factors, including the size and complexity of the dataset, the availability of labeled data, and the specific goals of the ML algorithm (157).
One such model is the “early warning score” (EWS), which uses ML algorithms to identify patients who are at high risk of developing adverse clinical events such as hypotension. EWS is a composite score that takes into account various patient parameters such as heart rate, blood pressure, respiratory rate, and level of consciousness. The algorithm assigns a score to each parameter, and the total score is used to predict the likelihood of adverse clinical events (147). Another machine learning-based approach is the use of predictive analytics to identify patients who are at risk of developing hypotension. This involves analyzing large datasets of patient information to identify patterns and risk factors for hypotension (158). The algorithms can be trained on large datasets of patient information to identify patterns and risk factors for hypotension, which can be used to develop personalized treatment plans for individual patients (159). While these ML-based approaches hold great promise for predicting and preventing hypotension in critically ill patients, further validation and testing are required before they can be implemented into clinical practice.
Conclusions
The Hypotension Prediction Index has been shown to reduce exposure to intraoperative hypotension. However, it remains unproven whether the introduction of HPI improves patient-centered outcomes. On the other hand, there is a broad potential for research in physiological aspects related to HPI and other underexplored fields, such as cardiac surgery and intensive care.
Author contributions
Conceptualization, investigation, writing—original draft preparation JR-M. All authors contributed to the article and approved the submitted version.
Conflict of interest
JR-M: Payments for conferences and served as advisor board from Edwards Lifesciences. JVL: Payments for conferences and served as advisor board from Edwards Lifesciences. LC-S: Payments for conferences from Edwards Lifesciences. MIM-G: has been employee and is currently clinical consultant for Edwards Lifesciences. AR-E, PF-V-B, IJ-L and AA-G declare that this review was conducted in the absence of any commercial or financial relationships that could be construed as a potential conflict of interest. Edwards Lifesciences was not involved in the design, conduct of the review, collection, management, analysis, or interpretation of the data, preparation, and review of the manuscript, or in the decision to publish.
Publisher's note
All claims expressed in this article are solely those of the authors and do not necessarily represent those of their affiliated organizations, or those of the publisher, the editors and the reviewers. Any product that may be evaluated in this article, or claim that may be made by its manufacturer, is not guaranteed or endorsed by the publisher.
References
1. Weiser TG, Haynes AB, Molina G, Lipsitz SR, Esquivel MM, Uribe-Leitz T, et al. Estimate of the global volume of surgery in 2012: an assessment supporting improved health outcomes. Lancet. (2015) 385(Suppl 2):S11. doi: 10.1016/S0140-6736(15)60806-6
2. Vincent J-L, Cecconi M, De Backer D. The fluid challenge. Crit Care. (2020) 24(1):703. doi: 10.1186/s13054-020-03443-y
3. Malbrain MLNG, Langer T, Annane D, Gattinoni L, Elbers P, Hahn RG, et al. Intravenous fluid therapy in the perioperative and critical care setting: executive summary of the international fluid academy (IFA). Ann Intensive Care. (2020) 24(10):64. doi: 10.1186/s13613-020-00679-3
4. Shin CH, Long DR, McLean D, Grabitz SD, Ladha K, Timm FP, et al. Effects of intraoperative fluid management on postoperative outcomes: a hospital registry study. Ann Surg. (2018) 267:1084–92. doi: 10.1097/SLA.0000000000002220
5. Gustafsson UO, Scott MJ, Hubner M, Nygren J, Demartines N, Francis N, et al. Guidelines for perioperative care in elective colorectal surgery: enhanced recovery after surgery (ERAS ®) society recommendations: 2018. World J Surg. (2019) 43:659–95. doi: 10.1007/s00268-018-4844-y
6. Engelman DT, Ben Ali W, Williams JB, Perrault LP, Reddy VS, Arora RC, et al. Guidelines for perioperative care in cardiac surgery: enhanced recovery after surgery society recommendations. JAMA Surg. (2019) 154:755–66. doi: 10.1001/jamasurg.2019.1153
7. Thiele RH, Raghunathan K, Brudney CS, Lobo DN, Martin D, Senagore A, et al. American society for enhanced recovery (ASER) and perioperative quality initiative (POQI) joint consensus statement on perioperative fluid management within an enhanced recovery pathway for colorectal surgery. Perioper Med. (2016) 5:1–15. doi: 10.1186/s13741-016-0049-9
8. Miller TE, Myles PS. Perioperative fluid therapy for major surgery. Anesthesiology. (2019) 130:825–32. doi: 10.1097/ALN.0000000000002603
9. Magder S. The meaning of blood pressure. Crit Care. (2018) 22:257. doi: 10.1186/s13054-018-2171-1
10. Jacob M, Chappell D, Becker BF. Regulation of blood flow and volume exchange across the microcirculation. Crit Care. (2016) 20:319. doi: 10.1186/s13054-016-1485-0
11. Bijker JB, van Klei WA, Kappen TH, van Wolfswinkel L, Moons KGM, Kalkman CJ. Incidence of intraoperative hypotension as a function of the chosen definition: literature definitions applied to a retrospective cohort using automated data collection. Anesthesiology. (2007) 107:213–20. doi: 10.1097/01.anes.0000270724.40897.8e
12. Wijnberge M, Geerts BF, Hol L, Lemmers N, Mulder MP, Berge P, et al. Effect of a machine learning-derived early warning system for intraoperative hypotension vs standard care on depth and duration of intraoperative hypotension during elective noncardiac surgery: the HYPE randomized clinical trial. JAMA. (2020) 323:1052–60. doi: 10.1001/jama.2020.0592
13. Hatib F, Jian Z, Buddi S, Lee C, Settels J, Sibert K, et al. Machine-learning algorithm to predict hypotension based on high-fidelity arterial pressure waveform analysis. Anesthesiology. (2018) 129:663–74. doi: 10.1097/ALN.0000000000002300
14. Vincent J-L. Measure, interpret, apply—the MIA rule in critical care monitoring. Br J Anaesth. (2016) 117:557–8. doi: 10.1093/bja/aew306
15. Ripolles-Melchor J, Chappell D, Aya HD, Espinosa A, Mhyten MG, Abad-Gurumeta A, et al. Fluid therapy recommendations for major abdominal surgery. Via RICA recommendations revisited. Part II: goal directed hemodynamic therapy. Rationale for optimising intravascular volume. Rev Esp Anestesiol Reanim. (2017) 64:339–47. doi: 10.1016/j.redar.2017.02.009
16. Saugel B, Thomsen KK, Maheshwari K. Goal-directed haemodynamic therapy: an imprecise umbrella term to avoid. Br J Anaesth. (2023) 130:390–93. doi: 10.1016/j.bja.2022.12.022
17. Jans Ø, Tollund C, Bundgaard-Nielsen M, Selmer C, Warberg J, Secher NH. Goal-directed fluid therapy: stroke volume optimisation and cardiac dimensions in supine healthy humans. Acta Anaesthesiol Scand. (2008) 52:536–40. doi: 10.1111/j.1399-6576.2008.01585.x
18. Cove ME, Pinsky MR. Perioperative hemodynamic monitoring. Best Pract Res Clin Anaesthesiol. (2012) 26:453–62. doi: 10.1016/j.bpa.2012.10.003
19. Bouattour K, Teboul JL, Varin L, Vicaut E, Duranteau J. Preload dependence is associated with reduced sublingual microcirculation during major abdominal surgery. Anesthesiology. (2019) 130:541–9. doi: 10.1097/ALN.0000000000002631
20. Ince C. Hemodynamic coherence and the rationale for monitoring the microcirculation. Crit Care. (2015) 19(Suppl 3):S8. doi: 10.1186/cc14726
21. Ramsingh D, Alexander B, Cannesson M. Clinical review: does it matter which hemodynamic monitoring system is used? Crit Care. (2013) 17:208. doi: 10.1186/cc11814
22. Shoemaker WC, Appel PL, Kram HB. Hemodynamic and oxygen transport responses in survivors and nonsurvivors of high-risk surgery. Crit Care Med. (1993) 21:977–90. doi: 10.1097/00003246-199307000-00010
23. Michard F, Giglio MT, Brienza N. Perioperative goal-directed therapy with uncalibrated pulse contour methods: impact on fluid management and postoperative outcome. Br J Anaesth. (2017) 119:22–30. doi: 10.1093/bja/aex138
24. Benes J, Giglio M, Brienza N, Michard F. The effects of goal-directed fluid therapy based on dynamic parameters on post-surgical outcome: a meta-analysis of randomized controlled trials. Crit Care. (2014) 18:584. doi: 10.1186/s13054-014-0584-z
25. Cecconi M, Corredor C, Arulkumaran N, Abuella G, Ball J, Grounds RM, et al. Clinical review: goal-directed therapy-what is the evidence in surgical patients? The effect on different risk groups. Crit Care. (2013) 17:209. doi: 10.1186/cc11823
26. Ripolles-Melchor J, Casans-Frances R, Espinosa A, Abad-Gurumeta A, Feldheiser A, Lopez-Timoneda F, et al. Goal directed hemodynamic therapy based in esophageal doppler flow parameters: a systematic review, meta-analysis and trial sequential analysis. Rev Esp Anestesiol Reanim. (2016) 63:384–405. doi: 10.1016/j.redar.2015.07.009
27. Miller TE, Roche AM, Mythen M. Fluid management and goal-directed therapy as an adjunct to enhanced recovery after surgery (ERAS). Can J Anaesth. (2015) 62:158–68. doi: 10.1007/s12630-014-0266-y
28. Ripollés-Melchor J, Aldecoa C. Goal-directed hemodynamic therapy: neither for anyone, neither the same for everyone. Anesthesiology. (2018) 128:682–3. doi: 10.1097/ALN.0000000000002050
29. Som A, Maitra S, Bhattacharjee S, Baidya DK. Goal directed fluid therapy decreases postoperative morbidity but not mortality in major non-cardiac surgery: a meta-analysis and trial sequential analysis of randomized controlled trials. J Anesth. (2017) 31:66–81. doi: 10.1007/s00540-016-2261-7
30. Jessen MK, Vallentin MF, Holmberg MJ, Bolther M, Hansen FB, Holst JM, et al. Goal-directed haemodynamic therapy during general anaesthesia for noncardiac surgery: a systematic review and meta-analysis. Br J Anaesth. (2022) 128:416–33. doi: 10.1016/j.bja.2021.10.046
31. Ripolles-Melchor J, Espinosa A, Martinez-Hurtado E, Abad-Gurumeta A, Casans-Frances R, Fernandez-Perez C, et al. Perioperative goal-directed hemodynamic therapy in noncardiac surgery: a systematic review and meta-analysis. J Clin Anesth. (2016) 28:105–15. doi: 10.1016/j.jclinane.2015.08.004
32. Messina A, Robba C, Calabrò L, Zambelli D, Iannuzzi F, Molinari E, et al. Association between perioperative fluid administration and postoperative outcomes: a 20-year systematic review and a meta-analysis of randomized goal-directed trials in major visceral/noncardiac surgery. Crit Care. (2021) 25:43. doi: 10.1186/s13054-021-03464-1
33. Ripolles J, Espinosa A, Martinez-Hurtado E, Abad-Gurumeta A, Casans-Frances R, Fernandez-Perez C, et al. Intraoperative goal directed hemodynamic therapy in noncardiac surgery: a systematic review and meta-analysis. Braz J Anesthesiol. (2016) 66:513–28. doi: 10.1016/j.bjan.2015.02.002
34. Ripollés-Melchor J, Chappell D, Aya HD, Espinosa Á, Mhyten MG, Abad-Gurumeta A, et al. Fluid therapy recommendations for major abdominal surgery. Via RICA recommendations revisited. Part III: goal directed hemodynamic therapy. Rationale for maintaining vascular tone and contractility. Rev Esp Anestesiol Reanim. (2017) 64:348–59. doi: 10.1016/j.redar.2017.03.002
35. Futier E, Lefrant J-Y, Guinot P-G, Godet T, Lorne E, Cuvillon P, et al. Effect of individualized vs standard blood pressure management strategies on postoperative organ dysfunction among high-risk patients undergoing major surgery: a randomized clinical trial. JAMA. (2017) 318:1346–57. doi: 10.1001/jama.2017.14172
36. Calvo-Vecino JM, Ripollés-Melchor J, Mythen MG, Casans-Francés R, Balik A, Artacho JP, et al. Effect of goal-directed haemodynamic therapy on postoperative complications in low–moderate risk surgical patients: a multicentre randomised controlled trial (FEDORA trial). Br J Anaesth. (2018) 120:734–44. doi: 10.1016/j.bja.2017.12.018
37. De Backer D, Cecconi M, Chew MS, Hajjar L, Monnet X, Ospina-Tascón GA, et al. A plea for personalization of the hemodynamic management of septic shock. Crit Care. (2022) 26:372. doi: 10.1186/s13054-022-04255-y
38. Nicklas JY, Diener O, Leistenschneider M, Sellhorn C, Schön G, Winkler M, et al. Personalised haemodynamic management targeting baseline cardiac index in high-risk patients undergoing major abdominal surgery: a randomised single-centre clinical trial. Br J Anaesth. (2020) 125:122–32. doi: 10.1016/j.bja.2020.04.094
39. von Groote TC, Ostermann M, Forni LG, Meersch-Dini M, Zarbock A. The AKI care bundle: all bundle components are created equal-are they? Intensive Care Med. (2022) 48:242–5. doi: 10.1007/s00134-021-06601-0
40. Huber W, Zanner R, Schneider G, Schmid R, Lahmer T. Assessment of regional perfusion and organ function: less and non-invasive techniques. Front Med. (2019) 6:1–15. doi: 10.3389/fmed.2019.00050
41. Wolff CB, Collier DJ, Shah M, Saxena M, Brier TJ, Kapil V, et al. A discussion on the regulation of blood flow and pressure. Adv Exp Med Biol. (2016) 876:129–35. doi: 10.1007/978-1-4939-3023-4_16
42. Østergaard L, Granfeldt A, Secher N, Tietze A, Iversen NK, Jensen MS, et al. Microcirculatory dysfunction and tissue oxygenation in critical illness. Acta Anaesthesiol Scand. (2015) 59:1246–59. doi: 10.1111/aas.12581
43. Pinsky MR. Functional hemodynamic monitoring. Crit Care Clin. (2015) 31:89–111. doi: 10.1016/j.ccc.2014.08.005
44. Michard F, Biais M, Lobo SM, Futier E. Perioperative hemodynamic management 4.0. Best Pract Res Clin Anaesthesiol. (2019) 33:247–55. doi: 10.1016/j.bpa.2019.04.002
45. Saugel B, Vincent J-L, Wagner JY. Personalized hemodynamic management. Curr Opin Crit Care. (2017) 23:334–41. doi: 10.1097/MCC.0000000000000422
46. Boekel MF, Venema CS, Kaufmann T, van der Horst ICC, Vos JJ, Scheeren TWL. The effect of compliance with a perioperative goal-directed therapy protocol on outcomes after high-risk surgery: a before-after study. J Clin Monit Comput. (2021) 35:1193–202. doi: 10.1007/s10877-020-00585-w
47. Cecconi M, De Backer D, Antonelli M, Beale R, Bakker J, Hofer C, et al. Consensus on circulatory shock and hemodynamic monitoring. Task force of the European society of intensive care medicine. Intensive Care Med. (2014) 40:1795–815. doi: 10.1007/s00134-014-3525-z
48. Molnar Z, Benes J, Saugel B. Intraoperative hypotension is just the tip of the iceberg: a call for multimodal, individualised, contextualised management of intraoperative cardiovascular dynamics. Br J Anaesth. (2020) 125:419–23. doi: 10.1016/j.bja.2020.05.048
49. Schenk J, van der Ven WH, Schuurmans J, Roerhorst S, Cherpanath TG V, Lagrand WK, et al. Definition and incidence of hypotension in intensive care unit patients, an international survey of the European society of intensive care medicine. J Crit Care. (2021) 65:142–8. doi: 10.1016/j.jcrc.2021.05.023
50. Sun LY, Chung AM, Farkouh ME, van Diepen S, Weinberger J, Bourke M, et al. Defining an intraoperative hypotension threshold in association with stroke in cardiac surgery. Anesthesiology. (2018) 129:440–7. doi: 10.1097/ALN.0000000000002298
51. Sessler DI, Bloomstone JA, Aronson S, Berry C, Gan TJ, Kellum JA, et al. Perioperative quality initiative consensus statement on intraoperative blood pressure, risk and outcomes for elective surgery. Br J Anaesth. (2019) 122:563–74. doi: 10.1016/j.bja.2019.01.013
52. Shah NJ, Mentz G, Kheterpal S. The incidence of intraoperative hypotension in moderate to high risk patients undergoing non-cardiac surgery: a retrospective multicenter observational analysis. J Clin Anesth. (2020) 66:109961. doi: 10.1016/j.jclinane.2020.109961
53. Mathis MR, Naik BI, Freundlich RE, Shanks AM, Heung M, Kim M, et al. Preoperative risk and the association between hypotension and postoperative acute kidney injury. Anesthesiology. (2020) 132:461–75. doi: 10.1097/ALN.0000000000003063
54. Salmasi V, Maheshwari K, Yang D, Mascha EJ, Singh A, Sessler DI, et al. Relationship between intraoperative hypotension, defined by either reduction from baseline or absolute thresholds, and acute kidney and myocardial injury after noncardiac surgery: a retrospective cohort analysis. Anesthesiology. (2017) 126:47–65. doi: 10.1097/ALN.0000000000001432
55. Walsh M, Devereaux PJ, Garg AX, Kurz A, Turan A, Rodseth RN, et al. Relationship between intraoperative mean arterial pressure and clinical outcomes after noncardiac surgery: toward an empirical definition of hypotension. Anesthesiology. (2013) 119:507–15. doi: 10.1097/ALN.0b013e3182a10e26
56. Bijker JB, van Klei WA, Vergouwe Y, Eleveld DJ, van Wolfswinkel L, Moons KGM, et al. Intraoperative hypotension and 1-year mortality after noncardiac surgery. Anesthesiology. (2009) 111:1217–26. doi: 10.1097/ALN.0b013e3181c14930
57. Hsieh JK, Dalton JE, Yang D, Farag ES, Sessler DI, Kurz AM. The association between mild intraoperative hypotension and stroke in general surgery patients. Anesth Analg. (2016) 123:933–9. doi: 10.1213/ANE.0000000000001526
58. Hirsch J, DePalma G, Tsai TT, Sands LP, Leung JM. Impact of intraoperative hypotension and blood pressure fluctuations on early postoperative delirium after non-cardiac surgery. Br J Anaesth. (2015) 115:418–26. doi: 10.1093/bja/aeu458
59. Babazade R, Yilmaz HO, Zimmerman NM, Stocchi L, Gorgun E, Kessler H, et al. Association between intraoperative low blood pressure and development of surgical site infection after colorectal surgery: a retrospective cohort study. Ann Surg. (2016) 264:1058–64. doi: 10.1097/SLA.0000000000001607
60. Mascha EJ, Yang D, Weiss S, Sessler DI. Intraoperative mean arterial pressure variability and 30-day mortality in patients having noncardiac surgery. Anesthesiology. (2015) 123:79–91. doi: 10.1097/ALN.0000000000000686
61. Abbott TEF, Pearse RM, Archbold RA, Ahmad T, Niebrzegowska E, Wragg A, et al. A prospective international multicentre cohort study of intraoperative heart rate and systolic blood pressure and myocardial injury after noncardiac surgery: results of the VISION study. Anesth Analg. (2018) 126:1936–45. doi: 10.1213/ANE.0000000000002560
62. Monk TG, Bronsert MR, Henderson WG, Mangione MP, Sum-Ping STJ, Bentt DR, et al. Association between intraoperative hypotension and hypertension and 30-day postoperative mortality in noncardiac surgery. Anesthesiology. (2015) 123:307–19. doi: 10.1097/ALN.0000000000000756
63. Sprung J, Abdelmalak B, Gottlieb A, Mayhew C, Hammel J, Levy PJ, et al. Analysis of risk factors for myocardial infarction and cardiac mortality after major vascular surgery. Anesthesiology. (2000) 93:129–40. doi: 10.1097/00000542-200007000-00023
64. Sessler DI, Meyhoff CS, Zimmerman NM, Mao G, Leslie K, Vásquez SM, et al. Period-dependent associations between hypotension during and for four days after noncardiac surgery and a composite of myocardial infarction and death: a substudy of the POISE-2 trial. Anesthesiology. (2018) 128:317–27. doi: 10.1097/ALN.0000000000001985
65. Cheng X-Q, Wu H, Zuo Y-M, Mei B, Zhang L, Cai Y-Z, et al. Perioperative risk factors and cumulative duration of “triple-low” state associated with worse 30-day mortality of cardiac valvular surgery. J Clin Monit Comput. (2017) 31:387–95. doi: 10.1007/s10877-016-9856-2
66. Willingham MD, Karren E, Shanks AM, O’Connor MF, Jacobsohn E, Kheterpal S, et al. Concurrence of intraoperative hypotension, low Minimum alveolar concentration, and low bispectral index is associated with postoperative death. Anesthesiology. (2015) 123:775–85. doi: 10.1097/ALN.0000000000000822
67. Tassoudis V, Vretzakis G, Petsiti A, Stamatiou G, Bouzia K, Melekos M, et al. Impact of intraoperative hypotension on hospital stay in major abdominal surgery. J Anesth. (2011) 25:492–9. doi: 10.1007/s00540-011-1152-1
68. Sun LY, Wijeysundera DN, Tait GA, Beattie WS. Association of intraoperative hypotension with acute kidney injury after elective noncardiac surgery. Anesthesiology. (2015) 123:515–23. doi: 10.1097/ALN.0000000000000765
69. Charlson ME, MacKenzie CR, Gold JP, Ales KL, Topkins M, Shires GT. Intraoperative blood pressure. What patterns identify patients at risk for postoperative complications? Ann Surg. (1990) 212:567–80. doi: 10.1097/00000658-199011000-00003
70. Hallqvist L, Granath F, Huldt E, Bell M. Intraoperative hypotension is associated with acute kidney injury in noncardiac surgery: an observational study. Eur J Anaesthesiol. (2018) 35:273–9. doi: 10.1097/EJA.0000000000000735
71. Radak D, Neskovic M, Otasevic P, Isenovic ER. Renal dysfunction following elective endovascular aortic aneurysm repair. Curr Vasc Pharmacol. (2019) 17:133–40. doi: 10.2174/1570161115666171116163203
72. House LM, Marolen KN, St Jacques PJ, McEvoy MD, Ehrenfeld JM. Surgical apgar score is associated with myocardial injury after noncardiac surgery. J Clin Anesth. (2016) 34:395–402. doi: 10.1016/j.jclinane.2016.05.009
73. Hallqvist L, Mårtensson J, Granath F, Sahlén A, Bell M. Intraoperative hypotension is associated with myocardial damage in noncardiac surgery: an observational study. Eur J Anaesthesiol. (2016) 33:450–6. doi: 10.1097/EJA.0000000000000429
74. Sabaté S, Mases A, Guilera N, Canet J, Castillo J, Orrego C, et al. Incidence and predictors of major perioperative adverse cardiac and cerebrovascular events in non-cardiac surgery. Br J Anaesth. (2011) 107:879–90. doi: 10.1093/bja/aer268
75. Devereaux PJ, Yang H, Yusuf S, Guyatt G, Leslie K, Villar JC, et al. Effects of extended-release metoprolol succinate in patients undergoing non-cardiac surgery (POISE trial): a randomised controlled trial. Lancet. (2008) 371:1839–47. doi: 10.1016/S0140-6736(08)60601-7
76. Bijker JB, Persoon S, Peelen LM, Moons KGM, Kalkman CJ, Kappelle LJ, et al. Intraoperative hypotension and perioperative ischemic stroke after general surgery: a nested case-control study. Anesthesiology. (2012) 116:658–64. doi: 10.1097/ALN.0b013e3182472320
77. Wang N-Y, Hirao A, Sieber F. Association between intraoperative blood pressure and postoperative delirium in elderly hip fracture patients. PLoS ONE. (2015) 10(4):e0123892. doi: 10.1371/journal.pone.0123892. eCollection 201525860338
78. Wesselink EM, Kappen TH, Torn HM, Slooter AJC, van Klei WA. Intraoperative hypotension and the risk of postoperative adverse outcomes: a systematic review. Br J Anaesth. (2018) 121:706–21. doi: 10.1016/j.bja.2018.04.036
79. Kouz K, Bergholz A, Timmermann LM, Brockmann L, Flick M, Hoppe P, et al. The relation between mean arterial pressure and cardiac Index in Major abdominal surgery patients: a prospective observational cohort study. Anesth Analg. (2022) 134:322–9. doi: 10.1213/ANE.0000000000005805
80. Magder SA. The highs and lows of blood pressure: toward meaningful clinical targets in patients with shock. Crit Care Med. (2014) 42:1241–51. doi: 10.1097/CCM.0000000000000324
81. Monnet X, Marik PE, Teboul JL. Prediction of fluid responsiveness: an update. Ann Intensive Care. (2016) 6:111. doi: 10.1186/s13613-016-0216-7
82. Valeanu L, Bubenek-Turconi S-I, Ginghina C, Balan C. Hemodynamic monitoring in sepsis-A conceptual framework of macro- and microcirculatory alterations. Diagnostics (Basel). (2021) 11:1559. doi: 10.3390/diagnostics11091559
83. Kouz K, Brockmann L, Timmermann LM, Bergholz A, Flick M, Maheshwari K, et al. Endotypes of intraoperative hypotension during major abdominal surgery: a retrospective machine learning analysis of an observational cohort study. Br J Anaesth. (2023) 130:253–61. doi: 10.1016/j.bja.2022.07.056
84. Maheshwari K, Khanna S, Bajracharya GR, Makarova N, Riter Q, Raza S, et al. A randomized trial of continuous noninvasive blood pressure monitoring during noncardiac surgery. Anesth Analg. (2018) 127:424–31. doi: 10.1213/ANE.0000000000003482
85. Kouz K, Wegge M, Flick M, Bergholz A, Moll-Khosrawi P, Nitzschke R, et al. Continuous intra-arterial versus intermittent oscillometric arterial pressure monitoring and hypotension during induction of anaesthesia: the AWAKE randomised trial. Br J Anaesth. (2022) 129:478–86. doi: 10.1016/j.bja.2022.06.027
86. Williams-Russo P, Sharrock NE, Mattis S, Liguori GA, Mancuso C, Peterson MG, et al. Randomized trial of hypotensive epidural anesthesia in older adults. Anesthesiology. (1999) 91:926–35. doi: 10.1097/00000542-199910000-00011
87. Carrick MM, Morrison CA, Tapia NM, Leonard J, Suliburk JW, Norman MA, et al. Intraoperative hypotensive resuscitation for patients undergoing laparotomy or thoracotomy for trauma: early termination of a randomized prospective clinical trial. J Trauma Acute Care Surg. (2016) 80:886–96. doi: 10.1097/TA.0000000000001044
88. Wanner PM, Wulff DU, Djurdjevic M, Korte W, Schnider TW, Filipovic M. Targeting higher intraoperative blood pressures does not reduce adverse cardiovascular events following noncardiac surgery. J Am Coll Cardiol. (2021) 78:1753–64. doi: 10.1016/j.jacc.2021.08.048
89. Gold JP, Charlson ME, Williams-Russo P, Szatrowski TP, Peterson JC, Pirraglia PA, et al. Improvement of outcomes after coronary artery bypass. A randomized trial comparing intraoperative high versus low mean arterial pressure. J Thorac Cardiovasc Surg. (1995) 110:1302–4. doi: 10.1016/S0022-5223(95)70053-6
90. Siepe M, Pfeiffer T, Gieringer A, Zemann S, Benk C, Schlensak C, et al. Increased systemic perfusion pressure during cardiopulmonary bypass is associated with less early postoperative cognitive dysfunction and delirium. Eur J Cardio-Thoracic Surg. (2011) 40:200–7. doi: 10.1016/j.ejcts.2010.11.024
91. Meng L, Yu W, Wang T, Zhang L, Heerdt PM, Gelb AW. Blood pressure targets in perioperative care provisional considerations based on a comprehensive literature review. Hypertension. (2018) 72:806–17. doi: 10.1161/HYPERTENSIONAHA.118.11688
92. Tran PNT, Kusirisin P, Kaewdoungtien P, Phannajit J, Srisawat N. Higher blood pressure versus normotension targets to prevent acute kidney injury: a systematic review and meta-regression of randomized controlled trials. Crit Care. (2022) 26:364. doi: 10.1186/s13054-022-04236-1
93. Charlson ME, Peterson JC, Krieger KH, Hartman GS, Hollenberg JP, Briggs WM, et al. Improvement of outcomes after coronary artery bypass II: a randomized trial comparing intraoperative high versus customized mean arterial pressure. J Card Surg. (2007) 22:465–72. doi: 10.1111/j.1540-8191.2007.00471.x
94. Azau A, Markowicz P, Corbeau JJ, Cottineau C, Moreau X, Baufreton C, et al. Increasing mean arterial pressure during cardiac surgery does not reduce the rate of postoperative acute kidney injury. Perfusion. (2014) 29:496–504. doi: 10.1177/0267659114527331
95. Monnet X, Teboul J-L. Passive leg raising: five rules, not a drop of fluid! Crit Care. (2015) 14; 19(1):18. doi: 10.1186/s13054-014-0708-5
96. Favaron E, Montomoli J, Hilty MP, Ince C. Fluid management in the perioperative setting: mind the kidney. J Emerg Crit Care Med. (2019) 3:50–50. doi: 10.21037/jeccm.2019.08.09
97. Davies SJ, Vistisen ST, Jian Z, Hatib F, Scheeren TWL. Ability of an arterial waveform analysis–derived hypotension prediction index to predict future hypotensive events in surgical patients. Anesth Analg. (2020) 130:352–9. doi: 10.1213/ANE.0000000000004121
98. Maheshwari K, Buddi S, Jian Z, Settels J, Shimada T, Cohen B, et al. Performance of the hypotension prediction Index with non-invasive arterial pressure waveforms in non-cardiac surgical patients. J Clin Monit Comput. (2020) 35:71–8. doi: 10.1007/s10877-020-00463-5
99. Ranucci M, Barile L, Ambrogi F, Pistuddi V. Discrimination and calibration properties of the hypotension probability indicator during cardiac and vascular surgery. Minerva Anestesiol. (2019) 85:724–30. doi: 10.23736/S0375-9393.18.12620-4
100. Enevoldsen J, Vistisen ST. Performance of the hypotension prediction index may be overestimated due to selection bias. Anesthesiology. (2022) 137:283–9. doi: 10.1097/ALN.0000000000004320
101. Sidiropoulou T, Tsoumpa M, Griva P, Galarioti V, Matsota P. Prediction and prevention of intraoperative hypotension with the hypotension prediction index: a narrative review. J Clin Med. (2022) 11:5551. doi: 10.3390/jcm11195551
102. Monge García MI, Saludes Orduña P, Cecconi M. Understanding arterial load. Intensive Care Med. (2016) 42:1625–7. doi: 10.1007/s00134-016-4212-z
103. Gelman S, Bigatello L. The physiologic basis for goal-directed hemodynamic and fluid therapy: the pivotal role of the venous circulation. Can J Anaesth. (2018) 65:294–308. doi: 10.1007/s12630-017-1045-3
104. Kumar A, Anel R, Bunnell E, Zanotti S, Habet K, Haery C, et al. Preload-independent mechanisms contribute to increased stroke volume following large volume saline infusion in normal volunteers: a prospective interventional study. Crit Care. (2004) 8:R128–36. doi: 10.1186/cc2844
105. Bentzer P, Griesdale DE, Boyd J, MacLean K, Sirounis D, Ayas NT. Will this hemodynamically unstable patient respond to a bolus of intravenous fluids? JAMA. (2016) 316:1298–309. doi: 10.1001/jama.2016.12310
106. Malbrain MLNG, Van Regenmortel N, Saugel B, De Tavernier B, Van Gaal P-J, Joannes-Boyau O, et al. Principles of fluid management and stewardship in septic shock: it is time to consider the four D's and the four phases of fluid therapy. Ann Intensive Care. (2018) 8:66. doi: 10.1186/s13613-018-0402-x
107. Joshi GP, Abdelmalak BB, Weigel WA, Harbell MW, Kuo CI, Soriano SG, et al. American Society of Anesthesiologists Practice Guidelines for Preoperative Fasting: Carbohydrate-containing Clear Liquids with or without Protein, Chewing Gum, and Pediatric Fasting Duration-A Modular Update of the 2017 American Society of Anesthesiologists Practice Guidelines for Preoperative Fasting. Anesthesiology. (2023) 138:132–51. doi: 10.1097/ALN.0000000000004381
108. Marik PE, Cavallazzi R, Vasu T, Hirani A. Dynamic changes in arterial waveform derived variables and fluid responsiveness in mechanically ventilated patients: a systematic review of the literature. Crit Care Med. (2009) 37:2642–7. doi: 10.1097/CCM.0b013e3181a590da
109. Perel A. Using dynamic variables to guide perioperative fluid management. Anesthesiology. (2020) 133:929–35. doi: 10.1097/ALN.0000000000003408
110. Zhang Z, Lu B, Sheng X, Jin N. Accuracy of stroke volume variation in predicting fluid responsiveness: a systematic review and meta-analysis. J Anesth. (2011) 25:904–16. doi: 10.1007/s00540-011-1217-1
111. Min JJ, Gil N-S, Lee J-H, Ryu DK, Kim CS, Lee SM. Predictor of fluid responsiveness in the “grey zone”: augmented pulse pressure variation through a temporary increase in tidal volume. Br J Anaesth. (2017) 119:50–6. doi: 10.1093/bja/aex074
112. Høiseth LØ, Hoff IE, Myre K, Landsverk SA, Kirkebøen KA. Dynamic variables of fluid responsiveness during pneumoperitoneum and laparoscopic surgery. Acta Anaesthesiol Scand. (2012) 56:777–86. doi: 10.1111/j.1399-6576.2011.02641.x
113. Guinot P-G, de Broca B, Bernard E, Abou Arab O, Lorne E, Dupont H. Respiratory stroke volume variation assessed by oesophageal doppler monitoring predicts fluid responsiveness during laparoscopy. Br J Anaesth. (2014) 112:660–4. doi: 10.1093/bja/aet430
114. Chen J, Zhao S, Zhu Q. Reliability of stroke volume or pulse pressure variation as dynamic predictors of fluid responsiveness in laparoscopic surgery: a systematic review. J Clin Monit Comput. (2022) [Epub ahead of print]. doi: 10.1007/s10877-022-00939-6
115. Huan S, Dai J, Song S, Zhu G, Ji Y, Yin G. Stroke volume variation for predicting responsiveness to fluid therapy in patients undergoing cardiac and thoracic surgery: a systematic review and meta-analysis. BMJ Open. (2022) 12:e051112. doi: 10.1136/bmjopen-2021-051112
116. Schneck E, Schulte D, Habig L, Ruhrmann S, Edinger F, Markmann M, et al. Hypotension prediction index based protocolized haemodynamic management reduces the incidence and duration of intraoperative hypotension in primary total hip arthroplasty: a single centre feasibility randomised blinded prospective interventional trial. J Clin Monit Comput. (2020) 34:1149–58. doi: 10.1007/s10877-019-00433-6
117. Kattan E, Castro R, Miralles-Aguiar F, Hernández G, Rola P. The emerging concept of fluid tolerance: a position paper. J Crit Care. (2022) 71:154070. doi: 10.1016/j.jcrc.2022.154070
118. García MI M, González H B. Why did arterial pressure not increase after fluid administration? Med Intensiva. (2017) 41:546–9. doi: 10.1016/j.medin.2017.03.005
119. Pinsky MR, Cecconi M, Chew MS, De Backer D, Douglas I, Edwards M, et al. Effective hemodynamic monitoring. Crit Care. (2022) 26:294. doi: 10.1186/s13054-022-04173-z
120. Monge García MI, Jian Z, Hatib F, Settels JJ, Cecconi M, Pinsky MR. Dynamic arterial elastance as a ventriculo-arterial coupling Index: an experimental animal study. Front Physiol. (2020) 11:284. doi: 10.3389/fphys.2020.00284
121. Monge García MI, Guijo González P, Gracia Romero M, Gil Cano A, Rhodes A, Grounds RM, et al. Effects of arterial load variations on dynamic arterial elastance: an experimental study. Br J Anaesth. (2017) 118:938–46. doi: 10.1093/bja/aex070
122. Monge García MI, Santos A. Understanding ventriculo-arterial coupling. Ann Transl Med. (2020) 8:795. doi: 10.21037/atm.2020.04.10
123. Monge García MI, Gil Cano A, Gracia Romero M. Dynamic arterial elastance to predict arterial pressure response to volume loading in preload-dependent patients. Crit Care. (2011) 15:R15. doi: 10.1186/cc9420
124. Cecconi M, Monge García MI, Gracia Romero M, Mellinghoff J, Caliandro F, Grounds RM, et al. The use of pulse pressure variation and stroke volume variation in spontaneously breathing patients to assess dynamic arterial elastance and to predict arterial pressure response to fluid administration. Anesth Analg. (2015) 120:76–84. doi: 10.1213/ANE.0000000000000442
125. Zhou X, Pan W, Chen B, Xu Z, Pan J. Predictive performance of dynamic arterial elastance for arterial pressure response to fluid expansion in mechanically ventilated hypotensive adults: a systematic review and meta-analysis of observational studies. Ann Intensive Care. (2021) 11:119. doi: 10.1186/s13613-021-00909-2
126. Murabito P, Astuto M, Sanfilippo F, La Via L, Vasile F, Basile F, et al. Proactive management of intraoperative hypotension reduces biomarkers of organ injury and oxidative stress during elective non-cardiac surgery: a pilot randomized controlled trial. J Clin Med. (2022) 11(2):392. doi: 10.3390/jcm11020392
127. Tsoumpa M, Kyttari A, Matiatou S, Tzoufi M, Griva P, Pikoulis E, et al. The use of the hypotension prediction index integrated in an algorithm of goal directed hemodynamic treatment during moderate and high-risk surgery. J Clin Med. (2021) 10(24):5884. doi: 10.3390/jcm10245884
128. de Waal EEC, Frank M, Scheeren TWL, Kaufmann T, de Korte-de Boer D, Cox B, et al. Perioperative goal-directed therapy in high-risk abdominal surgery. A multicenter randomized controlled superiority trial. J Clin Anesth. (2021) 75:110506. doi: 10.1016/j.jclinane.2021.110506
130. Suga H, Sagawa K. Instantaneous pressure-volume relationships and their ratio in the excised, supported canine left ventricle. Circ Res. (1974) 35:117–26. doi: 10.1161/01.RES.35.1.117
131. Wallace AG, Skinner NSJ, Mitchell JH. Hemodynamic determinants of the maximal rate of rise of left ventricular pressure. Am J Physiol. (1963) 205:30–6. doi: 10.1152/ajplegacy.1963.205.1.30
132. Geyer H, Caracciolo G, Abe H, Wilansky S, Carerj S, Gentile F, et al. Assessment of myocardial mechanics using speckle tracking echocardiography: fundamentals and clinical applications. J Am Soc Echocardiogr. (2010) 3:351–5. doi: 10.1016/j.echo.2010.02.015
133. Morimont P, Lambermont B, Desaive T, Janssen N, Chase G, D’Orio V. Arterial dP/dtmax accurately reflects left ventricular contractility during shock when adequate vascular filling is achieved. BMC Cardiovasc Disord. (2012) 12:13. doi: 10.1186/1471-2261-12-13
134. Garcia MI M, Jian Z, Settels JJ, Hunley C, Cecconi M, Hatib F, et al. Performance comparison of ventricular and arterial dP/dt(max) for assessing left ventricular systolic function during different experimental loading and contractile conditions. Crit Care. (2018) 22:325. doi: 10.1186/s13054-018-2260-1
135. Tartiere J-M, Logeart D, Beauvais F, Chavelas C, Kesri L, Tabet J-Y, et al. Non-invasive radial pulse wave assessment for the evaluation of left ventricular systolic performance in heart failure. Eur J Heart Fail. (2007) 9:477–83. doi: 10.1016/j.ejheart.2006.11.005
136. Vaquer S, Chemla D, Teboul J-L, Ahmad U, Cipriani F, Oliva JC, et al. Volume infusion markedly increases femoral dP/dtmax in fluid-responsive patients only. Crit Care Med. (2020) 48:1487–93. doi: 10.1097/CCM.0000000000004515
137. Maheshwari K, Shimada T, Yang D, Khanna S, Cywinski JB, Irefin SA, et al. Hypotension prediction index for prevention of hypotension during moderate- to high-risk noncardiac surgery: a pilot randomized trial. Anesthesiology. (2020) 6:1214–22. doi: 10.1097/ALN.0000000000003557
138. Solares G, Barredo F, Monge García MI. The value of hypotensive prediction index and dP/dt(max) to predict and treat hypotension in a patient with a dilated cardiomyopathy. Rev Esp Anestesiol Reanim. (2020) 67:563–7. doi: 10.1016/j.redar.2020.02.011
139. Maheshwari K, Shimada T, Yang D, Khanna S, Cywinski JB, Irefin SA, et al. Hypotension prediction index for prevention of hypotension during moderate- to high-risk noncardiac surgery. Anesthesiology. (2020) 133:1214–22. doi: 10.1097/ALN.0000000000003557
140. Li W, Hu Z, Yuan Y, Liu J, Li K. Effect of hypotension prediction index in the prevention of intraoperative hypotension during noncardiac surgery: a systematic review. J Clin Anesth. (2022) 83:110981. doi: 10.1016/j.jclinane.2022.110981
141. Grundmann CD, Wischermann JM, Fassbender P, Bischoff P, Frey UH. Hemodynamic monitoring with hypotension prediction index versus arterial waveform analysis alone and incidence of perioperative hypotension. Acta Anaesthesiol Scand. (2021) 65:1404–12. doi: 10.1111/aas.13964
142. Solares GJ, Garcia D, Monge Garcia MI, Crespo C, Rabago JL, Iglesias F, et al. Real-world outcomes of the hypotension prediction index in the management of intraoperative hypotension during non-cardiac surgery: a retrospective clinical study. J Clin Monit Comput. (2023) 37:211–20. doi: 10.1007/s10877-022-00881-7
143. Jacquet-Lagrèze M, Larue A, Guilherme E, Schweizer R, Portran P, Ruste M, et al. Prediction of intraoperative hypotension from the linear extrapolation of mean arterial pressure. Eur J Anaesthesiol. (2022) 39:574–81. doi: 10.1097/EJA.0000000000001693
144. Michard F, Biais M, Futier E, Romagnoli S. Mirror, mirror on the wall, who is going to become hypotensive? Eur J Anaesthesiol. (2022) 40:42–4. doi: 10.1097/EJA.0000000000001740
145. Rahman A, Chang Y, Dong J, Conroy B, Natarajan A, Kinoshita T, et al. Early prediction of hemodynamic interventions in the intensive care unit using machine learning. Crit Care. (2021) 25:388. doi: 10.1186/s13054-021-03808-x
146. Monahan KD. Effect of aging on baroreflex function in humans. Am J Physiol Regul Integr Comp Physiol. (2007) 293:R3–12. doi: 10.1152/ajpregu.00031.2007
147. Smith GB, Prytherch DR, Meredith P, Schmidt PE, Featherstone PI. The ability of the national early warning score (NEWS) to discriminate patients at risk of early cardiac arrest, unanticipated intensive care unit admission, and death. Resuscitation. (2013) 84:465–70. doi: 10.1016/j.resuscitation.2012.12.016
148. Nemati S, Holder A, Razmi F, Stanley MD, Clifford GD, Buchman TG. An interpretable machine learning model for accurate prediction of sepsis in the ICU. Crit Care Med. (2018) 46:547–53. doi: 10.1097/CCM.0000000000002936
149. Mathis MR, Engoren MC, Williams AM, Biesterveld BE, Croteau AJ, Cai L, et al. Prediction of postoperative deterioration in cardiac surgery patients using electronic health record and physiologic waveform data. Anesthesiology. (2022) 137:586–601. doi: 10.1097/ALN.0000000000004345
150. Prashanth A, Chakravarthy M, George A, Mayur R, Hosur R, Pargaonkar S. Sympatho-vagal balance, as quantified by ANSindex, predicts post spinal hypotension and vasopressor requirement in parturients undergoing lower segmental cesarean section: a single blinded prospective observational study. J Clin Monit Comput. (2017) 31:805–11. doi: 10.1007/s10877-016-9906-9
151. Zhao A, Elgendi M, Menon C. Machine learning for predicting acute hypotension: a systematic review. Front Cardiovasc Med. (2022) 9:937637. doi: 10.3389/fcvm.2022.937637
152. Sun Y, Rashedi N, Vaze V, Shah P, Halter R, Elliott JT, et al. Predicting future occurrence of acute hypotensive episodes using noninvasive and invasive features. Mil Med. (2021) 186(Suppl 1):445–51. doi: 10.1093/milmed/usaa418
153. Cherifa M, Blet A, Chambaz A, Gayat E, Resche-Rigon M, Pirracchio R. Prediction of an acute hypotensive episode during an ICU hospitalization with a super learner machine-learning algorithm. Anesth Analg. (2020) 130:1157–66. doi: 10.1213/ANE.0000000000004539
154. Yoon JH, Jeanselme V, Dubrawski A, Hravnak M, Pinsky MR, Clermont G. Prediction of hypotension events with physiologic vital sign signatures in the intensive care unit. Crit Care. (2020) 24:661. doi: 10.1186/s13054-020-03379-3
155. Lee S, Lee HC, Chu YS, Song SW, Ahn GJ, Lee H, et al. Deep learning models for the prediction of intraoperative hypotension. Br J Anaesth. (2021) 126:808–17. doi: 10.1016/j.bja.2020.12.035
156. Choe S, Park E, Shin W, Koo B, Shin D, Jung C, et al. Short-term event prediction in the operating room (STEP-OP) of five-minute intraoperative hypotension using hybrid deep learning: retrospective observational study and model development. JMIR Med Inform. (2021) 9:e31311. doi: 10.2196/31311
157. Jo YY, Jang JH, Kwon JM, Lee HC, Jung CW, Byun S, et al. Predicting intraoperative hypotension using deep learning with waveforms of arterial blood pressure, electroencephalogram, and electrocardiogram: retrospective study. PLoS ONE. (2022) 17:e0272055. doi: 10.3390/s22093108
158. Lee S, Lee M, Kim SH, Woo J. Intraoperative hypotension prediction model based on systematic feature engineering and machine learning. Sensors (Basel). (2022) 22:3108. doi: 10.3390/s22093108
Keywords: blood pressure, blood pressure determination/methods, hemodynamics*, hypotension/epidemiology, hypotension/prevention & control*, machine learning
Citation: Ripollés-Melchor J, Ruiz-Escobar A, Fernández-Valdes-Bango P, Lorente JV, Jiménez-López I, Abad-Gurumeta A, Carrasco-Sánchez L and Monge-García MI (2023) Hypotension prediction index: From reactive to predictive hemodynamic management, the key to maintaining hemodynamic stability. Front. Anesthesiol. 2:1138175. doi: 10.3389/fanes.2023.1138175
Received: 5 January 2023; Accepted: 13 March 2023;
Published: 13 April 2023.
Edited by:
Tamás Végh, University of Debrecen, HungaryReviewed by:
Aveek Jayant, Homi Bhabha Cancer Hospital and Research Centre, IndiaManuel Granell, Independent Researcher, Valencia, Spain
© 2023 Ripollés-Melchor, Ruiz-Escobar, Fernández-Valdes-Bango, Lorente, Jiménez-López, Abad-Gurumeta, Carrasco-Sánchez and Monge-García. This is an open-access article distributed under the terms of the Creative Commons Attribution License (CC BY). The use, distribution or reproduction in other forums is permitted, provided the original author(s) and the copyright owner(s) are credited and that the original publication in this journal is cited, in accordance with accepted academic practice. No use, distribution or reproduction is permitted which does not comply with these terms.
*Correspondence: Javier Ripollés-Melchor cmlwbzU0MkBnbWFpbC5jb20=
†These authors have contributed equally to this work
Specialty Section: This article was submitted to Cardiothoracic Anesthesiology, a section of the journal Frontiers in Anesthesiology