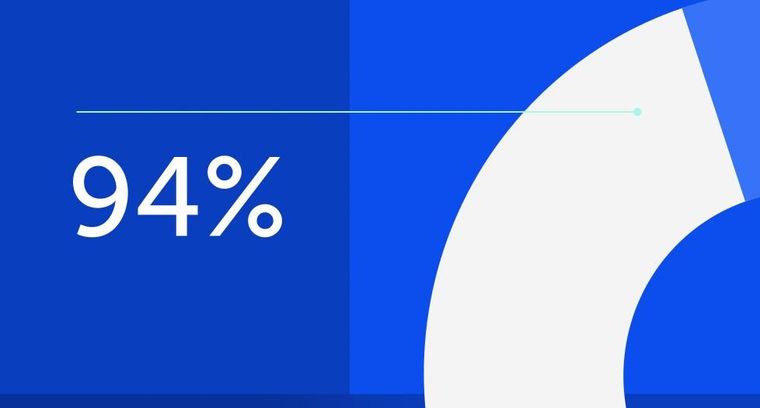
94% of researchers rate our articles as excellent or good
Learn more about the work of our research integrity team to safeguard the quality of each article we publish.
Find out more
ORIGINAL RESEARCH article
Front. Agron., 16 April 2025
Sec. Agroecological Cropping Systems
Volume 7 - 2025 | https://doi.org/10.3389/fagro.2025.1570567
Rising feed and fertilizer costs, climatic uncertainties, and the summer slump in forage production are key challenges for livestock farmers in the Midwest region of the United States. Therefore, this study evaluated the dry matter yield (DMY), forage nutritive value (FNV), water use efficiency (WUE), and economic viability of forage soybean (Glycine max (L.) Merr) for the Midwest rainfed cropping system. The research aimed to assess the suitability of forage soybean as an alternative summer forage crop that is drought-resilient, require lower inputs, and provide higher yield and forage quality compared to traditional forages. A three-year field experiment (2020-2022) using a randomized complete block design with four replications assessed two planting dates (mid-May, early July) and four growth stages (V2, V3, R1, R3). DMY was significantly influenced by planting dates and growth stages, with optimum planting (mid-May) yielding an average of 13.9 ± 0.5 Mg ha-¹ at the R3 stage, surpassing late planting (early July) by 51%. Significant variations in FNV parameters were observed between optimum and late planting dates and across different growth stages. Late planting improved forage nutritive value (FNV), with lower acid detergent fiber (ADF) (26% vs. 31%), neutral detergent fiber (NDF) (30% vs. 35%), and lignin (6% vs. 7%), alongside higher in vitro dry matter digestibility (IVDMD) (84% vs. 79%) and relative forage quality (RFQ) (237 vs. 197) when harvested at the R3 stage. Crude protein remained stable (19–21%) across growth stages. Overall forage quality (RFV and RFQ) remained stable across growth stages (from V2 to R3), ensuring consistent quality and flexible harvest timing. The forage soybean demonstrated a WUE of 20 kg ha-¹ mm-¹ and a net profit of $336 with 32% return on investment per hectare. These results position forage soybean as a drought-resilient, high-yielding, high-quality, and economically viable alternative to traditional forages, addressing seasonal shortages and enhancing sustainability in rainfed systems. Further research, particularly animal feeding trials and long-term soil health impacts, is recommended to validate its potential for widespread adoption.
The Midwestern region is a major agricultural production hub in the United States, with over 51 million hectares of farmland (USDA-NASS, 2024). Rainfed agriculture is a common practice in this region, where crop production relies heavily on natural rainfall. Approximately 75% of this farmland is dedicated to maize (Zea mays L.) and soybean (Glycine max (L.) Merrill), while the remaining 25% supports other crops, such as wheat (Triticum aestivum L.), alfalfa (Medicago sativa), oats (Avena sativa), and vegetables USDA-ESMIS (2024). Predominantly, the cropping system involves maize-soybean rotations, though continuous maize is common in high-livestock areas to meet feed and ethanol demands. States like Missouri and Kansas often practice wheat-corn-soybean rotations or double cropping such as winter wheat followed by soybean, maize, or sorghum (Sorghum bicolor) (Dhuyvetter et al., 1996; Holman et al., 2021). In the Central Great Plains, continuous rainfed wheat-fallow systems are widely practiced (Biermacher et al., 2006; Edwards et al., 2011; Nielsen, 2011; Patrignani et al., 2019).
Forages such as alfalfa, red clover (Trifolium pratense), tall fescue (Schedonorus arundinaceus), and sorghum-sudangrass (Sorghum bicolor x Sorghum sudanese) dominate hay and pasture systems. However, these forages have some limitations: alfalfa requires high water and fertility inputs; red clover has limited drought tolerance and a short lifespan; tall fescue may contain toxic alkaloids produced by an endophytic fungus; and sorghum-sudangrass poses risks of prussic acid and nitrate poisoning under stress.
Moreover, livestock farmers in the Midwest often face challenges related to forage shortages, particularly during late fall and early spring (Baath et al., 2024; Beck et al., 2022; Rao et al., 2005). These shortages are mainly caused by traditional cropping systems, rising feed and fertilizer prices (Schnitkey et al., 2022) and unpredictable weather events, such as variable rainfall patterns, periodic droughts, summer heat, and hail (NOAA-NIDIS, 2024; Roozeboom et al., 2008). These challenges have negatively impacted summer forage production, often leading to reduced forage acreage, lower yields, and decreased forage quality, thereby exacerbating the supply-demand gap in the forage market. Over the past 20 years (2002-2022), the cattle inventory, including calves, has decreased by 4% in the Midwestern states, while the forage production area has declined by 25% during the same period (USDA-NASS, 2024). This trend has driven the demand for more efficient forage production in the region. Due to the aforementioned factors and conditions, the growing forage supply-demand gap emphasizes the need to explore alternative summer forage crops that address seasonal shortages, require lower inputs, produce higher biomass, offer better forage quality, and improve soil health. In this context, integration of forage soybean [Glycine max (L.) Merr] into the U.S. rainfed cropping system presents numerous benefits, including enhanced soil fertility, improved yield potential, excellent forage quality, and effective weed management (Crusciol et al., 2012; Entz et al., 2002, Jahanzad et al., 2016; Machado et al., 2017; Nielsen, 2011; Sheaffer and Seguin, 2003; Sinclair and Vadez, 2012). Forage soybeans can be effectively integrated into double cropping systems, where they follow winter crops like wheat or barley. This strategy not only utilizes the land efficiently but also extends the growing season for forage production (Nurbekov et al., 2013). Its adaptability to various cropping practices and resilience in changing climatic conditions make it a promising summer crop for sustainable agricultural practices.
Previous studies have reported dry matter yields (DMY) exceeding 8 Mg ha-¹ when harvested between the early flowering (R1) and pod formation (R3-R7) stages (Baral, 2023; Nielsen, 2011; Sheaffer et al., 2001; Taylor, 2014). Despite these advantages, the adoption of forage soybean in the U.S. rainfed areas remains limited, with insufficient research on its potential as a summer forage crop in the region. Thus, the main objective of this study was to evaluate dry matter yield, forage nutritive value, water use efficiency, and the profitability of forage soybean grown under rainfed conditions in the U.S. Midwest regions.
A three-year field experiment (2020-2022) was conducted at the Ashland Bottoms Experiment Research Station in Manhattan, Kansas, USA (39.124945°N latitude, -96.635112°W longitude). The soil at the site is classified as silty clay loam with a 0 to 1% slope. Key soil properties include a pH of 6.4, total nitrogen of 0.13%, organic carbon of 1.38%, Mehlich extractable phosphorus of 20.44 μg g-1, potassium of 323 μg g-1, bulk density of 1 g cm-3, and volumetric water content of 33% at a depth of 0 to 20 cm. The previous cropping system was a sorghum-soybean-wheat conventional tillage rotation. The 30-year average summer crop growing season (March-October) rainfall is 710 mm, with monthly averages ranging from 46 mm in March to 123 mm in June. Average temperatures during this period range from 7°C in March to 27°C in July (Kansas Mesonet, 2024; Figure 1).
Figure 1. Average monthly temperature and rainfall distribution of study location (Ashland Bottoms, Manhattan, Kansas), during the study period (2020-2022) and the past 30 years’ average (1992-2022) (Kansas Mesonet, 2024).
The experiment was conducted for three years (May 2020 to October 2022) in a randomized complete block design with four replications. Each plot measured 12.19 meters in width and 15.24 meters in length (185.77 m2). Forage soybean (var. Large Lad RR™) seeds were sown in two planting dates after wheat harvest: an optimum planting date and a late planting date. For the optimum planting, seeds were sown in mid-May (17-22 May), while for the late planting, seeds were sown in early July (06-08 July). The seeds were planted at a depth of 1.2 cm with a row spacing of 76 cm, using a no-till drill. A seeding rate of 140,000 per hectare was used for both planting dates.
Samples were collected from a 0.14 m² area within each plot at the vegetative stages (second and third trifoliate leaves, V2 and V3) and reproductive stages (beginning of flowering, R1, and beginning of pod formation, R3). The growth stages of forage soybean are defined according to Fehr et al. (1971). Plants were harvested 2-5 cm above ground level using a hand sickle and dried at 60°C for 72 hours. Dried samples were weighed, and values from each 0.14 m² sample were converted to DMY in Megagrams per hectare (Mg ha-1).
Dried samples were finely ground (< 1 mm) using a Wiley mill (Wiley® Mill 4 1/2 HP, Thomas Scientific, NJ, USA). Key forage nutritive value (FNV) parameters including crude protein (CP), acid detergent fiber (ADF), neutral detergent fiber (NDF), in-vitro dry matter digestibility (IVDMD), net energy for maintenance (NEM), relative feed value (RFV), and relative forage quality (RFQ) were analyzed using near-infrared reflectance spectroscopy (NIRS). This analysis was conducted as described by Marten et al. (1985), using the Blue Sun Scientific Phoenix 5000 NIR instrument and BlueScan software.
The ground forage samples were scanned using an NIRS instrument, and the spectral data were analyzed with a Partial Least Squares Regression (PLSR) model. This model was calibrated for each study year by the NIRS Forage and Feed Consortium, Berea, Kentucky USA. The calibration dataset was developed using wet chemistry methods (AOAC, 2005). NDFD was determined using the following Equation 1:
Where:
● dNDF: Digestible neutral detergent fiber.
● aNDF: Amylase-treated neutral detergent fiber.
IVDMD was determined using the Goering and Van Soest (1970) method with modifications, where forage samples were fermented in flasks containing a composite inoculum of strained ruminal fluid and blended ruminal solids. Following fermentation, the residue was analyzed using the neutral detergent fiber (NDF) procedure to determine digestibility.
The statistical analysis was conducted using R 4.3.0 (Wickham et al., 2019) with multiple packages to evaluate the effects of planting time and growth stage on forage dry matter yield and forage nutritive value parameters. Given the randomized complete block design (RCBD), replication (block) was explicitly included as a random effect to account for variability among experimental units. A two-way analysis of variance (ANOVA) was initially performed using the aov function in the stats package, incorporating replication as a blocking factor Equation 2:
Where response variable represents each measured variable (DMY, CP, ADF, NDF, TDN, IVDMD, NEM, Lignin, RFV and RFQ). The ANOVA was conducted using the aov function in the stats package. However, since replication is a random effect, a linear mixed-effects model (LMM) was also fitted using the lmer function in the lme4 package to more appropriately account for variance Equation 3:
Post hoc analysis was conducted using the emmeans package to compute least squares means (LSMeans), providing adjusted mean estimates for each treatment combination. Tukey’s Honest Significant Difference (HSD) test was used to determine significant differences among treatment combinations at α = 0.05. The compact letter display (CLD) method was used to assign grouping letters based on statistical significance using the cld function from the multcompView package. To ensure result reliability, standard errors (SEs) and confidence intervals (CIs) were reported for all response variables. Additionally, ggplot2 was used for data visualization, while dplyr and tidyr have been used for data manipulation and restructuring before analysis. These statistical methods ensured robust and reliable comparisons among treatments while appropriately accounting for variability and controlling multiple testing errors.
The water use efficiency (WUE) of forage soybean was calculated by analyzing DMY as a function of growing season crop evapotranspiration (ETc) using a linear production function model (Baral et al., 2022; French and Schultz, 1984; Nielsen, 2011; Ullah et al., 2019; Equation 4). DMY data were collected from field experiments, while ETc was estimated by multiplying reference evapotranspiration (ETo), recorded at Kansas Mesonet weather station located 150 meters from the experimental field, with crop-specific coefficients (Kc) corresponding to the soybean growth stages (Allen et al., 1998).
Where:
● ETc: Actual crop evapotranspiration in millimeters (mm) recorded during forage growing season (from planting to forage biomass harvest period).
● ETo: Reference evapotranspiration over the same period.
● Kc: Crop coefficient for forage soybean at specific growth stages, as reported by Allen et al. (1998).
Then, the linear regression model was developed with DMY as the dependent variable and ETc as the independent variable, and WUE was determined as the slope of the regression line, representing the kilograms of dry matter produced per hectare per millimeter of water used. The model’s performance was evaluated using statistical metrics such as R² and p-values to confirm the strength and significance of the DMY-ETc relationship. The regression equation for the model can be expressed as Equation 5:
Where:
● DMY: Dry matter yield (Mg ha-1).
● ETc: Growing season crop evapotranspiration (mm).
● : Intercept, representing the DMY when ETc is zero (theoretically, baseline yield without water use).
● : Slope, representing WUE or the increase in DMY per unit increase in ETc (kg ha-1 mm-1).
Net farm income was computed using three years’ average observed DMY harvested at the R3 growth stage, with a 20% deduction for assumed harvesting and storage loss (Idowu et al., 2013; Orloff and Mueller, 2008; Undersander, 2001). The variable costs include seed, fertilizer, planting, and the entire haying operation, while the fixed costs cover land rental rates, crop insurance, machinery repair and maintenance, and farm equipment depreciation. Seed and chemical input costs were based on K-State Research and Extension recommendations (Ciampitti et al., 2016) and the current market value. Other variable costs were based on Kansas Custom Rates 2022 (Kansas Department of Agriculture, 2022). Land rental rates were determined using the Kansas 2022 Farm Real Estate Value and Cash Rent (USDA-NASS, 2022). The analysis covered the entire haying operation including cutting, conditioning, raking, baling, hauling, and stacking. The hay prices were used as the average annual hay price in Kansas in 2022, reported by the Kansas Direct Hay Report (USDA-AMS, 2023). A 5% overhead cost and a 6.5% interest rate were incorporated into the overall operating expenses. The Kansas income tax rate of 5.7% was added to the total pre-tax income to get net farm income. The net profit after tax was calculated by subtracting total costs including operating costs, interests and taxes, from total revenue. The formula used for calculating net profit is as Equation 6:
This calculation reflects the net farm income after all financial obligations have been met. These calculations are essential for assessing economic viability and guiding future investment decisions. Return on Investment (ROI) was determined by using the following Equation 7:
Both planting time and growth stage had a highly significant effect on DMY (p< 0.001). Additionally, the interaction between planting time and growth stage was also highly significant (p< 0.001) (Supplementary Tables S1, S2). At the V2 stage, the average DMY was recorded at 3.3 ± 0.6 Mg ha-¹ for the optimum planting, which was 15% higher than the 2.9 ± 0.6 Mg ha-¹ observed under late planting (Figure 2). Similarly, at the V3 stage, DMY was 5 ± 0.6 Mg ha-¹ for both planting dates.
Figure 2. Forage dry matter yield of forage soybean at different growth stages (V2, V3, R1, and R3) and planting times (optimum and late).
As the crop advanced to the reproductive stages, the differences between planting dates became more pronounced. At the R1 stage, the DMY for optimum planting increased significantly to 12.2 ± 0.6 Mg ha-¹, approximately 40% higher than the 8.7 Mg ha-¹ observed under late planting. This trend continued at the R3 stage, where the DMY for optimum planting reached 13.9 ± 0.5 Mg ha-¹, exceeding the 9.2 ± 0.8 Mg ha-¹ recorded under late planting by 51%. In both planting dates, the trend demonstrated a substantial increase in DMY as the crop advanced through the growth stages.
The forage nutritive value parameters varied between optimum and late planting dates and growth stages, with significant effects observed for most parameters (Supplementary Tables S1, S2).
Planting time did not have a significant effect on CP (p = 0.199). However, growth stage had a significant effect on CP (p< 0.05). The interaction between planting time and growth stage was not significant (p = 0.574). CP decreased as the plants matured. At the V2 stage, CP was 22 ± 1% for both optimum and late planting dates, but at the R1 stage, CP declined to 17 ± 1% and 20 ± 1% for optimum and late, respectively (Figure 3). Interestingly, at the R3 stage, CP remained relatively stable, with values of 19 ± 1% for the optimum planting date and 20 ± 2% for the late planting date. These results suggest that CP content is generally higher at earlier growth stages but remains relatively stable (19-21%) from V3 to R3, indicating prolonged retention of protein content in forage soybean, irrespective of planting time.
Figure 3. Crude protein content of forage soybean at different growth stages (V2, V3, R1, and R3) and planting times (optimum and late).
Both acid detergent fiber (ADF) and neutral detergent fiber (NDF) were significantly influenced by planting time and growth stage (p< 0.05), with fiber levels increasing as plants matured. The interaction between planting time and growth stage did not significantly affect ADF (p > 0.05). ADF values were lower for late planting at all growth stages, suggesting better forage digestibility (Figure 4). For example, at the V2 stage, optimum planting had an ADF of 33 ± 2%, while late planting recorded 28 ± 2%. This trend continued across other stages, such as R1, where optimum planting showed 38 ± 2% compared to 31 ± 2% for late planting.
Figure 4. Fiber content (ADF and NDF) of forage soybean at different growth stages (V2, V3, R1, and R3) and planting times (optimum and late).
NDF was also consistently lower for late planting, which is desirable for improved forage intake by livestock. At the R1 stage, NDF was 43 ± 3% for optimum planting compared to 35 ± 3% for late planting. This indicates that late planting may result in forage with higher palatability and intake potential.
Planting time had a significant effect on TDN (p< 0.05), while growth stage had a highly significant effect (p< 0.01). The interaction between planting time and growth stage was not significant (p = 0.838). Late planting consistently resulted in slightly higher TDN across all stages (Figure 5). For example, TDN at the V2 stage was 61 ± 2% for late planting and 58 ± 3% for optimum planting. At the R3 stage, TDN for late planting increased to 62 ± 2% compared to 60 ± 3% for optimum planting.
Figure 5. Total digestible nutrient content of forage soybean at different growth stages (V2, V3, R1, and R3) and planting times (optimum and late).
Planting time (p< 0.01), and growth stage (p< 0.05) had a significant effect on lignin content. The interaction between planting time and growth stage was not significant (p = 0.874). Late planting consistently had slightly lower lignin content. For instance, at the V2 stage, lignin content was 7 ± 0.4% for optimum planting compared to 6 ± 0.4% for late planting (Figure 6). At the R3 stage, the lignin content was 7.1 ± 0.3% for optimum planting and 5.8 ± 0.6% for late planting. This trend persisted across all stages, with lower lignin levels in late planting contributing to better forage digestibility.
Figure 6. Lignin content of forage soybean at different growth stages (V2, V3, R1, and R3) and planting times (optimum and late).
Planting time significantly affected IVDMD (p< 0.01), and growth stage also had a significant effect (p< 0.05). The interaction between planting time and growth stage was not significant (p = 0.752). Late planting consistently had higher IVDMD across all stages, indicating improved digestibility (Figure 7). At the V2 stage, optimum planting recorded 81 ± 2% compared to 84 ± 2% for optimum planting, and at the R3 stage, optimum planting maintained a higher value of 79 ± 1% compared to 84 ± 3% for late planting.
Figure 7. In-vitro dry matter digestibility of forage soybean at different growth stages (V2, V3, R1, and R3) and planting times (optimum and late).
Planting time and growth stage both influenced the NEM and NEL (p< 0.05). Optimum planting consistently resulted in lower NEM and NEL values compared to late planting across all stages (Figure 8). For instance, at the V2 stage, optimum planting had a NEM of 0.564 ± 0.022 Mcal pound-1 and a NEL of 0.590 ± 0.016 Mcal pound-1, while late planting had higher values with a NEM of 0.615 ± 0.022 Mcal pound-1 and a NEL of 0.628 ± 0.016 Mcal pound-1. This trend was consistent across all growth stages, suggesting that late planting may enhance both the energy maintenance and lactation potential of forage soybean.
Figure 8. Energy content for maintenance (left) and for lactation (right) of forage soybean at different growth stages (V2, V3, R1, and R3) and planting times (optimum and late).
Planting time had a significant effect on both RFV (p< 0.05) and RFQ (p< 0.01). However, growth stage did not significantly affect RFV (p = 0.104) or RFQ (p = 0.066). Additionally, the interaction between planting time and growth stage was not significant for either RFV (p = 0.583) or RFQ (p = 0.792). RFV and RFQ was consistently higher under late planting, indicating superior forage quality. At the V2 stage, RFV and RFQ for late planting were 206 ± 16 and 246 ± 18, respectively, compared to 169 ± 16 and 202 ± 18 for optimum planting (Figure 9). At the R3 stage, RFV and RFQ for late planting were 218 ± 22 and 237 ± 26, respectively, while optimum planting recorded lower values of 183 ± 13 and 197 ± 15.
Figure 9. Forage quality (RFV and RFQ) of forage soybean at different growth stages (V2, V3, R1, and R3) and planting times (optimum and late).
The relationship between growing season ETc and DMY for forage soybean was evaluated using a linear production function model (Figure 10). Our result indicated that forage soybean exhibits a linear correlation between ETc and DMY, with a production function slope of 0.02 Mg ha-1 mm-1, suggests that for every millimeter increase in ETc, the dry matter yield increases by 20 kg ha-1. The coefficient of determination (R2) value of 0.85 indicates that 85% of the variation in dry matter yield is explained by the growing season evapotranspiration.
Figure 10. The relationship between dry matter yield and growing season crop evapotranspiration for forage soybean, with water use efficiency estimated using linear production function model.
The financial analysis of forage soybean hay production revealed a revenue of $1,391 per hectare, with total operating costs amounting to $1,035 per hectare, which included variable costs of $838 and fixed costs of $198 (Figure 11). After deducting income taxes and operating expenses, the net profit after tax was $336 per hectare with 32% ROI. These findings suggest that forage soybean farming is financially profitable after accounting for all expenses and taxes.
Figure 11. Financial overview of forage soybean planted in mid-May and harvested at R3 growth stage.
The findings of this study revealed the significant potential of forage soybean as a valuable summer annual forage option for forage producers, particularly due to its high DMY, nutritious forage, water-efficient growth, and economic viability.
The average estimated DMY of 13.9 ± 05 Mg ha-1 at R3 growth stage, indicates its capacity to produce substantial biomass even under rainfed scenarios. The DMY was notably higher for the optimum planting date compared to the late planting date, particularly as the crop advanced to reproductive stages. This trend may be attributed to the rainfall distribution pattern, as significant rainfall occurred during the critical growth period in June and July. In contrast, late-planted soybean experienced suboptimal moisture conditions due to lower rainfall or increased evapotranspiration demand later in the season, limiting growth and reducing DMY. At the R1 and R3 stages, the DMY for optimum planting was significantly higher by 40% and 51%, respectively, compared to late planting. This indicates that timely planting can substantially enhance biomass production, which is crucial for maximizing forage yield. The greater DMY for optimum planting may be attributed to a longer growing period and probably more rainfall, allowing the plants to accumulate more biomass before senescence. These results are consistent with previous studies demonstrating the adverse impact of delayed planting on vegetative growth and overall yield of soybean (Bastidas et al., 2008; Bateman et al., 2020; Hu and Wiatrak, 2012). Baghdadi et al. (2016) found that intercropping soybean with corn resulted in dry matter yields comparable to or exceeding those of monocropped corn DMY (∼14.10 t/ha), Furthermore, intercropping forage soybean with perennial grasses can maintain or even enhance overall yield. For example, intercropping with palisadegrass has shown to be effective, particularly when using early cycle soybean cultivars, which do not negatively impact the yield of component crop (Crusciol et al., 2012). These findings suggest that forage soybean can effectively contribute to overall forage production when intercropped with crops that are compatible with it.
Notably, the DMY of 13.9 Mg ha-¹ at R3 is competitive with traditional forages like sorghum-sudangrass (7–15 Mg ha-¹) and alfalfa (5–19 Mg ha-¹), as reported by Anfinrud et al. (2013), Machicek et al. (2019), Majeski et al. (2022), McDonald et al. (2021a, b) and Baral et al. (2022, 2023). This positions forage soybean as a reliable alternative for diversifying forage systems.
While late planting reduced DMY, it improved forage quality, evidenced by lower ADF, NDF, and lignin content, as well as higher IVDMD and RFQ. Lower ADF and NDF values are desirable as they correlate with higher digestibility and palatability, which can improve livestock overall performance (Ball et al., 2001). This trade-off between yield and quality suggests that farmers could prioritize late planting for high-value livestock feed or opt for mid-May planting to maximize biomass for silage. The stability of CP (19–21%) from V3 to R3, irrespective of planting date.
Research indicates that CP content in soybean is generally higher at R3-R4 stage compared to the R1-R2 stage because the R3 stage corresponds to the beginning of pod development (Kirnak et al., 2008). During this stage, the plant allocates more resources towards reproductive growth, including the synthesis of proteins necessary for pod and seed development (Fehr et al., 1971). This increased protein synthesis results in higher CP content in the plant tissues. This result is contrasts with findings in alfalfa, where CP declines sharply with maturity (Min, 2016; Baral, 2023). This stability offers flexibility in harvest timing without compromising protein content, a critical advantage for ensuring a consistent supply of high-quality forage, managing labor and equipment logistics. It can also reduce reliance on supplemental feeds, potentially lowering overall feeding costs. Additionally, the higher RFV and RFQ for late planting demonstrate the advantage of late-planted forage soybean in terms of its digestibility and potential palatability for livestock.
Furthermore, hay harvested from V2 to R3 met or exceeded USDA standards for high-quality hay (USDA-AMS, 2023), making it suitable for diverse livestock classes without compromising quality. Additionally, incorporating forage soybean into corn or sorghum silage improves its nutritive value due to presence of high protein content in forage soybean (Baghdadi et al., 2014; Ni et al., 2018). The enhanced protein levels make forage soybean an excellent option for meeting the dietary needs of livestock, particularly dairy cows that require high-quality feed.
WUE is another critical factor for forage production, especially in regions where water availability may be limited. The WUE of 20 kg ha-¹ mm-¹ aligns with Nielsen (2011), confirming forage soybean’s adaptability to water-limited environments. Its linear response to evapotranspiration suggests that forage soybean can maximize biomass production while utilizing available water resources effectively, making it a suitable crop for rainfed systems. Despite some variability, the trend indicates that higher evapotranspiration leads to greater forage yield, suggesting that sufficient water supply during the growing season can further enhance yield. Drought resilience and nitrogen-fixing capacity of forage soybean further enhance its competitiveness, particularly under rainfed conditions (Nielsen, 2011; Sheaffer and Seguin, 2003; Sinclair and Vadez, 2012). Moreover, Nadeem et al. (2019) found lower biomass and forage quality in low fertile soil with a pH of 6.8 compared to pH 6.0 and 5.1. This suggests that forage soybeans can perform well by producing better yield and quality forage even on acidic and low fertile soil.
The economic viability of forage soybean is equally important. The financial analysis of this study suggests that forage soybean production has the potential to contribute positively to farm profitability, as revenue exceeds costs, leading to a substantial net profit with a 32% return on investment. The economic viability of forage soybean hay production is supported by its ability to generate a profit after accounting for both fixed and variable costs and income tax. This indicates effective cost management, especially in terms of the relatively low fixed costs, which leave room for increased profitability as production scales up. A significant portion of the total costs in this analysis found variable costs such as seed costs, amount of fertilizer used, and the haying operation costs. These expenses fluctuate with production volume and management practices. Therefore, effectively managing these costs is crucial to maintaining profitability. A ROI of 32% indicates favorable economic returns, especially in the context of the agricultural sector, which is often subject to external risks such as market fluctuations and climatic conditions.
Furthermore, there are opportunities for increasing profitability by reducing variable costs without compromising production capacity. Strategic management of resources, such as optimizing labor and minimizing input waste, could lead to greater cost efficiency and higher profit margins. The economic assessment of hay produced from winter wheat, triticale, soybean, cowpea and forage soybean (Baral, 2023) has indicated that well-managed forage systems can yield competitive returns, supporting the inclusion of forage soybean in diversified cropping systems. These returns, coupled with lower input requirements and nitrogen-fixing capabilities, making it a sustainable alternative to traditional forages like alfalfa and sorghum-sudangrass, enhance its appeal for sustainable intensification.
Forage soybean’s ability to produce high yields, meet nutritional demands, efficiently utilize water, and provide a positive return on investment makes it a valuable addition to crop rotations in the Midwest region and the surroundings. However, adoption barriers remain, including limited awareness of forage soybean’s agronomic benefits and a lack of animal performance data. Future research should prioritize feeding trials to validate palatability and livestock productivity, as well as long-term studies on soil health impacts under diverse rotations. Addressing these knowledge gaps will support broader adoption across diverse farming systems.
Forage soybean exhibited tremendous potential as a summer forage crop in the U.S. Midwest, combining high dry matter yield (13.9 Mg ha-¹ at R3), stable forage quality, and drought resilience. This study demonstrated that forage soybean can serve as a reliable summer forage option for farmers in the Midwest, with planting date significantly influencing yield and nutritive value. Forage soybeans planted in mid-May and harvested at the R3 growth stage produced the highest dry matter yield (13.9 Mg ha-¹) without a significant decline in forage quality compared to those planted in early July. The overall forage quality remained stable across different growth stages (from V2 to R3), and late planting enhanced digestibility, offering farmers flexibility based on production goals.
Furthermore, forage soybean’s ability to meet the nutritional requirements of various livestock types throughout its vegetative and reproductive stages makes it an excellent choice for high quality hay and silage production. Its role in bridging seasonal forage shortages, combined with its strong dry matter yields, superior forage quality, and promising economic returns (32% ROI), makes it a viable option for enhancing forage systems, particularly in regions facing water constraints. The water use efficiency (20 kg ha-¹ mm-¹) further supports its integration into rainfed systems. To fully realize its potential, subsequent studies should focus on animal performance metrics and long-term soil health impacts.
Overall, forage soybean appears to offer a profitable, sustainable, and resilient forage option for the forage producers of Midwest region, contributing to improved forage systems and agricultural adaptability in water-limited environments.
The raw data supporting the conclusions of this article will be made available by the authors, without undue reservation.
RB: Conceptualization, Data curation, Formal analysis, Investigation, Methodology, Software, Visualization, Writing – original draft, Writing – review & editing. JK: Formal analysis, Visualization, Writing – review & editing. BB: Formal analysis, Visualization, Writing – review & editing. HK: Software, Visualization, Writing – review & editing. IM: Writing – review & editing. ED: Writing – review & editing. CG: Writing – review & editing. SC: Writing – review & editing. JR: Writing – review & editing. JO: Writing – review & editing. PH: Writing – review & editing. IC: Funding acquisition, Methodology, Project administration, Supervision, Validation, Writing – review & editing. CR: Funding acquisition, Methodology, Project administration, Validation, Writing – review & editing. DM: Conceptualization, Funding acquisition, Investigation, Methodology, Project administration, Supervision, Validation, Writing – review & editing.
The author(s) declare that financial support was received for the research and/or publication of this article. This research study was funded by USDA National Institute of Food and Agriculture, the Agriculture and Food Research Initiative’s Sustainable Agricultural Systems (AFRI SAS) (Grant no: 2019–68012-29888).
The authors gratefully acknowledge the resources and administrative support provided by the Department of Agronomy at Kansas State University. This work is a contribution (No. 23-317-J) from the Kansas Agricultural Experiment Station. The data used in this paper is adapted from the field research data generated by Baral (2023).
The authors declare that the research was conducted in the absence of any commercial or financial relationships that could be construed as a potential conflict of interest.
The author(s) declare that no Generative AI was used in the creation of this manuscript.
All claims expressed in this article are solely those of the authors and do not necessarily represent those of their affiliated organizations, or those of the publisher, the editors and the reviewers. Any product that may be evaluated in this article, or claim that may be made by its manufacturer, is not guaranteed or endorsed by the publisher.
The Supplementary Material for this article can be found online at: https://www.frontiersin.org/articles/10.3389/fagro.2025.1570567/full#supplementary-material
Allen R. G., Pereira L. S., Raes D., Smith M. (1998). Crop evapotranspiration-Guidelines for computing crop water requirements-FAO Irrigation and drainage paper 56 (Rome: FAO). Available online at: https://www.fao.org/4/x0490e/x0490e00.htm (Accessed January 15, 2025).
Anfinrud R., Cihacek L., Johnson B. L., Ji Y., Berti M. T. (2013). Sorghum and kenaf biomass yield and quality response to nitrogen fertilization in the Northern Great Plains of the USA. Ind. Crops Products 50, 159–165. doi: 10.1016/j.indcrop.2013.07.022
AOAC (2005). Amylase-treated neutral detergent fiber in feeds. Method 2002.04. Official methods of analysis. AOAC International. 49–55.
Baath G. S., Sarkar S., Sapkota B. R., Flynn K. C., Northup B. K., Gowda P. H. (2024). Forage yield and nutritive value of summer legumes as affected by row spacing and harvest timing. Farming System 2, 100069. doi: 10.1016/j.farsys.2023.100069
Baghdadi A., Halim R. A., Ghasemzadeh A., Ebrahimi M., Othman R., Yusof M. M. (2016). Effect of intercropping of corn and soybean on dry matter yield and nutritive value of forage corn. Legume Research-An Int. J. 39, 976–981. doi: 10.18805/lr.v39i6.6643
Baghdadi A., Halim R., Othman R., Martini M. (2014). Published. Increased Forage Protein through Corn and Legume Intercropping (International Agriculture Congress (IAC), 25–27.
Ball D. M., Collins M., Lacefield G. D., Martin N. P., Mertens D. A., Olson K. E., et al. (2001). Understanding forage quality Vol. 1 (American Farm Bureau Federation Publication), 1–15.
Baral R. B. (2023). Assessing yield, quality, water use efficiency and profitability of forage crops in rainfed agricultural management systems. Kansas State University, Manhattan Kansas, USA.
Baral R., Bhandari K., Kumar R., Min D. (2022). Yield gap analysis of alfalfa grown under rainfed condition in Kansas. Agronomy 12, 2190. doi: 10.3390/agronomy12092190
Baral R., Jagadish S. K., Hein N., Lollato R. P., Shanoyan A., Giri A. K., et al. (2023). Exploring the impact of soil water variability and varietal diversity on alfalfa yield, nutritional quality, and farm profitability. Grassland Res. 2, 266–278. doi: 10.1002/glr2.12067
Bastidas A., Setiyono T., Dobermann A., Cassman K. G., Elmore R. W., Graef G. L., et al. (2008). Soybean sowing date: The vegetative, reproductive, and agronomic impacts. Crop Sci. 48, 727–740. doi: 10.2135/cropsci2006.05.0292
Bateman N. R., Catchot A. L., Gore J., Cook D. R., Musser F. R., Irby J. T. (2020). Effects of planting date for soybean growth, development, and yield in the southern USA. Agronomy 10, 596. doi: 10.3390/agronomy10040596
Beck P. A., Coblentz W., Jennings J., Beck M. R. (2022). 198 using annual forage crops to extend grazing: what are the benefits to production and livestock enterprise economics. J. Anim. Sci. 100, 89–89. doi: 10.1093/jas/skac247.174
Biermacher J. T., Epplin F. M., Keim K. R. (2006). Cropping systems for the southern Great Plains of the United States as influenced by federal policy. Renewable Agric. Food Syst. 21, 77–83. doi: 10.1079/RAF2005119
Ciampitti I., Schapaugh W., Shoup D., Duncan S., Diaz D., Peterson D., et al. (2016). Soybean Production Handbook. K-State research and Extension (Kansas State University, Agriculture Experiment Station and Cooperative Extension Service). Available online at: https://bookstore.ksre.ksu.edu/pubs/c449.pdf (Accessed May 15, 2024).
Crusciol C. A. C., Mateus G., Nascente A., Martins P., Borghi E., Pariz C. (2012). An innovative crop–forage intercrop system: early cycle soybean cultivars and palisadegrass. Agron. J. 104, 1085–1095. doi: 10.2134/agronj2012.0002
Dhuyvetter K. C., Thompson C. R., Norwood C. A., Halvorson A. D. (1996). Economics of dryland cropping systems in the Great Plains: A review. J. Production Agric. 9, 216–222. doi: 10.2134/jpa1996.0216
Edwards J., Carver B., Horn G., Payton M. (2011). Impact of dual-purpose management on wheat grain yield. Crop Sci. 51, 2181–2185. doi: 10.2135/cropsci2011.01.0043
Entz M. H., Baron V. S., Carr P. M., Meyer D. W., Smith S. R. Jr., Mccaughey W. P. (2002). Potential of forages to diversify cropping systems in the Northern Great Plains. Agron. J. 94, 240–250. doi: 10.2134/agronj2002.2400
Fehr W. R., Caviness C. E., Burmood D. T., Pennington J. S. (1971). Stage of development descriptions for soybeans, Glycine Max (L.) Merrill 1. Crop Sci. 11, 929–931. doi: 10.2135/cropsci1971.0011183X001100060051x
French R., Schultz J. (1984). Water use efficiency of wheat in a Mediterranean-type environment. II. Some limitations to efficiency. Aust. J. Agric. Res. 35, 765–775. doi: 10.1071/AR9840765
Goering H. H., Van Soest J. (1970). Forage fiber analysis (apparatus, reagents, procedures and some applications). Agriculture Handbook 379 (Washington, DC, USA: United States Department of Agriculture).
Holman J. D., Assefa Y., Obour A. K. (2021). Cover-crop water use and productivity in the high plains wheat–fallow crop rotation. Crop Sci. 61, 1374–1385. doi: 10.1002/csc2.20365
Hu M., Wiatrak P. (2012). Effect of planting date on soybean growth, yield, and grain quality. Agron. J. 104, 785–790. doi: 10.2134/agronj2011.0382
Idowu J., Grover K., Marsalis M., Lauriault L. (2013). Reducing Harvest and Post-Harvest Losses of Alfalfa and Other Hay. New Mexico State University Circular-668, Cooperative Extension Service-College of Agricultural, Consumer and Environmental Sciences, Las Cruces NM, USA, 1–5.
Jahanzad E., Sadeghpour A., Hashemi M., Afshar R., Hosseini M., Barker A. (2016). Silage fermentation profile, chemical composition and economic evaluation of millet and soya bean grown in monocultures and as intercrops. Grassl. Sci. 71, 584–594. doi: 10.1111/gfs.2016.71.issue-4
Kansas Department of Agriculture (2022). Kansas custom rates 2022 (Department of Agriculture in cooperation with Kansas State University Land Use Survey). Available online at: https://www.agmanager.info/sites/default/files/pdf/2022_CustomRates_05-19-22.pdf (Accessed May 10, 2024).
Kansas Mesonet (2024). Historical weather (Manhattan Kansas: Kansas Msonet, Kansas State University). Available online at: http://mesonet.k-state.edu/weather/historical/ (Accessed June 06, 2024).
Kirnak H., Dogan E., Alpaslan M., Celik S., Boydak E., Copur O. (2008). Drought stress imposed at different reproductive stages influences growth, yield and seed composition of soybean. The Philippine Agricultural Scientist, 91 (3), 261–268.
MaChado L. A. Z., Cecato U., Comunello E., Concenço G., Ceccon G. (2017). Establishment of perennial forages intercropped with soybean for integrated crop-livestock systems. Pesquisa Agropecuária Bras. 52, 521–529. doi: 10.1590/s0100-204x2017000700006
Machicek J. A., Blaser B. C., Darapuneni M., Rhoades M. B. (2019). Harvesting regimes affect brown midrib sorghum-Sudangrass and brown midrib pearl millet forage production and quality. Agronomy 9, 416. doi: 10.3390/agronomy9080416
Majeski M., Noack R., Scianna J., Pokorny M. (2022). Sorghum-Sudangrass varietal production (Bridger, Montana: USDA-NRCS. Final Study Report. Bridger Plant Materials Center).
Marten G. C., Shenk J., Barton F. (1985). Near infrared reflectance spectroscopy (NIRS): Analysis of forage quality (US Department of Agriculture, Agricultural Research Service).
Mcdonald I., Baral R., Min D. (2021a). Effects of alfalfa and alfalfa-grass mixtures with nitrogen fertilization on dry matter yield and forage nutritive value. J. Anim. Sci. Technol. 63, 305. doi: 10.5187/jast.2021.e33
Mcdonald I., Min D., Baral R. (2021b). Effect of a fall cut on dry matter yield, nutritive value, and stand persistence of alfalfa. J. Anim. Sci. Technol. 63, 799. doi: 10.5187/jast.2021.e65
Min D. (2016). Effects of cutting interval between harvests on dry matter yield and nutritive value in alfalfa. Am. J. Plant Sci. 7, 1226. doi: 10.4236/ajps.2016.78118
Nadeem M., Pham T. H., Nieuwenhuis A., Ali W., Zaeem M., Ashiq W., et al. (2019). Adaptation strategies of forage soybeans cultivated on acidic soils under cool climate to produce high quality forage. Plant Sci. 283, 278–289. doi: 10.1016/j.plantsci.2019.03.014
Ni K., Zhao J., Zhu B., Su R., Pan Y., Ma J., et al. (2018). Assessing the fermentation quality and microbial community of the mixed silage of forage soybean with crop corn or sorghum. Bioresource Technol. 265, 563–567. doi: 10.1016/j.biortech.2018.05.097
Nielsen D. C. (2011). Forage soybean yield and quality response to water use. Field Crops Res. 124, 400–407. doi: 10.1016/j.fcr.2011.07.007
NOAA-NIDIS (2024). Data and maps: state drought information (National Oceanic and Atmospheric Administration, National Integrated Drought Information System). Available online at: https://www.drought.gov/states (Accessed January 23, 2024).
Nurbekov A., Jamoliddinov A., Joldoshev K., Rischkowskv B., Nishanov N., Rai K., et al. (2013). Potential of pearl millet as a forage crop in wheat-based double cropping system in Central Asia. J. SAT Agric. Res. 11, 1–5.
Orloff S., Mueller S. (2008). Harvesting, curing, and preservation of alfalfa. Irrigated alfalfa management in Mediterranean and Desert zones Vol. 8300 (Oakland CA, USA: University of California Agriculture and Natural Resources Publication).
Patrignani A., Godsey C. B., Ochsner T. E. (2019). No-Till diversified cropping systems for efficient allocation of precipitation in the Southern Great Plains. Agrosystems Geosciences Environ. 2, 1–8. doi: 10.2134/age2018.08.0026
Rao S., Mayeux H., Northup B. (2005). Performance of forage soybean in the southern Great Plains. Crop Sci. 45, 1973–1977. doi: 10.2135/cropsci2004.0598
Roozeboom K. L., Holman J., Shoup D., Blasi D. (2008). Nontraditional Forages as Emergency Or Supplemental Feedstuffs, Agricultural Experiment Station and Cooperative Extension Service (Manhattan KS, USA: Kansas State University Manhattan Kansas).
Schnitkey G., Paulson N., Zulauf C., Swanson K., Baltz J. (2022). Fertilizer Prices, Rates, and Costs for 2023 (Urbana IL, USA: farmdoc daily).
Sheaffer C. C., Orf J. H., Devine T. E., Jewett J. G. (2001). Yield and quality of forage soybean. Agron. J. 93, 99–106. doi: 10.2134/agronj2001.93199x
Sheaffer C. C., Seguin P. (2003). Forage legumes for sustainable cropping systems. J. Crop Production 8, 187–216. doi: 10.1300/J144v08n01_08
Sinclair T. R., Vadez V. (2012). The future of grain legumes in cropping systems. Crop Pasture Sci. 63, 501–512. doi: 10.1071/CP12128
Taylor E. G. (2014). Evaluation of forage soybean yield and quality characteristics and potential as a feed resource for developing replacement beef heifers (West Lafayette IN, USA: Purdue University).
Ullah H., Santiago-Arenas R., Ferdous Z., Attia A., Datta A. (2019). Improving water use efficiency, nitrogen use efficiency, and radiation use efficiency in field crops under drought stress: A review. Adv. Agron. 156, 109–157. doi: 10.1016/bs.agron.2019.02.002
Undersander D. (2001). Alfalfa yield and stand (Madison WI, USA: Department of Agronomy. University of Wisconsin-Extension).
USDA-AMS (2023). Kansas direct hay report (United States Department of Agriculture, Agricultural Market Service Livestock, Poultry & Grain Market News KS Dept of Ag Market News). Available online at: https://usda.library.cornell.edu/concern/publications/3j333228j?locale=enrelease-items (Accessed May 12, 2024).
USDA-ESMIS (2024). Acreage report (The U.S. Department of Agriculture, Economics, Statistics and Market Information System (USDA-ESMIS). Available online at: https://usda.library.cornell.edu/concern/publications/j098zb09z (Accessed January 20, 2025).
USDA-NASS (2022). Kansas 2022 farm real estate value and cash rent (United State Department of Agriculture, National Agricultural Statistics Service). Available online at: https://www.nass.usda.gov/Statistics_by_State/Kansas/Publications/Economic_Releases/Cash_Rents_and_Land_Values/2022/KS-crent2208.pdf (Accessed May 20, 2024).
USDA-NASS (2024). Data and statistics (United States Department of Agriculture, National Agricultural Statistics Service). Available online at: https://www.nass.usda.gov/Data_and_Statistics/index.php (Accessed January 20, 2025).
Keywords: forage soybean, rainfed cropping systems, dry matter yield, forage nutritive value, water use efficiency, net profit, return on investment
Citation: Baral R, Kim J, Bhattarai B, Koirala H, Massigoge I, Denson E, Guareschi C, Cominelli S, Rud JP, Bezerra de Oliveira J, Helguera PG, Ciampitti IA, Rice CW and Min D (2025) Cropping potential of forage soybean as a summer forage in Midwest U.S. rainfed systems. Front. Agron. 7:1570567. doi: 10.3389/fagro.2025.1570567
Received: 03 February 2025; Accepted: 17 March 2025;
Published: 16 April 2025.
Edited by:
Desouza Blaise, Central Institute for Cotton Research (ICAR), IndiaReviewed by:
Victor Idowu Olugbemiga Olowe, Federal University of Agriculture, Abeokuta, NigeriaCopyright © 2025 Baral, Kim, Bhattarai, Koirala, Massigoge, Denson, Guareschi, Cominelli, Rud, Bezerra de Oliveira, Helguera, Ciampitti, Rice and Min. This is an open-access article distributed under the terms of the Creative Commons Attribution License (CC BY). The use, distribution or reproduction in other forums is permitted, provided the original author(s) and the copyright owner(s) are credited and that the original publication in this journal is cited, in accordance with accepted academic practice. No use, distribution or reproduction is permitted which does not comply with these terms.
*Correspondence: Rudra Baral, cnVkcmFiYXJhbEBtaXNzb3VyaS5lZHU=; Doohong Min, ZG1pbkBrc3UuZWR1
Disclaimer: All claims expressed in this article are solely those of the authors and do not necessarily represent those of their affiliated organizations, or those of the publisher, the editors and the reviewers. Any product that may be evaluated in this article or claim that may be made by its manufacturer is not guaranteed or endorsed by the publisher.
Research integrity at Frontiers
Learn more about the work of our research integrity team to safeguard the quality of each article we publish.