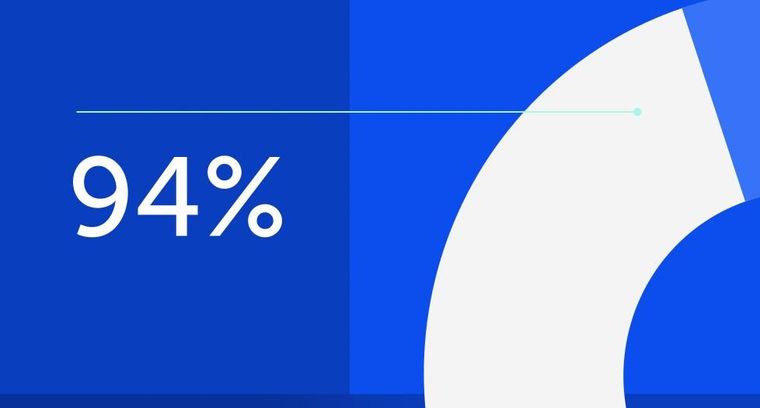
94% of researchers rate our articles as excellent or good
Learn more about the work of our research integrity team to safeguard the quality of each article we publish.
Find out more
MINI REVIEW article
Front. Anal. Sci., 31 March 2023
Sec. Omics
Volume 3 - 2023 | https://doi.org/10.3389/frans.2023.1119438
This article is part of the Research TopicPerspectives in Omics 2022View all 7 articles
Identifying specific diagnostic and prognostic biological markers of COVID-19 can improve disease surveillance and therapeutic opportunities. Mass spectrometry combined with machine and deep learning techniques has been used to identify pathways that could be targeted therapeutically. Moreover, circulating biomarkers have been identified to detect individuals infected with SARS-CoV-2 and at high risk of hospitalization. In this review, we have surveyed studies that have combined mass spectrometry-based omics techniques (proteomics, lipdomics, and metabolomics) and machine learning/deep learning to understand COVID-19 pathogenesis. After a literature search, we show 42 studies that applied reproducible, accurate, and sensitive mass spectrometry-based analytical techniques and machine/deep learning methods for COVID-19 biomarker discovery and validation. We also demonstrate that multiomics data results in classification models with higher performance. Furthermore, we focus on the combination of MALDI-TOF Mass Spectrometry and machine learning as a diagnostic and prognostic tool already present in the clinics. Finally, we reiterate that despite advances in this field, more optimization in the analytical and computational parts, such as sample preparation, data acquisition, and data analysis, will improve biomarkers that can be used to obtain more accurate diagnostic and prognostic tools.
The first detection of SARS-CoV-2, the causing agent of Coronavirus Disease 19 (COVID-19), infection in humans was dated late 2019 in Wuhan, China (Lamers and Haagmans, 2022). Since its emergence, efforts have been made to understand the disease and find new biomarkers to develop diagnostic and prognostic methods. The development of diagnostic methods is essential to detect the disease as soon as possible for disease control, while prognostic methods are essential to predict if a patient will develop clinical manifestations ranging from asymptomatic, mild, to severe COVID-19 symptoms. Mild cases of COVID-19 may present with symptoms similar to the common cold or flu, such as fever, cough, sore throat, fatigue, body aches, and loss of smell or taste. Severe cases of COVID-19 can include shortness of breath, chest pain or pressure, confusion, and bluish lips or face. Severe cases can rapidly progress and require hospitalization. Some patients require mechanical ventilation or other intensive care that can evolve into acute respiratory distress syndrome, septic shock and/or multiple organ failure (Berlin et al., 2020).
Several factors can indicate whether or not an individual will develop severe COVID-19. There are reports in the literature indicating that higher age is associated with an increased chance of admission to ICU (intensive care unit) or death (Romero Starke et al., 2020; CDC COVID-19 Response Team, 2020; Romero Starke et al., 2021). Male individuals were also reported to have an increased risk for severe COVID-19 (Klein et al., 2020; Penna et al., 2020; Alwani et al., 2021). Regarding comorbidities, patients with cardiovascular diseases, diabetes, obesity, chronic kidney disease, hypertension, HIV, and tuberculosis were reported to have an increased risk of developing severe COVID-19 (Pranata et al., 2020; Collard et al., 2021; Gimeno-Miguel et al., 2021; McGurnaghan et al., 2021; Ortiz et al., 2021; Zhou et al., 2021). Although the individual’s characteristics provide insights into COVID-19 severity, the molecular patterns allow the understating of the biological mechanisms responsible for such responses. Analyzing the changes in proteins, metabolites, lipids, and other molecules can help find biomarkers that can be further used to develop diagnostic and prognostic methods. For instance, the increased expression of the protein ACE2 (angiotensin-converting enzyme 2), which serves as the receptor for the SARS-CoV-2 S protein, was reported to be correlated with increased risk of severe COVID-19 (Pinto et al., 2020; Gheware et al., 2022), it was also reported that ACE2 expression in nasal and bronchial airways is lower in children than in adults, which may be a factor that contributes to children having a lower risk for severe COVID-19 (Aslam et al., 2017; McArdle et al., 2021).
The effects of SARS-CoV-2 infection are complex and multiple mechanisms may explain severity differences between groups. Differences in immune responses can also be used to describe why certain groups are more prone to severe COVID-19; for instance, it has been reported that females have an increased dosage of TLR7, a toll-like receptor capable of recognizing coronavirus RNA, which controls the viral replication through type I interferon activation (Alwani et al., 2021). This increased dosage may be responsible for enhancing viral response, conferring to females increased protection against SARS-CoV-2 when compared to male individuals (Spiering and de Vries, 2021). Diabetes is another example of the complexity behind COVID-19 severity mechanisms. Patients with severe COVID-19 have an increased presence of inflammatory markers such as procalcitonin (PCT), C-reactive protein (CRP), interleukin-6, 10, and 2R (IL-6, IL-10, IL-2R), and serum amyloid A (SAA) (Zhou et al., 2020; Mahat et al., 2021). Diabetic patients are known to have a proinflammatory state, which could contribute to an increased chance of developing severe COVID-19 (ref 21), especially by exacerbating the cytokine responses leading to a severe immune reaction, an event called “cytokine storm” (Erener, 2020). The higher risk for patients with diabetes was also correlated with the activation of the NF-kappa-B pathway, which is known to have an essential role in the cytokine storm event (Aslam et al., 2017). These studies demonstrate the heterogeneous effectors that causes COVID-19 severity.
Given this complexity, a systemic view of the infection can provide valuable information on disease progression by searching for more specific molecules or a set of molecules that can detect and monitor the disease.
Since COVID-19 affects many biological pathways, several biomolecules are regulated during infection. Thus, selecting the molecules with the highest impact on disease progression is challenging. High-throughput techniques can be employed to understand COVID-19 at the molecular level and capture the complex changes in the host. In this context, mass spectrometry is a technique that can be used to map systemic changes and has been applied in COVID-19 biomarker identification. However, finding the proteins, metabolites, lipids, or other biomolecules that directly impact COVID-19 pathogenesis can be challenging due to the large amount of data generated. In this case, machine and deep learning can be employed to build classification models to diagnose if a patient has COVID-19 or will develop a severe condition.
This review shows how mass spectrometry can be applied to obtain a systemic view of the viral infection and use this information to find molecules that can be used as biomarkers for diagnosis and prognosis of the disease. We also show how machine learning and deep learning (a subfield of machine learning) can assist omics data analysis, building models for diagnosis and prognosis, focusing on the supervised learning approach. Finally, we demonstrate how the Matrix-assisted laser desorption ionization–time of flight mass spectrometry (MALDI-TOF MS) combined with machine learning can serve as a method for diagnosis/prognosis of COVID-19 and discuss the challenges and prospectives of this field.
In this review, we searched for PubMed articles with the keys “Proteomics and Machine Learning”, “Lipdomics and Machine Learning,” and “Lipdomics and Machine Learning,” all of them followed by “COVID-19 diagnosis” or “COVID-19 prognosis.” The articles that contained multiple omics in their methods were marked as “Multiomics.” Articles that contained omics obtained from other techniques than MS were considered in the review only if they were a multiomics approach containing at least one omics dataset obtained by MS. Table 1 includes 42 studies that passed these criteria.
TABLE 1. Omics approaches combined with machine and deep learning data analyses to identify diagnostic and prognostic biomarkers for COVID-19.
A biomarker can be a biomolecule that indicates a change in biological structures or functions and can be used to evaluate the state of a living organism (Silberring and Ciborowski, 2010). Since most clinical decisions are based on laboratory tests, biomarkers can influence clinical outcomes. Many studies aim to find specific biomarkers for certain diseases, and mass spectrometry is a common approach to search for them. In short, mass spectrometry instruments measure the mass-to-charge ratio ions in the gas phase of proteins, peptides, metabolites, lipids and other molecules from a given sample (i.e., cells, biofluids and tissues) to determine their molecular weight, structures, and quantities (Aksenov et al., 2017). Since this technique allows the identification and quantification of thousands of molecules, it is one of the most used technologies in “omics” studies. Proteomics is the science that aims to identify and quantify the complete set of proteins, their localization, interactions and post-translational modifications present in cells, tissues, biofluids, or other biological materials from an organism (Aslam et al., 2017). Several studies have applied proteomics to identify and quantify circulating and tissue biomarkers that could help in the clinical decision-making process (McArdle et al., 2021) and understand the mechanism of SARS-CoV-2 infection (Bojkova et al., 2020; Kim et al., 2023). Metabolomics is defined as the qualitative and quantitative study of the complete set of small molecules (metabolites) and their interactions within a biological sample (Hasan et al., 2021). Alterations in metabolic pathways was also demonstrated to be linked with many human diseases, including viral infection (Birungi et al., 2010; Chandler et al., 2016; Ussher et al., 2016; Uchiyama et al., 2017). Moreover, metabolomics has been used to identify and prioritize diagnostic and prognostic COVID-19 biomarkers (Shen et al., 2020; McCreath et al., 2021). Lipidomics is the study of the lipidome, which can be defined as the set of lipid species expressed in a biological system, such as biofluids, tissues or cells (Han and Gross, 2003; Yang and Hhan, 2016). Lipids are known to play a central role in viral infections, as they are required to compose the structures for both the virus and the cell (Abu-Farha et al., 2020). The proteome, metabolome, and lipidome are dynamic and modified based on biotic and abiotic insults such as viral infections. This review will focus on mass spectrometry-based omics analyses; however, genomics and transcriptomics are also considered when used in a multiomic approach.
Mass spectrometry-based omics approaches were used to characterize the SARS-CoV-2 infection using a wide range of biological samples, such as plasma, serum, urine, organ autopsies, airway mucus, bronchoalveolar lavage fluid, and saliva (Zeng et al., 2021; Liu Y. et al., 2022; Zhang Z. et al., 2022; Mansouri et al., 2022; Muñoz-Prieto et al., 2022). The molecular landscape of these studies contributed to elucidating the SARS-CoV-2 infection mechanisms and suggested many protein candidates as biomarkers (Shen et al., 2020; Danlos et al., 2021; Ren et al., 2021; Shi et al., 2021; Spick et al., 2021; Liu J. F. et al., 2022; Schuurman et al., 2022). Proteomics of serum samples obtained by LC-MS/MS determined a total of 93 proteins and 204 metabolites differentially expressed when compared between severe and non-severe COVID-19 patients (Shen et al., 2020); The urinary proteome mapped by LC-MS/MS identified 56 proteins differentially expressed when comparing mild and severe COVID-19 patients (Li et al., 2020). Many studies have focused on selecting individual biomarkers among the identified regulated proteins, mainly associated to as platelet degranulation, acute inflammatory response, and complement activation-related proteins (D’Alessandro et al., 2020; Li et al., 2020; Park et al., 2020; Shen et al., 2020; Liu et al., 2021; Mohammed et al., 2022). Due to the complexity of the disease, a single biomarker may be insufficient to indicate with accuracy the changes in the host system and if the disease is progressing to a severe case. Machine learning can be applied to search for specific biomarkers among hundreds of candidates and use sets of biomarkers to build robust classification models to classify patients or predict if a patient will progress to severe COVID-19. The combination of mass spectrometry-based omics and machine learning will be detailed below in the context of COVID-19, Table 1.
Machine learning is a technique that allows a computer to recognize patterns and thus “learn” from a given data. It is a heavily statistic-based technique that uses algorithms to find exploitable regularities in data (Laponogov et al., 2021). The most common applications are supervised and unsupervised learning (Rajoub, 2020); the former will be the focus of this work. In a given dataset, supervised learning assumes the existence of an unknown function
A classification task can help discriminate groups, but training accurate models to perform such a task can be challenging. Sample collection, sample preparation, data acquisition, and preprocessing should be carefully planned to generate models that can generalize outside the training dataset. For instance, if a model is being trained for COVID-19 diagnosis, it is necessary to include patients with other infectious diseases in the control group to ensure that the model will be specific and will not misclassify other virus-infected patients as COVID-19 positive. Sample preparation and data acquisition are two variables that can be explored to maximize the classification performance of a model. Different protein fractions and preparation methods lead to different protein identifications, which can be more specific or less specific for the classification task. Data preprocessing is essential to ensure that the data will be comparable and avoid overfitting during model training (when the model “memorizes” the training dataset and performs poorly in the test dataset).
The most common approach described in the literature for COVID-19 prognosis is the use of omics data to train models for predicting if a patient has the disease or if they will develop severe COVID-19. One of the earliest studies involving this approach used serum samples of 18 non-severe and 13 severe COVID-19 patients to obtain a proteomic and metabolomic profile using LC-MS/MS and UPLC-MS/MS, respectively. Then, the identified proteins (894) and metabolites (941) were used to train a random forest algorithm for sample classification with a 10-fold cross-validation, a resampling technique used to train and test a model several times using the same dataset, which is helpful for small datasets. This resulted in a model with a high Receiver Operating characteristic curve area under the curve of 0.957 (high values indicates that the model is good at distinguishing between the classes); the authors proceeded to perform a validation step with two independent cohorts, one containing 10 patients, which 7 of them were correctly classified and the other containing 19 patients which 16 of them were correctly classified (Shen et al., 2020).
Usually, machine learning techniques work best with larger datasets since it is hard to accurately evaluate the model with a low error (Combrisson and Jerbi, 2015). It is best to use data obtained by the same method for model validation. The dataset size limitation is challenging to address, mainly if acquiring data is time-consuming and expensive but also when the samples are difficult to obtain. A low number of samples in an omics study usually leads to a substantially higher number of features than samples, which is called the curse of dimensionality, causing most machine learning methods vulnerable to overfitting (Mirza et al., 2019). Increasing the number of samples and reducing data dimensionality can reduce model overfitting, usually resulting in reduced training accuracy and improved performance in test sets. For instance, plasma proteomics of 18 non-severe and 33 severe COVID-19 patients using label-free LC-MS/MS identified 1200 proteins, of which 38 were regulated. The authors used the regulated proteins to train five machine learning algorithms for classification, achieving an accuracy of 88% using a support vector machine algorithm; however, they reduced the number of features (proteins) by performing a PLS-DA (Partial Least-Squares Discriminant) analysis and retrieving the 20 most important proteins. They trained the same models again with 20 features and achieved an accuracy of 84% (Suvarna et al., 2021). It is difficult to confirm if the overfitting was reduced with the dimensionality reduction without a test dataset, but the results obtained after the PLS-DA analysis indicate that the model performance before dimensionality reduction was slightly overestimated.
Both studies used as examples above use a mass spectrometry technique that can identify the proteins present in the samples, which is useful for biomarker discovery using machine learning. As we stated earlier, identifying the most important features for classification requires the use of less complex and interpretable models such as Bayesian, decision trees and linear models; for instance, using linear regression models allows us to access the weights that describe the coefficients of a curve, thus indicating which feature has more influence (Mann et al., 2021). This is useful in omics since it provides a way to search biomarkers among thousands of molecules without manually analyzing them. This is useful in omics since it provides a way to search biomarkers among thousands of molecules without manually analyzing them. This approach yielded a list of proteins, metabolites, and lipids that can be used as biomarkers for COVID-19 diagnosis and prognosis, including Serum Amyloid (SAA1 and SAA2), C-reactive Protein (CRP), SERPINA3, SERPING, FGG, Kynurenine, Ursodeoxycholic acid, Carnitine 3:0, Diglyceride 36:0, IL6, Gal-9, ITGB1BP2 and many other biomolecules (Shen et al., 2020; Suvarna et al., 2021; Yaşar et al., 2021; Ahern and COvid-19 Multi-omics Blood ATlas COMBAT Consortium, 2022; Castañé et al., 2022).
The use of proteomics and machine learning for the prognosis of COVID-19 has been demonstrated to be accurate compared to the use of clinical parameters. A study by Demichev et al. (2021) showed that a model trained with proteomic data achieved a similar performance of a model trained with clinical diagnostic parameters (ROC-AUC = 0.98 and ROC-AUC = 0.97 respectively); also, they demonstrated that the machine learning models significantly outperformed other predictive scores of COVID-19 risk factors such as age, BMI, CCI, and molecular predictors such as CRP and IL-6 Demichev et al. (2021). Another study also demonstrated that proteomic data could achieve similar performance of clinical parameters for prognosis classification. Sardar et al. (2021) achieved an accuracy of 89.47% in a model trained with clinical parameters and 89.01% in a model trained with proteomic data. This supports the relevance of proteomic data and machine learning as an important tool for COVID-19 Sardar et al. (2021).
Since mass-spectrometry can provide information on different types of “omics,” each one of them depicting the disease in different ways and explaining the system either by regulation of proteins, lipids or metabolites, the combination of different types of “omics” data can elucidate the causative changes that are responsible for the disease (Hasin et al., 2017). In this context multiple omics integration can provide more in-depth knowledge of what changes occur during COVID-19 infection, including omics data that are not obtained by mass spectrometry, such as transcriptomics and genomics. For instance, it is already reported that the addition of proteomic data to genomic and transcriptomic data help elucidate a disease, demonstrating that the information at protein level complemented the genomics information, leading to the identification of multiple pathways and processes taking place during disease pathogenesis (Subramanian et al., 2020). However, data integration between multiple omics approaches can be challenging, especially due to the heterogeneity of the different omics data and the amount of data generated by them (Subramanian et al., 2020). Machine learning can be applied to analyze multiomics data to address some of these limitations. Although machine learning is still new in multiomics data, it was already employed in several studies to understand several diseases (Reel et al., 2021).
For COVID-19 research, the integration of several omics data helped the elucidation of several pathways. A study performed by Overmyer et al. (2021) obtained the proteome, metabolome, lipidome and leukocyte mRNA expressions from 102 COVID-19 patients and 26 non-COVID-19 patients, where they found 219 biomolecules that were associated with COVID-19 severity. They demonstrated that the multiomics approach yielded a much more detailed picture of the system behavior during COVID-19 infection, showing the regulation of biological processes related to lipid transport, acute phase response, neutrophil degranulation and blood coagulation. Interestingly, the authors performed a correlation analysis between all omics data (which they called “cross-ome analysis”) to uncover the connections between different biomolecule classes. The multiomics data were also used to train machine learning models for COVID-19 prognosis. They demonstrate that the data combination yielded higher average precision and AUC-ROC (area under the receiver operating characteristic curve) than each dataset.
Another study by Byeon et al. (2022) also reported an increase in the performance of predictive models when merging multiple omics datasets. They quantified 1463 cytokines and circulatory proteins, 902 lipids, and 1018 metabolites from 455 COVID-19 patients belonging to three groups depending on disease severity. They found that the models trained with all omics data outperformed each model in the held-out test dataset. The same was observed in another study where machine learning models were trained to predict patient survival, Richard et al. (2022) obtained the proteomic and metabolomic profile of 40 COVID-19 patients, they further trained models for patient survival prediction; the model trained using the proteomics and metabolomics data outperformed both datasets individually.
Although multiomics approach can provide more in-depth knowledge of the disease and generate models with higher performance, the data analysis still imposes some challenges. As pointed out in a review made by Reel et al. (2021), problems such as data heterogeneity, especially when merging dataset that uses different types of normalization and scaling (i.e., proteomics and transcriptomics), class imbalance, and high data dimensionality (when the number of features is much higher than the number of observations) should be taken into consideration to develop appropriate pipelines for model training. The use of deep learning techniques for multiomics has increased in popularity in the past years, especially in analyzing large-scale multiomics datasets (Reel et al., 2021). Deep learning has also demonstrated to have superior performances when dealing with non-linear problems, having great advances in cancer survival research (Chai et al., 2021). Deep learning is a subfield of machine learning with a structure composed of multiple layers of non-linear processing units for feature extraction and processing (Shinde and Shah, 2018). For multiomics applications, deep learning is more appropriate, since it can analyze and extract patterns from large amounts of data obtained from different sources (Shinde and Shah, 2018). In omics, Deep Neural Networks, Recurrent Neural Networks and Convolutional Neural Networks have been applied to predicting DNA and RNA sequence structure, drug design, protein structure/function and protein interactions (Zhang et al., 2019). Moreover, deep learning was also used to develop predictive models for the diagnosis and prognosis of many types of cancers (Chai et al., 2021) (Chaudhary et al., 2018; Xie et al., 2019; Tong et al., 2020; Rong et al., 2021).
For COVID-19, multiomics integration with deep learning is still in its early stages. However, the technique’s potential has already been demonstrated. A study developed by Rahnavard et al. (2022) obtained the proteomic and metabolomic profile of 28 patients with severe COVID-19 and compared it to a control group composed of 28 healthy patients, 25 non-COVID-19 patients with similar clinical symptoms and 25 non-severe COVID-19 patients. They evaluated the performance of three machine learning models - KNN (k-nearest neighbors), RF (random forest) and LR (linear regression) and a deep neural network (DNN) for COVID-19 prognosis. Their results demonstrated that DNN outperformed the other models’ overall accuracy and was better at classifying the severe COVID-19 patients as well.
In summary, some works have used machine learning models for multiomics data (Sun et al., 2021; Richard et al., 2022; Liu et al., 2023), but the number of features used in these studies were relatively low (less than 300), which could justify the use of machine learning. Another point to consider is the necessity for sample processing, choosing deep learning can alleviate this need, since steps such as feature reduction could be omitted, while choosing machine learning models would require extensive data treatment to reduce the features. The integration of multiomics data for COVID-19 holds great promise.
One of the main reasons to search for biomarkers is to use these molecules as a surrogate of the disease and its progression to develop diagnostic and prognostic methods.
A fast, low cost and accurate technique for proteomic data acquisition is essential to maximizing the potential of machine learning for diagnosis and prognosis. Matrix-assisted laser desorption/ionization-time of flight mass spectrometry (MALDI-TOF MS) is a technique that can analyze biomolecules without prior chromatographic separation; it is easy to use, requires a low sample amount, and sample preparation is simplified (Hajduk et al., 2016). However, MALDI-TOF MS does not provide the details and scale of protein identifications as other mass spectrometry techniques. Instead of protein/peptide identifications, the MALDI-TOF MS spectra mainly provide a series of mass-to-charge ratio peaks corresponding to the biomolecules analyzed and their intensities. The set of identified peaks composes a profile with distinctive features depending on the analyzed sample; for instance, a serum sample from a severe COVID-19 patient may have a different MALDI-TOF MS profile than a mild COVID-19 patient. While it may not be possible to visually recognize patterns in these spectra for diagnosis/prognosis purposes, a machine learning approach may be sufficient to perform this task with high precision. Thus, acquiring proteomic spectra using MALDI-TOF MS combined with training machine learning models is a tool to detect the patterns of each group of interest. However, this approach comes at some costs, such as a lower identification rate (a low number of peaks can be assigned to a specific biomolecule), and low precision in quantifying and sampling a subset of molecules due to ionization suppression (Hajduk et al., 2016).
The first work that performed this analysis for COVID-19 used 362 nasal mucous secretion samples (nasal swabs) for diagnostic purposes using the proteomic profile acquired by MALDI-TOF MS (Nachtigall et al., 2020). A total of 211 COVID-19-positive and 151 negative samples were processed, and the spectra obtained were used to train six different machine-learning algorithms. In this case, instead of proteins, the data consisted in m/z peaks that represent unidentified proteins. The authors found 88 protein peaks that were used to train the models. Their findings indicate that a Support Vector Machine Radial was the best algorithm for COVID-19 diagnosis, with an accuracy, specificity, and sensitivity of 93.9%, 94.7%%, and 92.6%, respectively (Nachtigall et al., 2020). The number of samples is higher than in most works that use other mass spectrometry techniques. This is facilitated by MALDI-TOF MS experiments requiring less resources than a LC-MS/MS, for example. The absence of protein identification did not impede training the classification models. This aspect highlights the advantages of using MALDI-TOF as a data acquisition method. However, the authors did not validate the findings using a test set. Another similar study performed nasal swabs MALDI-TOF MS proteomics of 226 samples split into 82 for training/validation and 117 for the test (assessing the model’s true performance) (Tran et al., 2021). The authors used an automated platform denominated Machine Intelligence Learning Optimizer (MILO) to train and test the models (Jen et al., 2021). This platform generated 379,269 models, achieving an accuracy of 96.6%. A study with serum samples also achieved high performance on the training set for COVID-19 diagnosis, reaching a value of 99% accuracy, 98% sensitivity, and 100% specificity in a dataset of 298 samples (146 COVID-19 positives and 152 controls) (Yan et al., 2021).
Although MALDI-TOF MS allowed the analysis of large samples, some aspects still need to be optimized, such as data preprocessing.
Optimizing a pipeline involving mass spectrometry and machine learning for biomarker discovery in the context of diagnosis and prognosis is essential for developing high-performance models that can be generalized outside the training data. Although the studies mentioned in this review are promising, the combinations and impact of different analytical sample preparation, data acquisition, and machine learning methods are still poorly explored. Several protein fractions could be analyzed for COVID-19, such as glycoproteins, phosphoproteins, protein in vesicles, and protein aggregates, which were already described to influence COVID-19 and other diseases such as cancer (Harsha and Pandey, 2010; Nagaraj et al., 2010; Drake and Kislinger, 2014; Pan et al., 2016; Basile et al., 2022; Pongracz et al., 2022). This variety of biomolecules can be accessed through different sample preparation methods such as solid phase extraction (SPE), magnetic beads, silica beads, ultrafiltration, dialysis, hydrophilic interaction chromatography (HILIC) and metal oxide affinity chromatography (MOAC) for example, (Hajduk et al., 2016). Also, samples such as plasma and serum could benefit from depletion methods to remove the highly abundant proteins, since the top 20 most abundant proteins compose roughly 97% of the total protein mass in plasma samples, for example, (Anderson and Anderson, 2002). The depletion of these proteins results in increased sensitivity of the mass spectrometer to detect less abundant proteins (Qian et al., 2008).
Although sample preparation is important, the patient cohort should be carefully chosen before starting the experiments. Patients with a certain disease must be paired with individuals that do not have that disease but present similar symptoms to avoid bias. For instance, severe COVID-19 patients should be paired with patients suffering from a severe condition caused by other respiratory viruses to ensure that the analysis will yield specific markers for severe COVID-19. Selecting the appropriate number of samples is also important since it improves the accuracy of machine learning algorithms. Sometimes it is not possible to have a large patients cohort thus, it is important to evaluate the epidemiological and analytical factors involving the studied disease and the mass spectrometry platform used to acquire the data, in order ensure that the protein expression variability will be enough to capture differences or the number of samples should be larger (Nakayasu et al., 2021). A power analysis could also be performed to find a minimal sample size (Cohen, 1992).
Sample storage is also important to ensure the integrity of the experiments. It is recommended to aliquote serum samples for single use since multiple cycles of freezing and thawing can result in protein degradation, resulting in low-quality samples that will fail or limit the identification of potential biomarkers (Valo et al., 2022). For urine, it is recommended to store the samples immediately after removal of cells or debris at −20°C and subsequently at −80°C (Thongboonkerd, 2007). Implementing appropriate pre-analytical factors such as biofluid collection methods and storage conditions, can be based on guidelines reported in the literature.
MALDI-TOF MS target plate preparation is another step that requires optimization. A process known as the analyte suppression effect occurs in complex biological samples, which is the competition between co-existing components of the sample for desorption and ionization (Lou et al., 2015). This means that the ionization efficiency will vary with the presence of ions that can suppress the peak intensity of other ions; also, low reproducibility of peak intensity can be caused by matrix amount and crystallization process variability (Hajduk et al., 2016). Therefore, it is advisable to perform a standardization step prior to sample processing. In this standardization step, the target molecules that will be measured in the experiment should be evaluated since the MALDI matrices will impact this step. For instance, the alpha-cyano-4-hydroxycinnamic acid matrix is suited for peptides, while sinapinic acid is suitable for proteins larger than 3 kDa (O’Rourke et al., 2016). However, if the targets are low molecular weight compounds, some organic matrices may present signals in the low mass-to-charge range, which will interfere with the analyte signals; a review performed by Calvano et al. (2018) discusses the matrices for low molecular weight compounds and provides a comprehensive overview on MALDI matrices and their applications.
Data processing can also be addressed to achieve more stable results. Usually, the procedures for MALDI data processing involve quality control, normalization, transformation, smoothing, baseline correction, peak detection, and binning (alignment) (Hajduk et al., 2015; Hajduk et al., 2016). There are many method variations to perform each one of these tasks, and each type of data will respond better to a certain method. Thus, evaluating them to find the optimized data processing pipeline is necessary. Also, sample processing on different days or with the same equipment in other laboratories can cause peak mass shift (Rossel et al., 2021); subsequently, data shift will impair the classification model’s performance. Internal standards could be implemented to solve this issue.
While multiomics holds great promise in improving the understanding of a disease or in the generation of more robust machine learning models, data integration is considered a major bottleneck to multiomics studies (Pinu et al., 2019). If choosing for an interpretable model using a machine learning approach, the high dimensionality of the dataset will impose a great challenge for the analysis. Therefore, reducing features is a necessary step. This can be done by performing a feature selection step, which will select a smaller set of features that holds enough information to characterize the groups, or perform a feature extraction method to combine the features and transform them in another set of features (such as a Principal Component Analysis) that also holds enough information of the entire dataset (Picard et al., 2021). If interpretability is not an issue (meaning that it will not be possible to understand which features are used for learning and used to find biomarkers), a Deep Learning approach could be used in concatenated multi-omics data (Picard et al., 2021). The handling of missing data, which frequently arises during multi-omics data integration, should be considered since many statistical approaches do not process missing values. Data imputation often introduces less bias than the complete removal of the features with missing values (Liebal et al., 2020).
The virus and host interaction during the infection triggers many biological pathways. A systemic view of the host system regulation is necessary to capture disease progression. Mass spectrometry can be used for this purpose by identifying systemic alterations of proteins, peptides, lipids, metabolites, and other biomolecules. Finding biomarkers or using this information for disease prognosis can be complex, but machine learning can be helpful in this task. Mass spectrometry and machine learning combined yielded interesting results, generating models for COVID-19 prognosis and diagnosis. However, the lack of analytical and computational validation resulted in several studies finding the same biomarkers for COVID-19, which were not translated into a clinical routine. Method optimization is needed to increase model performance and to find new biomarkers. This can generate more robust models for COVID-19 diagnosis and prognosis. The development of prognosis and diagnosis methods may not need the identification of biomolecules; the MALDI-TOF MS profile can be used in this context without the need for biomarker identification, generating models capable of classifying samples using only MS features. Finally, deep learning and multi-omics approaches are a combination that is still poorly explored and yield better results than the combination of single-omics data and machine learning. Deep learning could also be used to reduce the data processing steps required for simple machine learning applications. This review describes the application of mass spectrometry and machine learning as potential tool for COVID-19 diagnosis and prognosis. The methods described here apply to other diseases.
LL, GS, and GP designed the format and content of the review. All authors have written, revised and approved the final text.
This work was supported by Fundação de Amparo à Pesquisa do Estado de São Paulo (FAPESP), GP (2018/18257-1, 2018/15549-1, 2020/04923-0), GS (2018/13283-4), JM-D-S (2021/00140-3). GP is supported by Conselho Nacional de Desenvolvimento Científico e Tecnológico (CNPq). L R-F and LL are supported by Coordenação de Aperfeiçoamento de Pessoal de Nível Superior (CAPES).
The authors declare that the research was conducted in the absence of any commercial or financial relationships that could be construed as a potential conflict of interest.
All claims expressed in this article are solely those of the authors and do not necessarily represent those of their affiliated organizations, or those of the publisher, the editors and the reviewers. Any product that may be evaluated in this article, or claim that may be made by its manufacturer, is not guaranteed or endorsed by the publisher.
Abu-Farha, M., Thanaraj, T. A., Qaddoumi, M. G., Hashem, A., Abubaker, J., and Al-Mulla, F. (2020). The role of lipid metabolism in COVID-19 virus infection and as a drug target. Int. J. Mol. Sci. 21, 3544. doi:10.3390/ijms21103544
Ahern, D. J., and COvid-19 Multi-omics Blood ATlas COMBAT Consortium (2022). A blood atlas of COVID-19 defines hallmarks of disease severity and specificity. Cell 185, 916–938.e58. doi:10.1016/j.cell.2022.01.012
Aksenov, A. A., Da Silva, R., Knight, R., Lopes, N. P., and Dorrestein, P. C. (2017). Global chemical analysis of biology by mass spectrometry. Nat. Rev. Chem. 1, 0054–120. doi:10.1038/s41570-017-0054
Alwani, M., Yassin, A., Al-Zoubi, R. M., Aboumarzouk, O. M., Nettleship, J., Kelly, D., et al. (2021). Sex-based differences in severity and mortality in COVID-19. Rev. Med. Virol. 31, e2223. doi:10.1002/rmv.2223
Anderson, N. L., and Anderson, N. G. (2002). The human plasma proteome: History, character, and diagnostic prospects. Mol. Cell. Proteomics 1, 845–867. doi:10.1074/mcp.r200007-mcp200
Aslam, B., Basit, M., Nisar, M. A., Khurshid, M., and Rasool, M. H. (2017). Proteomics: Technologies and their applications. J. Chromatogr. Sci. 55, 182–196. doi:10.1093/chromsci/bmw167
Basile, M. S., Cavalli, E., McCubrey, J., Hernandez-Bello, J., Munoz-Valle, J. F., Fagone, P., et al. (2022). The PI3K/Akt/mTOR pathway: A potential pharmacological target in COVID-19. Drug Discov. Today 27, 848–856. doi:10.1016/j.drudis.2021.11.002
Beltrán-Camacho, L., Eslava-Alcon, S., Rojas-Torres, M., Sanchez-Morillo, D., Martinez-Nicolas, M. P., Martin-Bermejo, V., et al. (2022). The serum of COVID-19 asymptomatic patients up-regulates proteins related to endothelial dysfunction and viral response in circulating angiogenic cells ex-vivo. Mol. Med. 28, 40. doi:10.1186/s10020-022-00465-w
Bennet, S., Kaufmann, M., Takami, K., Sjaarda, C., Douchant, K., Moslinger, E., et al. (2022). Small-molecule metabolome identifies potential therapeutic targets against COVID-19. Sci. Rep. 12, 10029. doi:10.1038/s41598-022-14050-y
Berlin, D. A., Gulick, R. M., and Martinez, F. J. (2020). Severe covid-19. N. Engl. J. Med. 383, 2451–2460. doi:10.1056/NEJMcp2009575
Birungi, G., Chen, S. M., Loy, B. P., Ng, M. L., and Li, S. F. Y. (2010). Metabolomics approach for investigation of effects of dengue virus infection using the EA.hy926 cell line. J. Proteome Res. 9, 6523–6534. doi:10.1021/pr100727m
Bojkova, D., Klann, K., Koch, B., Widera, M., Krause, D., Ciesek, S., et al. (2020). Proteomics of SARS-CoV-2-infected host cells reveals therapy targets. Nature 583, 469–472. doi:10.1038/s41586-020-2332-7
Byeon, S. K., Madugundu, A. K., Garapati, K., Ramarajan, M. G., Saraswat, M., Kumar-M, P., et al. (2022). Development of a multiomics model for identification of predictive biomarkers for COVID-19 severity: A retrospective cohort study. Lancet Digit. heal. 4, e632–e645. doi:10.1016/S2589-7500(22)00112-1
Calvano, C. D., Monopoli, A., Cataldi, T. R. I., and Palmisano, F. (2018). MALDI matrices for low molecular weight compounds: An endless story? Anal. Bioanal. Chem. 410, 4015–4038. doi:10.1007/s00216-018-1014-x
Carapito, R., Li, R., Helms, J., Carapito, C., Gujja, S., Rolli, V., et al. (2022). Identification of driver genes for critical forms of COVID-19 in a deeply phenotyped young patient cohort. Sci. Transl. Med. 14, eabj7521. doi:10.1126/scitranslmed.abj7521
Castañé, H., Iftimie, S., Baiges-Gaya, G., Rodriguez-Tomas, E., Jimenez-Franco, A., Lopez-Azcona, A. F., et al. (2022). Machine learning and semi-targeted lipidomics identify distinct serum lipid signatures in hospitalized COVID-19-positive and COVID-19-negative patients. Metabolism 131, 155197. doi:10.1016/j.metabol.2022.155197
CDC COVID-19 Response Team (2020). Severe outcomes among patients with coronavirus disease 2019 (COVID-19) — United States, february 12–march 16, 2020. MMWR. Morb. Mortal. Wkly. Rep. 69, 343–346. doi:10.15585/mmwr.mm6912e2
Celaya-Padilla, J. M., Villagrana-Banuelos, K. E., Oropeza-Valdez, J. J., Monarrez-Espino, J., Castaneda-Delgado, J. E., Oostdam, A. S. H. V., et al. (2021). Kynurenine and hemoglobin as sex-specific variables in COVID-19 patients: A machine learning and genetic algorithms approach. Diagnostics 11, 2197–2230. doi:10.3390/diagnostics11122197
Chai, H., Zhou, X., Zhang, Z., Rao, J., Zhao, H., and Yang, Y. (2021). Integrating multi-omics data through deep learning for accurate cancer prognosis prediction. Comput. Biol. Med. 134, 104481. doi:10.1016/j.compbiomed.2021.104481
Chandler, J. D., Hu, X., Ko, E. J., Park, S., Lee, Y. T., Orr, M., et al. (2016). Metabolic pathways of lung inflammation revealed by high-resolution metabolomics (HRM) of H1N1 influenza virus infection in mice. Am. J. Physiol. - Regul. Integr. Comp. Physiol. 311, R906–R916. doi:10.1152/ajpregu.00298.2016
Chaudhary, K., Poirion, O. B., Lu, L., and Garmire, L. X. (2018). Deep learning–based multi-omics integration robustly predicts survival in liver cancer. Clin. Cancer Res. 24, 1248–1259. doi:10.1158/1078-0432.CCR-17-0853
Ciccarelli, M., Merciai, F., Carrizzo, A., Sommella, E., Di Pietro, P., Caponigro, V., et al. (2022). Untargeted lipidomics reveals specific lipid profiles in COVID-19 patients with different severity from Campania region (Italy). J. Pharm. Biomed. Anal. 217, 114827. doi:10.1016/j.jpba.2022.114827
Cohen, J. (1992). Statistical power analysis. Curr. Dir. Psychol. Sci. 1, 98–101. doi:10.1111/1467-8721.ep10768783
Collard, D., Nurmohamed, N. S., Kaiser, Y., Reeskamp, L. F., Dormans, T., Moeniralam, H., et al. (2021). Cardiovascular risk factors and COVID-19 outcomes in hospitalised patients: A prospective cohort study. BMJ Open 11, e045482–e045487. doi:10.1136/bmjopen-2020-045482
Combrisson, E., and Jerbi, K. (2015). Exceeding chance level by chance: The caveat of theoretical chance levels in brain signal classification and statistical assessment of decoding accuracy. J. Neurosci. Methods 250, 126–136. doi:10.1016/j.jneumeth.2015.01.010
Costa, M. M., Martin, H., Estellon, B., Dupé, F. X., Saby, F., Benoit, N., et al. (2022). Exploratory study on application of MALDI-TOF-MS to detect SARS-CoV-2 infection in human saliva. J. Clin. Med. 11, 295. doi:10.3390/jcm11020295
D’Alessandro, A., Thomas, T., Dzieciatkowska, M., Hill, R. C., Francis, R. O., Hudson, K. E., et al. (2020). Serum proteomics in COVID-19 patients: Altered coagulation and complement status as a function of IL-6 level. J. Proteome Res. 19, 4417–4427. doi:10.1021/acs.jproteome.0c00365
D’Alessandro, A., Akpan, I., Thomas, T., Reisz, J., Cendali, F., Gamboni, F., et al. (2021). Biological and clinical factors contributing to the metabolic heterogeneity of hospitalized patients with and without COVID-19. Res. Sq. doi:10.21203/RS.3.RS-480167/V1
Danlos, F. X., Grajeda-Iglesias, C., Durand, S., Sauvat, A., Roumier, M., Cantin, D., et al. (2021). Metabolomic analyses of COVID-19 patients unravel stage-dependent and prognostic biomarkers. Cell Death Dis. 12, 258. doi:10.1038/s41419-021-03540-y
De Almeida, C. M., Motta, L. C., Folli, G. S., Marcarini, W. D., Costa, C. A., Vilela, A. C. S., et al. (2022). MALDI(+) FT-ICR mass spectrometry (MS) combined with machine learning toward saliva-based diagnostic screening for COVID-19. J. Proteome Res. 21, 1868–1875. doi:10.1021/acs.jproteome.2c00148
Delafiori, J., Navarro, L. C., Siciliano, R. F., de Melo, G. C., Busanello, E. N. B., Nicolau, J. C., et al. (2021). Covid-19 automated diagnosis and risk assessment through metabolomics and machine learning. Anal. Chem. 93, 2471–2479. doi:10.1021/acs.analchem.0c04497
Demichev, V., Tober-Lau, P., Lemke, O., Nazarenko, T., Thibeault, C., Whitwell, H., et al. (2021). A time-resolved proteomic and prognostic map of COVID-19. Cell Syst. 12, 780–794.e7. doi:10.1016/j.cels.2021.05.005
Demichev, V., Tober-Lau, P., Nazarenko, T., Lemke, O., Kaur Aulakh, S., Whitwell, H. J., et al. (2022). A proteomic survival predictor for COVID-19 patients in intensive care. PLOS Digit. Heal. 1, e0000007. doi:10.1371/journal.pdig.0000007
Dillard, L. R., Wase, N., Ramakrishnan, G., Park, J. J., Sherman, N. E., Carpenter, R., et al. (2022). Leveraging metabolic modeling to identify functional metabolic alterations associated with COVID-19 disease severity. Metabolomics 18, 51. doi:10.1007/s11306-022-01904-9
Drake, R. R., and Kislinger, T. (2014). The proteomics of prostate cancer exosomes. Expert Rev. Proteomics 11, 167–177. doi:10.1586/14789450.2014.890894
Erener, S. (2020). Diabetes, infection risk and COVID-19. Mol. Metab. 39, 101044. doi:10.1016/j.molmet.2020.101044
Gheware, A., Ray, A., Rana, D., Bajpai, P., Nambirajan, A., Arulselvi, S., et al. (2022). ACE2 protein expression in lung tissues of severe COVID-19 infection. Sci. Rep. 12, 4058–4110. doi:10.1038/s41598-022-07918-6
Gimeno-Miguel, A., Bliek-Bueno, K., Poblador-Plou, B., Carmona-Pirez, J., Poncel-Falco, A., Gonzalez-Rubio, F., et al. (2021). Chronic diseases associated with increased likelihood of hospitalization and mortality in 68,913 COVID-19 confirmed cases in Spain: A population-based cohort study. PLoS One 16, 02598222–e259914. doi:10.1371/journal.pone.0259822
Gisby, J., Clarke, C. L., Medjeral-Thomas, N., Malik, T. H., Papadaki, A., Mortimer, P. M., et al. (2021). Longitudinal proteomic profiling of dialysis patients with Covid-19 reveals markers of severity and predictors of death. Elife 10, e64827. doi:10.7554/eLife.64827
Gou, W., Fu, Y., Yue, L., Chen, G. D., Cai, X., Shuai, M., et al. (2021). Gut microbiota, inflammation, and molecular signatures of host response to infection. J. Genet. Genomics 48, 792–802. doi:10.1016/j.jgg.2021.04.002
Gutmann, C., Takov, K., Burnap, S. A., Singh, B., Ali, H., Theofilatos, K., et al. (2021). SARS-CoV-2 RNAemia and proteomic trajectories inform prognostication in COVID-19 patients admitted to intensive care. Nat. Commun. 12, 3406. doi:10.1038/s41467-021-23494-1
Hajduk, J., Matysiak, J., Kokot, P., Nowicki, P., Dereziński, P., and Kokot, Z. J. (2015). The application of fuzzy statistics and linear discriminant analysis as criteria for optimizing the preparation of plasma for matrix-assisted laser desorption/ionization mass spectrometry peptide profiling. Clin. Chim. Acta 448, 174–181. doi:10.1016/j.cca.2015.06.025
Hajduk, J., Matysiak, J., and Kokot, Z. J. (2016). Challenges in biomarker discovery with MALDI-TOF MS. Clin. Chim. Acta 458, 84–98. doi:10.1016/j.cca.2016.04.033
Han, X., and Gross, R. W. (2003). Global analyses of cellular lipidomes directly from crude extracts of biological samples by ESI mass spectrometry: A bridge to lipidomics. J. Lipid Res. 44, 1071–1079. doi:10.1194/jlr.R300004-JLR200
Harsha, H. C., and Pandey, A. (2010). Phosphoproteomics in cancer. Mol. Oncol. 4, 482–495. doi:10.1016/j.molonc.2010.09.004
Hasan, M. R., Suleiman, M., and Pérez-López, A. (2021). Metabolomics in the diagnosis and prognosis of COVID-19. Front. Genet. 12, 721556. doi:10.3389/fgene.2021.721556
Hasin, Y., Seldin, M., and Lusis, A. (2017). Multi-omics approaches to disease. Genome Biol. 18, 83–15. doi:10.1186/s13059-017-1215-1
Jen, K. Y., Albahra, S., Yen, F., Sageshima, J., Chen, L. X., Tran, N., et al. (2021). Automated en masse machine learning model generation shows comparable performance as classic regression models for predicting delayed graft function in renal allografts. Transplantation 105, 2646–2654. doi:10.1097/TP.0000000000003640
Jia, H., Liu, C., Li, D., Huang, Q., Liu, D., Zhang, Y., et al. (2022). Metabolomic analyses reveal new stage-specific features of COVID-19. Eur. Respir. J. 59, 2100284. doi:10.1183/13993003.00284-2021
Kelchtermans, P., Bittremieux, W., De Grave, K., Degroeve, S., Ramon, J., Laukens, K., et al. (2014). Machine learning applications in proteomics research: How the past can boost the future. Proteomics 14, 353–366. doi:10.1002/pmic.201300289
Kim, D. K., Weller, B., Lin, C. W., Sheykhkarimli, D., Knapp, J. J., Dugied, G., et al. (2023). A proteome-scale map of the SARS-CoV-2–human contactome. Nat. Biotechnol. 41, 140–149. doi:10.1038/s41587-022-01475-z
Klein, S. L., Dhakal, S., Ursin, R. L., Deshpande, S., Sandberg, K., and Mauvais-Jarvis, F. (2020). Biological sex impacts COVID-19 outcomes. PLoS Pathog. 16, e1008570–e1008575. doi:10.1371/journal.ppat.1008570
Lamers, M. M., and Haagmans, B. L. (2022). SARS-CoV-2 pathogenesis. Nat. Rev. Microbiol. 20, 270–284. doi:10.1038/s41579-022-00713-0
Laponogov, I., Gonzalez, G., Shepherd, M., Qureshi, A., Veselkov, D., Charkoftaki, G., et al. (2021). Network machine learning maps phytochemically rich “Hyperfoods” to fight COVID-19. Hum. Genomics 15, 1–11. doi:10.1186/s40246-020-00297-x
Lazari, L. C., Ghilardi, F. D. R., Rosa-Fernandes, L., Assis, D. M., Nicolau, J. C., Santiago, V. F., et al. (2021). Prognostic accuracy of MALDI-TOF mass spectrometric analysis of plasma in COVID-19. Life Sci. Alliance 4, 2020009466–e202001012. doi:10.26508/lsa.202000946
Lazari, L. C., Zerbinati, R. M., Rosa-Fernandes, L., Santiago, V. F., Rosa, K. F., Angeli, C. B., et al. (2022). MALDI-TOF mass spectrometry of saliva samples as a prognostic tool for COVID-19. J. Oral Microbiol. 14, 2043651. doi:10.1080/20002297.2022.2043651
Li, Y., Wang, Y., Liu, H., Sun, W., Ding, B., Zhao, Y., et al. (2020). Urine proteome of COVID-19 patients. Urine 2, 1–8. doi:10.1016/j.urine.2021.02.001
Liebal, U. W., Phan, A. N. T., Sudhakar, M., Raman, K., and Blank, L. M. (2020). Machine learning applications for mass spectrometry-based metabolomics. Metabolites 10, 243–323. doi:10.3390/metabo10060243
Lipman, D., Safo, S. E., and Chekouo, T. (2022). Multi-omic analysis reveals enriched pathways associated with COVID-19 and COVID-19 severity. PLoS One 17, e0267047. doi:10.1371/journal.pone.0267047
Liu, X., Cao, Y., Fu, H., Wei, J., Chen, J., Hu, J., et al. (2021). Proteomics analysis of serum from COVID-19 patients. ACS Omega 6, 7951–7958. doi:10.1021/acsomega.1c00616
Liu, J., Li, Z. B., Lu, Q. Q., Yu, Y., Zhang, S. Q., Ke, P. F., et al. (2022). Metabolite profile of COVID-19 revealed by UPLC-MS/MS-based widely targeted metabolomics. Front. Immunol. 13, 894170–894213. doi:10.3389/fimmu.2022.894170
Liu, J. F., Zhou, Y. N., Lu, S. Y., Yang, Y. H., Wu, S. F., Liu, D. P., et al. (2022). Proteomic and phosphoproteomic profiling of COVID-19-associated lung and liver injury: A report based on rhesus macaques. Signal Transduct. Target. Ther. 7, 27. doi:10.1038/s41392-022-00882-7
Liu, Y., Song, L., Zheng, N., Shi, J., Wu, H., Yang, X., et al. (2022). A urinary proteomic landscape of COVID-19 progression identifies signaling pathways and therapeutic options. Sci. China Life Sci. 65, 1866–1880. doi:10.1007/s11427-021-2070-y
Liu, X., Hasan, M. R., Ahmed, K. A., and Hossain, M. Z. (2023). Machine learning to analyse omic-data for COVID-19 diagnosis and prognosis. BMC Bioinforma. 24, 7–20. doi:10.1186/s12859-022-05127-6
Lou, X., De Waal, B. F. M., Milroy, L. G., and Van Dongen, J. L. J. (2015). A sample preparation method for recovering suppressed analyte ions in MALDI TOF MS. J. Mass Spectrom. 50, 766–770. doi:10.1002/jms.3587
Mahat, R. K., Panda, S., Rathore, V., Swain, S., Yadav, L., and Sah, S. P. (2021). The dynamics of inflammatory markers in coronavirus disease-2019 (COVID-19) patients: A systematic review and meta-analysis. Clin. Epidemiol. Glob. Heal. 11, 100727. doi:10.1016/j.cegh.2021.100727
Mann, M., Kumar, C., Zeng, W. F., and Strauss, M. T. (2021). Artificial intelligence for proteomics and biomarker discovery. Cell Syst. 12, 759–770. doi:10.1016/j.cels.2021.06.006
Mansouri, V., Tavirani, M. R., Okhovatian, F., and Abbaszadeh, H. A. (2022). Introducing markers which are involved in COVID-19 disease severe condition versus mild state, a network analysis. J. Cell. Mol. Anesth. 7, 109–115.
McArdle, A., Washington, K. E., Chazarin Orgel, B., Binek, A., Manalo, D. M., Rivas, A., et al. (2021). Discovery proteomics for COVID-19: Where we are now. J. Proteome Res. 20, 4627–4639. doi:10.1021/acs.jproteome.1c00475
McCreath, G., Whitfield, P. D., Roe, A. J., Watson, M. J., and Sim, M. A. B. (2021). A metabolomics approach for the diagnosis of SecondAry InfeCtions in COVID-19 (MOSAIC): A study protocol. BMC Infect. Dis. 21, 1204–1206. doi:10.1186/s12879-021-06832-y
McGurnaghan, S. J., Weir, A., Bishop, J., Kennedy, S., Blackbourn, L. A. K., McAllister, D. A., et al. (2021). Risks of and risk factors for COVID-19 disease in people with diabetes: A cohort study of the total population of scotland. Lancet Diabetes Endocrinol. 9, 82–93. doi:10.1016/S2213-8587(20)30405-8
Meizlish, M. L., Pine, A. B., Bishai, J. D., Goshua, G., Nadelmann, E. R., Simonov, M., et al. (2021). A neutrophil activation signature predicts critical illness and mortality in COVID-19. Blood Adv. 5, 1164–1177. doi:10.1182/bloodadvances.2020003568
Mirza, B., Wang, W., Wang, J., Choi, H., Chung, N. C., and Ping, P. (2019). Machine learning and integrative analysis of biomedical big data. Genes (Basel). 10, 87. doi:10.3390/genes10020087
Mohammed, Y., Goodlett, D. R., Cheng, M. P., Vinh, D. C., Lee, T. C., Mcgeer, A., et al. (2022). Longitudinal plasma proteomics analysis reveals novel candidate biomarkers in acute COVID-19. J. Proteome Res. 21, 975–992. doi:10.1021/acs.jproteome.1c00863
Muñoz-Prieto, A., Rubić, I., Gonzalez-Sanchez, J. C., Kuleš, J., Martinez-Subiela, S., Ceron, J. J., et al. (2022). Saliva changes in composition associated to COVID-19: A preliminary study. Sci. Rep. 12, 10879–10914. doi:10.1038/s41598-022-14830-6
Nachtigall, F. M., Pereira, A., Trofymchuk, O. S., and Santos, L. S. (2020). Detection of SARS-CoV-2 in nasal swabs using MALDI-MS. Nat. Biotechnol. 38, 1168–1173. doi:10.1038/s41587-020-0644-7
Nagaraj, N. S., Singh, O. V., and Merchant, N. B. (2010). Proteomics: A strategy to understand the novel targets in protein misfolding and cancer therapy. Expert Rev. Proteomics 7, 613–623. doi:10.1586/epr.10.70
Nakayasu, E. S., Gritsenko, M., Piehowski, P. D., Gao, Y., Orton, D. J., Schepmoes, A. A., et al. (2021). Tutorial: Best practices and considerations for mass-spectrometry-based protein biomarker discovery and validation. Nat. Protoc. 16, 3737–3760. doi:10.1038/s41596-021-00566-6
O’Rourke, M. B., Djordjevic, S. P., and Padula, M. P. (2016). The quest for improved reproducibility in MALDI mass spectrometry. Mass Spectrom. Rev. 37, 217–228. doi:10.1002/mas.21515
Ortiz, A., Cozzolino, M., Fliser, D., Fouque, D., Goumenos, D., Massy, Z. A., et al. (2021). Chronic kidney disease is a key risk factor for severe COVID-19: A call to action by the ERA-edta. Nephrol. Dial. Transpl. 36, 87–94. doi:10.1093/ndt/gfaa314
Overmyer, K. A., Shishkova, E., Miller, I. J., Balnis, J., Bernstein, M. N., Peters-Clarke, T. M., et al. (2021). Large-scale multi-omic analysis of COVID-19 severity. Cell Syst. 12, 23–40.e7. doi:10.1016/j.cels.2020.10.003
Pan, S., Brentnall, T. A., and Chen, R. (2016). Glycoproteins and glycoproteomics in pancreatic cancer. World J. Gastroenterol. 22, 9288–9299. doi:10.3748/wjg.v22.i42.9288
Park, J., Kim, H., Kim, S. Y., Kim, Y., Lee, J. S., Dan, K., et al. (2020). In-depth blood proteome profiling analysis revealed distinct functional characteristics of plasma proteins between severe and non-severe COVID-19 patients. Sci. Rep. 10, 22418–22510. doi:10.1038/s41598-020-80120-8
Penna, C., Mercurio, V., Tocchetti, C. G., and Pagliaro, P. (2020). Sex-related differences in COVID-19 lethality. Br. J. Pharmacol. 177, 4375–4385. doi:10.1111/bph.15207
Picard, M., Scott-Boyer, M. P., Bodein, A., Périn, O., and Droit, A. (2021). Integration strategies of multi-omics data for machine learning analysis. Comput. Struct. Biotechnol. J. 19, 3735–3746. doi:10.1016/j.csbj.2021.06.030
Pinto, B. G. G., Oliveira, A. E. R., Singh, Y., Jimenez, L., Goncalves, A. N. A., Ogava, R. L. T., et al. (2020). ACE2 expression is increased in the lungs of patients with comorbidities associated with severe COVID-19. J. Infect. Dis. 222, 556–563. doi:10.1093/infdis/jiaa332
Pinu, F. R., Beale, D. J., Paten, A. M., Kouremenos, K., Swarup, S., Schirra, H. J., et al. (2019). Systems biology and multi-omics integration: Viewpoints from the metabolomics research community. Metabolites 9, 76–31. doi:10.3390/metabo9040076
Pongracz, T., Nouta, J., Wang, W., van Meijgaarden, K. E., Linty, F., Vidarsson, G., et al. (2022). Immunoglobulin G1 Fc glycosylation as an early hallmark of severe COVID-19. eBioMedicine 78, 103957. doi:10.1016/j.ebiom.2022.103957
Pozzi, C., Levi, R., Braga, D., Carli, F., Darwich, A., Spadoni, I., et al. (2022). A ‘multiomic’ approach of saliva metabolomics, microbiota, and serum biomarkers to assess the need of hospitalization in coronavirus disease 2019. Gastro Hep Adv. 1, 194–209. doi:10.1016/j.gastha.2021.12.006
Pranata, R., Lim, M. A., Huang, I., Raharjo, S. B., and Lukito, A. A. (2020). Hypertension is associated with increased mortality and severity of disease in COVID-19 pneumonia: A systematic review, meta-analysis and meta-regression. J. Renin-Angiotensin-Aldosterone Syst. 21, 1470320320926899. doi:10.1177/1470320320926899
Qian, W. J., Kaleta, D. T., Petritis, B. O., Jiang, H., Liu, T., Zhang, X., et al. (2008). Enhanced detection of low abundance human plasma proteins using a tandem IgY12-SuperMix immunoaffinity separation strategy. Mol. Cell. Proteomics 7, 1963–1973. doi:10.1074/mcp.M800008-MCP200
Rahnavard, A., Mann, B., Giri, A., Chatterjee, R., and Crandall, K. A. (2022). Metabolite, protein, and tissue dysfunction associated with COVID-19 disease severity. Sci. Rep. 12, 12204–12216. doi:10.1038/s41598-022-16396-9
Rajoub, B. (2020). “Supervised and unsupervised learning,” in Biomedical signal processing and artificial intelligence in healthcare (Elsevier). doi:10.1016/B978-0-12-818946-7.00003-2
Reel, P. S., Reel, S., Pearson, E., Trucco, E., and Jefferson, E. (2021). Using machine learning approaches for multi-omics data analysis: A review. Biotechnol. Adv. 49, 107739. doi:10.1016/j.biotechadv.2021.107739
Ren, Z., Wang, H., Cui, G., Lu, H., Wang, L., Luo, H., et al. (2021). Alterations in the human oral and gut microbiomes and lipidomics in COVID-19. Gut 70, 1253–1265. doi:10.1136/gutjnl-2020-323826
Renuse, S., Vanderboom, P. M., Maus, A. D., Kemp, J. V., Gurtner, K. M., Madugundu, A. K., et al. (2021). A mass spectrometry-based targeted assay for detection of SARS-CoV-2 antigen from clinical specimens. EBioMedicine 69, 103465. doi:10.1016/j.ebiom.2021.103465
Richard, V. R., Gaither, C., Popp, R., Chaplygina, D., Brzhozovskiy, A., Kononikhin, A., et al. (2022). Early prediction of COVID-19 patient survival by targeted plasma multi-omics and machine learning. Mol. Cell. Proteomics 21, 100277–100314. doi:10.1016/j.mcpro.2022.100277
Romero Starke, K., Petereit-Haack, G., Schubert, M., Kämpf, D., Schliebner, A., Hegewald, J., et al. (2020). The age-related risk of severe outcomes due to Covid-19 infection: A rapid review, meta-analysis, and meta-regression. Int. J. Environ. Res. Public Health 17, 1–24. doi:10.3390/ijerph17165974
Romero Starke, K., Reissig, D., Petereit-Haack, G., Schmauder, S., Nienhaus, A., Seidler, A., et al. (2021). The isolated effect of age on the risk of COVID-19 severe outcomes: A systematic review with meta-analysis. BMJ Glob. Heal. 6, 1–12. doi:10.1136/bmjgh-2021-006434
Rong, Z. H. U., Lingyun, D. A. I., Jinxing, L. I. U., and Ying, G. U. O. (2021). Diagnostic classification of lung cancer using deep transfer learning technology and multi-omics data. Chin. J. Electron. 30, 843–852. doi:10.1049/cje.2021.06.006
Rossel, S., Barco, A., Kloppmann, M., Martinez Arbizu, P., Huwer, B., and Knebelsberger, T. (2021). Rapid species level identification of fish eggs by proteome fingerprinting using MALDI-TOF MS. J. Proteomics 231, 103993. doi:10.1016/j.jprot.2020.103993
Sardar, R., Sharma, A., and Gupta, D. (2021). Machine learning assisted prediction of prognostic biomarkers associated with COVID-19, using clinical and proteomics data. Front. Genet. 12, 636441. doi:10.3389/fgene.2021.636441
Schuurman, A. R., Leopold, V., Pereverzeva, L., Chouchane, O., Reijnders, T. D. Y., Brabander, J. d., et al. (2022). The platelet lipidome is altered in patients with COVID-19 and correlates with platelet reactivity. Thromb. Haemost. 122, 1683–1692. doi:10.1055/s-0042-1749438
Shen, B., Yi, X., Sun, Y., Bi, X., Du, J., Zhang, C., et al. (2020). Proteomic and metabolomic characterization of COVID-19 patient sera. Cell 182, 59–72.e15. doi:10.1016/j.cell.2020.05.032
Shi, D., Yan, R., Lv, L., Jiang, H., Lu, Y., Sheng, J., et al. (2021). The serum metabolome of COVID-19 patients is distinctive and predictive. Metabolism 118, 154739. doi:10.1016/j.metabol.2021.154739
Shinde, P. P., and Shah, S. (2018). “A review of machine learning and deep learning applications,” in Proceedings of the 2018 Fourth International Conference on Computing Communication Control and Automation (ICCUBEA). doi:10.1109/ICCUBEA.2018.8697857
Shu, T., Ning, W., Wu, D., Xu, J., Han, Q., Huang, M., et al. (2020). Plasma proteomics identify biomarkers and pathogenesis of COVID-19. Immunity 53, 1108–1122.e5. doi:10.1016/j.immuni.2020.10.008
Silberring, J., and Ciborowski, P. (2010). Biomarker discovery and clinical proteomics. Trac. - Trends Anal. Chem. 29, 128–140. doi:10.1016/j.trac.2009.11.007
Sindelar, M., Stancliffe, E., Schwaiger-Haber, M., Anbukumar, D. S., Adkins-Travis, K., Goss, C. W., et al. (2021). Longitudinal metabolomics of human plasma reveals prognostic markers of COVID-19 disease severity. Cell Rep. Med. 2, 100369. doi:10.1016/j.xcrm.2021.100369
Spick, M., Longman, K., Frampas, C., Lewis, H., Costa, C., Walters, D. D., et al. (2021). Changes to the sebum lipidome upon COVID-19 infection observed via rapid sampling from the skin. EClinicalMedicine 33, 100786. doi:10.1016/j.eclinm.2021.100786
Spiering, A. E., and de Vries, T. J. (2021). Why females do better: The X chromosomal TLR7 gene-dose effect in COVID-19. Front. Immunol. 12, 756262–756313. doi:10.3389/fimmu.2021.756262
Subramanian, I., Verma, S., Kumar, S., Jere, A., and Anamika, K. (2020). Multi-omics data integration, interpretation, and its application. Bioinform. Biol. Insights 14, 1177932219899051–1177932219899059. doi:10.1177/1177932219899051
Sun, C., Bai, Y., Chen, D., He, L., Zhu, J., Ding, X., et al. (2021). Accurate classification of COVID-19 patients with different severity via machine learning. Clin. Transl. Med. 11, e323–e327. doi:10.1002/ctm2.323
Suvarna, K., Biswas, D., Pai, M. G. J., Acharjee, A., Bankar, R., Palanivel, V., et al. (2021). Proteomics and machine learning approaches reveal a set of prognostic markers for COVID-19 severity with drug repurposing potential. Front. Physiol. 12, 652799–652818. doi:10.3389/fphys.2021.652799
Thongboonkerd, V. (2007). Practical points in urinary proteomics. J. Proteome Res. 6, 3881–3890. doi:10.1021/pr070328s
Tong, L., Mitchel, J., Chatlin, K., and Wang, M. D. (2020). Deep learning based feature-level integration of multi-omics data for breast cancer patients survival analysis. BMC Med. Inf. Decis. Mak. 20, 225–312. doi:10.1186/s12911-020-01225-8
Tran, N. K., Howard, T., Walsh, R., Pepper, J., Loegering, J., Phinney, B., et al. (2021). Novel application of automated machine learning with MALDI-TOF-MS for rapid high-throughput screening of COVID-19: A proof of concept. Sci. Rep. 11, 8219–8310. doi:10.1038/s41598-021-87463-w
Uchiyama, K., Yagi, N., Mizushima, K., Higashimura, Y., Hirai, Y., Okayama, T., et al. (2017). Serum metabolomics analysis for early detection of colorectal cancer. J. Gastroenterol. 52, 677–694. doi:10.1007/s00535-016-1261-6
Ussher, J. R., Elmariah, S., Gerszten, R. E., and Dyck, J. R. B. (2016). The emerging role of metabolomics in the diagnosis and prognosis of cardiovascular disease. J. Am. Coll. Cardiol. 68, 2850–2870. doi:10.1016/j.jacc.2016.09.972
Valo, E., Colombo, M., Sandholm, N., McGurnaghan, S. J., Blackbourn, L. A. K., Dunger, D. B., et al. (2022). Effect of serum sample storage temperature on metabolomic and proteomic biomarkers. Sci. Rep. 12, 4571–4610. doi:10.1038/s41598-022-08429-0
Vanderboom, P. M., Renuse, S., Maus, A. D., Madugundu, A. K., Kemp, J. V., Gurtner, K. M., et al. (2022). Machine learning-based fragment selection improves the performance of qualitative PRM assays. J. Proteome Res. 21, 2045–2054. doi:10.1021/acs.jproteome.2c00156
Wang, C., Li, X., Ning, W., Gong, S., Yang, F., Fang, C., et al. (2021). Multi-omic profiling of plasma reveals molecular alterations in children with COVID-19. Theranostics 11, 8008–8026. doi:10.7150/thno.61832
Wang, Z., Cryar, A., Lemke, O., Tober-Lau, P., Ludwig, D., Helbig, E. T., et al. (2022). A multiplex protein panel assay for severity prediction and outcome prognosis in patients with COVID-19: An observational multi-cohort study. EClinicalMedicine 49, 101495. doi:10.1016/j.eclinm.2022.101495
Xie, G., Dong, C., Kong, Y., Zhong, J. F., Li, M., and Wang, K. (2019). Group lasso regularized deep learning for cancer prognosis from multi-omics and clinical features. Genes (Basel). 10, 240. doi:10.3390/genes10030240
Yan, L., Yi, J., Huang, C., Zhang, J., Fu, S., Li, Z., et al. (2021). Rapid detection of COVID-19 using MALDI-TOF-based serum peptidome profiling. Anal. Chem. 93, 4782–4787. doi:10.1021/acs.analchem.0c04590
Yang, K., and Hhan, X. (2016). Lipidomics: Techniques, applications, and outcomes related to biomedical sciences. Trends Biochem. Sci. 41, 954–969. doi:10.1016/j.tibs.2016.08.010
Yang, Z., Wu, D., Lu, S., Qiu, Y., Hua, Z., Tan, F., et al. (2022). Plasma metabolome and cytokine profile reveal glycylproline modulating antibody fading in convalescent COVID-19 patients. Proc. Natl. Acad. Sci. U. S. A. 119, e2117089119. doi:10.1073/pnas.2117089119
Yaşar, Ş., Çolak, C., and Yoloğlu, S. (2021). Artificial intelligence-based prediction of covid-19 severity on the results of protein profiling. Comput. Methods Programs Biomed. 202, 105996. doi:10.1016/j.cmpb.2021.105996
Zeng, X., Song, X., Ma, T., Pan, X., Zhou, Y., Hou, Y., et al. (2020). Repurpose open data to discover therapeutics for COVID-19 using deep learning. J. Proteome Res. 19, 4624–4636. doi:10.1021/acs.jproteome.0c00316
Zeng, H. L., Chen, D., Yan, J., Yang, Q., Han, Q. Q., Li, S. S., et al. (2021). Proteomic characteristics of bronchoalveolar lavage fluid in critical COVID-19 patients. FEBS J. 288, 5190–5200. doi:10.1111/febs.15609
Zhang, Z., Zhao, Y., Liao, X., Shi, W., Li, K., Zou, Q., et al. (2019). Deep learning in omics: A survey and guideline. Brief. Funct. Genomics 18, 41–57. doi:10.1093/bfgp/ely030
Zhang, Y., Cai, X., Ge, W., Wang, D., Zhu, G., Qian, L., et al. (2022). Potential use of serum proteomics for monitoring COVID-19 progression to complement RT-PCR detection. J. Proteome Res. 21, 90–100. doi:10.1021/acs.jproteome.1c00525
Zhang, Z., Liu, F., Li, Q., Li, Y., Zhu, Z., Guo, H., et al. (2022). Proteomic profiling reveals a distinctive molecular signature for critically ill COVID-19 patients compared with asthma and chronic obstructive pulmonary disease. Int. J. Infect. Dis. 116, 258–267. doi:10.1016/j.ijid.2022.01.008
Zhou, F., Yu, T., Du, R., Fan, G., Liu, Y., Liu, Z., et al. (2020). Clinical course and risk factors for mortality of adult inpatients with COVID-19 in wuhan, China: A retrospective cohort study. Lancet 395, 1054–1062. doi:10.1016/S0140-6736(20)30566-3
Keywords: COVID-19, mass spectrometry, machine learning, biomarkers, omics
Citation: Lazari LC, Santos de Oliveira G, Macedo-Da-Silva J, Rosa-Fernandes L and Palmisano G (2023) Mass spectrometry and machine learning in the identification of COVID-19 biomarkers. Front. Anal. Sci. 3:1119438. doi: 10.3389/frans.2023.1119438
Received: 08 December 2022; Accepted: 14 March 2023;
Published: 31 March 2023.
Edited by:
Manveen K. Sethi, Boston University, United StatesReviewed by:
Sayantani Chatterjee, Boston University, United StatesCopyright © 2023 Lazari, Santos de Oliveira, Macedo-Da-Silva, Rosa-Fernandes and Palmisano. This is an open-access article distributed under the terms of the Creative Commons Attribution License (CC BY). The use, distribution or reproduction in other forums is permitted, provided the original author(s) and the copyright owner(s) are credited and that the original publication in this journal is cited, in accordance with accepted academic practice. No use, distribution or reproduction is permitted which does not comply with these terms.
*Correspondence: Giuseppe Palmisano, cGFsbWlzYW5vLmdwQGdtYWlsLmNvbQ==
†These authors have contributed equally to this work
Disclaimer: All claims expressed in this article are solely those of the authors and do not necessarily represent those of their affiliated organizations, or those of the publisher, the editors and the reviewers. Any product that may be evaluated in this article or claim that may be made by its manufacturer is not guaranteed or endorsed by the publisher.
Research integrity at Frontiers
Learn more about the work of our research integrity team to safeguard the quality of each article we publish.