- 1Forensic Chemistry and Materials Lab, Applied Bioscience Graduate Program, Ontario Tech University, Oshawa, ON, Canada
- 2Wildlife and Applied Genomics Lab, Department of Forensic Science, Trent University, Peterborough, ON, Canada
- 3Wildlife and Applied Genomics Lab, Environmental and Life Sciences Graduate Program, Trent University, Peterborough, ON, Canada
- 4Forensic Chemistry and Materials Lab, Faculty of Science, Forensic Science, Ontario Tech University, Oshawa, ON, Canada
Estimating the time since deposition (TSD) of a bloodstain can provide important medico-legal information for crime scene investigation. Research in this area primarily investigates the degradation of either hemoglobin or genetic material over time. In this work, we present a comprehensive meta-analysis on bloodstain TSD research. Our results are interpreted from 25 quantitative studies used to probe the effect of biomolecule studied, analytical technique used, substrate porosity, environmental conditions, and blood source on TSD estimates. There was an overall strong effect of time across studies (Fisher’s Zr = 1.66, r = 0.93), and generally, we found that the type of biomolecule studied (e.g., hemoglobin, DNA) had equal effect sizes for TSD estimation. Differences in the mean TSD effect size were also observed between substrate porosity. Interestingly, the blood source does not significantly influence the magnitude of the effect sizes in TSD estimation. Despite the clear effect of time, forensically relevant prediction of bloodstain TSD remains complicated by inter-donor variability, type of substrate and environmental conditions. We recommend that future bloodstain TSD research increase sample size, include summary statistics and standardize experimental methodologies so that we can develop a quantitative understanding of the physicochemical processes involved in whole blood degradation in ex vivo conditions.
Introduction
Crime scene investigators are tasked with determining the events of a crime. This normally consists of answering forensically relevant questions like who, what, where, how, and, when, regarding the observed and collected evidence at the crime scene. Biological material such as tissue and bodily fluids left at a crime scene can assist in answering these questions. A commonly encountered type of biological evidence at a crime scene is blood (Box 1). As blood dries, it forms bloodstains with a variety of morphologies largely depending on formation mechanics and target surface properties (Adam, 2012). Source identification of a bloodstain is conducted through DNA profiling (Butler, 2006; Verdon et al., 2011, 2013) while bloodstain pattern analysis (BPA) provides potential interpretations of the physical events that created the bloodshed (Adam, 2012).
Both DNA profiling and BPA are important to an investigation, yet they do not provide an estimate of when the crime occurred. Determining the amount of time that has elapsed since a bloodstain was deposited, or time since deposition (TSD), could provide important context to investigators. This is especially useful in cases where blood is the only biological evidence present; a timeline could be established and used to corroborate physical evidence, witness testimonies and the suspect alibis (Bremmer et al., 2012; Zadora and Menzyk, 2018). Alternatively, TSD can be used to determine whether a bloodstain is relevant to the current investigation (Zadora and Menzyk, 2018). Bloodstain TSD is typically estimated by modelling the physicochemical degradation of blood biomolecules over time; experimental methodologies generating spectroscopic (Hanson and Ballantyne, 2010; Doty et al., 2016; Zhang et al., 2017), genetic (Anderson et al., 2011; Fang et al., 2019; Salzmann et al., 2021b) and photographic (Thanakiatkrai et al., 2013; Shin et al., 2017) metrics have been developed and used to estimate bloodstain TSD. Factors like individual variation, type of substrate and environmental conditions influence the timewise degradation of bloodstains and their biomolecules, resulting in large variability in the accuracy and precision of TSD estimation models (Zadora and Menzyk, 2018; Weber and Lednev, 2020; Menżyk, 2021).
BOX 1
Blood is a non-Newtonian fluid responsible for the maintenance of homeostasis in vivo by providing transport of oxygen, carbon dioxide, minerals, and electrolytes, among others, to and from every part of the human body. Plasma, which makes up approximately 55% of whole blood, is mostly water but does contain some proteins, minerals, and electrolytes while the cellular components, which make up the remaining 45% of whole blood, include erythrocytes (red blood cells; RBC), leukocytes (white blood cells; WBC) and thrombocytes (platelets) (Bremmer et al., 2012). The cellular components and plasma proteins perform specific physiological functions in vivo that are disrupted when the blood leaves the body (ex vivo).
Hemoglobin (Hb), an important protein in RBCs that is responsible for the transport of oxygen in the body, consists of two α and two β chains (Zadora and Menzyk, 2018). Each subunit or chain contains a heme group, with a centralized iron atom in a protoporphyrin ring, directly capable of reversibly binding oxygen (Bergmann et al., 2021). Oxygen-bound hemoglobin is referred to as oxyhemoglobin (oxyHb) while Hb without oxygen is referred to as deoxyhemoglobin (deoxyHb) (Bergmann et al., 2021). In vivo, every day roughly 3% of oxyHb is autoxidized to methemoglobin (metHb), a derivative incapable of binding and carrying oxygen (Bonaventura et al., 2013; Zadora and Menzyk, 2018). In deoxyHb, iron is present in its ferrous state (Fe2+); upon oxidation to oxyHb, the iron is in either its ferrous or ferric state (Fe3+) (Yamamoto and Palmer, 1973; Spiro and Strekas, 1974; Kitagawa, 1987; Ferraro et al., 2003; Nagatomo et al., 2011). This differs from the autoxidized metHb in which iron is solely present in its ferric state (Doty et al., 2016; Zadora and Menzyk, 2018; Bergmann et al., 2021). In vivo, internal reduction mechanisms including enzymes glutathione peroxidase and methemoglobin reductase are responsible for maintaining low levels of metHb by converting Hb to its healthy derivative (Bonaventura et al., 2013; Zadora and Menzyk, 2018). Ex vivo, when blood is deposited, the combination of environmental stressors and loss of the internal reduction mechanisms lead to accelerated oxidative changes in Hb derivatives (Bonaventura et al., 2013; Doty et al., 2016; Zadora and Menzyk, 2018). Continued degradation creates further conformational changes to the structure of Hb, leading to the formation of hemichromes (HCs) (Bergmann et al., 2021). The iron in this Hb derivative binds a distal histidine residue rather than oxygen or water (Doty et al., 2016; Zadora and Menzyk, 2018). This ex vivo degradation pathway has been of great interest to bloodstain TSD research due to the continuous structural and redox changes in the Hb (Weber and Lednev, 2020).
Other important molecules in blood include DNA and RNA present in WBCs; DNA is double-stranded while RNA is single-stranded and can form extensive secondary and tertiary structures (Nowakowski and Tinoco, 1997; Fordyce et al., 2013). RNA encompasses larger ribosomal RNA and the shorter messenger RNA (mRNA) and micro RNA (miRNA), among others (Wu et al., 2014). Ex vivo, DNA and RNA are both degraded similarly; enzymes cleave their phosphodiester bonds most effectively in hydrated environments (Fordyce et al., 2013), which occurs before desiccation of the bloodstain. Reactive oxygen species, alkylating agents and UV irradiation damage the nucleotides and can induce crosslinking, pyrimidine dimers and chain breaks (Wurtmann and Wolin, 2009; Fordyce et al., 2013; Rahi et al., 2021). As a result, base-pairing is disrupted, leading to fragmentation and unstable molecules. However, shorter RNAs like mRNA and miRNA have been shown to persist for long periods in desiccated environments (Fordyce et al., 2013; Salzmann et al., 2021b), suggesting that the degradation means suggested above are biased towards larger fragments of nucleic acid. Although RNA might provide insight for long-term studies and complement the short-term degradation of hemoglobin, they have yet to be combined to estimate bloodstain TSD.
Commonly analyzed biomolecules for TSD estimation models include hemoglobin (Hb) from red blood cells (RBCs) and genetic material from white blood cells (WBCs). Spectroscopic techniques, such as Raman (Premasiri et al., 2012; Doty et al., 2016, 2017; Sun et al., 2017b; Takamura et al., 2019; Menżyk et al., 2020), infrared (Botoniic-Sehic et al., 2009; Lin et al., 2017; Zhang et al., 2017; Hassan et al., 2019), reflectance (Bremmer et al., 2011a, 2011b; Li et al., 2011; Edelman et al., 2012a, 2016; Sun et al., 2017a) and UV-Vis absorbance (Hanson and Ballantyne, 2010; Hanson et al., 2011; Agudelo et al., 2015; Bergmann et al., 2017, 2021; Kaur et al., 2020; Stotesbury et al., 2020; Cossette et al., 2021) spectroscopies have all probed the timewise degradation of Hb. Photography (Thanakiatkrai et al., 2013; Shin et al., 2017; Choi et al., 2019) and hyperspectral imaging (Edelman et al., 2012b; Li et al., 2013; Majda et al., 2018) have measured the colour changes in blood while electron spin resonance and electrochemistry analyzed the conformational changes of the heme groups (Miki et al., 1987; Matsuoka et al., 1995; Fujita et al., 2005). Atomic force microscopy has investigated the changes in RBC physiology over time (Strasser et al., 2007; Wu et al., 2009; Cavalcanti and Silva, 2019) while mass-spectrometry (Seok et al., 2018; Rankin-Turner et al., 2019), chromatography (Inoue et al., 1991, 1992; Andrasko, 1997; Arany and Ohtani, 2011; Acar et al., 2020) and fluorescence spectroscopy (Guo et al., 2013; Mc Shine et al., 2017; Weber et al., 2021) have measured changes in metabolites and protein composition of deposited bloodstains. DNA (Kohlmeier and Schneider, 2012; Sirker et al., 2013, 2016; Rahikainen et al., 2016; Stotesbury et al., 2020; Cossette et al., 2021), total RNA, mRNA and miRNA (Bauer et al., 2003; Anderson et al., 2005, 2011; Simard et al., 2012; Qi et al., 2013; Alshehhi et al., 2017; Fang et al., 2019; Fu and Allen, 2019; Mayes et al., 2019; Alshehhi and Haddrill, 2020; Asaghiar and Williams, 2020; Salzmann et al., 2021b; Heneghan et al., 2021; Li et al., 2021; Manasatienjig and Nimnual, 2021; Zhao et al., 2021) degradation have also been targeted in longer time series. Recently, microbial profiling of bloodstains has described the timewise changes in microbial communities inhabiting the bloodstains (Salzmann et al., 2019; 2021a). Most of these works are summarized in greater depth in the recent reviews by Zadora and Menzyk and Weber and Lednev (Zadora and Menzyk, 2018; Weber and Lednev, 2020). Interestingly, some studies have attempted to determine the time of day of deposition by evaluating circadian biomarkers (Ackermann et al., 2010; Lech et al., 2016, 2018) and the age of the donor by examining changes in methylation (Zbieć-Piekarska et al., 2015; Thong et al., 2017) or signal joint T-cell receptor rearrangement excision circles (Ou et al., 2012; Yamanoi et al., 2018).
The type of substrate on which the bloodstain is deposited also influences TSD estimates due to differing degradation pathway kinetics (Zadora and Menzyk, 2018). The rate of biomolecule degradation in bloodstains is partially dictated by substrate porosity; for example, hemoglobin oxidation is a faster process in dried states than in liquid states (Marrone and Ballantyne, 2009). Substrates range from common materials like glass, plastic and textiles to less common materials like soil (Hassan et al., 2019), wallpaper (Shin et al., 2017), leather (Kohlmeier and Schneider, 2012) and brick stones (Wang et al., 2019). The same applies to environmental conditions; warmer environments catalyze biomolecular degradation while colder environments preserve the biomolecules in their native states, slowing the degradation (Zadora and Menzyk, 2018). A variety of environmental conditions have been studied in bloodstain TSD research; this includes exposure to outdoor environments (Lin et al., 2017; Salzmann et al., 2021a, 2021b; Li et al., 2021; Zhao et al., 2021), varying temperatures (−20–61°C) and a wide range of humidity values (10–99% RH) (Hanson and Ballantyne, 2010; Mayes et al., 2019; Cossette et al., 2021; Heneghan et al., 2021; Li et al., 2021; Zhao et al., 2021). Statistical analyses also differ between studies; regressions are common, but the type of fit varies (linear (Fu and Allen, 2019), polynomial (Cavalcanti and Silva, 2019), logarithmic (Sun et al., 2017b), exponential (Gautam et al., 2020), multivariate (Cossette et al., 2021)) depending on the observed time-series trends. A number of studies used dimensionality-reduction tools for their analyses (Sun et al., 2017a; Doty et al., 2017) while others incorporated cross-validation to obtain prediction accuracies (Bremmer et al., 2011b; Edelman et al., 2012b). The inter-study variability in experimental conditions and statistical analyses raises two fundamental questions: how do we interpret all this research together and what is the best way forward for bloodstain TSD research?
We present a meta-analysis that examines the association between the degradation of biomolecules and cellular elements found within blood and its TSD. The primary goals here are to determine the strength of these relationships and whether certain analytical techniques and biomolecules provide more robust blood TSD estimates. Specifically, we 1) determined the mean effect size–a quantitative value measuring the strength of a relationship–of bloodstain TSD studies, 2) explored how effect sizes varied by class of biomolecule, analytical technique, substrate porosity, blood source, temperature, and type of summary statistic, and, 3) searched and analyzed compiled data for a publication bias. By using a meta-analytic approach, we synthesized quantitative data on blood TSD estimates, complementing recent qualitative reviews (Bremmer et al., 2012; Zadora and Menzyk, 2018; Weber and Lednev, 2020). The combination of quantitative and qualitative data provides a holistic overview and way forward for bloodstain TSD research and ultimately, crime scene implementation.
Materials and methods
This meta-analysis was conducted using the Preferred Reporting Items for Systematic Reviews and Meta-Analyses (PRISMA) guidelines for reporting articles in systematic reviews and meta-analyses (Page et al., 2021b). The process for this reporting system is normally reflected in a step-by-step flowchart indicating the number of articles that were screened for inclusion in the meta-analysis.
Literature search and strategy
Literature searches were conducted in two databases: Web of Science and Scopus. The searches included full-text articles, summaries, and abstracts. Searches were conducted in English and did not include a restriction for the year of publication. Following the searches, which were completed 12 May 2021, databases were searched using a combination of the following keywords: “DNA,” “RNA,” “time since deposition,” “bloodstains,” “hemoglobin,” “taphonomy,” “blood,” “degradation,” “forensic,” “spectroscopy,” “metabolites,” “proteins,” “plasma proteins,” “aging,” “volatile organic compounds,” “transcriptome,” “methylation.” The combination of keywords was done through the Boolean operator “AND” and resulted in 26 searches. The number of hits for each search is found in Supplementary Table S1. Hits were imported into EndNote citation manager for eligibility assessment.
Eligibility criteria and data collection
Articles were first screened by title, followed by abstracts and finally full text. Articles selected were peer-reviewed English publications quantitatively examining the TSD of blood. The following articles were excluded: 1) commentaries, editorials, reviews, opinions; 2) articles examining the TSD of other biological fluids, 3) articles providing a qualitative assessment of blood TSD in which there were no convertible summary statistics (r, R2, F), 4) articles in which blood was not deposited on a substrate, 5) articles not in English; and 6) articles where the full text was not available. Data from retained articles were extracted and inserted into a summary table. Data collected, if reported, included summary statistics (r, R2, F, t, p-value), the number of biological replicates, the class of biomolecule examined (whole blood and cellular elements, genetic material, proteins), the analytical technique used to analyze the timewise trends, blood source (e.g., human, bovine), the temperature, substrate porosity, length of time series, and author affiliation (country).
Three-level meta-analysis
All statistical analyses were conducted in R version 4.0.3. The R script and datasets for this study are publicly available and deposited at “https://gitlab.com/f4301/TSD.git”. Reported summary statistics from each article were first converted to the correlation coefficient r if not provided in the texts and used to calculate effect size (Fisher’s
and
where
where
In some scenarios, values above 1 were obtained for the correlation coefficient r; effect sizes could not be computed in those instances. For studies stating that the p-values were non-significant without reporting the numerical value, they were assigned a conservative value of 0.5 (Shafer and Wolf, 2013).
Effect sizes (Fisher’s Zr) can range from −5 to 5, corresponding to correlation coefficients r of −0.9999 and 0.9999, respectively. Effect sizes closest to 0 demonstrated no correlation with time, while those furthest away in both directions represented stronger relationships (e.g. Fisher’s Zr = 0.5 (r = 0.46), Fisher’s Zr = 1.5 (r = 0.91)). We computed effect sizes using the absolute values of the reported summary statistic as we were interested in the magnitude and strength of the observed relationship rather than the directionality of the trend (we note that 98% were positive).
A three-level meta-analytic model was conducted using the R package metafor (Viechtbauer, 2010). Levels 1, 2 and 3 represent the individuals, within-study variances, and between-study variances, respectively (Cheung, 2014). Most articles included in this meta-analysis provided multiple summary statistics; a three-level model allowed for the computation of an overall mean effect size while accounting for between-study and within-study heterogeneity. We reported the overall effect size of the three-level meta-analytic model and the Higgins and Thompson’s I2, the amount of variation that is not attributed to sampling error, for between-study and within-study heterogeneity variances (Higgins and Thompson, 2002). An ANOVA was computed to compare two and three-level models to ensure that the model selected provided the best fit for the data. To investigate a potential publication bias, effect sizes were plotted against sample size. Egger’s regression was employed where an intercept differing from 0 in this test indicates asymmetry and possible publication bias (Egger et al., 1997).
Subgroup analyses
Subgroup analyses were conducted to assess differences in the effect sizes of the different classes of biomolecules, analytical techniques, substrate porosity, blood source, temperature and type of summary statistic reported. This was completed using three-level mixed-effects models specifying the subgroup to be evaluated (Harrer et al., 2021). Unless indicated otherwise in the studies, ambient temperature was regarded as room temperature (18–25°C). Effect sizes and confidence intervals for each subgroup were plotted for visual comparisons; F statistics and p-values were recorded.
Sample size allowed us to divide our dataset by substrate porosity and construct linear mixed-effects models using data from a single porosity to explore potential drivers of Zr: we regressed Zr values against a combination of quantitative variables (e.g., blood source, temperature, and class of biomolecule) which served as fixed effects and individual studies were included as a random effect.
Results
We collected data from 33 articles where summary statistics between biomolecule degradation and TSD of bloodstains were reported; however, effect sizes were only computed from 25 articles due to insufficient sample size in eight of the retained articles (Figure 1 and Supplementary Table S2). A sample size below four cannot produce the required standard error based on Eq. 2. Most studies reported more than one summary statistic; the 25 articles reported 109 effect sizes (Fisher’s Zr) with a mean sample size of 9.7. R2 was the most reported summary statistic, accounting for 70 of the 109, followed by correlation coefficients with 21 and p-values with 18. The mean effect size (Fisher’s Zr) of our three-level meta-analytic model was 1.66 (r = 0.93, 95% CI: 0.88–0.96, p-value <0.001; Figure 2); crudely, this means that 86% of the variation is explained by time. The estimated between-study variance was 0.74 (I2 = 74.8%), while the estimated within-study variance was 0.15 (I2 = 14.9%). We noted that the three-level model provided a better fit than the two-level model which did not account for clustering (χ2 = 63.04, p-value < 0.001, three-level: AIC = 208.4, BIC = 216.4; two-level: AIC = 269.4, BIC = 274.8).
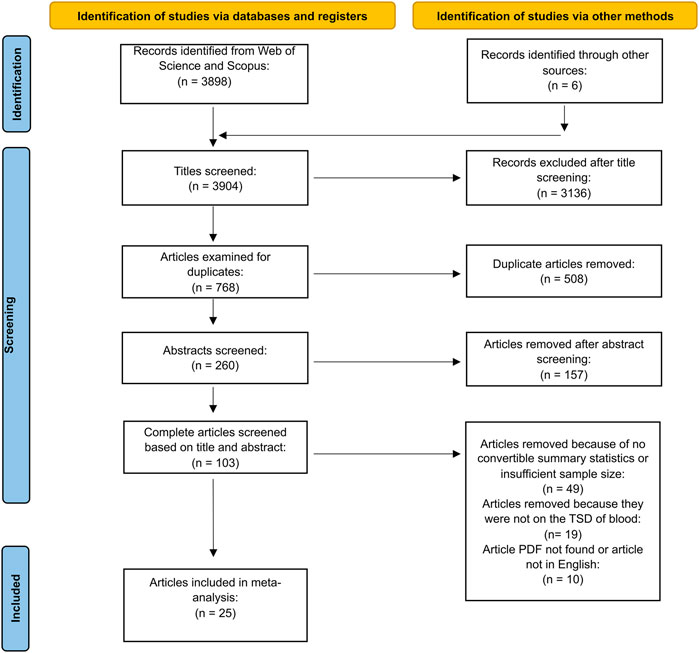
FIGURE 1. Article inclusion based on the PRISMA flow diagram for new systematic reviews which included searches of databases, registers, and other sources.
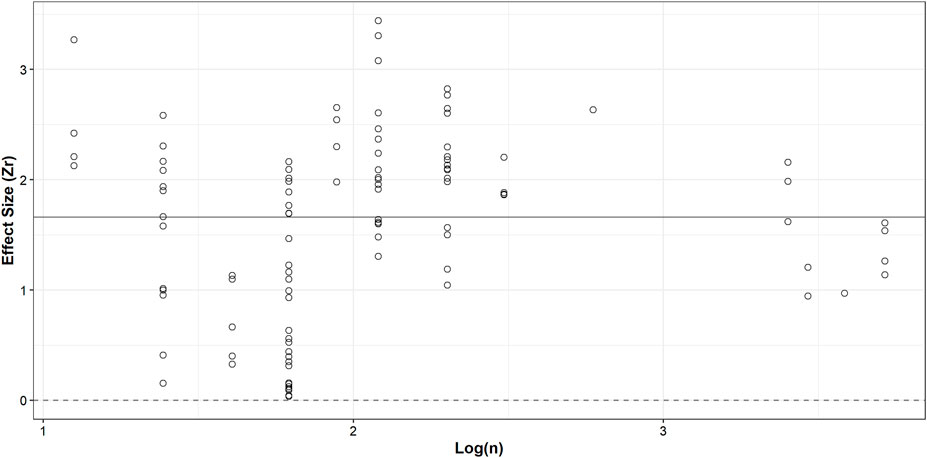
FIGURE 2. Effect sizes (Zr) plotted against log-transformed (natural logarithm) sample size, representing the number of biological replicates. The solid line at 1.66 indicates the overall mean effect size. The dashed line at 0 indicates an effect size of zero (no relationship between biomolecule degradation and TSD of bloodstains).
A total of 107 effect sizes were divided into three subgroups consisting of proteins, genetic material and whole blood and cellular elements. Whole blood and cellular elements had the highest effect size and correlation with TSD, followed by genetic material and proteins (Figure 3A and Supplementary Table S3), but no significant differences were found between subgroups (F = 0.35, p-value = 0.71). Although whole blood and cellular elements had the largest effect size, it also demonstrated the largest standard error, likely arising because of the small sample size (Figure 3A and Supplementary Table S3). For analytical techniques, 109 effect sizes were divided into 14 subgroups; mean effect sizes varied between techniques but no differences were found (F = 1.33, p-value = 0.21, Figures 3B–D). Most techniques exhibited broad confidence intervals because of mean effect size computation using a small number of effect sizes (Supplementary Table S3).
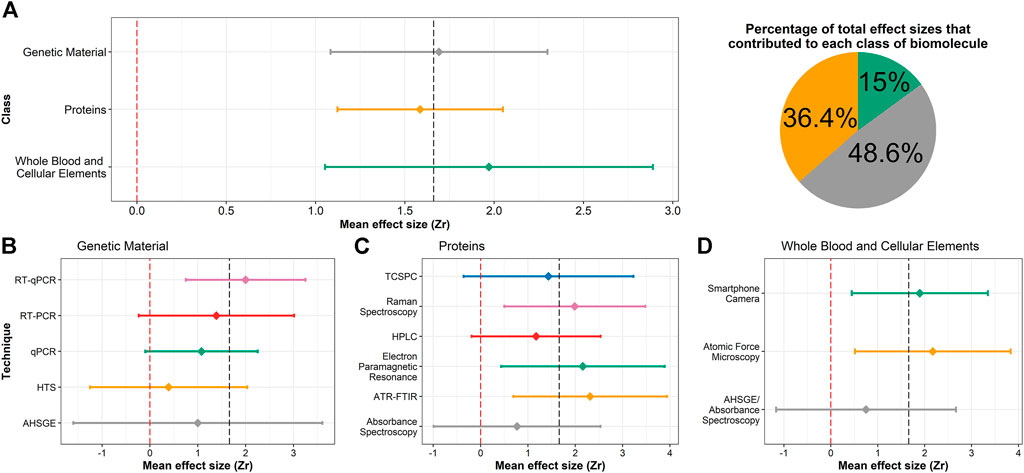
FIGURE 3. Forest plots of bloodstain TSD effect sizes for (A) class of biomolecules; (B–D) analytical techniques used in the 25 retained studies, separated by the biomolecule investigated. Diamonds indicate the mean effect size of that biomolecule or technique and the horizontal lines represent the 95% confidence interval. The red and grey dashed lines represent an effect size of 0 and the overall mean effect size of the meta-analysis, respectively. Note that the x-axis is scaled differently for (A) than (B–D). The pie chart is divided according to the number of summary statistics that contributed to each class of biomolecule; colours match those used in (A).
Effect sizes were divided into porous and non-porous substrates (Supplementary Table S3). 71% of time-series experiments were conducted using porous substrates (Figure 4A) and the effect sizes differed from non-porous substrates (F = 22.37, p-value <0.001), with the latter demonstrating a greater mean effect size (Figure 4A). Blood sources were divided into six subgroups; 84% of statistics were computed from studies using human blood (Supplementary Table S3 and Figure 4B) and no difference was found between subgroups (F = 1.15, p-value = 0.34). Temperature was divided into four subgroups (Supplementary Table S3 and Figure 4C) and no differences were observed (F = 0.45, p-value = 0.72). Nearly 90% of experiments were conducted at room temperature (18–25°C). Timepoints sampled in the retained studies varied, with most studies analyzing blood after one and 2 weeks of deposition (Supplementary Figure S1). Studies that analyzed genetic material typically had longer time series than protein-based approaches (Supplementary Figure S1).
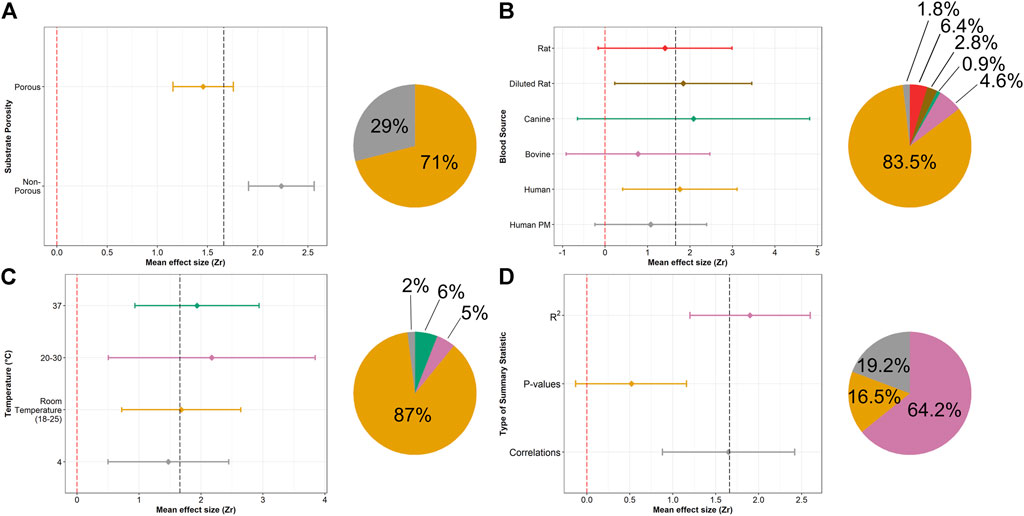
FIGURE 4. Forest plot of bloodstain TSD mean effect sizes, divided by: (A) substrate porosity, (B) blood source, (C) temperature and (D) summary statistics. Piecharts are divided according to the number of effect sizes in each subgroup. Colours from the piecharts correspond to those in the forest plots. NOTE: the scale for the x-axis differs for each subgroup.
We observed minimal overlap between experimental conditions for porous and non-porous substrates, especially for the analytical techniques (Supplementary Table S4). Mixed models aimed at understanding variation in porosity Zr values showed the fixed effects for non-porous Zr often displayed significant slopes compared to porous models. Of note, conditional R2 values were noticeably higher—approximately 35% – than the marginal values, suggesting high interstudy variation (Supplementary Table S5).
Egger’s regression test produced an intercept of 2.51 (95% CI: 2.43–2.58), which was significantly different than 0 and indicative of asymmetry in our data (t = −5.74, p-value <0.001), a finding consistent with the presence of a publication bias. A significant difference in reported summary statistics was found between subgroups (F = 7.65, p-value < 0.001). Even with the conservative approach taken to calculate effect sizes, R2 had the greatest mean effect size of the three subgroups and was reported 64% of the time (Supplementary Table S3 and Figure 4D). p-values had the lowest mean effect size and the entire 95% confidence interval fell below the overall mean effect size (1.66).
Discussion
Our meta-analysis is the first quantitative and interdisciplinary review of bloodstain TSD and revealed that there is a strong relationship between protein, genetic material, and cellular degradation in bloodstains and its TSD (Fishers Zr = 1.66, r = 0.93). We also demonstrated that blood deposited on non-porous substrates displayed greater relationships between degradation and TSD than their porous counterparts.
Subgroup analyses
While this indicates bloodstain ageing has clear, measurable, and interpretable degradation patterns, there was a high degree of variation among studies–this is reflected by the random effect of study explaining an additional 35% on average (Supplementary Table S5). Likewise, the within-study individual variation of TSD signals can be strikingly high (Cossette et al., 2021). This leads to the TSD paradox: the overall effect time has on blood is large, but the predictive power of TSD models is small. Our meta-analysis suggests near uniformity in effect sizes related to the class of biomolecule studied, analytical technique used to measure degradation, the temperature the bloodstain was aged in, and the type of blood source used. While differences exist in the molecular properties of mammalian blood sources (Windberger et al., 2003; Kim et al., 2016; Kapusta et al., 2017; Windberger, 2019; Sparer et al., 2020; Ecker et al., 2021; Farré et al., 2021), the findings of our meta-analysis suggest that they share similar timewise physicochemical degradation pathways of the biomolecules. This leads to a first key takeaway: most readily available mammal blood sources can be used as a human blood substitute in proof-of-concept TSD studies. Moreover, addition of anticoagulants allows for important experimental flexibility, mirrors human properties (Sparer et al., 2020; Orr et al., 2021) and in at least one TSD study, had minimal effect on the recorded metrics ((Elliott et al., 2022) but see (Bergmann et al., 2021)). While a variety of suitable mammal alternatives exist (Windberger et al., 2003; Ecker et al., 2021; Orr et al., 2021) and would facilitate expanded TSD research, we still recommend that for integration into forensic casework, experimentation with human blood will be required for validation.
Another noteworthy pattern is that both biomolecule and analytical techniques showed robust effect sizes, suggesting a holistic TSD approach incorporating multiple metrics is likely warranted, and in some cases, will improve model fit (Stotesbury et al., 2020), which leads to some important statistical recommendations discussed below (Table 1). The degradation of hemoglobin displayed strong timewise trends within the first weeks of deposition (Bergmann et al., 2021); the slowed rate of change once hemichromes formed limits the long-term utility of hemoglobin for bloodstain TSD estimates. mRNA and miRNA degradation appeared to be a slower process; both persisted for a long time after deposition, sometimes up to 1 year (Weinbrecht et al., 2017; Fang et al., 2019; Salzmann et al., 2021b). A combination of spectroscopic and genomic techniques, probing hemoglobin, and nucleic acid degradation, respectively, could provide key information for bloodstain TSD research and should be combined moving forward.
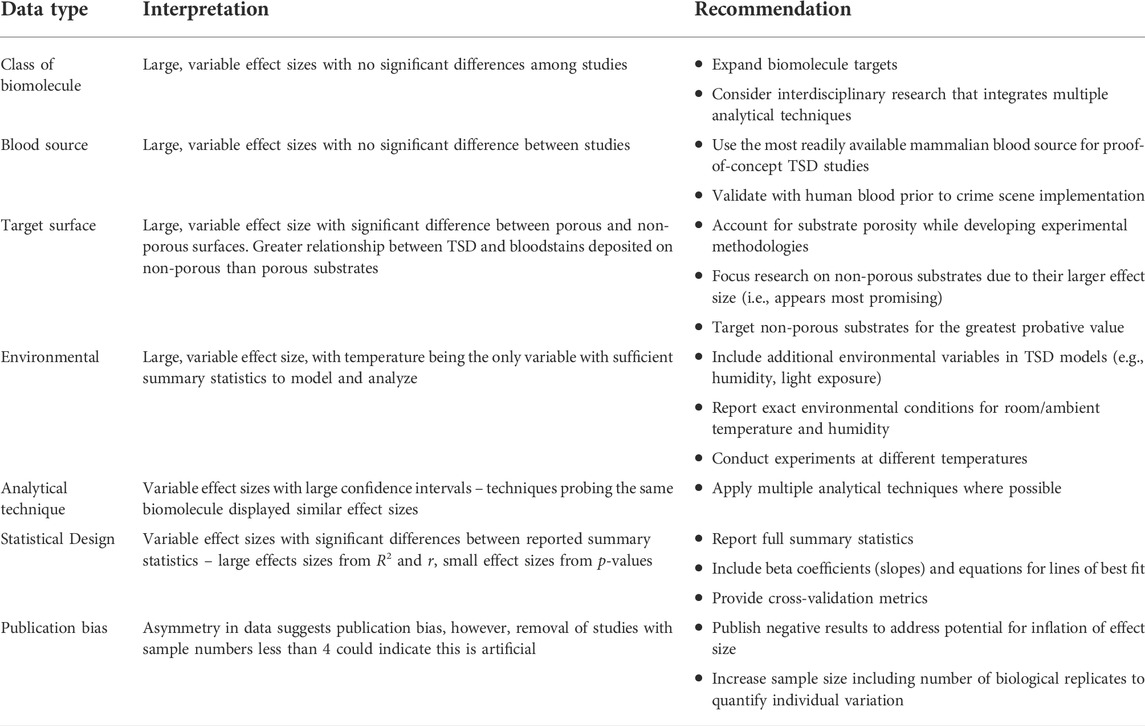
TABLE 1. | Summary of the meta-analysis results and recommendations for future work regarding each quantitative variable.
Substrate porosity
We observed a difference between substrate porosity but could not attribute a specific experimental cause to the observed discrepancy (Supplementary Table S5). We suspect that the strong TSD signature observed on non-porous surfaces is linked to environmental exposure and a lack of protection from and affinity towards the surface. Marrone and Hanson (Marrone and Ballantyne, 2009; Hanson and Ballantyne, 2010) both suggested that hemoglobin oxidation occurs at faster rates in dried states compared to liquid states—since desiccation and clotting of blood take longer to occur on porous substrates than on non-porous substrates (Laber and Epstein, 2001), conversion between hemoglobin derivatives are expected to occur sooner for blood deposited on non-porous surfaces. This faster process is expected to demonstrate stronger relationships with TSD than the slower occurring processes on porous substrates, which is corroborated by the larger slopes observed for non-porous substrates in our modelling. Porous substrates might also protect the blood and biomolecules within from physicochemical degradation; greater amounts of DNA could be extracted from porous substrates than their non-porous counterparts (Verdon et al., 2011). Based on the findings of our meta-analysis, we suspect that the choice of substrate porosity is dependent in part on the proposed analytical technique, which inherently influenced experimental conditions and results. However, this leads to a second key takeaway: TSD signatures are strongest when the fluid is deposited on non-porous surfaces. To have real-world and probative value, TSD studies should focus on these types of surfaces (e.g., Doty et al., 2016; Fang et al., 2019; Cossette et al., 2021). Additionally, recently proposed relative dating approaches - in which a bloodstain from the same individual as those from the crime scene are aged under identical conditions–might mitigate many sources of variability present in absolute dating techniques and should be further explored (Menżyk, 2021).
Future directions
TSD research often has disparate experimental methodologies, particularly around sample number, type, instrumental analysis, target biomolecule, environmental ageing conditions and statistical analysis.
Despite the large effect sizes, we are far from a predictive TSD model and implementation into casework. To help address these issues, all metadata have been made available on a public repository (https://gitlab.com/f4301/TSD.git). All summary statistics describing the relationship between relevant response variables and bloodstain TSD should be reported (r, R2, p-values, beta coefficients), and we encourage a move toward predictive modelling, cross-validation, and independent replicates. We must also increase sample sizes to fully capture variance, particularly among individuals, and report key variables like temperature and humidity values used during the study. Larger sample sizes are required for predictive modelling approaches like cross-validation. In addition, the reporting of summary statistics and use of larger sample sizes would allow for a greater percentage of studies to meet the inclusion criteria of future meta-analyses (Figure 1). Lastly, particularly regarding the development of predictive models and applications to casework, there needs to be a place for confirmatory and negative results. Our meta-analysis suggests a publication bias, not uncommon (Coltman and Slate, 2003; Shafer and Wolf, 2013; Page et al., 2021a), but in the context of developing predictive TSD models is problematic. We encourage the community to submit results to the above repository to build a database similar to DNA-Trac which documents DNA transfer studies (Gosch and Courts, 2019). Ultimately, tools are being developed to increase the precision and accuracy of bloodstain TSD estimates; these could eventually be combined with techniques estimating the time of day of deposition and the age of the donor, providing a holistic framework for all bloodstain time-related matters.
Data availability statement
The datasets presented in this study can be found in online repositories. The names of the repository/repositories and accession number(s) can be found in the article/Supplementary Material.
Author contributions
CE: Visualization, Writing - original draft, Formal analysis, Investigation, Funding acquisition, Software, Data curation, Methodology. AS: Conceptualization, Supervision, Funding acquisition, Resources, Writing - review and editing, Project administration, Methodology, Validation. TS: Conceptualization, Supervision, Funding acquisition, Resources, Writing - review and editing, Project administration, Methodology, Validation.
Funding
This work was supported by one NSERC Undergraduate Student Research Award (Canada) and one NSERC Canada Graduate Scholarship–Master’s program (Canada) to CE, Natural Sciences and Engineering Research Council of Canada, Grant/Award Number: RGPIN-2017-03934 to AS and RGPIN-2020-05816 to TS.
Acknowledgments
The authors would like to thank Mitchell Tiessen of the Forensic Chemistry and Materials Lab group at Ontario Tech University for insights and feedback on writing and visualizations.
Conflict of interest
The authors declare that the research was conducted in the absence of any commercial or financial relationships that could be construed as a potential conflict of interest.
Publisher’s note
All claims expressed in this article are solely those of the authors and do not necessarily represent those of their affiliated organizations, or those of the publisher, the editors and the reviewers. Any product that may be evaluated in this article, or claim that may be made by its manufacturer, is not guaranteed or endorsed by the publisher.
Supplementary material
The Supplementary Material for this article can be found online at: https://www.frontiersin.org/articles/10.3389/frans.2022.928573/full#supplementary-material
References
Acar, K., Kurtulus Dereli, A., Avci, E., Zeybek, V., Kutlu, E., Demir, S., et al. (2020). Determination of haemoglobin A1c levels using high-performance liquid chromatography of bloodstains. Med. Sci. Law 60, 19–25. doi:10.1177/0025802419879272
Ackermann, K., Ballantyne, K. N., and Kayser, M. (2010). Estimating trace deposition time with circadian biomarkers: A prospective and versatile tool for crime scene reconstruction. Int. J. Leg. Med. 124, 387–395. doi:10.1007/s00414-010-0457-1
Adam, C. D. (2012). Fundamental studies of bloodstain formation and characteristics. Forensic Sci. Int. 219, 76–87. doi:10.1016/j.forsciint.2011.12.002
Agudelo, J., Huynh, C., and Halámek, J. (2015). Forensic determination of blood sample age using a bioaffinity-based assay. Analyst 140, 1411–1415. doi:10.1039/c4an02269f
Alshehhi, S., and Haddrill, P. R. (2020). Evaluating the effect of body fluid mixture on the relative expression ratio of blood-specific RNA markers. Forensic Sci. Int. 307, 110116. doi:10.1016/j.forsciint.2019.110116
Alshehhi, S., McCallum, N. A., and Haddrill, P. R. (2017). Quantification of RNA degradation of blood-specific markers to indicate the age of bloodstains. Forensic Sci. Int. Genet. Suppl. Ser. 6, e453–e455. doi:10.1016/j.fsigss.2017.09.175
Anderson, S. E., Hobbs, G. R., and Bishop, C. P. (2011). Multivariate analysis for estimating the age of a bloodstain. J. Forensic Sci. 56, 186–193. doi:10.1111/j.1556-4029.2010.01551.x
Anderson, S., Howard, B., Hobbs, G. R., and Bishop, C. P. (2005). A method for determining the age of a bloodstain. Forensic Sci. Int. 148, 37–45. doi:10.1016/j.forsciint.2004.04.071
Andrasko, J. (1997). The estimation of age of bloodstains by HPLC analysis. J. Forensic Sci. 42, 14171J–14607J. doi:10.1520/jfs14171j
Arany, S., and Ohtani, S. (2011). Age estimation of bloodstains: A preliminary report based on aspartic acid racemization rate. Forensic Sci. Int. 212, 36–39. doi:10.1016/j.forsciint.2011.05.015
Asaghiar, F., and Williams, G. A. (2020). Evaluating the use of hypoxia sensitive markers for body fluid stain age prediction. Sci. Justice 60, 547–554. doi:10.1016/j.scijus.2020.09.001
Bauer, M., Polzin, S., and Patzelt, D. (2003). Quantification of RNA degradation by semi-quantitative duplex and competitive RT-PCR: A possible indicator of the age of bloodstains? Forensic Sci. Int. 138, 94–103. doi:10.1016/j.forsciint.2003.09.008
Bergmann, T., Heinke, F., and Labudde, D. (2017). Towards substrate-independent age estimation of blood stains based on dimensionality reduction and k-nearest neighbor classification of absorbance spectroscopic data. Forensic Sci. Int. 278, 1–8. doi:10.1016/j.forsciint.2017.05.023
Bergmann, T., Leberecht, C., and Labudde, D. (2021). Analysis of the influence of EDTA-treated reference samples on forensic bloodstain age estimation. Forensic Sci. Int. 325, 110876. doi:10.1016/j.forsciint.2021.110876
Bonaventura, C., Henkens, R., Alayash, A. I., Banerjee, S., and Crumbliss, A. L. (2013). Molecular controls of the oxygenation and redox reactions of hemoglobin. Antioxid. Redox Signal. 18, 2298–2313. doi:10.1089/ars.2012.4947
Botoniic-Sehic, E., Brown, C. W., Lamontaane, M., and Tsaparikos, M. (2009). Forensic application of near-infrared spectroscopy: Aging of bloodstains. Spectroscopy 24, 42–48.
Bremmer, R. H., de Bruin, D. M., de Joode, M., Buma, W. J., van Leeuwen, T. G., and Aalders, M. C. G. (2011a). Biphasic oxidation of Oxy-Hemoglobin in bloodstains. PLoS One 6, e21845–e21846. doi:10.1371/journal.pone.0021845
Bremmer, R. H., De Bruin, K. G., Van Gemert, M. J. C., Van Leeuwen, T. G., and Aalders, M. C. G. (2012). Forensic quest for age determination of bloodstains. Forensic Sci. Int. 216, 1–11. doi:10.1016/j.forsciint.2011.07.027
Bremmer, R. H., Nadort, A., van Leeuwen, T. G., van Gemert, M. J. C., and Aalders, M. C. G. (2011b). Age estimation of blood stains by hemoglobin derivative determination using reflectance spectroscopy. Forensic Sci. Int. 206, 166–171. doi:10.1016/j.forsciint.2010.07.034
Butler, J. M. (2006). Genetics and genomics of core short tandem repeat loci used in human identity testing. J. Forensic Sci. 51, 253–265. doi:10.1111/j.1556-4029.2006.00046.x
Cavalcanti, D. R., and Silva, L. P. (2019). Application of atomic force microscopy in the analysis of time since deposition (TSD) of red blood cells in bloodstains: A forensic analysis. Forensic Sci. Int. 301, 254–262. doi:10.1016/j.forsciint.2019.05.048
Cheung, M. W. (2014). Modeling dependent effect sizes with three-level meta-analyses: A structural equation modeling approach. Psychol. Methods 19, 211–229. doi:10.1037/a0032968
Choi, W., Shin, J., Hyun, K. A., Song, J., and Hyo-Il, J. (2019). Highly sensitive and accurate estimation of bloodstain age using smartphone. Biosens. Bioelectron. 130, 414–419. doi:10.1016/j.bios.2018.09.017
Coltman, D. W., and Slate, J. (2003). Microsatellite measures of inbreeding: A meta-analysis. Evolution 57, 971–983. doi:10.1111/j.0014-3820.2003.tb00309.x
Cossette, M. L., Stotesbury, T., and Shafer, A. B. A. (2021). Quantifying visible absorbance changes and DNA degradation in aging bloodstains under extreme temperatures. Forensic Sci. Int. 318, 110627. doi:10.1016/j.forsciint.2020.110627
Doty, K. C., McLaughlin, G., and Lednev, I. K. (2016). A Raman “spectroscopic clock” for bloodstain age determination: The first week after deposition. Anal. Bioanal. Chem. 408, 3993–4001. doi:10.1007/s00216-016-9486-z
Doty, K. C., Muro, C. K., and Lednev, I. K. (2017). Predicting the time of the crime: Bloodstain aging estimation for up to two years. Forensic Chem. 5, 1–7. doi:10.1016/j.forc.2017.05.002
Ecker, P., Sparer, A., Lukitsch, B., Elenkov, M., Seltenhammer, M., Crevenna, R., et al. (2021). Animal blood in translational research: How to adjust animal blood viscosity to the human standard. Physiol. Rep. 9, 1–10. doi:10.14814/phy2.14880
Edelman, G. J., Roos, M., Bolck, A., and Aalders, M. C. (2016). Practical implementation of blood stain age estimation using spectroscopy. IEEE J. Sel. Top. Quantum Electron. 22, 415–421. doi:10.1109/JSTQE.2016.2536655
Edelman, G., Manti, V., Van Ruth, S. M., Van Leeuwen, T., and Aalders, M. (2012a). Identification and age estimation of blood stains on colored backgrounds by near infrared spectroscopy. Forensic Sci. Int. 220, 239–244. doi:10.1016/j.forsciint.2012.03.009
Edelman, G., van Leeuwen, T. G., and Aalders, M. C. G. (2012b). Hyperspectral imaging for the age estimation of blood stains at the crime scene. Forensic Sci. Int. 223, 72–77. doi:10.1016/j.forsciint.2012.08.003
Egger, M., Smith, G. D., Schneider, M., and Minder, C. (1997). Bias in meta-analysis detected by a simple, graphical test. BMJ 315, 629–634. doi:10.1136/bmj.315.7109.629
Elliott, C. I., Stotesbury, T. E., and Shafer, A. B. A. (2022). Using total RNA quality metrics for time since deposition estimates in degrading bloodstains. J. Forensic Sci. 1, 1776–1785. doi:10.1111/1556-4029.15072
Fang, C., Zhao, J., Li, J., Qian, J., Liu, X., Sun, Q., et al. (2019). Massively parallel sequencing of microRNA in bloodstains and evaluation of environmental influences on miRNA candidates using realtime polymerase chain reaction. Forensic Sci. Int. Genet. 38, 32–38. doi:10.1016/j.fsigen.2018.10.001
Farré, X., Molina, R., Barteri, F., Timmers, P. R. H. J., Joshi, P. K., Oliva, B., et al. (2021). Comparative analysis of mammal genomes unveils key genomic variability for human life span. Mol. Biol. Evol. 38, 4948–4961. doi:10.1093/molbev/msab219
Ferraro, J., Nakamoto, K., and Brown, C. W. (2003). Introductory Raman spectroscopy. San Diego: Academic Press.
Fisher, R. (1921). On the “probable error” of a coefficient of correlation deduced from a small sample. Metron 1, 3–32.
Fordyce, S. L., Kampmann, M. L., van Doorn, N. L., and Gilbert, M. T. P. (2013). Long-term RNA persistence in postmortem contexts. Investig. Genet. 4, 7. doi:10.1186/2041-2223-4-7
Fu, J., and Allen, R. W. (2019). A method to estimate the age of bloodstains using quantitative PCR. Forensic Sci. Int. Genet. 39, 103–108. doi:10.1016/j.fsigen.2018.12.004
Fujita, Y., Tsuchiya, K., Abe, S., Takiguchi, Y., Kubo, S. I., and Sakurai, H. (2005). Estimation of the age of human bloodstains by electron paramagnetic resonance spectroscopy: Long-term controlled experiment on the effects of environmental factors. Forensic Sci. Int. 152, 39–43. doi:10.1016/j.forsciint.2005.02.029
Gautam, R., Peoples, D., Jansen, K., O’Connor, M., Thomas, G., Vanga, S., et al. (2020). Feature selection and rapid characterization of bloodstains on different substrates. Appl. Spectrosc. 74, 1238–1251. doi:10.1177/0003702820937776
Gosch, A., and Courts, C. (2019). On DNA transfer: The lack and difficulty of systematic research and how to do it better. Forensic Sci. Int. Genet. 40, 24–36. doi:10.1016/j.fsigen.2019.01.012
Guo, K., Zhegalova, N., Achilefu, S., and Berezin, M. Y. (2013). Bloodstain age analysis: Toward solid state fluorescent lifetime measurements. Adv. Biomed. Clin. Diagn. Syst. XI 8572, 1–7. doi:10.1117/12.2007756
Hanson, E., Albornoz, A., and Ballantyne, J. (2011). Validation of the hemoglobin (Hb) hypsochromic shift assay for determination of the time since deposition (TSD) of dried bloodstains. Forensic Sci. Int. Genet. Suppl. Ser. 3, 1–11. doi:10.1016/j.fsigss.2011.09.016
Hanson, E. K., and Ballantyne, J. (2010). A blue spectral shift of the hemoglobin soret band correlates with the age (time since deposition) of dried bloodstains. PLoS One 5, 128300–e12911. doi:10.1371/journal.pone.0012830
Harrer, M., Cuijpers, P., Furukawa, T. A., and Ebert, D. D. (2021). Doing meta-analysis with R: A hands-on guide. Boca Raton and London: Chapmann & Hall/CRC Press.
Hassan, N. F. N., Sandran, D. D., Mohamad, M., Zakaria, Y., and Muslim, N. Z. M. (2019). Estimation of the age of bloodstains on soil matrices by ATR-FTIR spectroscopy and chemometrics. Int. J. Innov. Technol. Explor. Eng. 9, 4750–4755. doi:10.35940/ijitee.A4454.119119
Heneghan, N., Fu, J., Pritchard, J., Payton, M., and Allen, R. W. (2021). The effect of environmental conditions on the rate of RNA degradation in dried blood stains. Forensic Sci. Int. Genet. 51, 102456. doi:10.1016/j.fsigen.2020.102456
Higgins, J. P. T., and Thompson, S. G. (2002). Quantifying heterogeneity in a meta-analysis. Stat. Med. 21, 1539–1558. doi:10.1002/sim.1186
Inoue, H., Takabe, F., Iwasa, M., and Maeno, Y. (1991). Identification of fetal hemoglobin and simultaneous estimation of bloodstain age by high-performance liquid chromatography. Int. J. Leg. Med. 104, 127–131. doi:10.1007/BF01369715
Inoue, H., Takabe, F., Iwasa, M., Maeno, Y., and Seko, Y. (1992). A new marker for estimation of bloodstain age by high performance liquid chromatography. Forensic Sci. Int. 57, 17–27. doi:10.1016/0379-0738(92)90041-T
Kapusta, A., Suh, A., and Feschotte, C. (2017). Dynamics of genome size evolution in birds and mammals. Proc. Natl. Acad. Sci. U. S. A. 114, E1460–E1469. doi:10.1073/pnas.1616702114
Kaur, S., Saini, V., and Dalal, R. (2020). UV-visible spectroscopic effect on haemoglobin & DNA degradation: A forensic approach. Forensic Sci. Int. 307, 110078. doi:10.1016/j.forsciint.2019.110078
Kim, S., Cho, Y. S., Kim, H. M., Chung, O., Kim, H., Jho, S., et al. (2016). Comparison of carnivore, omnivore, and herbivore mammalian genomes with a new leopard assembly. Genome Biol. 17, 211–212. doi:10.1186/s13059-016-1071-4
Kitagawa, T. (1987). “Resonance Raman spectra of heme and metalloproteins,” in Biological applications of Raman spectroscopy. Editor T. G. Spiro (New York, NY: Wiley).
Kohlmeier, F., and Schneider, P. M. (2012). Successful mRNA profiling of 23 years old blood stains. Forensic Sci. Int. Genet. 6, 274–276. doi:10.1016/j.fsigen.2011.04.007
Laber, T. L., and Epstein, B. P. (2001). Substrate effects on the clotting time of human blood. Can. Soc. Forensic Sci. J. 34, 209–214. doi:10.1080/00085030.2001.10757531
Lech, K., Liu, F., Ackermann, K., Revell, V. L., Lao, O., Skene, D. J., et al. (2016). Evaluation of mRNA markers for estimating blood deposition time: Towards alibi testing from human forensic stains with rhythmic biomarkers. Forensic Sci. Int. Genet. 21, 119–125. doi:10.1016/j.fsigen.2015.12.008
Lech, K., Liu, F., Davies, S. K., Ackermann, K., Ang, J. E., Middleton, B., et al. (2018). Investigation of metabolites for estimating blood deposition time. Int. J. Leg. Med. 132, 25–32. doi:10.1007/s00414-017-1638-y
Li, B., Beveridge, P., O’Hare, W. T., and Islam, M. (2013). The age estimation of blood stains up to 30 days old using visible wavelength hyperspectral image analysis and linear discriminant analysis. Sci. Justice 53, 270–277. doi:10.1016/j.scijus.2013.04.004
Li, B., Beveridge, P., O’Hare, W. T., and Islam, M. (2011). The estimation of the age of a blood stain using reflectance spectroscopy with a microspectrophotometer, spectral pre-processing and linear discriminant analysis. Forensic Sci. Int. 212, 198–204. doi:10.1016/j.forsciint.2011.05.031
Li, Z., Chen, D., Wang, Q., Tian, H., Tan, M., Peng, D., et al. (2021). mRNA and microRNA stability validation of blood samples under different environmental conditions. Forensic Sci. Int. Genet. 55, 102567. doi:10.1016/j.fsigen.2021.102567
Lin, H., Zhang, Y., Wang, Q., Li, B., Huang, P., and Wang, Z. (2017). Estimation of the age of human bloodstains under the simulated indoor and outdoor crime scene conditions by ATR-FTIR spectroscopy. Sci. Rep. 7, 13254–13259. doi:10.1038/s41598-017-13725-1
Majda, A., Wietecha-Posłuszny, R., Mendys, A., Wójtowicz, A., and Łydżba-Kopczyńska, B. (2018). Hyperspectral imaging and multivariate analysis in the dried blood spots investigations. Appl. Phys. A 124, 312–318. doi:10.1007/s00339-018-1739-6
Manasatienjig, C., and Nimnual, A. (2021). Forensic blood stain aging using reverse transcription real-time PCR. Forensic Sci. Int. Rep. 3, 100205–5. doi:10.1016/j.fsir.2021.100205
Marrone, A., and Ballantyne, J. (2009). Changes in dry state hemoglobin over time do not increase the potential for oxidative DNA damage in dried blood. PLoS One 4, e5110–e5118. doi:10.1371/journal.pone.0005110
Matsuoka, T., Taguchi, T., and Okuda, J. (1995). Estimation of bloodstain age by rapid determinations of oxyhemoglobin by use of oxygen electrode and total hemoglobin. Biol. Pharm. Bull. 18, 1031–1035. doi:10.1248/bpb.18.1031
Mayes, C., Houston, R., Seashols-Williams, S., LaRue, B., and Hughes-Stamm, S. (2019). The stability and persistence of blood and semen mRNA and miRNA targets for body fluid identification in environmentally challenged and laundered samples. Leg. Med. 38, 45–50. doi:10.1016/j.legalmed.2019.03.007
Mc Shine, S., Suhling, K., Beavil, A., Daniel, B., and Frascione, N. (2017). The applicability of fluorescence lifetime to determine the time since the deposition of biological stains. Anal. Methods 9, 2007–2013. doi:10.1039/c6ay03099h
Menżyk, A., Damin, A., Martyna, A., Alladio, E., Vincenti, M., Martra, G., et al. (2020). Toward a novel framework for bloodstains dating by Raman spectroscopy: How to avoid sample photodamage and subsampling errors. Talanta 209, 120565. doi:10.1016/j.talanta.2019.120565
Menżyk, A. (2021). “Toward a spectroscopy-based approach for estimating time elapsed since bloodstains deposition: Development of a novel framework for blood evidence evaluation,” (Katowice (POL): University of Silesia). [dissertation].
Miki, T., Kai, A., and Ikeya, M. (1987). Electron spin resonance of bloodstains and its application to the estimation of time after bleeding. Forensic Sci. Int. 35, 149–158. doi:10.1016/0379-0738(87)90051-x
Nagatomo, S., Nagai, M., and Kitagawa, T. (2011). A new way to understand quaternary structure changes of hemoglobin upon ligand binding on the basis of UV-resonance Raman evaluation of intersubunit interactions. J. Am. Chem. Soc. 133, 10101–10110. doi:10.1021/ja111370f
Nakagawa, S., Ockendon, N., Gillespie, D. O. S., Hatchwell, B. J., and Burke, T. (2007). Assessing the function of house sparrows’ bib size using a flexible meta-analysis method. Behav. Ecol. 18, 831–840. doi:10.1093/beheco/arm050
Nowakowski, J., and Tinoco, I. (1997). RNA structure and stability. Semin. Virol. 8, 153–165. doi:10.1006/smvy.1997.0118
Orr, A., Gualdieri, R., Cossette, M. L., Shafer, A., and Stotesbury, T. (2021). Whole bovine blood use in forensic research: Sample preparation and storage considerations. Sci. Justice. 61, 214–220. doi:10.1016/j.scijus.2021.02.004
Ou, X., Gao, J., Wang, H., Wang, H., Lu, H., and Sun, H. (2012). Predicting human age with bloodstains by sjTREC quantification. PLoS One 7, e42412–e42418. doi:10.1371/journal.pone.0042412
Page, M. J., Egger, M., Sterne, J. A. C., and Higgins, J. P. T. (2021a). Investigating and dealing with publication bias and other reporting biases in meta-analyses of health research: A review. Res. Synth. Methods 12, 248–259. doi:10.1002/jrsm.1468
Page, M. J., Moher, D., Bossuyt, P. M., Boutron, I., Hoffmann, T. C., Mulrow, C. D., et al. (2021b). PRISMA 2020 explanation and elaboration: Updated guidance and exemplars for reporting systematic reviews. BMJ 372, 1–36. doi:10.1136/bmj.n160
Premasiri, W. R., Lee, J. C., and Ziegler, L. D. (2012). Surface-enhanced Raman scattering of whole human blood, blood plasma, and red blood cells: Cellular processes and bioanalytical sensing. J. Phys. Chem. B 116, 9376–9386. doi:10.1021/jp304932g
Qi, B., Kong, L., and Lu, Y. (2013). Gender-related difference in bloodstain RNA ratio stored under uncontrolled room conditions for 28 days. J. Forensic Leg. Med. 20, 321–325. doi:10.1016/j.jflm.2012.09.014
Rahi, G. S., Adams, J. L., Yuan, J., Devone, D., and Mahmud, K. (2021). Whole human blood DNA degradation associated with artificial ultraviolet and solar radiations as a function of exposure time. Forensic Sci. Int. 319, 110674. doi:10.1016/j.forsciint.2020.110674
Rahikainen, A. L., Palo, J. U., de Leeuw, W., Budowle, B., and Sajantila, A. (2016). DNA quality and quantity from up to 16 years old post-mortem blood stored on FTA cards. Forensic Sci. Int. 261, 148–153. doi:10.1016/j.forsciint.2016.02.014
Rankin-Turner, S., Turner, M. A., Kelly, P. F., King, R. S. P., and Reynolds, J. C. (2019). Transforming presumptive forensic testing: In situ identification and age estimation of human bodily fluids. Chem. Sci. 10, 1064–1069. doi:10.1039/c8sc04133d
Salzmann, A. P., Arora, N., Russo, G., Kreutzer, S., Snipen, L., and Haas, C. (2021a). Assessing time dependent changes in microbial composition of biological crime scene traces using microbial RNA markers. Forensic Sci. Int. Genet. 53, 102537. doi:10.1016/j.fsigen.2021.102537
Salzmann, A. P., Russo, G., Aluri, S., and Haas, C. (2019). Transcription and microbial profiling of body fluids using a massively parallel sequencing approach. Forensic Sci. Int. Genet. 43, 102149. doi:10.1016/j.fsigen.2019.102149
Salzmann, A. P., Russo, G., Kreutzer, S., and Haas, C. (2021b). Degradation of human mRNA transcripts over time as an indicator of the time since deposition (TsD) in biological crime scene traces. Forensic Sci. Int. Genet. 53, 102524. doi:10.1016/j.fsigen.2021.102524
Seok, A. E., Lee, J., Lee, Y. R., Lee, Y. J., Kim, H. J., Ihm, C., et al. (2018). Estimation of age of bloodstains by mass-spectrometry: A metabolomic approach. Anal. Chem. 90, 12431–12441. doi:10.1021/acs.analchem.8b01367
Shafer, A. B. A., and Wolf, J. B. W. (2013). Widespread evidence for incipient ecological speciation: A meta-analysis of isolation-by-ecology. Ecol. Lett. 16, 940–950. doi:10.1111/ele.12120
Shin, J., Choi, S., Yang, J. S., Song, J., Choi, J. S., and Jung, H. Il (2017). Smart Forensic Phone: Colorimetric analysis of a bloodstain for age estimation using a smartphone. Sensors Actuators B Chem. 243, 221–225. doi:10.1016/j.snb.2016.11.142
Simard, A. M., DesGroseillers, L., and Sarafian, V. (2012). Assessment of RNA stability for age determination of body fluid stains. Can. Soc. Forensic Sci. J. 45, 179–194. doi:10.1080/00085030.2012.10757192
Sirker, M., Gomes, I., Rothschild, M. A., and Schneider, P. M. (2013). A 1-year time course study of human RNA degradation in body fluids under dry and humid environmental conditions. Forensic Sci. Int. Genet. Suppl. Ser. 4, 164–165. doi:10.1016/j.fsigss.2013.10.085
Sirker, M., Schneider, P. M., and Gomes, I. (2016). A 17-month time course study of human RNA and DNA degradation in body fluids under dry and humid environmental conditions. Int. J. Leg. Med. 130, 1431–1438. doi:10.1007/s00414-016-1373-9
Sparer, A., Serp, B., Schwarz, L., and Windberger, U. (2020). Storability of porcine blood in forensics: How far should we go? Forensic Sci. Int. 311, 110268. doi:10.1016/j.forsciint.2020.110268
Spiro, T. G., and Strekas, T. C. (1974). Resonance Raman spectra of heme proteins. Effects of oxidation and spin state. J. Am. Chem. Soc. 96, 338–345. doi:10.1021/ja00809a004
Stotesbury, T., Cossette, M.-L., Newell-Bell, T., and Shafer, A. B. A. (2020). An exploratory time since deposition analysis of whole blood using metrics of DNA degradation and visible absorbance spectroscopy. Pure Appl. Geophys. 178, 735–743. doi:10.1007/s00024-020-02494-0
Strasser, S., Zink, A., Kada, G., Hinterdorfer, P., Peschel, O., Heckl, W. M., et al. (2007). Age determination of blood spots in forensic medicine by force spectroscopy. Forensic Sci. Int. 170, 8–14. doi:10.1016/j.forsciint.2006.08.023
Sun, H., Dong, Y., Zhang, P., Meng, Y., Wen, W., Li, N., et al. (2017a). Accurate age estimation of bloodstains based on visible reflectance spectroscopy and chemometrics methods. IEEE Photonics J. 9, 1–14. doi:10.1109/JPHOT.2017.2651580
Sun, H., Meng, Y., Zhang, P., Li, Y., Li, N., Li, C., et al. (2017b). Non-invasive prediction of bloodstain age using the principal component and a back propagation artificial neural network. Laser Phys. Lett. 14, 095601. doi:10.1088/1612-202X/aa7c48
Takamura, A., Watanabe, D., Shimada, R., and Ozawa, T. (2019). Comprehensive modeling of bloodstain aging by multivariate Raman spectral resolution with kinetics. Commun. Chem. 2, 1–10. doi:10.1038/s42004-019-0217-1
Thanakiatkrai, P., Yaodam, A., and Kitpipit, T. (2013). Age estimation of bloodstains using smartphones and digital image analysis. Forensic Sci. Int. 233, 288–297. doi:10.1016/j.forsciint.2013.09.027
Thong, Z., Liang, X., Chan, S., Ying, J., Tan, Y., Loo, E. S., et al. (2017). Evaluation of DNA methylation-based age prediction on blood. Forensic Sci. Int. Genet. Suppl. Ser. 6, 249–251. doi:10.1016/j.fsigss.2017.09.095
Verdon, T. J., Mitchell, R. J., and Van Oorschot, R. A. H. (2011). Evaluating the efficiency of DNA extraction methods from different substrates. Forensic Sci. Int. Genet. Suppl. Ser. 3, 93–94. doi:10.1016/j.fsigss.2011.08.046
Verdon, T. J., Mitchell, R. J., and Oorschot, R. A. H. Van (2013). The influence of substrate on DNA transfer and extraction efficiency. Forensic Sci. Int. Genet. 7, 167–175. doi:10.1016/j.fsigen.2012.09.004
Viechtbauer, W. (2010). Conducting meta-analyses in R with the metafor package. J. Stat. Softw. 36, 1–48. doi:10.18637/jss.v036.i03
Wang, Q., Teng, G., Zhao, Y., Cui, X., and Wei, K. (2019). Identification and determination of the bloodstains dry time in the crime scenes using laser-induced breakdown spectroscopy. IEEE Photonics J. 11, 1–12. doi:10.1109/JPHOT.2019.2912580
Weber, A. R., and Lednev, I. K. (2020). Crime clock – analytical studies for approximating time since deposition of bloodstains. Forensic Chem. 19, 1–16. doi:10.1016/j.forc.2020.100248
Weber, A., Wójtowicz, A., and Lednev, I. K. (2021). Post deposition aging of bloodstains probed by steady-state fluorescence spectroscopy. J. Photochem. Photobiol. B 221, 112251. doi:10.1016/j.jphotobiol.2021.112251
Weinbrecht, K. D., Fu, J., Payton, M., and Allen, R. (2017). Time-dependent loss of mRNA transcripts from forensic stains. Res. Rep. Forensic Med. Sci. 7, 1–12. doi:10.2147/rrfms.s125782
Windberger, U., Bartholovitsch, A., Plasenzotti, R., Korak, K. J., Heinze, G., and Bartholovitsch, A. (2003). Whole blood viscosity , plasma viscosity and erythrocyte aggregation in nine mammalian species: Reference values and comparison of data. Exp. Physiol. 88, 431–440. doi:10.1113/eph8802496
Windberger, U. (2019). “Blood suspensions in animals,” in Dynamics of blood cell suspensions in microflows (Boca Raton, Florida, United States: CRC Press), 371–419.
Wu, J., Xiao, J., Zhang, Z., Wang, X., Hu, S., and Yu, J. (2014). Ribogenomics: The science and knowledge of RNA. Genomics Proteomics Bioinforma. 12, 57–63. doi:10.1016/j.gpb.2014.04.002
Wu, Y., Hu, Y., Cai, J., Ma, S., Wang, X., Chen, Y., et al. (2009). Time-dependent surface adhesive force and morphology of RBC measured by AFM. Micron 40, 359–364. doi:10.1016/j.micron.2008.10.003
Wurtmann, E. J., and Wolin, S. L. (2009). RNA under attack: Cellular handling of RNA damage. Crit. Rev. Biochem. Mol. Biol. 44, 34–49. doi:10.1080/10409230802594043
Yamamoto, T., Palmer, G., Gill, D., Salmeen, I. T., and Rimai, L. (1973). The valence and spin state of iron in oxyhemoglobin as inferred from resonance Raman spectroscopy. J. Biol. Chem. 248, 5211–5213. doi:10.1016/s0021-9258(19)43692-2
Yamanoi, E., Uchiyama, S., Sakurada, M., and Ueno, Y. (2018). sjTREC quantification using SYBR quantitative PCR for age estimation of bloodstains in a Japanese population. Leg. Med. 32, 71–74. doi:10.1016/j.legalmed.2018.03.003
Zadora, G., and Menzyk, A. (2018). In the pursuit of the holy grail of forensic science – spectroscopic studies on the estimation of time since deposition of bloodstains. TrAC Trends Anal. Chem. 105, 137–165. doi:10.1016/j.trac.2018.04.009
Zbieć-Piekarska, R., Spólnicka, M., Kupiec, T., Makowska, Z., Spas, A., Parys-Proszek, A., et al. (2015). Examination of DNA methylation status of the ELOVL2 marker may be useful for human age prediction in forensic science. Forensic Sci. Int. Genet. 14, 161–167. doi:10.1016/j.fsigen.2014.10.002
Zhang, Y., Wang, Q., Li, B., Wang, Z., Li, C., Yao, Y., et al. (2017). Changes in attenuated total reflection Fourier transform infrared spectra as blood dries out. J. Forensic Sci. 62, 761–767. doi:10.1111/1556-4029.13324
Keywords: forensic chemistry, applied genomics, time since deposition (TSD), bloodstains, spectroscopy, biomolecules, substrate porosity
Citation: Elliott CI, Shafer ABA and Stotesbury TE (2022) The crux of time: A meta-analysis of ex vivo whole blood degradation. Front. Anal. Sci. 2:928573. doi: 10.3389/frans.2022.928573
Received: 25 April 2022; Accepted: 10 October 2022;
Published: 24 October 2022.
Edited by:
Jan Halamek, Texas Tech University, United StatesReviewed by:
Flaminia Vincenti, Sapienza University of Rome, ItalyAlicja Menżyk, University of Silesia of Katowice, Poland
Copyright © 2022 Elliott, Shafer and Stotesbury. This is an open-access article distributed under the terms of the Creative Commons Attribution License (CC BY). The use, distribution or reproduction in other forums is permitted, provided the original author(s) and the copyright owner(s) are credited and that the original publication in this journal is cited, in accordance with accepted academic practice. No use, distribution or reproduction is permitted which does not comply with these terms.
*Correspondence: Theresa E. Stotesbury, theresa.stotesbury@ontariotechu.ca