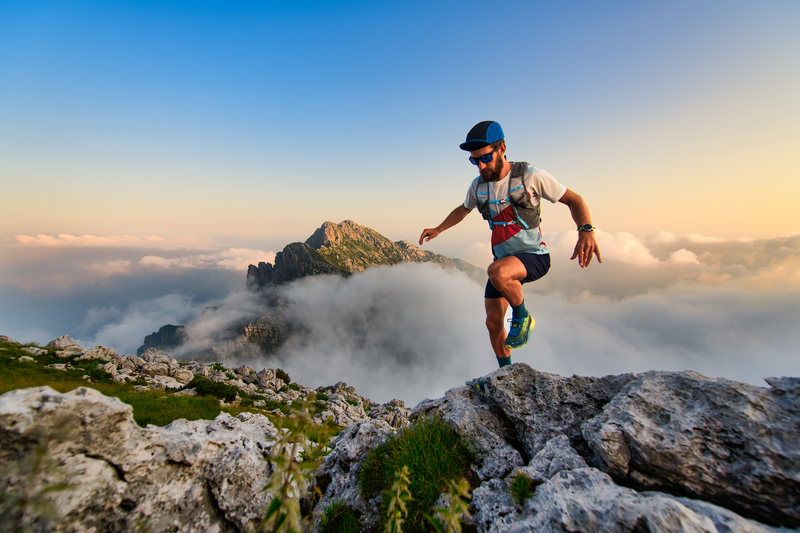
94% of researchers rate our articles as excellent or good
Learn more about the work of our research integrity team to safeguard the quality of each article we publish.
Find out more
MINI REVIEW article
Front. Anal. Sci. , 23 March 2023
Sec. Omics
Volume 3 - 2023 | https://doi.org/10.3389/frans.2023.1157582
This article is part of the Research Topic Perspectives in Omics 2022 View all 7 articles
Hundreds of molecular species make up the cellular lipidome. In this minireview, considerations for interpreting membrane and storage lipid profile changes that are often the focal point of lipidomic studies are discussed. In addition, insights how the most conserved molecular patterns are formed in eukaryotic systems and the consequences for the perturbation of lipid homeostasis are addressed. The implications of lipid identification specificity and experimental variability on modeling membrane structure and systemic responses are also discussed. The profile changes of membrane and storage lipids are bound to the kinetics of the metabolic system, and experimental design and functional interpretation in lipidomic research should be adapted accordingly.
Lipids generally have three biological functions as follows: 1) storage of chemical energy, 2) essential structural components for biological membranes, and 3) signaling of molecular events (van Meer, 2005; Cockcroft, 2021). In many lipidomics applications, membrane and storage lipids are measured and exploited as the basis for functional associations. For some structural lipid classes, such as lysolipids, ceramides, and diacylglycerols (and others), interpretations are often complicated as these lipids can also act as signaling molecules that might start a cascade of biosynthetic events. However, conventional omics data interpretation strategies, such as those established for expression data and proteomics, can be applied much more easily for signaling lipids because of the similar thresholds for fold-changes of two or more and its clearer functional association. This might be an oversimplification, but lipid signaling cascades, for instance, for small lipid mediators, phosphatidylinositides, and sphingosines (and others), can be equivalent to the activation of protein signaling cascades (Wymann and Schneiter, 2008; Posor et al., 2022). In both cases, a primary event, which is initiated by a small number of often low abundant molecular entities, leads to systemic changes on the cellular or organismal level.
From this perspective, lipid metabolic processes that change the make-up of the cell with respect to the membrane systems, and/or storage compartments follow slower kinetic and fold-changes and can be rather small but affect a large portion of the lipidome. The resulting consequences for experiment planning and data interpretation of lipid profiles will be discussed.
Up-to-date mass spectrometry-based lipidomics procedures are not specific enough to identify lipids at the structure-defined level (Liebisch et al., 2013; Liebisch et al., 2020). Specifically, the number of isomeric structures that are actually unresolved by chosen analytical approach should at least lead to the application of this recently introduced hierarchical nomenclature. In the case of complex lipids that are comprised of two or more aliphatic chains, the number of isomeric molecules can reach hundreds, which is the result of the limit of specificity at the molecular species identification level (Liebisch et al., 2013; Liebisch et al., 2020). For the major and abundant lipid classes of eukaryotes that make up the membrane systems and the storage pools, the exact composition of the aliphatic chains are generally not defined with regards to the sn-position, double-bond position, and cis/trans isomerism (Shevchenko and Simons, 2010; Schwudke et al., 2011). However, the higher specificity for lipid identification in large-scale studies is on the horizon with the application of ion mobility in lipidomics (Moran-Garrido et al., 2022). Because changes in the gas phase collision cross section (CCS) can be minute (≤1%), higher resolution ion mobility is required, and the associated informatics approaches that properly detect these mass spectrometric features in three-dimensional datasets (rt, CCS, and m/z) are just now becoming publicly available (Kirkwood et al., 2022).
The structure of lipid molecules has a direct influence on the physical parameters of a biological membrane (Figure 1). The most obvious impact of structural lipid isomers is found in the packaging density, thickness, compressibility, and phase properties of a membrane (Figure 1A). The pleiotropic nature of the effects on membrane biology are a focal point of ongoing research (Palusinska-Szysz et al., 2022; Saha et al., 2022) [reviewed in Sezgin et al. (2017); Sych et al. (2022)] and are mostly defined by the direct interaction of lipids with proteins and the modulation of lipid domains (London, 2022). Another important effect of lipid profile changes concerning the aliphatic chains and lipid class composition is the maintenance of membrane asymmetry and morphology that is only possible within a certain compositional framework (Lorent et al., 2020). A well-established model is the application of lipid shapes that, based on the steric requirement of each lipid, allow possible membrane curvatures to be predicted [Figure 1A; Israelachvili et al. 1977; Cullis and de Kruijff, 1979; Strandberg et al., 2012; Joardar et al., 2022)]. From this perspective, the need of preferable identification on a structural refined level and absolute quantitation of lipids (in molar amounts) is easy to perceive (Shevchenko and Simons, 2010). Large-scale lipid quantitation and its accuracy remain an open issue in lipidomics research and also reflect the wide range of analytical strategies based on LC-MSn and shotgun approaches (Zullig et al., 2020; Han and Gross, 2022).
FIGURE 1. Concept for the direct impact of lipid profile alterations on membrane properties. (A) Small structural changes of individual lipid species change the intermolecular interaction in a membrane, which is demonstrated for simplification only on one leaflet [but in detail discussed in Strandberg et al. (2012)]. The shift of the double-bond position, cis/trans configuration, and sn-position has a direct impact on the average distance between lipids and the strength of interaction. As result, molecular movements are differently restricted and different phase behavior can be observed, including domain formation (London, 2022). Changes in the lipid composition further affect thickness of membranes, and differently shaped lipids induce membrane curvature. Such morphological changes can be achieved by different lipid compositions either by changing a large portion of major abundant molecular species (*) or changes in the overall mixture (#). (B) Lipid metabolic snapshot in the form of a profile reflects a specific sampling point, which should be defined by biological checkpoints. Such a stable metabolic state (1) might go through several transitional stages until a new homeostatic state (2) is reached. The endogenous kinetics of the underlying lipid metabolic networks is generally not known and yields uncertainty, and the turnover time for individual lipid species has to be considered. Created with BioRender.com.
However, the scientific community should not focus on favorite analytic strategies and the largest ID-number but instead use the scientific question and data quality as a first priority for the selection of a lipidomics approach (Kofeler et al., 2021). With regard to the overall membrane composition, the molar composition is required to associate membrane phenotypes to lipid profiles that go beyond a pure description of changes but rather help reconstitute model membranes and perform functional studies. Furthermore, I would argue that a lack of standardization and proper normalization of lipid molarities to parameters like cell number, weight, protein content, and/or quantifiable phenotype limits the applicability of lipidomics. To overcome this essentially descriptive nature of lipidomics, a study plan and biological hypothesis should be formulated in an interdisciplinary discourse that includes the essential steps of the analytical method development concerning sampling and sample preparation. When considering the heterogenic nature of the biological sample matrix and its consequences for lipid identification and quantitation, the correlation between phenotypes and their putative link to the lipidome can be explored.
An intriguing illustration for analytical and biological challenges that one must face in lipidomics research was formulated by Andrej Shevchenko (Arnaud, 2011) in an analogy of knights and armies of the Middle Ages as follows: “Knights are like proteins. Each knight has a pedigree, a story, an emblem, a flag. Lipids are like armies. Knights are more visible. It’s easier to write poetry about them, but in the end it’s the army that wins, the collective of individuals. That’s the lipids.”
Indeed, a lipid metabolic perturbation that affects the state of a cell, tissue, organ, or organism often induces quantitative changes in many related molecules (Figure 1B). Such biosynthetic activities, which are the basis for cell membrane homeostasis, trafficking, and autophagy (to mention only the most obvious), require time by nature to be executed by the lipid metabolic system. This action of a biosynthetic system has an endogenous inertia to convert lipids. The turnover rates for membrane and storage lipids have only sparsely been determined if at all. These measurements should cover time scales in the range of seconds to days depending on the studied biological system to gain insights into the kinetics of a transition between different lipid metabolic states (Figure 1B). Furthermore, a possible occurrence of a pathology, where the biological system cannot be converted into another stable homeostatic state, can be characterized with much better resolution.
Model organisms such as D. melanogaster, Caenorhabditis elegans, and S. cerevisiae as well as cell culture systems are the ideal study objects to observe associations between the perturbation of lipid homeostasis and biological phenotypes. In a drosophila model of lysosomal storage disease, Hebbar et al. (2017) studied the emergence of lipid profile changes in association with the occurrence of neurodegenerative phenotypes. They showed that as an early phenotype, ceramide and sphingosine levels are altered before signs of failure of cell autophagy in the form of endomembranous structures and lipofuscin accumulation occurred. After 14 days of systemic lipid metabolism perturbation, significant behavioral alteration caused by neurodegeneration could be determined. A similar systemic breakdown of lipid homeostasis was observed for LET-767 RNAi, which is responsible for the elongation of fatty acids in C. elegans that only showed slight changes at first in TAG profiles but had in the second generation a fatal effect that led to developmental arrest and death (Entchev et al., 2008). A detailed lipid analysis revealed that the breakdown of sphingolipid biosynthesis was the phenotypic driver caused by a lack of branched chain fatty acids, which are an essential educt for sphingosine synthesis. Detailed lipidomics analysis during the polarization of MDCK cells showed that general reorganization of the membrane system and maintenance of apical and basolateral surfaces required molecular adjustments throughout the lipidome (Sampaio et al., 2011). Within a time frame of approximately 7 days, a new lipid homeostatic state was reached signified by elongated sphingolipid acid chain lengths, increased hydroxylation, and less double bonds. At the same time, for glycerophospholipids, a higher degree of poly-unsaturated chains in combination with a tendency for longer chain length was observed.
These examples underline the requirements for systematic lipidomic studies to establish well-defined sampling times and consider the kinetic aspects of the biological process to be studied. In addition, for the study objectives where a lipid metabolic switch is investigated and not a single “golden molecule,” this should denote a systemic alteration. Furthermore, one can postulate that there always should be a panel of lipids connected by a metabolic network that responds to the systemic perturbation of lipid homeostasis. From my experience and reported studies, aliphatic chains of structural lipids are one of the building blocks that are usually modified due to environmental stress, genetic defects, and cell biological processes. This has two practical consequences as follows: first, lipid class profiles are defined by the underlying fatty acid composition, and second, the detection of many systemic lipidome changes are dependent on the enzyme kinetics of fatty acid transformation, transport, and exchange. From these findings, it can be argued that major abundant structural lipids should always reflect the overall fatty acid composition. For instance, this is the case in most eukaryotic system palmitic acids (FA 16:0), oleic acid (FA 18:1), and stearic acid (FA 18:0). The resulting profiles of lipid classes— phosphatidylcholine (PC), phosphatidylethanolamine (PE), and phosphatidylserine (PS)—are consequently built of 16:0_16:0, 16:0_18:0, 16:0_18:1, 18:1_18:1, and 18:0_18:1 aliphatic backbones that represent a large molar percentage of the eukaryotic lipidome.
Regarding the aforementioned examples of systemic lipidome alterations, the publication of apparently structurally and/or synthetically unrelated (quite distanced in the metabolic pathway with many enzymatic steps in between) lipid biomarkers only based on a descriptive study should be read with caution. It has been reported that a proper experimental group size, well-described experimental procedures, a set of quality controls, and a critical assessment on the possible errors and statistics should be subsequently considered and outlined (McDonald et al., 2022). Furthermore, tracer and flux analyses should become an integral part of functional lipidomic studies whenever possible (Skotland et al., 2016; Kim et al., 2020; Brandenburg et al., 2022).
When lipid molecular species are preferentially transformed within a metabolic network leading to profile changes, several sources of experimental variability and their impact should be critically discussed. For functional studies, it is evident that there is interest in detecting the primary lipid metabolic effect. However, biomedical studies are driven by a phenotypic characterization based upon imaging, fitness, survival, and other morphological metrics that introduce a bias toward late sampling time points that will already be dominated by secondary metabolic events. Driven by the need to have a phenotype characterized, when it is noticeable without a doubt, one can easily understand that kinetic aspects of systemic metabolic changes are often underrepresented in the literature. I would encourage to increase the number of experimental groups from only one pair consisting of “Control” and its counterpart like “Diseased,” “Mutant,” and/or “KnockDown,” to a reasonable number of phenotypically and kinetically defined experimental groups (Hebbar et al., 2017). In the context of the adaptive power of lipid metabolic systems, i.e. the molecular snapshot that is usually taken in the form of lipid profiles and interpreted, the reversibility of an observed state/phenotype, its stability, and the number of involved regulative processes should be considered (Figure 1B).
A further decisive choice for experimental success in pinpointing primary lipid metabolic alterations is the limit of spatial resolution to cover tissue heterogeneity and cell biological compartmentalization, which to some extent can be studied with state-of-the-art lipid MS-imaging (Fu et al., 2021; Bednarik et al., 2022), coupling of laser microdissection (Hebbar et al., 2014; Knittelfelder et al., 2018), and cell fractionation techniques (Schmitt et al., 2022) (Tharkeshwar et al., 2017) with lipidomics. These complex methodical approaches require further considerations concerning quantitation, possible contamination, and limits of specificity, which are discussed elsewhere in detail (Ellis et al., 2013; Eiersbrock et al., 2020). If such enrichment strategies for the specimen of interest is not possible, a critical assessment of the averaging effects of mixing with other molecular pools in the isolated sample is necessary.
Regarding these concerns, one should examine whether the fold-change for a lipid quantity is realistically measurable after completing the analytical workflow. In my experience, fold-changes of 0.25 for a lipid are detectable in well-planned and executed lipidomic studies. This lower limit of detectable lipid metabolic alteration integrates the experimental error propagation that is occurring during homogenization and volumetric processing, and due to batch effects of internal standards as well as instrument instability. Additionally, I would suggest that functional lipid metabolic studies be performed with at least six biological replicates to ensure statistical validity. In the context of large-scale experiments and an increasing number of variables in biomedical applications, an appropriate power calculation for experimental group sizes should be performed. However, in my experience, high foldchanges of minor lipid species in the context of systemic metabolic switches are often overvalued in comparison to smaller changes of major abundant components of a lipidome, even when the affected molar percentage is magnitudes higher.
In this minireview, I have provided some personal viewpoints on the experimental limits and interpretation guidelines for the application of lipidomics to study lipid homeostasis. This is to raise awareness of some principles in studying systemic lipid metabolic changes concerning experimental planning and general data interpretation. The reader is invited to further the discussion on lipid profiles as a snapshot of membrane compositions in the context of biophysics and spatial and temporal resolution. The interest in lipids in biomedical research is rightfully increasing, and the lipidomic community should pursue the development of appropriate analytical methods and the provision of high quality data with a determination to integrate lipidomics into interdisciplinary research.
DS conceptualized and wrote the manuscript.
DS is grateful for the funding of his research from the German Center for Infection Research (DZIF) of the research topic “Thematic Translational Unit Tuberculosis” (TTU 709, 813) and the German Network for Bioinformatics Infrastructure (de.NBI, project LIFS2 FKZ 031L0108B).
The author declares that the research was conducted in the absence of any commercial or financial relationships that could be construed as a potential conflict of interest.
All claims expressed in this article are solely those of the authors and do not necessarily represent those of their affiliated organizations, or those of the publisher, the editors, and the reviewers. Any product that may be evaluated in this article, or claim that may be made by its manufacturer, is not guaranteed or endorsed by the publisher.
Arnaud, C. H. (2011). Lipids take charge. Washington: Chemical and Engineering News, 89. Available at: https://pubsapp.acs.org/cen/support/contact.html.
Bednarik, A., Prysiazhnyi, V., Bezdekova, D., Soltwisch, J., Dreisewerd, K., and Preisler, J. (2022). Mass spectrometry imaging techniques enabling visualization of lipid isomers in biological tissues. Anal. Chem. 94, 4889–4900. doi:10.1021/acs.analchem.1c05108
Brandenburg, J., Heyckendorf, J., Marwitz, F., Zehethofer, N., Linnemann, L., Gisch, N., et al. (2022). Tuberculostearic acid-containing phosphatidylinositols as markers of bacterial burden in tuberculosis. ACS Infect. Dis. 8, 1303–1315. doi:10.1021/acsinfecdis.2c00075
Cockcroft, S. (2021). Mammalian lipids: Structure, synthesis and function. Essays Biochem. 65, 813–845. doi:10.1042/EBC20200067
Cullis, P. R., and de Kruijff, B. (1979). Lipid polymorphism and the functional roles of lipids in biological membranes. Biochim. Biophys. Acta 559, 399–420. doi:10.1016/0304-4157(79)90012-1
Eiersbrock, F. B., Orthen, J. M., and Soltwisch, J. (2020). Validation of MALDI-MS imaging data of selected membrane lipids in murine brain with and without laser postionization by quantitative nano-HPLC-MS using laser microdissection. Anal. Bioanal. Chem. 412, 6875–6886. doi:10.1007/s00216-020-02818-y
Ellis, S. R., Brown, S. H., Het Panhuis, M., Blanksby, S. J., and Mitchell, T. W. (2013). Surface analysis of lipids by mass spectrometry: More than just imaging. Prog. Lipid Res. 52, 329–353. doi:10.1016/j.plipres.2013.04.005
Entchev, E. V., Schwudke, D., Zagoriy, V., Matyash, V., Bogdanova, A., Habermann, B., et al. (2008). LET-767 is required for the production of branched chain and long chain fatty acids in Caenorhabditis elegans. J. Biol. Chem. 283, 17550–17560. doi:10.1074/jbc.M800965200
Fu, T., Knittelfelder, O., Geffard, O., Clement, Y., Testet, E., Elie, N., et al. (2021). Shotgun lipidomics and mass spectrometry imaging unveil diversity and dynamics in Gammarus fossarum lipid composition. iScience 24, 102115. doi:10.1016/j.isci.2021.102115
Han, X., and Gross, R. W. (2022). The foundations and development of lipidomics. J. Lipid Res. 63, 100164. doi:10.1016/j.jlr.2021.100164
Hebbar, S., Khandelwal, A., Jayashree, R., Hindle, S. J., Chiang, Y. N., Yew, J. Y., et al. (2017). Lipid metabolic perturbation is an early-onset phenotype in adult spinster mutants: A Drosophila model for lysosomal storage disorders. Mol. Biol. Cell 28, 3728–3740. doi:10.1091/mbc.E16-09-0674
Hebbar, S., Schulz, W. D., Sauer, U., and Schwudke, D. (2014). Laser capture microdissection coupled with on-column extraction LC-MS(n) enables lipidomics of fluorescently labeled Drosophila neurons. Anal. Chem. 86, 5345–5352. doi:10.1021/ac500276r
Israelachvili, J. N., Mitchell, D. J., and Ninham, B. W. (1977). Theory of self-assembly of lipid bilayers and vesicles. Biochim. Biophys. Acta 470, 185–201. doi:10.1016/0005-2736(77)90099-2
Joardar, A., Pattnaik, G. P., and Chakraborty, H. (2022). Mechanism of membrane fusion: Interplay of lipid and peptide. J. Membr. Biol. 255, 211–224. doi:10.1007/s00232-022-00233-1
Kim, I. Y., Park, S., Jang, J., and Wolfe, R. R. (2020). Quantifications of lipid kinetics in vivo using stable isotope tracer methodology. J. Lipid Atheroscler. 9, 110–123. doi:10.12997/jla.2020.9.1.110
Kirkwood, K. I., Pratt, B. S., Shulman, N., Tamura, K., Maccoss, M. J., Maclean, B. X., et al. (2022). Utilizing Skyline to analyze lipidomics data containing liquid chromatography, ion mobility spectrometry and mass spectrometry dimensions. Nat. Protoc. 17, 2415–2430. doi:10.1038/s41596-022-00714-6
Knittelfelder, O., Traikov, S., Vvedenskaya, O., Schuhmann, A., Segeletz, S., Shevchenko, A., et al. (2018). Shotgun lipidomics combined with laser capture microdissection: A tool to analyze histological zones in cryosections of tissues. Anal. Chem. 90, 9868–9878. doi:10.1021/acs.analchem.8b02004
Kofeler, H. C., Eichmann, T. O., Ahrends, R., Bowden, J. A., Danne-Rasche, N., Dennis, E. A., et al. (2021). Quality control requirements for the correct annotation of lipidomics data. Nat. Commun. 12, 4771. doi:10.1038/s41467-021-24984-y
Liebisch, G., Fahy, E., Aoki, J., Dennis, E. A., Durand, T., Ejsing, C. S., et al. (2020). Update on LIPID MAPS classification, nomenclature, and shorthand notation for MS-derived lipid structures. J. Lipid Res. 61, 1539–1555. doi:10.1194/jlr.S120001025
Liebisch, G., Vizcaino, J. A., Kofeler, H., Trotzmuller, M., Griffiths, W. J., Schmitz, G., et al. (2013). Shorthand notation for lipid structures derived from mass spectrometry. J. Lipid Res. 54, 1523–1530. doi:10.1194/jlr.M033506
London, E. (2022). Ordered domain (raft) formation in asymmetric vesicles and its induction upon loss of lipid asymmetry in artificial and natural membranes. Membr. (Basel) 12, 870. doi:10.3390/membranes12090870
Lorent, J. H., Levental, K. R., Ganesan, L., Rivera-Longsworth, G., Sezgin, E., Doktorova, M., et al. (2020). Plasma membranes are asymmetric in lipid unsaturation, packing and protein shape. Nat. Chem. Biol. 16, 644–652. doi:10.1038/s41589-020-0529-6
Mcdonald, J. G., Ejsing, C. S., Kopczynski, D., Holcapek, M., Aoki, J., Arita, M., et al. (2022). Introducing the lipidomics minimal reporting checklist. Nat. Metab. 4, 1086–1088. doi:10.1038/s42255-022-00628-3
Moran-Garrido, M., Camunas-Alberca, S. M., Gil-de-La Fuente, A., Mariscal, A., Gradillas, A., Barbas, C., et al. (2022). Recent developments in data acquisition, treatment and analysis with ion mobility-mass spectrometry for lipidomics. Proteomics 22, e2100328. doi:10.1002/pmic.202100328
Palusinska-Szysz, M., Jurak, M., Gisch, N., Waldow, F., Zehethofer, N., Nehls, C., et al. (2022). The human LL-37 peptide exerts antimicrobial activity against Legionella micdadei interacting with membrane phospholipids. Biochim. Biophys. Acta Mol. Cell Biol. Lipids 1867, 159138. doi:10.1016/j.bbalip.2022.159138
Posor, Y., Jang, W., and Haucke, V. (2022). Phosphoinositides as membrane organizers. Nat. Rev. Mol. Cell Biol. 23, 797–816. doi:10.1038/s41580-022-00490-x
Saha, S., Das, A., Patra, C., Anilkumar, A. A., Sil, P., Mayor, S., et al. (2022). Active emulsions in living cell membranes driven by contractile stresses and transbilayer coupling. Proc. Natl. Acad. Sci. U. S. A. 119, e2123056119. doi:10.1073/pnas.2123056119
Sampaio, J. L., Gerl, M. J., Klose, C., Ejsing, C. S., Beug, H., Simons, K., et al. (2011). Membrane lipidome of an epithelial cell line. Proc. Natl. Acad. Sci. U. S. A. 108, 1903–1907. doi:10.1073/pnas.1019267108
Schmitt, D., Bozkurt, S., Henning-Domres, P., Huesmann, H., Eimer, S., Bindila, L., et al. (2022). Lipid and protein content profiling of isolated native autophagic vesicles. EMBO Rep. 23, e53065. doi:10.15252/embr.202153065
Schwudke, D., Schuhmann, K., Herzog, R., Bornstein, S. R., and Shevchenko, A. (2011). Shotgun lipidomics on high resolution mass spectrometers. Cold Spring Harb. Perspect. Biol. 3, a004614. doi:10.1101/cshperspect.a004614
Sezgin, E., Levental, I., Mayor, S., and Eggeling, C. (2017). The mystery of membrane organization: Composition, regulation and roles of lipid rafts. Nat. Rev. Mol. Cell Biol. 18, 361–374. doi:10.1038/nrm.2017.16
Shevchenko, A., and Simons, K. (2010). Lipidomics: Coming to grips with lipid diversity. Nat. Rev. Mol. Cell Biol. 11, 593–598. doi:10.1038/nrm2934
Skotland, T., Ekroos, K., Kavaliauskiene, S., Bergan, J., Kauhanen, D., Lintonen, T., et al. (2016). Determining the turnover of glycosphingolipid species by stable-isotope tracer lipidomics. J. Mol. Biol. 428, 4856–4866. doi:10.1016/j.jmb.2016.06.013
Strandberg, E., Tiltak, D., Ehni, S., Wadhwani, P., and Ulrich, A. S. (2012). Lipid shape is a key factor for membrane interactions of amphipathic helical peptides. Biochim. Biophys. Acta 1818, 1764–1776. doi:10.1016/j.bbamem.2012.02.027
Sych, T., Levental, K. R., and Sezgin, E. (2022). Lipid-protein interactions in plasma membrane organization and function. Annu. Rev. Biophys. 51, 135–156. doi:10.1146/annurev-biophys-090721-072718
Tharkeshwar, A. K., Trekker, J., Vermeire, W., Pauwels, J., Sannerud, R., Priestman, D. A., et al. (2017). A novel approach to analyze lysosomal dysfunctions through subcellular proteomics and lipidomics: The case of NPC1 deficiency. Sci. Rep. 7, 41408. doi:10.1038/srep41408
Wymann, M. P., and Schneiter, R. (2008). Lipid signalling in disease. Nat. Rev. Mol. Cell Biol. 9, 162–176. doi:10.1038/nrm2335
Keywords: lipidomics, biophysics, lipid homeostasis, membrane, analytical precision
Citation: Schwudke D (2023) What information is contained in experimentally determined lipid profiles?. Front. Anal. Sci. 3:1157582. doi: 10.3389/frans.2023.1157582
Received: 02 February 2023; Accepted: 06 March 2023;
Published: 23 March 2023.
Edited by:
Jared B. Shaw, e-MSion, Inc., United StatesReviewed by:
Carmen Bedia, Institute of Environmental Assessment and Water Research (CSIC), SpainCopyright © 2023 Schwudke. This is an open-access article distributed under the terms of the Creative Commons Attribution License (CC BY). The use, distribution or reproduction in other forums is permitted, provided the original author(s) and the copyright owner(s) are credited and that the original publication in this journal is cited, in accordance with accepted academic practice. No use, distribution or reproduction is permitted which does not comply with these terms.
*Correspondence: Dominik Schwudke, ZHNjaHd1ZGtlQGZ6LWJvcnN0ZWwuZGU=
Disclaimer: All claims expressed in this article are solely those of the authors and do not necessarily represent those of their affiliated organizations, or those of the publisher, the editors and the reviewers. Any product that may be evaluated in this article or claim that may be made by its manufacturer is not guaranteed or endorsed by the publisher.
Research integrity at Frontiers
Learn more about the work of our research integrity team to safeguard the quality of each article we publish.