- Department of Material Process Engineering, Nagoya University, Nagoya, Japan
Coal has been an important energy source worldwide; however, it is the largest source of nitrogen oxide (NOx) emissions because the amount of nitrogen in coal is larger than that of other fossil fuels. Precise control of NOx emissions is required in operations of coal-fired power plants from the viewpoint of air pollution control. Although theoretical analyses of NOx generation from a coal-fired power plant have been conducted, it is difficult to precisely predict NOx generation in an actual plant. NOx generation is affected by various factors, such as furnace design and operating conditions, and there are complicated relationships among them. Thus, it is necessary to identify important operating factors that affect NOx generation in actual coal-fired power plants. A linear non-Gaussian acyclic model (LiNGAM) is an exploratory causal analysis method that identifies a causal ordering of variables and their connection strengths without any prior knowledge of causal relationships among variables. In this study, we analyzed real operation data collected from a coal-fired power plant using LiNGAM to identify factors of NOx generation. The causal relationship between process variables and NOx generation was estimated by means of LiNGAM, and the connectional strengths of the variables on NOx generation were derived. The analysis results agreed with previous reports on NOx generation mechanisms, such as combustion air temperature, steam temperature on a specific side of the furnace, and air flow rate of forced draft fans. In addition, we found the steam flow rate and the furnace pressure as new candidate factors of NOx generation through causal analysis using LiNGAM, which heretofore has not been suggested. Our analysis result should contribute to reducing NOx emissions from coal-fired power plants in the future.
1 Introduction
A coal-fired power plant must be monitored and controlled well to maintain sustainable and clean emissions despite variations in external factors. In particular, nitrogen oxide (NOx) emission is one of the most critical matters in the operation of coal-fired power plants because it is the largest source of NOx emission. The amount of nitrogen in coal is larger than that of other fossil fuels (Gonzalez-Salazar et al. (2018)). To understand deeply the relationship between plant operation and NOx emissions is critical for process monitoring and control although some factors affecting NOx emission are known such as coal properties, operation settings, equipment maintenance, and power demand (Strbac (2008); Ampimah et al. (2018)).
Process state estimation and factor identification of NOx generation in a coal-fired power plant based on first principle models have been attempted; however, these model-based methods remain applicable only to pilot systems (Vo et al. (2019); Ke et al. (2022)). It is difficult to comprehensively consider influence from external factors on daily operations using first principle models (Kadlec et al. (2009)).
The large amount of historical process data from long-running monitoring may contain rich information about NOx generation. Thus, data-driven approaches based on process data may be helpful in identifying factors of NOx generation and assisting optimal process management.
Deep learning models have been successfully applied to predict NOx emissions from boilers. Adams et al. applied a combination of a deep neural network and a least square support vector machine to predict SOx-NOx emissions in a coal-fired power plant (Adams et al. (2020)). They demonstrated the effect of coal properties on SOx-NOx emissions by comparing performances of models on NOx prediction, with and without coal properties. Liukkonen et al. also applied self-organizing maps (SOM) and k-means to estimate process states from combustion process data of a circulating Fluidized Bed (CFB) boiler (Liukkonen et al. (2011)). They individually selected input variables and developed NOx emission prediction models for multiple states. Wang. et al. developed an NOx emission prediction model using a Gaussian process (GP) (Wang et al. (2018)). The model confirmed that air-staged combustion, which is a well-known low NOx combustion technology, has significant impact on NOx generation.
Long short-term memory (LSTM), a kind of neural network, is a model suitable for processing time series data. Many studies have applied LSTM and reported good performance in dynamic NOx prediction (Tan et al. (2019); Yang et al. (2020); Song et al. (2022); Xie et al. (2020); Wang et al. (2022); Tuttle et al. (2021)). Some studies have reported that convolutional neural networks (CNN) accurately predicted emissions of NOx from coal-fired boilers (Li and Hu (2020); Saif-Ul-Allah et al. (2022,?)). In addition, deep hybrid neural networks also have been applied to CFB gas emission prediction (Hu et al. (2020)). The computational complexity thereof was almost the same as CNN and LSTM, and the prediction performance was approximately five times better than that of CNN and 7.5 times better than LSTM.
Most machine-learning (ML) models are black-boxes, and it is difficult for us to interpret the relationships between process variables and NOx generation. Attention mechanisms (AM) may help interpretation of ML models. In an analysis of gasoline engines, quantitative impact of each process variable on NOx emission was investigated using graph neural networks with AM (Chen et al. (2023)). Several studies have also applied AM to develop NOx emission models in coal-fired power plants; however, they did not always obtain good-interpretable models (Wan et al. (2022); Wang et al. (2022); Li et al. (2023)).
Path analysis or the structural equation modeling (SEM) approach is useful for process interpretation because it estimates the structural relationships between process variables. PLS-SEM (Hair et al. (2011)) and process PLS (van Kollenburg et al. (2021)) have been applied to structural modeling of industrial production processes (van Kollenburg et al. (2020); Offermans et al. (2021)). However, the relationship of process variables must be manually determined before constructing a model with these structural methods. Prior knowledge about causal relationships between process variables is only sometimes fully known in large and complex processes, including coal-fired power plants. Thus, we should adopt a method that does not require prior knowledge about processes.
Causal discovery methods are gaining popularity in industrial process analysis (Chen et al. (2018); Sun et al. (2022); Menegozzo et al. (2022)). Linear non-gaussian acyclic model (LiNGAM) is a useful causal discovery method for estimating a causal structure among variables based only on data without any prior knowledge of the structure (Shimizu et al. (2006; Shimizu et al. (2011)). LiNGAM has been widely adopted in various fields, such as the social sciences (Ferkingstad et al. (2011); Lai and Bessler (2015)) and neuroscience (Smith et al. (2011); Bielczyk et al. (2019)), and has been applied to fault diagnosis and causal discovery in industrial processeses in recent years (Uchida et al. (2022); Cao et al. (2022)). The analysis results using LiNGAM provide better insight into the process operation to process operators and engineers.
In this study, we applied LiNGAM to real process data of an actual coal-fired power plant in order to identify significant factors of NOx emission based on causality between process variables. By utilizing LiNGAM, we did not need prior knowledge of the process, which is not the case with other structural methods. We discuss the significant factors of NOx emission identified by LiNGAM based on the physicochemical knowledge of the coal-fired power plant. It was confirmed that some identified factors were consistent with previous studies; however, we found two new factors of NOx emission—-steam flow rate and furnace pressure—-that have not been mentioned in previous studies.
2 Materials and methods
2.1 Coal-fired power plant
This study focuses on a coal-fired power plant with a 700 MW supercritical tangential firing boiler built in the Kansai region of Japan and is operated using mixtures of various types of coal in consideration of economic efficiency. Figure 1 shows a schematic diagram of the overall system configuration (Zhang. (2013)).
Combustion air is classified into primary air, secondary air, and over-fire air (OFA), and is fed from a primary air fan and a forced draft fan, as indicated by (12) and (13), after being heated by a air preheater (8) before entering a furnace (3) in Figure 1.
The operation data of the coal-fired power plant consist of momentary values measured every hour, collected over approximately 6 years. NOx emission was measured at the inlet of the denitration equipment and represented as the dry gas basis at 6% O2. We clipped them only when the boiler load was over 680 MW because only then can it be regarded as a steady-state operation. The unsteady-state operation data, such as data obtained during coal brand changes, were removed. Outliers and noisy data were also eliminated by means of visual observation. In addition, some manipulated variables that did not change throughout the entire period were omitted from the analysis since these variables did not contain any information. Finally, the total number of analyzed samples was 10,763. Table 1 summarizes the process variables used for analysis. Each variable was standardized with zero mean and a standard deviation of one before analysis.
2.2 LiNGAM
LiNGAM is a model expressing the causal structure among variables from data containing confounders (Shimizu et al. (2006)). An example of a causal structure is shown in Figure 2. The vertices represent variables, and the directed edges express causal dependencies among the variables. For example, there is a directed edge from vertex x1 to x2, which means x1 has a causal effect on x2.
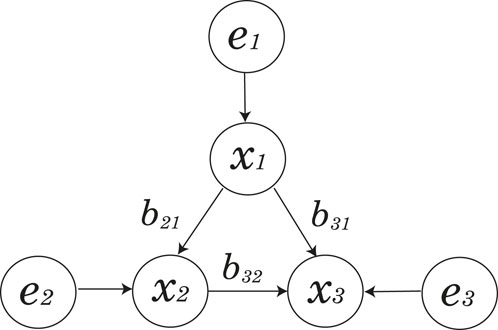
FIGURE 2. Example of causal structure among variables. Reproduced under CC-BY-4.0,Uchida et al. (2022).
LiNGAM assumes that each variable is generated as the linear combination of the causal antecedent variables and an exogenous variable. In the LiNGAM assumption, the causal structure is expressed as a directed acyclic graph (DAG) that is a directed graph without a cycle. An example of a LiNGAM model in Figure 2 is as shown by 1 and (2); Eq. (3)).
Where xi and ei (i = 1, 2, 3) are observed variables and exogenous noise, respectively. b21, b31, and b32 are coefficients.
When the data include p variables, the LiNGAM model is expressed as a linear Equation 4:
where
The goal of causal discovery with LiNGAM is to estimate the coefficient matrix B, which describes the causal relationships among the variables. Although there are some assumptions in the LiNGAM model, the most important assumption is that all variables in the data need to be continuous.
The LiNGAM coefficients on a row vector in B that are associated with NOx emissions express the causal effects of the factors on NOx emissions. Their absolute values and positive/negative signs indicate the strength and the direction of the causal effects. Thus, focus should be placed on a specific row vector β in B in this analysis. The absolute values and positive/negative signs of the extracted LiNGAM coefficients were compared to estimate the causal effects on NOx emissions.
Although several LiNGAM algorithms have been proposed, such as ICA-based LiNGAM (Shimizu et al. (2006)), Direct LiNGAM (Shimizu et al. (2011)), and Pairwise LiNGAM (Hyvärinen and Smith. (2013)), we adopted DirectLiNGAM for its ease of practical use.
3 Results
Figure 3 shows the causal effects of each variable on NOx emissions estimated by means of LiNGAM. The bars indicate the strengths and directions of the causal effects. The variables with positive values may increase NOx emission, and those with negative values may decrease NOx emission. The absolute values indicate the strength of the causal effects.
The steam flow rate (SFR) and the furnace pressure (FP) had the first and the second largest positive effects on NOx emission. The combustion air temperature (CAT) has a positive effect, which is a factor of thermal NOx. The steam temperature right wall of the furnace (STR) has a strong negative effect, and the steam temperature left wall of the furnace (STL) has a weak positive effect on NOx emissions. The forced draft fan-air flow rate (FDF-AFR) has a negative effect on NOx, which is understandable because FDF-AFR is associated with Fuel NOx.
4 Discussion
In this section, we discuss the significant factors of NOx emission identified with LiNGAM: the combustion air temperature (CAT), the steam temperature near the walls of the furnace (STR and STL), the forced draft fan-air flow rate (FDF-AFR), and the steam flow rate (SFR) and the furnace pressure (FP).
4.1 Combustion air temperature
The positive causal effect of CAT estimated with LiNGAM is supported by a previous research which showed that thermal NOx strongly depends on the combustion temperature. The Extended Zeldovich mechanism, analyzed by Bowman and Seery (Fenimore and Jones. (1957)), describes the generation process of thermal NOx as follows:
In a conventional combustion of pulverized coal, fuel NOx and thermal NOx contribute to the majority of the total NOx emissions, while prompt NOx makes negligible contribution (Choi and Kim. (2009); Zhao et al. (2017); Ma et al. (2019)). Since cracking of stable N2-binding shown in the first reaction in Eq. 5 requires high activation energy (Hill and Douglas Smoot. (2000)), most of the thermal NOx is generated in the areas around flames from the burners. Accordingly, NOx emissions exponentially decrease as the flame temperature decreases. Hence, CAT affects NOx emissions, which is consistent with our analysis.
4.2 Steam temperatures near walls of furnace
Our results also showed that steam temperature on a specific side of the furnace wall (STR and STL) was another factor in the NOx generation. Previous researchers have indicated that the furnace design, such as the angles of the OFA nozzles and the burner nozzles, affect the characteristics of the combustion and NOx emission, on the basis of computational fluid dynamics (CFD) simulations (Wang et al. (2018); Choi and Kim. (2009); Li et al. (2009)). When the burner nozzle angle increases, the temperature distribution and the turbulent mixing in the furnace change significantly, which then alters NOx emissions (Tan et al. (2017)). Thus, the furnace design may cause uneven combustion and differences in STR and STL, thereby affecting NOx generation.
4.3 Air flow rate of forced draft fan
The combustion air fed from the forced draft fan (FDF) is divided into secondary air and over fire air (OFA) related to the Fuel NOx. Fuel NOx is generated from the nitrogen bound in the fuel through either the homogenous gas phase reaction or the heterogeneous reaction on the coal char surface as follows (Miller and Bowman. (1989); Molina et al. (2000)).
The N2-bounding is cracked during the devolatilization process, and a portion of the nitrogen is rapidly converted to HCN, while the remaining fuel nitrogen forms NH3. These two reactions forming either NO or N2 depend on the local conditions of the fuel. In fuel-rich regions, HCN and NH3 will typically be reduced to N2 as shown in Eqs. 8–9, while they are oxidized to form NO in fuel-lean regions as shown in Eqs. 6–7 (Eaton et al. (1999)).
Air staging with OFA makes a fuel-rich zone near the burners by supplying the combustion air into the secondary burnout zone. The increase in FDF air flow rate may form a hypoxic environment near the burners in the primary combustion zone and allow most of the coal nitrogen to be released into a fuel-rich region. This mechanism suggests a rapid formation of N2 from NOx. Thus, it is concluded that FDF-AFR has causality with NOx emission. Previous research has shown that the rate of OFA has a significant impact on NOx emissions (Wang et al. (2018)), which is consistent with the results of this study.
4.4 Steam flow rate and furnace pressure
The discussion above indicates the feasibility of causal analysis using LiNGAM in NOx emissions in a coal-fired power plant, since the identified factors were consistent with previous studies.
However, SFR and FP have not been mentioned in previous research, although they had the first and second largest effects on NOx emissions, as shown in Figure 3. FP is the pressure inside the furnace during coal combustion, and there exists a physical causal relationship with it and NOx emission. FP is a factor of NOx emissions newly identified in this study, which should be confirmed through experiments and additional data, to thereby generate a new hypothesis that explains how FP affects NOx generation.
4.5 Limitation
Limitations of this work include the operation data; the data did not contain properties of the coals that were used and their mixtures. Thus, the effects of the coals that were used on NOx emission was taken into account in this study. We need to collect data on the utilized coal for further analysis.
5 Conclusion
This study analyzed real operation data collected from a coal-fired power plant to identify the factors of NOx emissions with LiNGAM. As a result, some of the known NOx emission factors were correctly identified by LiNGAM, while new and spurious factors, the steam flow rate, and the furnace pressure were also estimated.
In future works, we will confirm whether unknown factors also affect NOx emissions, and discuss the challenges of applying causal discovery algorithms to estimate causal relationships in the context of complex industrial processes.
Data availability statement
Due to commercial restrictions of the operation data of the coal-fired power plant, the authors do not agree for their data to be shared publicly, so supporting data is not readily available. Requests to access the datasets should be directed to Koichi Fujiwara, fujiwara.koichi@hps.material.nagoya-u.ac.jp.
Author contributions
TS and KF analyzed the data and composed the manuscript. All authors contributed to the article and approved the submitted version.
Funding
This study was partially supported by the Kobe Steel Inc. The funder was not involved in the study design, collection, analysis, interpretation of data, the writing of this article, or the decision to submit it for publication.
Conflict of interest
The authors declare that the research was conducted in the absence of any commercial or financial relationships that could be construed as a potential conflict of interest.
Publisher’s note
All claims expressed in this article are solely those of the authors and do not necessarily represent those of their affiliated organizations, or those of the publisher, the editors and the reviewers. Any product that may be evaluated in this article, or claim that may be made by its manufacturer, is not guaranteed or endorsed by the publisher.
References
Adams, D., Oh, D. H., Kim, D. W., Lee, C. H., and Oh, M. (2020). Prediction of sox–nox emission from a coal-fired cfb power plant with machine learning: Plant data learned by deep neural network and least square support vector machine. J. Clean. Prod. 270, 122310. doi:10.1016/j.jclepro.2020.122310
Ampimah, B. C., Sun, M., Han, D., and Wang, X. (2018). Optimizing sheddable and shiftable residential electricity consumption by incentivized peak and off-peak credit function approach. Appl. Energy 210, 1299–1309. doi:10.1016/j.apenergy.2017.07.097
Bielczyk, N. Z., Uithol, S., van Mourik, T., Anderson, P., Glennon, J. C., and Buitelaar, J. K. (2019). Disentangling causal webs in the brain using functional magnetic resonance imaging: A review of current approaches. Netw. Neurosci. 3, 237–273. doi:10.1162/netn_a_00062
Cao, L., Su, J., Wang, Y., Cao, Y., Siang, L. C., Li, J., et al. (2022). Causal discovery based on observational data and process knowledge in industrial processes. Industrial Eng. Chem. Res. 61, 14272–14283. doi:10.1021/acs.iecr.2c01326
Chen, X., Wang, J., and Zhou, J. (2018). Process monitoring based on multivariate causality analysis and probability inference. IEEE Access 6, 6360–6369. doi:10.1109/access.2018.2795535
Chen, Y., Liang, C., Liu, D., Niu, Q., Miao, X., Dong, G., et al. (2023). Embedding-graph-neural-network for transient nox emissions prediction. Energies 16, 3. doi:10.3390/en16010003
Choi, C. R., and Kim, C. N. (2009). Numerical investigation on the flow, combustion and nox emission characteristics in a 500 mwe tangentially fired pulverized-coal boiler. Fuel 88, 1720–1731. doi:10.1016/j.fuel.2009.04.001
Eaton, A., Smoot, L., Hill, S., and Eatough, C. (1999). Components, formulations, solutions, evaluation, and application of comprehensive combustion models. Prog. Energy Combust. Sci. 25, 387–436. doi:10.1016/s0360-1285(99)00008-8
Fenimore, C. P., and Jones, G. W. (1957). The water-catalyzed oxidation of carbon monoxide by oxygen at high temperature. J. Phys. Chem. 61, 651–654. doi:10.1021/j150551a033
Ferkingstad, E., Løland, A., and Wilhelmsen, M. (2011). Causal modeling and inference for electricity markets. Energy Econ. 33, 404–412. doi:10.1016/j.eneco.2010.10.006
Gonzalez-Salazar, M. A., Kirsten, T., and Prchlik, L. (2018). Review of the operational flexibility and emissions of gas-and coal-fired power plants in a future with growing renewables. Renew. Sustain. Energy Rev. 82, 1497–1513. doi:10.1016/j.rser.2017.05.278
Hair, J. F., Ringle, C. M., and Sarstedt, M. (2011). Pls-sem: Indeed a silver bullet. J. Mark. theory Pract. 19, 139–152. doi:10.2753/mtp1069-6679190202
Hill, S., and Douglas Smoot, L. (2000). Modeling of nitrogen oxides formation and destruction in combustion systems. Prog. Energy Combust. Sci. 26, 417–458. doi:10.1016/s0360-1285(00)00011-3
Hu, X., Niu, P., Wang, J., and Zhang, X. (2020). Multi-objective prediction of coal-fired boiler with a deep hybrid neural networks. Atmos. Pollut. Res. 11, 1084–1090. doi:10.1016/j.apr.2020.04.001
Hyvärinen, A., and Smith, S. M. (2013). Pairwise likelihood ratios for estimation of non-Gaussian structural equation models. J. Mach. Learn. Res. JMLR 14, 111–152.
Kadlec, P., Gabrys, B., and Strandt, S. (2009). Data-driven soft sensors in the process industry. Comput. Chem. Eng. 33, 795–814. doi:10.1016/j.compchemeng.2008.12.012
Ke, X., Engblom, M., Yang, H., Brink, A., Lyu, J., Zhang, M., et al. (2022). Prediction and minimization of nox emission in a circulating fluidized bed combustor: A comprehensive mathematical model for cfb combustion. Fuel 309, 122133. doi:10.1016/j.fuel.2021.122133
Lai, P. C., and Bessler, D. A. (2015). Price discovery between carbonated soft drink manufacturers and retailers: A disaggregate analysis with pc and lingam algorithms. J. Appl. Econ. 18, 173–197. doi:10.1016/s1514-0326(15)30008-8
Li, N., and Hu, Y. (2020). The deep convolutional neural network for nox emission prediction of a coal-fired boiler. IEEE Access 8, 85912–85922. doi:10.1109/access.2020.2992451
Li, N., Lv, Y., and Hu, Y. (2023). Prediction of nox emissions from a coal-fired boiler based on convolutional neural networks with a channel attention mechanism. Energies 16, 76. doi:10.3390/en16010076
Li, S., Xu, T., Hui, S., Zhou, Q., and Tan, H. (2009). Optimization of air staging in a 1 mw tangentially fired pulverized coal furnace. Fuel Process. Technol. 90, 99–106. doi:10.1016/j.fuproc.2008.08.005
Liukkonen, M., Heikkinen, M., Hiltunen, T., Hälikkä, E., Kuivalainen, R., and Hiltunen, Y. (2011). Artificial neural networks for analysis of process states in fluidized bed combustion. Energy 36, 339–347. doi:10.1016/j.energy.2010.10.033
Ma, L., Fang, Q., Yin, C., Wang, H., Zhang, C., and Chen, G. (2019). A novel corner-fired boiler system of improved efficiency and coal flexibility and reduced nox emissions. Appl. energy 238, 453–465. doi:10.1016/j.apenergy.2019.01.084
Menegozzo, G., Dall’Alba, D., and Fiorini, P. (2022). “Cipcad-bench: Continuous industrial process datasets for benchmarking causal discovery methods,” in 2022 IEEE 18th International Conference on Automation Science and Engineering (CASE), 20-24 August 2022, Mexico City, Mexico (IEEE). 2124–2131.
Miller, J. A., and Bowman, C. T. (1989). Mechanism and modeling of nitrogen chemistry in combustion. Prog. Energy Combust. Sci. 15, 287–338. doi:10.1016/0360-1285(89)90017-8
Molina, A., Eddings, E., Pershing, D., and Sarofim, A. (2000). Char nitrogen conversion: Implications to emissions from coal-fired utility boilers. Prog. Energy Combust. Sci. 26, 507–531. doi:10.1016/s0360-1285(00)00010-1
Offermans, T., Hendriks, L., Van Kollenburg, G. H., Szymańska, E., Buydens, L. M., and Jansen, J. J. (2021). Improved understanding of industrial process relationships through conditional path modelling with process pls. Front. Anal. Sci. 2021, 721657. doi:10.3389/frans.2021.721657
Saif-Ul-Allah, M. W., Khan, J., Ahmed, F., Salman, C. A., Gillani, Z., Hussain, A., et al. (2022). Computationally inexpensive 1d-cnn for the prediction of noisy data of nox emissions from 500 mw coal-fired power plant. Front. Energy Res. 10, 945769. doi:10.3389/fenrg.2022.945769
Shimizu, S., Hoyer, P. O., Hyvärinen, A., Kerminen, A., and Jordan, M. (2006). A linear non-Gaussian acyclic model for causal discovery. J. Mach. Learn. Res. 7, 2003–2030.
Shimizu, S., Inazumi, T., Sogawa, Y., Hyvarinen, A., Kawahara, Y., Washio, T., et al. (2011). Directlingam: A direct method for learning a linear non-Gaussian structural equation model. J. Mach. Learn. Research-JMLR 12, 1225–1248.
Smith, S. M., Miller, K. L., Salimi-Khorshidi, G., Webster, M., Beckmann, C. F., Nichols, T. E., et al. (2011). Network modelling methods for fmri. Neuroimage 54, 875–891. doi:10.1016/j.neuroimage.2010.08.063
Song, M., Xue, J., Gao, S., Cheng, G., Chen, J., Lu, H., et al. (2022). Prediction of nox concentration at scr inlet based on bmifs-lstm. Atmosphere 13, 686. doi:10.3390/atmos13050686
Strbac, G. (2008). Demand side management: Benefits and challenges. Energy policy 36, 4419–4426. doi:10.1016/j.enpol.2008.09.030
Sun, Y. N., Qin, W., Hu, J. H., Xu, H. W., and Sun, P. Z. (2022). A causal model-inspired automatic feature-selection method for developing data-driven soft sensors in complex industrial processes. Engineering. doi:10.1016/j.eng.2022.06.019
Tan, P., He, B., Zhang, C., Rao, D., Li, S., Fang, Q., et al. (2019). Dynamic modeling of nox emission in a 660 mw coal-fired boiler with long short-term memory. Energy 176, 429–436. doi:10.1016/j.energy.2019.04.020
Tan, P., Tian, D., Fang, Q., Ma, L., Zhang, C., Chen, G., et al. (2017). Effects of burner tilt angle on the combustion and nox emission characteristics of a 700mwe deep-air-staged tangentially pulverized-coal-fired boiler. Fuel 196, 314–324. doi:10.1016/j.fuel.2017.02.009
Tuttle, J. F., Blackburn, L. D., Andersson, K., and Powell, K. M. (2021). A systematic comparison of machine learning methods for modeling of dynamic processes applied to combustion emission rate modeling. Appl. Energy 292, 116886. doi:10.1016/j.apenergy.2021.116886
Uchida, Y., Fujiwara, K., Saito, T., and Osaka, T. (2022). Causal plot: Causal-based fault diagnosis method based on causal analysis. Processes 10, 2269. doi:10.3390/pr10112269
van Kollenburg, G., Bouman, R., Offermans, T., Gerretzen, J., Buydens, L., van Manen, H. J., et al. (2021). Process pls: Incorporating substantive knowledge into the predictive modelling of multiblock, multistep, multidimensional and multicollinear process data. Comput. Chem. Eng. 154, 107466. doi:10.1016/j.compchemeng.2021.107466
van Kollenburg, G. H., van Es, J., Gerretzen, J., Lanters, H., Bouman, R., Koelewijn, W., et al. (2020). Understanding chemical production processes by using pls path model parameters as soft sensors. Comput. Chem. Eng. 139, 106841. doi:10.1016/j.compchemeng.2020.106841
Vo, N. D., Oh, D. H., Hong, S. H., Oh, M., and Lee, C. H. (2019). Combined approach using mathematical modelling and artificial neural network for chemical industries: Steam methane reformer. Appl. Energy 255, 113809. doi:10.1016/j.apenergy.2019.113809
Wan, A., Yang, J., Chen, T., Jinxing, Y., Li, K., and Qinglong, Z. (2022). Dynamic pollution emission prediction method of a combined heat and power system based on the hybrid cnn-lstm model and attention mechanism. Environ. Sci. Pollut. Res. 29, 69918–69931. doi:10.1007/s11356-022-20718-9
Wang, C., Liu, Y., Zheng, S., and Jiang, A. (2018a). Optimizing combustion of coal fired boilers for reducing nox emission using Gaussian process. Energy 153, 149–158. doi:10.1016/j.energy.2018.01.003
Wang, Q., Chen, Z., Wang, J., Zeng, L., Zhang, X., Li, X., et al. (2018b). Effects of secondary air distribution in primary combustion zone on combustion and NO emissions of a large-scale down-fired boiler with air staging. Energy 165, 399–410. doi:10.1016/j.energy.2018.09.194
Wang, X., Liu, W., Wang, Y., and Yang, G. (2022). A hybrid nox emission prediction model based on ceemdan and am-lstm. Fuel 310, 122486. doi:10.1016/j.fuel.2021.122486
Xie, P., Gao, M., Zhang, H., Niu, Y., and Wang, X. (2020). Dynamic modeling for nox emission sequence prediction of scr system outlet based on sequence to sequence long short-term memory network. Energy 190, 116482. doi:10.1016/j.energy.2019.116482
Yang, G., Wang, Y., and Li, X. (2020). Prediction of the NO emissions from thermal power plant using long-short term memory neural network. Energy 192, 116597. doi:10.1016/j.energy.2019.116597
Zhang, D. (2013). Ultra-supercritical coal power plants: Materials, technologies and optimisation. Sawston: Woodhead Publishing Limited.
Keywords: causal analysis, coal-fired power plant, lingam, NOx emission, data-driven approach
Citation: Saito T and Fujiwara K (2023) Causal analysis of nitrogen oxides emissions process in coal-fired power plant with LiNGAM. Front. Anal. Sci. 3:1045324. doi: 10.3389/frans.2023.1045324
Received: 15 September 2022; Accepted: 23 January 2023;
Published: 16 February 2023.
Edited by:
Federico Marini, Sapienza University of Rome, ItalyReviewed by:
Faisal Ahmed, COMSATS University Islamabad, Lahore Campus, PakistanKeval Nikam, Dr D Y Patil Institute of Engineering Management and Research Akurdi Pune, India
Copyright © 2023 Saito and Fujiwara. This is an open-access article distributed under the terms of the Creative Commons Attribution License (CC BY). The use, distribution or reproduction in other forums is permitted, provided the original author(s) and the copyright owner(s) are credited and that the original publication in this journal is cited, in accordance with accepted academic practice. No use, distribution or reproduction is permitted which does not comply with these terms.
*Correspondence: Koichi Fujiwara, ZnVqaXdhcmEua29pY2hpQGhwcy5tYXRlcmlhbC5uYWdveWEtdS5hYy5qcA==