- Evaluation Intelligence Department, L’Oréal R&I, Kawasaki, Kanagawa, Japan
Cosmetic researchers recruit consumers to evaluate new formulas as part of the product development process. This screens out poorly performing formulas in favor of better ones for further testing. Trained experts score new formulas on a battery of sensory attributes until a few formulas are selected for more costly, blind-use tests (BUTs) featuring randomly recruited consumers. Once formulas pass a BUT, they are ready for commercialization. Resources would be more efficiently used if BUT results could be predicted from earlier rounds of testing. However, predicting the relationship between sensory testing and BUT testing is limited by the lack of data in common between the two methods. Even though hundreds of consumer responses are recorded, only their means are merged into the set of data used for analysis. This reduces the amount of data available for decision-making and introduces the challenges associated with analyzing small samples. This paper proposes improving on this mean-based approach by adding bootstrapping when combining sensory expert responses with BUT responses. It compares the BUT predictions captured via bootstrapping versus the predictions obtained using only the means from the original data sets.
1 Introduction
Protecting skin from sunlight-related damage is an important way to reduce the appearance of aging and a growing portion of the cosmetics market (Hughes et al., 2013). Consumers spent an estimated $8.5 billion on sun care cosmetics in 2022, with sales expected to reach $16 billion by 2030, based on an 8.3% compound annual growth rate (CAGR) estimate (Grand View Research, 2024). This growth is driven by rising awareness of the benefits of photoprotection over time, as shown by research in the United States on middle and high school students who demonstrated increasing use of sunscreen from 2007 to 2019 (Rajagopal et al., 2021).
To remain competitive in this growing market, product researchers work to improve the sensorial attributes of photoprotection products without sacrificing their UV-shielding efficacy. New formulations are measured on their sensorial attributes and categorized by their different sensory profiles. Researchers use these categories to benchmark new formulas against sensorially similar known market winners and to look for promising sensorial white space.
After sensorial testing is completed, the most promising formulas are approved for more costly, blind-use testing that features randomly recruited samples of current photoprotection product consumers. This test is usually the last step in validating a new formula, as scoring significantly higher than competing formulas in a blind-use test remains one of the best ways to eliminate confirmation bias (Kardish et al., 2015).
Being able to accurately predict a BUT result based on data collected from a sensorial panel would significantly improve the process of validating new formulas. Researchers would know in advance how a new formula performs sensorially and would adjust it before proceeding to the final stage of BUT validation. However, these sorts of predictions are made difficult thanks to the lack of a common dataset on which to base the analysis work. Test subjects and protocols are completely different, so the data must first be merged in an unbiased way.
A common approach to combining these two types of tests is to first calculate means by formula and then pair them across the test results (Dijksterhuis, 1995). This approach to merging and analyzing data hails back to the days of Pearson and is called data fusion, as carefully documented by Marcoulides (2017) in her thesis. However, even though many sensory and blind-use responses are recorded, only a single mean from each test remains for predictive analysis. Thus, the BUT prediction comes from a small (often fewer than 30) sample of means and carries with it all the risks associated with a small sample size.
This paper describes one method for increasing the reliability of predictions made from small sample sizes, such as these, through a re-sampling technique known as bootstrapping. Bootstrapping is used in a variety of ways, such as to make better use of electronic health record (EHR) databases when researching clinical events and diseases (Wanyan et al., 2021; Garg and Shah, 2016), to build sufficiently large databases for the training of machine learning algorithms (Hu et al., 2023), to assess the feasibility of different controlled study designs (Sengupta et al., 2023), and to use real-world data (RWD) for clinical trial simulation (CTS) in preparation for conducting actual trials (Chen et al., 2021). This paper compares BUT predictions made using the means-based approach with those made using the bootstrapping approach.
2 Materials and methods
Research on photoprotection formulas was conducted between 2018 and 2021 in a series of eight BUTs held in China and Spain. Twenty-one of the formulas in these BUTs were matched against previously executed sensorial test results. This combined data set was then used to build a linear regression model to predict the BUT results of four newly created formulas based on their performance in sensory testing. These predictions were compared against the actual study results of the four new photo-protection formulas tested in the 2023 BUT.
Agreement with the descriptive statement “Lets skin breathe” was chosen as the attribute to predict based on research showing a correlation (R = 0.51) with how well consumers liked each formula overall. It is abbreviated as Breath and was measured using a 5-point Likert rating scale (Likert, 1932). The sensorial attributes of Slipperiness on the skin, Penetration of the formula into the skin, and Greasiness of the formula on the skin were chosen as predictive attributes based on their supposed relationship to Breath. These three attributes are abbreviated as Slipperiness, Penetration, and Greasiness and are based on a 15-point line (Gomide et al., 2021) rating scale. Descriptive statistics for these variables are calculated in Table 1 for both the original means-based dataset and the bootstrapped dataset.
Regression analysis was used to predict the BUT attribute Breath by using Slipperiness, Penetration, and Greasiness as regressors, as shown in Table 2. The results are significant—each of the sensory attributes is significant with p < 0.05. The model passes the Shapiro–Wilks test for normality and the F test for model fit with p < 0.05. R2 is below 0.5, but a literature review shows that an R2 between 0.10 and 0.50 is acceptable when “some or most of the explanatory variables are statistically significant” for data obtained from human behavior (Ozili, 2022), which is the case here.
However, there are reasons to be concerned about the predictive capability of the model because of its small sample size. Here is where the bootstrapping technique provided useful, additional understanding. As background, bootstrapping is a commonly applied technique for managing around the difficulties of small sample sizes (Wright and Field, 2011) by resampling the underlying data that were originally summarized. Through bootstrapping, the means were calculated anew and then randomly paired from the sensory data to the BUT data hundreds of times. The results from these randomly resampled means were then analyzed to understand the likelihood of the regression result, given the distribution of the underlying data. Bootstrapping the predictive model this way provided confidence intervals for regression coefficients and goodness of fit measures based on the empirical distribution of the estimates drawn from all the available data.
Bootstrapping was performed using the Bayesian bootstrapping technique proposed by Rubin (Rubin, 1981), which is implemented by the bayesboot (Bååth, 2018) library package accessible through the R software (R Core Team, 2023) environment. The regression analysis was calculated using the lm procedure, and confidence intervals for each statistic were calculated using the hdi function from the bayestestR (Makowski et al., 2019) library, also accessible through R. Finally, the prediction was compared to the actual research conducted in 2023 on the new formulas.
3 Results
The bootstrapped regression results are shown in Table 3. Despite the significant results obtained for each dependent variable when performing regression using the original means, the bootstrapped results show that the highest density intervals (hdi) for F and R2 calculations were close to 0 on the low end and included 0 when adjusted R2 was measured.
Table 4 compares the results for Breath calculated via the bootstrapped prediction model versus the results calculated using the original means-based model. The prediction based on the bootstrapped data was closer to the actual data in three of the four new formulas tested. This is not surprising—bootstrap estimates, which are based on the empirical distribution of the coefficients, are known to be more robust and potentially less biased than the corresponding estimates from classical theory-based approaches.
To visualize the difference between the two predictions, an independent regression analysis was performed against Breath for each of the three dependent variables. These results differ from those of the regression analysis shown in Tables 2, 3 because they are univariate regression analyses rather than multivariate. Even so, these plots illustrate how the bootstrapped result differed from the original means-based result. In these univariate visualizations, the regression coefficients are consistently smaller for the bootstrapped results, which is consistent with the differences in the multivariate analysis. Additionally, the means of the 2023 test results are visualized, so the difference in the predictions can also be seen via two regression line plots.
3.1 Key to visualizations
In the visualizations, colored circles with white centers indicate the 2023 test result means for the new formulas, and circles with black centers indicate the prior results that the prediction was based on. Each small colored dot indicates a randomly matched bootstrapped mean. The black dashed line indicates the regression line based on the original means, and the blue dashed line indicates the regression line based on the bootstrapped data. The thin, solid blue lines indicate regression lines drawn from the 95% highest density interval for the regression coefficient.
Figure 1 illustrates the relationship between Penetrates and Breath. Three of the four 2023 formula results are higher than expected, and the relationship is maintained.
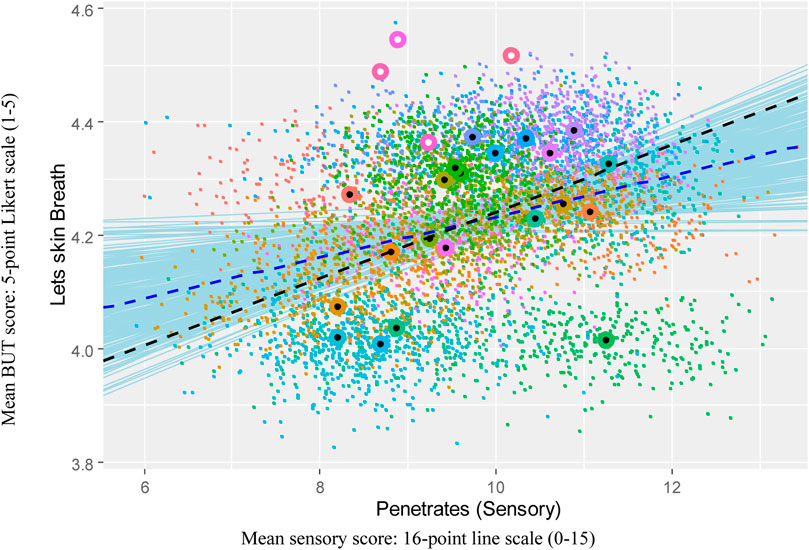
Figure 1. Visualization of univariate regression analysis of Penetrates against Breath with 2023 formula means.
Figure 2 illustrates the relationship between Penetrates and Slipperiness. Three of the four 2023 formula results are higher than expected, and the relationship is maintained.
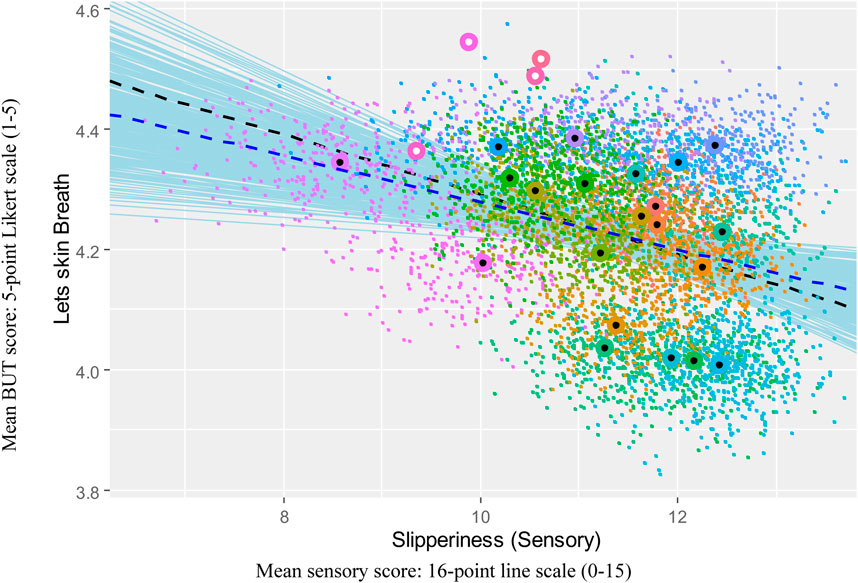
Figure 2. Visualization of univariate regression analysis of Slipperiness against Breath with 2023 formula means.
Figure 3 illustrates the relationship between Penetrates and Greasiness. Three of the four 2023 formula results are higher than expected, and the relationship is cast into doubt.
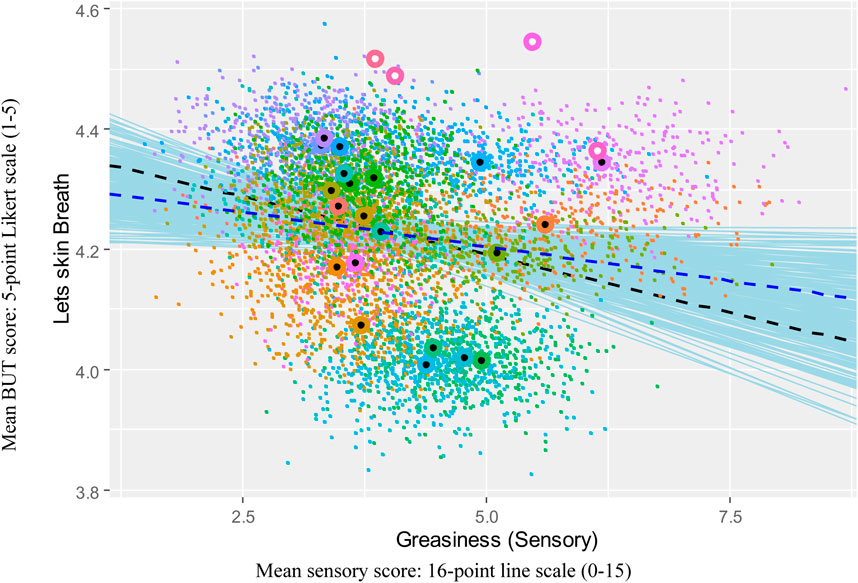
Figure 3. Visualization of univariate regression analysis of Greasiness against Breath with 2023 formula means.
4 Conclusion
The classical approach using the 21 means calculated for each formula yielded statistically significant results for the three sensory attributes and for the overall regression model, as shown in Table 2. These results proved less robust than they initially appeared when data obtained from the most recent 2023 BUT scored beyond the predictions of the regression model, as shown in Table 4. Even though the original means-based regression met all the criteria for model fit, the bootstrapped analysis result led to smaller coefficients of regression and a tighter confidence interval that yielded predictions that deviated less from the actual 2023 data. Bootstrapping improved on the assumptions of the classical approach and provided a clearer picture of the risk of prediction error by calculating the highest density interval for each statistic. This approach addressed the risks associated with small sample sizes by returning to the larger sample of data on which the original means were based. In this way, the BUT database of 4,980 responses and the sensorial database of 412 responses were more fully utilized to make the predictions.
This improved understanding of predictive accuracy is crucial in the fast-paced cosmetics industry, where timely and reliable product validation can significantly enhance competitive advantage. The bootstrapping approach not only mitigates the risks associated with small sample sizes but also leverages the richness of the underlying data, paving the way for more informed and efficient product development cycles. Further research could explore the integration of additional sensory attributes into the predictive model, as well as the application of this method to other product categories within the cosmetics industry.
5 Discussion
As can be seen from the visualizations, the new formulas from the 2023 BUT scored higher than expected, given their sensory results. In fact, three of the four new formulas earned the highest mean scores in the entire data set. However, these new high scores for breathability did not correspond well with the scores in the sensorial evaluations. While the higher BUT scores are a natural result of continuously improving the formulas over the 5-year period studied, they may also reflect improvements to the breathability of the new formulas that remain uncaptured in the scope of current sensory evaluations. Additional sensorial measures may be needed to capture the root causes of these gains.
The bootstrapped coefficients improved on the original means-based prediction, but they were more useful in describing the precision of the prediction itself. Despite significant results for all three dependent variables in the original means-based regression analysis, the predictions were substantially lower than the actual results. Slipperiness and Penetration remain valuable predictors; the predictive relevance of Greasiness should be re-evaluated given the recent BUT outcome. The results from the 2023 BUT illustrate the peril of making predictions from a small sample size and the need to continuously update predictive models to align with evolving consumer preferences.
Although Bayesian bootstrapping was used in this case, the wealth of data available to sample from suggests that any reasonable bootstrapping approach would have yielded similar results. The results from the Bayesian approach were more convenient because of the smoother outcome they tend to generate and the ease of using the bayesboot package.
An additional class of techniques that could be useful in this type of analysis are measurement error correction models, such as those provided in the mecor (Nab et al., 2021) package. These techniques are particularly powerful when validation studies are part of the data. Specifically designed validation studies could be added to the sensorial data at a relatively low cost to enable the use of this approach. This is an improvement opportunity to suggest to sensory evaluation teams to further reduce measurement error.
Data availability statement
The data analyzed in this study are subject to the following licenses/restrictions: The data largely come from our China organization. Recent changes to privacy laws in China make sharing any datasets from China impossible. Internal privacy teams are currently reviewing the options regarding data sharing, but at the time of writing, it is not a possibility. Requests to access these datasets should be directed to YWFyb24ucGluZ0Bsb3JlYWwuY29t.
Ethics statement
The studies involving humans were approved by the L’Oreal Research & Innovation Evaluation Intelligence visa committee. The studies were conducted in accordance with the local legislation and institutional requirements. The participants provided their written informed consent to participate in this study. Written informed consent was not obtained from the individual(s) for the publication of any potentially identifiable images or data included in this article because no personally identifiable information (PII) data are included in this research project.
Author contributions
AP: writing–original draft and writing–review and editing.
Funding
The author(s) declare that financial support was received for the research, authorship, and/or publication of this article. This work was funded by L’Oréal Research and Innovation. The funder was not involved in the study design, collection, analysis, interpretation of data, the writing of this article, or the decision to submit it for publication.
Acknowledgments
The author thanks Philippe Bastien and Vahid Masuda for their mentorship and advice during the writing and proofreading of this article.
Conflict of interest
AP is an employee of Nihon L’Oréal KK, a wholly owned subsidiary of L’Oréal SA.
Publisher’s note
All claims expressed in this article are solely those of the authors and do not necessarily represent those of their affiliated organizations, or those of the publisher, the editors, and the reviewers. Any product that may be evaluated in this article, or claim that may be made by its manufacturer, is not guaranteed or endorsed by the publisher.
References
Bååth, R. (2018). Bayesboot: an implementation of rubin's (1981) bayesian bootstrap. R. package version 0.2.2. doi:10.32614/CRAN.package.bayesboot
Chen, Z., Zhang, H., Guo, Y., George, T. J., Prosperi, M., Hogan, W. R., et al. (2021). Exploring the feasibility of using real-world data from a large clinical data research network to simulate clinical trials of Alzheimer’s disease. npj Digit. Med. 4, 84. doi:10.1038/s41746-021-00452-1
Dijksterhuis, G. (1995). Multivariate data analysis in sensory and consumer science: an overview of developments. Trends Food Sci. & Technol. 6 (6), 206–211. doi:10.1016/S0924-2244(00)89056-1
Garg, D., and Shah, J. (2016), A bootstrap machine learning approach to identify rare disease patients from electronic health records. doi:10.48550/arXiv.1609.01586
Gomide, S., Nascimento, , Nascimento, M., Minim, L. A., and Minim, V. P. R. (2021). Study of the influence of line scale length (9 and 15 cm) on the sensory evaluations of two descriptive methods. J. Food Sci. Technol. 58, 2815–2824. doi:10.1007/s13197-020-04890-9
Grand View Research (2024). Sun care cosmetics market size, share & trends report 2023-2030. Available at: https://www.grandviewresearch.com/industry-analysis/sun-care-cosmetics-market-report.
Hu, R., Luo, K., and Gupta, L. (2023), REBOOT: reuse data for bootstrapping efficient real-world dexterous manipulation. doi:10.48550/arXiv.2309.03322
Hughes, W., Baker, G., Baker, P., and Green, A. C. (2013). Sunscreen and prevention of skin aging. Ann. Intern. Med. 158 (11), 781–790. doi:10.7326/0003-4819-158-11-201306040-00002
Kardish, M., Mueller, U. G., Amador-Vargas, S., Dietrich, E., Ma, R., Barrett, B., et al. (2015). Blind trust in unblinded observation in ecology, evolution, and behavior. Front. Ecol. Evol. 3. doi:10.3389/fevo.2015.00051
Makowski, D., Ben-Shachar, M. S., and Lüdecke, D. (2019). bayestestR: describing effects and their uncertainty, existence and significance within the bayesian framework. J. Open Source Softw. 4 (40), 1541. doi:10.21105/joss.01541
Marcoulides, K. M. (2017). A bayesian synthesis approach to data fusion using augmented data-dependent priors. Multivar. Behav. Res. 52 (1), 111–112. doi:10.1080/00273171.2016.1263927
Nab, van S., Keogh, G., Keogh, R. H., and Groenwold, R. H. H. (2021). Mecor: an R package for measurement error correction in linear regression models with a continuous outcome. Comput. Methods Programs Biomed. 208, 106238. doi:10.1016/j.cmpb.2021.106238
Ozili, P. K. (2022). The acceptable R-square in empirical modelling for social science research. SSRN. Available at: https://api.semanticscholar.org/CorpusID:249858498
Rajagopal, T., Chuy, C., Cheng, A. L., and Dall, L. (2021). Trends in sunscreen use among US middle and high school students, 2007-2019. Cureus 13 (7), e16468. doi:10.7759/cureus.16468
R Core Team (2023). R: a language and environment for statistical computing. Vienna, Austria: R Foundation for Statistical Computing. Available at: https://www.R-project.org/.
Rubin, D. B. (1981). The bayesian bootstrap. Ann. Statistics 9 (1), 130–134. doi:10.1214/aos/1176345338
Sengupta, S., Ntambwe, I., Tan, K., Liang, Q., Paulucci, D., Castellanos, E., et al. (2023). Emulating randomized controlled trials with hybrid control arms in oncology: a case study. Clin. Pharmacol. & Ther. 113 (4). doi:10.1002/cpt.2841
Wanyan, Z., Ding, A., and Wang, G. (2021). Bootstrapping your own positive sample: contrastive learning with electronic health record data. Proc. Mach. Learn. Res. doi:10.48550/arXiv.2104.02932
Keywords: bootstrap, UV, cosmetic science, sensory analysis, Bayesian, regression
Citation: Ping A (2024) Predicting blind-use test (BUT) results from sensory testing using Bayesian bootstrapping. Front. Anal. Sci. 4:1414039. doi: 10.3389/frans.2024.1414039
Received: 08 April 2024; Accepted: 16 October 2024;
Published: 14 November 2024.
Edited by:
Jeroen Jansen, Radboud University, NetherlandsReviewed by:
Chunfen Jin, Honeywell UOP, United StatesJosé Andrés Herrera-Corredor, Colegio de Postgraduados - Campus Córdoba, Mexico
Copyright © 2024 Ping. This is an open-access article distributed under the terms of the Creative Commons Attribution License (CC BY). The use, distribution or reproduction in other forums is permitted, provided the original author(s) and the copyright owner(s) are credited and that the original publication in this journal is cited, in accordance with accepted academic practice. No use, distribution or reproduction is permitted which does not comply with these terms.
*Correspondence: Aaron Ping, YWFyb24ucGluZ0Bsb3JlYWwuY29t