- 1Laboratory of Plant Pathology, Department of Marine Sciences and Applied Biology, University of Alicante, Alicante, Spain
- 2Desertification Research Centre (CIDE, CSIC-UV-GV), Moncada, Valencia, Spain
- 3Research Institute of Water and Environmental Engineering (IIAMA), Universitat Politècnica de València, Valencia, Spain
Chitosan effects on soil properties were analysed both under laboratory conditions by incubation with constant humidity and temperature and under field conditions in two persimmon field plots with conventional and ecological management. Chitosan was applied in solution or as coacervates. Application of chitosan reduced soil pH, conductivity (CE), and cation exchange capacity (CEC) in pots when applied at field capacity. Chitosan did not affect field soil respiration, which is greatly dependent of soil moisture and temperature. Metabarcoding showed that chitosan significantly modifies the fungal genera composition of ecologically managed field soil. On the contrary, chitosan caused no significant differences in bacterial taxa composition of soil under field conditions. Chitosan coacervates increased naturally occurring nematophagous fungus Purpureocillium (ca. 50-fold) in soil with respect to chitosan solution-treated soil and untreated controls. In addition, chitosan reduced the inoculum of plant pathogenic fungi Alternaria and Fusarium (20% and 50%, respectively) in field soil. Soil microbial network analysis for ITS2+V1–V2 regions revealed that the nematophagous fungus Pochonia promoted network clustering into modules. Furthermore, network analysis for ITS2+V3–V4 regions showed that the nematode trapping-fungus Orbilia and bacteria belonging to Acidimicrobiales and Cytophagales significantly contributed to network clustering in field soil. Our results show that chitosan coacervates increased soil nematophagous microbiota and that both nematode egg parasites and trapping fungi help to structure soil microbiota.
1 Introduction
The use of chemical pesticides, imposed by demographic changes, is the most common strategy to improve agricultural productivity. However, there is a trend towards the use of ecological additives, such as chitosan, with low environmental impact, instead of chemical synthesis agrochemicals such as nematicides (Bautista-Baños et al., 2005; Lopez-Nuñez et al., 2022). Chitosan is also a source of nitrogen for stimulating plant growth (Pichyangkura and Chadchawan, 2015). The behaviour of chitosan in soil is related to its cationic nature. This allows electrical interactions with the negatively charged surfaces of clay minerals, modifying its behaviour in soil (Hataf et al., 2018).
Chitosan can modify some soil properties (Reddy et al., 2018). This biopolymer can act as a cohesive agent for clay particles (Hataf et al., 2018). Arid soils are often low on natural polysaccharides, which stabilise soil structure (Orts et al., 2000). Chitosan can bind metal ions and limit their leachability, even in the presence of K+, Cl−, and NO3−, the dominant ions in soil (Kamari et al., 2011). Furthermore, it can reduce the bioavailability of nickel (Turan, 2019; Heidari et al., 2020) and immobilises chromium when combined with other adsorbents (Najafi et al., 2021). Chitosan is a source of nitrogen, promoting plant growth (Pichyangkura and Chadchawan, 2015). Chitosan is also an elicitor of plant defences that can trigger physiological and structural responses in the plant, inducing jasmonic acid (JA) and salicylic acid (SA) production (Lopez-Moya et al., 2019; Suarez-Fernandez et al., 2020). Chitosan is active against plant pathogenic nematodes (Khalil and Badawy, 2012), has antiviral and antifungal activity, and induces tolerance to abiotic and biotic stresses in several horticultural crops (Iriti and Varoni, 2015; Malerba and Cerana, 2016).
Chitosan sensitivity of filamentous fungi and yeasts increases with carbon and nitrogen limitation (Lopez-Moya et al., 2015). Chitosan permeabilises the membrane of the fungus Neurospora crassa, in an energy-dependent manner. Conidia are most sensitive to chitosan membrane permeabilization followed by germlings and vegetative hyphae. Therefore, chitosan causes conidial lysis and death within minutes (Palma-Guerrero et al., 2009). Membrane fluidity is a key factor in fungal sensitivity to chitosan (Palma-Guerrero et al., 2010a; Zavala-González et al., 2016). Chitosan-sensitive fungi such as important plant pathogens (e.g., Fusarium spp. and Alternaria spp.) have a high content of polyunsaturated fatty acids (Ren et al., 2021; Chen et al., 2014). Plant diseases caused by species of the genus Fusarium consist of vascular wilts and consequent rotting of roots, stems, and the rest of the plant (Torres, 2000). Blight disease is one of the most dominant diseases causing an average yield loss of 32%–57% caused by the Alternaria genus (Mamgain et al., 2013). In contrast, chitosan-resistant fungi such as nematophagous (e.g., Pochonia chlamydosporia) or entomopathogens (e.g., Beauveria bassiana) have a lower presence of polyunsaturated fatty acids in membrane lipids. These fungi express, upon exposure to chitosan, extracellular hydrolytic enzymes (chitosanases, chitinases, and proteases) involved in nematode egg penetration. Furthermore, chitosan increases conidiation in nematophagous and entomopathogenic fungi (Palma-Guerrero et al., 2010b, 2010c).
Current work on chitosan biological activity of chitosan has focussed mostly on axenic systems. No data are available on the effect of chitosan on soil microbiota under natural conditions. Therefore, in this work, we studied the effect of chitosan on the abundance of ecological agriculture soil microbiota using metabarcoding and evaluated fungal and bacterial co-occurrence networks. The effects of chitosan solutions or coacervates on soil physicochemical properties were also studied both in the laboratory and in the field.
2 Materials and methods
2.1 Chitosan solutions and coacervates
Chitosan powder (Marine Bioproducts GmbH, Germany) was dissolved in 0.25 M HCl to obtain an initial concentration of 10 mg/mL, and pH was adjusted to 5.6. The resulting solution was then dialysed against distilled water for 2 days and autoclaved. Chitosan solutions were stored at 4°C until used for a maximum of 30 days. Control solutions were prepared likewise but without adding chitosan.
Chitosan was dissolved in sodium acetate buffer (pH 5) to obtain a 3% solution. Chitosan coacervates (T8C) were formed by dropping a 3% chitosan solution into 10% sodium hydroxide using a plastic syringe (Terumo Europe NV), with a 0.2-mm-diameter outlet. T8C were left for 5 min in the sodium hydroxide solution. T8C were then washed in sterile distilled water to reach pH 8. T8C were dried onto sterile filter paper in a laminar flow hood (Telstar BV-100) for 24 h. T8C were then stored at room temperature in sterile containers.
2.2 Application of chitosan to agricultural field soil
Persimmon fields in Pedralba (Valencia, E, Spain), conventionally (39° 35′ 55.25′′ N, 0° 43′ 47.31 W) and ecologically (39° 35′ 52.47′′ N, 0° 43′ 41.47 W) farmed, were selected for experiments (Table 1). Soil properties were determined; soil was air-dried soil and sieved through a 2-mm sieve. Soil pH was measured in a 1:2.5 (w/v) aqueous solution using a pH meter (2001, Crison, Barcelona, Spain). Electrical conductivity was determined in a 1:5 (w/v) aqueous solution using a conductivity meter (model, Crison). The carbonate content was determined using a Bernard calcimeter. Soil organic matter (OM) was determined by wet oxidation using the Walkley–Black titration method (Walkley and Black, 1934). Soil texture was determined by the Bouyoucos method (Bouyoucos, 1927). Surface soil (0 cm–10 cm) from both plots was taken for the incubation experiment with pots in growth chambers. Also, these plots were used for an experiment of chitosan application in the field where two treatments were selected: coacervates, only one application at the beginning of the experiment and soluble chitosan applied monthly along 9 months with the dose divided between the number of applications.
2.2.1 Field experiments
Three 1 × 1 m plots were marked in each field (Figure 1). Each plot was subdivided into six 33 × 50 cm subplots. Three subplots per plot were randomly selected for treatments. These included Control (C) (no Chitosan), 1 mg/mL Chitosan solution (T8L), and Chitosan coacervates (T8C). Selected T8C subplots were treated with chitosan coacervates (9 g/subplot) at the start of the experiment. C and T8L subplots were irrigated monthly (1 L/subplot) for 9 months with either distilled water (C and T8C) or 1 mg/mL chitosan (T8L). Field soil moisture, temperature, and electrical conductivity were measured monthly (for nine months) using a WET-2 sensor (HH2 Moisture Meter, Delta-T Devices, Burwell, UK). Respiration rate and CO2 concentration were also measured monthly (for 9 months) using an EGM-4 environmental gas monitor device (PP System Company, Amesbury, MA, USA). At the end of the experiment, four core samples were collected from each treated subplot with a cylindrical auger (5.35 cm in diameter and 12.77 cm in length). Soil cores were placed in 15 × 20 cm sterile airtight bags. Soil subsamples (10 g) were sieved through a 2-mm mesh and then air dried to measure cation exchange capacity, pH, soil moisture (see below), and mineral nitrogen. Soil mineral nitrogen (nitrate and ammonium) was extracted in 2 M KCl and analysed colorimetrically by flow injection (FIAstar 5000, Foss Tecator, Höganäs, Sweden) (Rhoades, 1982). Cation exchange capacity was determined by the sodium acetate sodium chloride method (Rhoades, 1982).
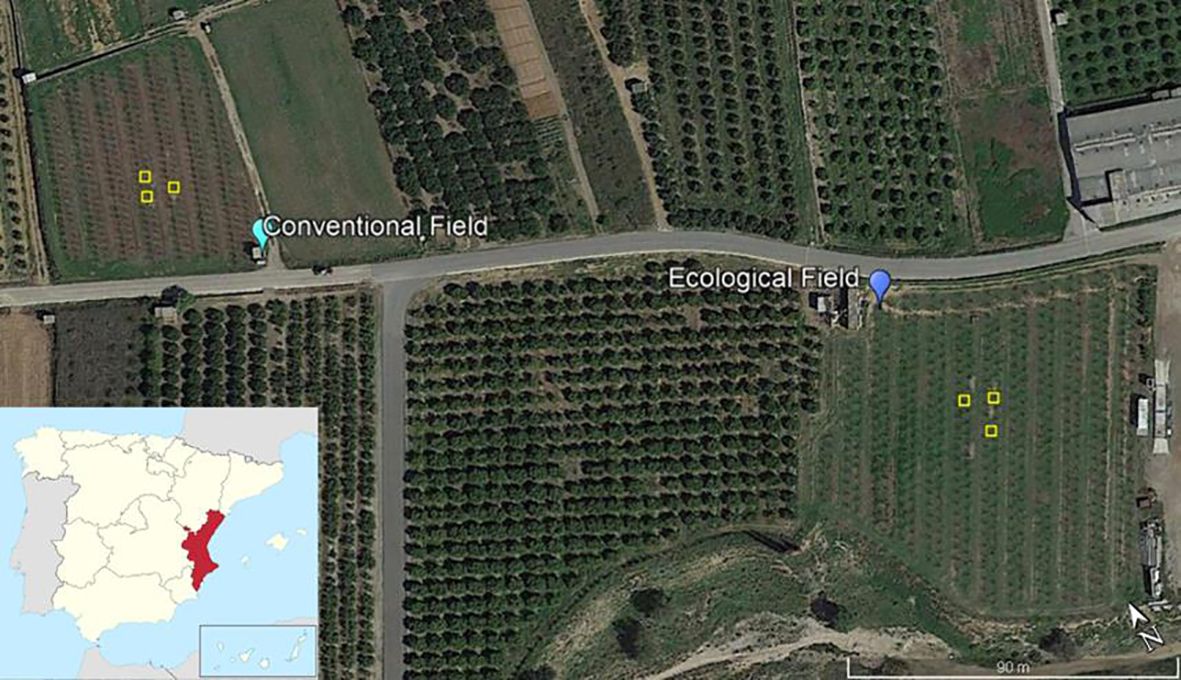
Figure 1. Persimmon experimental fields. Fields were in Pedralba, Valencian Community (East, Spain). Conventional Field received mineral fertilisation and usual agronomic practices. Ecological Field received organic fertilisation only, and no agrochemicals were applied. Experimental plots (1 × 1 m) where treatments were applied, and soil samples collected are marked by yellow boxes. Google Earth (2024). https://www.google.com/earth/.
2.2.2 Laboratory trials
Polystyrene cups (200 mL) with a hole in the base covered with glass wool were filled with soil collected from each of the Pedralba plots. Cups were incubated in a growth chamber (SANYO, MLR-351H) at 24°C and 60% relative humidity under a 16-h light/8-h dark photoperiod. Cups with soil were irrigated periodically (2–3 days) to maintain soil moisture to field capacity according to the texture of each soil (see below). There were 10 replicate pots set per soil (conventional and ecological management) and treatment: Control (C) (no Chitosan), at 1 mg/mL Chitosan solution (T8L), and Chitosan coacervates (T8C) 1 g/plot.
For the determination of the moisture of each soil at field capacity, we placed 12.5 cm of soil in a 15.5-cm-long and 3.5-cm-wide percolation tube. Water was then added to wet the first 5 cm of soil. The top of the tube was capped with Parafilm® and aluminium foil, leaving the tap open for 48 h–72 h. We then discarded the first centimetre of soil, took a sample of moist soil, and weighed it. We dried the soil at 105°C to constant weight. We calculated soil field capacity with the formula described in Llorca-Llorca (1991):
Soil Moisture at Field capacity = (Moist Soil Weight – Dry Soil Weight)/Dry Soil Weight
After 30 days, the soil from three pots per soil type and treatment was pooled and homogenised per triplicate (nine pots sampled). Then, soil humidity, pH, electrical conductivity, and cation exchange capacity were analysed. This experiment was carried out in duplicate.
2.3 Physicochemical analysis of soils
Soil samples for both regimes (ecological and conventional) were taken from each subplot and treatment for physicochemical determinations at the end of the experiment. Soil moisture, conductivity/salinity, pH, texture, and cation exchange capacity were determined for all soil samples collected (Llorca-Llorca, 1991). Three measurements were taken per each physicochemical parameter for treatment and soil type.
2.4 Soil metabarcoding
On the same day of collection (only for ecological soil), DNA was extracted from fresh soil (250 mg per soil sample), using DNeasy PowerSoil Pro Kit (Qiagen, Germantown, MD, USA). DNA samples were sent to Macrogen Inc. (Seoul, Korea), where they were amplified and using specific fungi (ITS2) and bacteria (V1–V2, V3–V4) primers (Table 2) and sequenced by the Illumina MiSeq platform using the v3 reagent kit. DNA reads obtained were analysed using the OmicsBox 3.0 package to identify the microorganisms present in soil samples. Metabarcoding data are available in the NCBI BioProject accession number PRJNA1164777 (https://www.ncbi.nlm.nih.gov/sra/PRJNA1164777).
2.5 Soil microbe co-occurrence networks
Fungal and bacterial communities characterised from ITS2, V1–V2, and V3–V4 amplicon sequencing were analysed through co-occurrence networks by using the SParse InversE Covariance Estimation for Ecological Association Inference (SPIEC.EASI) pipeline in R package (Kurtz et al., 2015). This network-based approach allowed to frame both fungal and bacterial communities into a similar co-occurrence network (Wagg et al., 2019). Before network inferring, OTUs that occurred >1% and more than five samples were maintained in the datasets and rescaled to the proportion of the minimum sequencing depth (32,672 reads for fungi in the ITS2 dataset, 38,709 for bacteria in the V1–V2 and 38,011 for bacteria in the V3–V4). The inference was carried out by combining the amplicon pair dataset, ITS2+V1–V2 and ITS2+V3–V4. We fitted the spiec.easi function with Meinshausen–Bühlmann’s neighbourhood selection method, and the lambda minimum ratio at 0.01. From the spiec.easi object, we extracted the OTU adjacency matrix with the symBeta function to infer the network graphs and network properties of OTUS from the Gephi software (Bastian et al., 2009). In particular, we determined the degree centrality, which counts the number of links per OTU and its metric weighted by the occurrence frequency per linked OTU pairs (Gouveia et al., 2021); the modularity class for each OTU embedded in the network, i.e., the module which an OTU belong to; and the clustering coefficient, which measures the extent of an OTU to cluster with others into a module (Latapy, 2008).
2.6 Statistical analysis
Results from pot tests were analysed with a three-way ANOVA to determine statistical differences for each variable tested (pH, electrical conductivity, cation exchange capacity), with the factors soil, treatment (fixed and orthogonal), and experiment (random and orthogonal) at the end of the experiment (30 days).
For the field test variables (pH, conductivity, cation exchange capacity, and mineral nitrogen), a two-way ANOVA of soil and treatment (fixed and orthogonal) was performed for the last data collection time of the field experiment (9 months).
Then, a three-way ANOVA was performed to analyse the differences of each variable (respiration rate, electrical conductivity, soil moisture, and soil temperature), with the factors soil, treatment, and time (fixed and orthogonal). The ANOVA requirements were tested with the DHARMa package (Hartig, 2022).
For the ecological soil metabarcoding analysis, the OmicsBox 3.0 program was used to obtain relative abundances of phylum, order, genus, and species for the ITS2, V1–V2, and V3–V4 primers, with the Kraken 2.1.2 function (Wood et al., 2019; Wood and Salzberg, 2014). Abundances above 1% (relative abundance) were taken for statistical analyses. The mean relative abundance and standard error were calculated with Excel.
To study the differences of phylum, genus, order, and species present in the ecological soil according to treatment, a multivariate generalised linear model (GLM) with a Gaussian distribution of the error (“manyglm” function in the “mvabund” package) was performed. A univariate GLM with a Gaussian family error distribution was then performed for each variable to analyse the differences between abundances in genera and species for ITS primers. Treatment was considered as a predictor variable in the analysis. We conducted pairwise comparisons with estimated marginal means (“emmeans” function and package; Lenth et al., 2023) using Sidak’s HSD test for GLM data.
The effect of taxonomy on network metrics was assessed by fitting linear regression models for each amplicon pair data set, ITS2+V1–V2 and ITS2+V3–V4. A t-test was performed on the estimated values to detect taxa that significantly explained the results of the network metrics.
All statistical analyses were performed with R software (version 4.2.2) (R Core Team, 2023).
3 Results
3.1 Chitosan reduced potted soil pH conductivity and cation exchange capacity but not under field conditions
Chitosan solutions significantly reduced soil pH (ANOVA; p value=0.001) (Figures 2A, B; Supplementary Table S1) and electrical conductivity (EC) (ANOVA; p value=0.04) (Figures 2C, D, Supplementary Table S3) when water content was maintained at field capacity in the pot experiment. Both chitosan solutions and coacervates reduced soil cation exchange capacity (CEC) with respect to controls in the pot experiment (ANOVA; p value=0.03) (Figures 2E, F, Supplementary Table S5). However, under field conditions when applied monthly, chitosan did not alter field soil pH, EC, and CEC (ANOVA; p value = 0.5, p value= 0.3, p value= 0.1; Figures 2B, D, F; Supplementary Tables S2, S4, S6). In the field experiment, soil EC was lower for March–July than for November–February recordings for both soil managements (Supplementary Figure S1). In June and July, in the organic soil, conductivity could not be recorded because of low soil humidity for high temperatures and low rainfall (Supplementary Figures S2, S3). Chitosan application to field soil had no significant effect on soil mineral nitrogen content (Supplementary Figure S4).
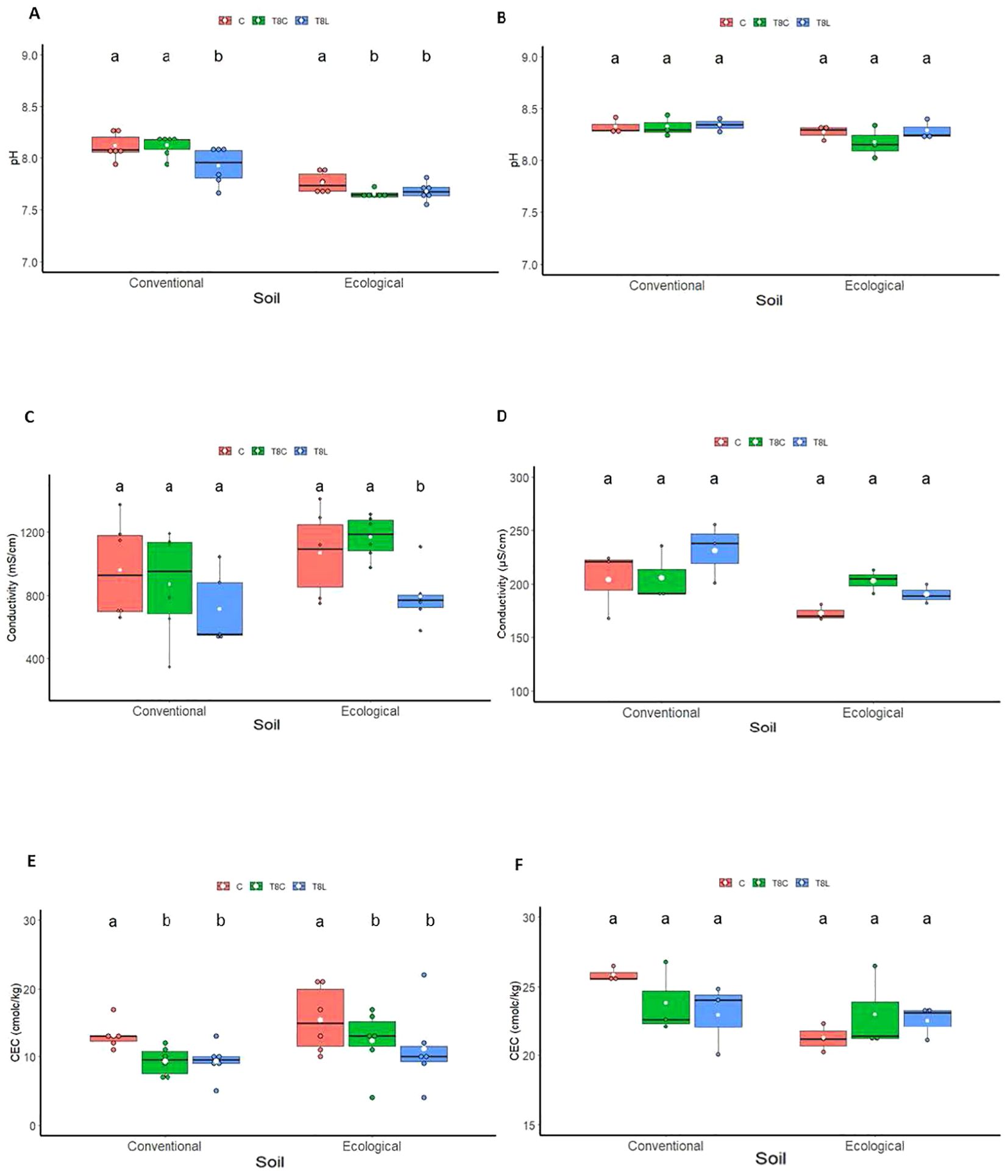
Figure 2. Effect of chitosan on soil chemical properties: soil pH (A, B), conductivity (C, D) and cation exchange capacity (E, F). Treatments: control [(C), untreated], chitosan coacervates (T8C), and chitosan solution (T8L). Experiments: growth chambers (A, C, E), field (B, D, F). Lowercase letters indicate significant differences between treatments for each soil type.
3.2 Chitosan did not affect field soil respiration under field conditions
A trend of increased respiration was observed in the chitosan treatments mainly from March to June for the conventional field soil (Figure 3A), and from March and May for the ecological field soil (Figure 3B). Irrespective of treatments, field soil respiration significantly (ANOVA; p-value > 0.001, Supplementary Table S7) increased in both management regimes (conventional and ecological) from March until July. This period corresponds with a steady significant increase in soil temperature for both conventional and ecological regimes (Supplementary Figures S2A, B). Soil moisture increased in the March recording (Supplementary Figures S2C, D). This corresponded, in turn, with an increase in precipitation and temperature (Supplementary Figure S3).
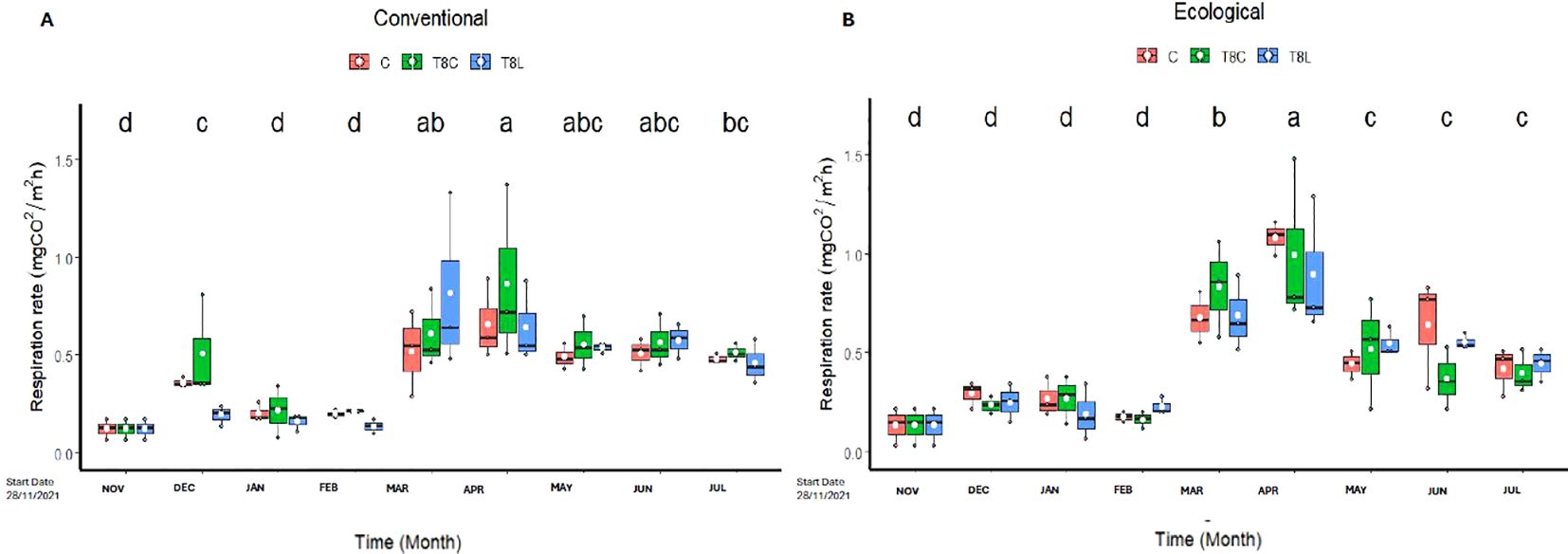
Figure 3. Effect of chitosan on field soil respiration. Soil was under conventional (A), or ecological (B) regimes. Treatments: field (C, untreated), chitosan coacervates (T8C), and chitosan solution (T8L). Lowercase letters show significant differences between the different times. Level of significant differences p-value<0.05.
3.3 Chitosan modified soil mycobiota by reducing naturally occurring plant pathogenic fungi in soil under field conditions
Chitosan significantly (multivariate GLM, p value 0.001, Supplementary Table S8) modified fungal genera composition of ecological field soil (Figures 4A, B, 5A). Conversely, chitosan caused no significant differences in bacterial taxa composition of the same soil respect to untreated controls (multivariate GLM, p value > 0.001; Figure 5B). The fungus Fusarium was the fungal genus most present (33%–23%) in field samples (Figure 5A), followed by Lachnellula (22%–13%), Wickerhamiella (17%–14%), and Filobasidium (11%–7%) (Supplementary Table S9). Other genera, including Alternaria, showed 5% or less relative abundance (Figure 5A). Chitosan coacervates tended to reduce the relative abundance of Fusarium and Alternaria, although no significant differences were found. Presence of the plant pathogenic species Fusarium falciforme (50% reduction, Supplementary Table S10) in soil was significantly reduced (univariate GLM, p.value = 0.03, Supplementary Table S11), by chitosan solution (Figure 4B). Chitosan coacervates significantly reduced (univariate GLM, p value = 0.01, Supplementary Table S11) the relative abundance of the phytopathogenic species Alternaria atra (20% reduction, Supplementary Table S10), with respect to untreated controls.
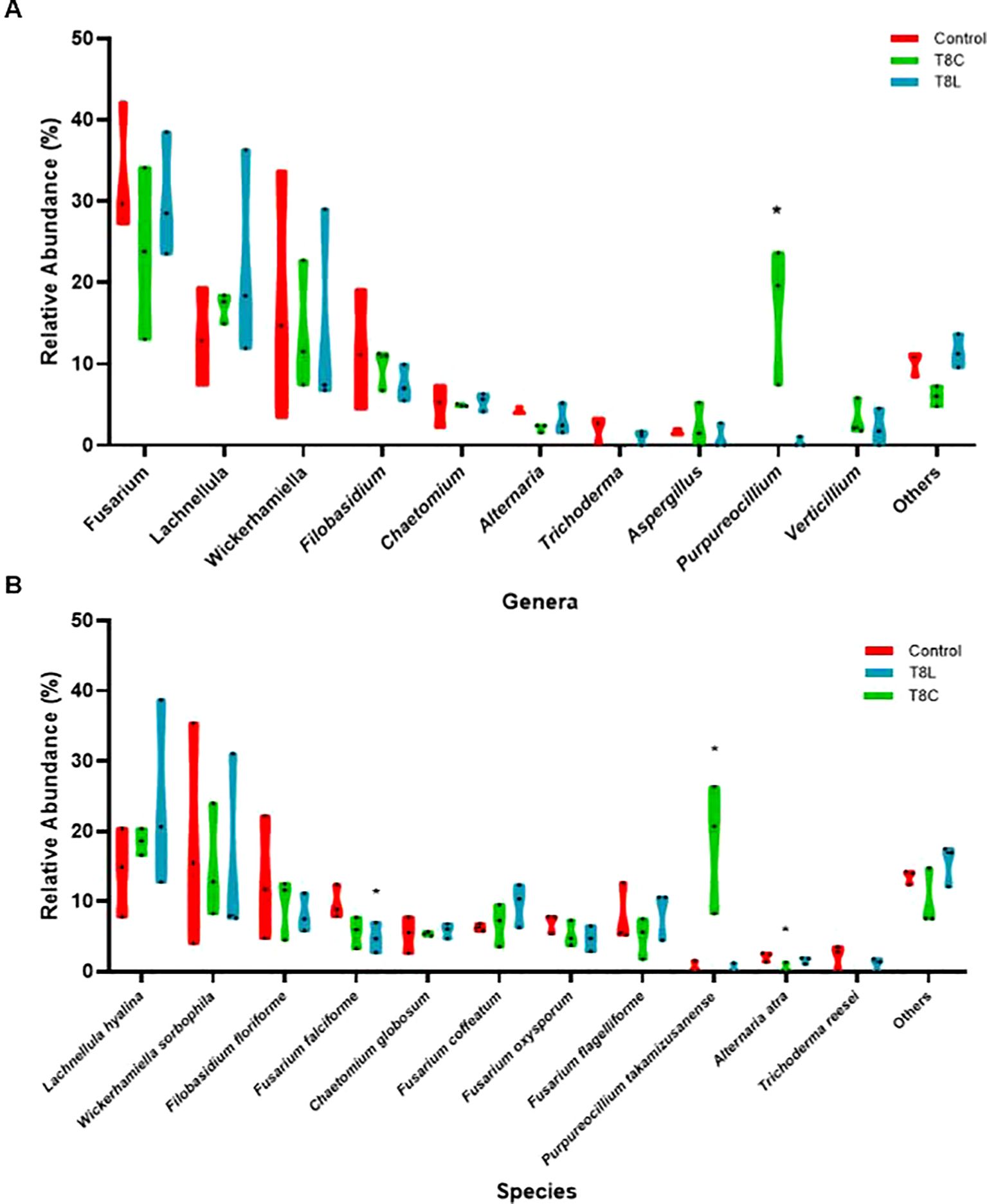
Figure 4. Relative abundance (%). Asterisks mark significant differences (p value < 0.005), of the treatments with respect to the control for each genus (A) and species (B).
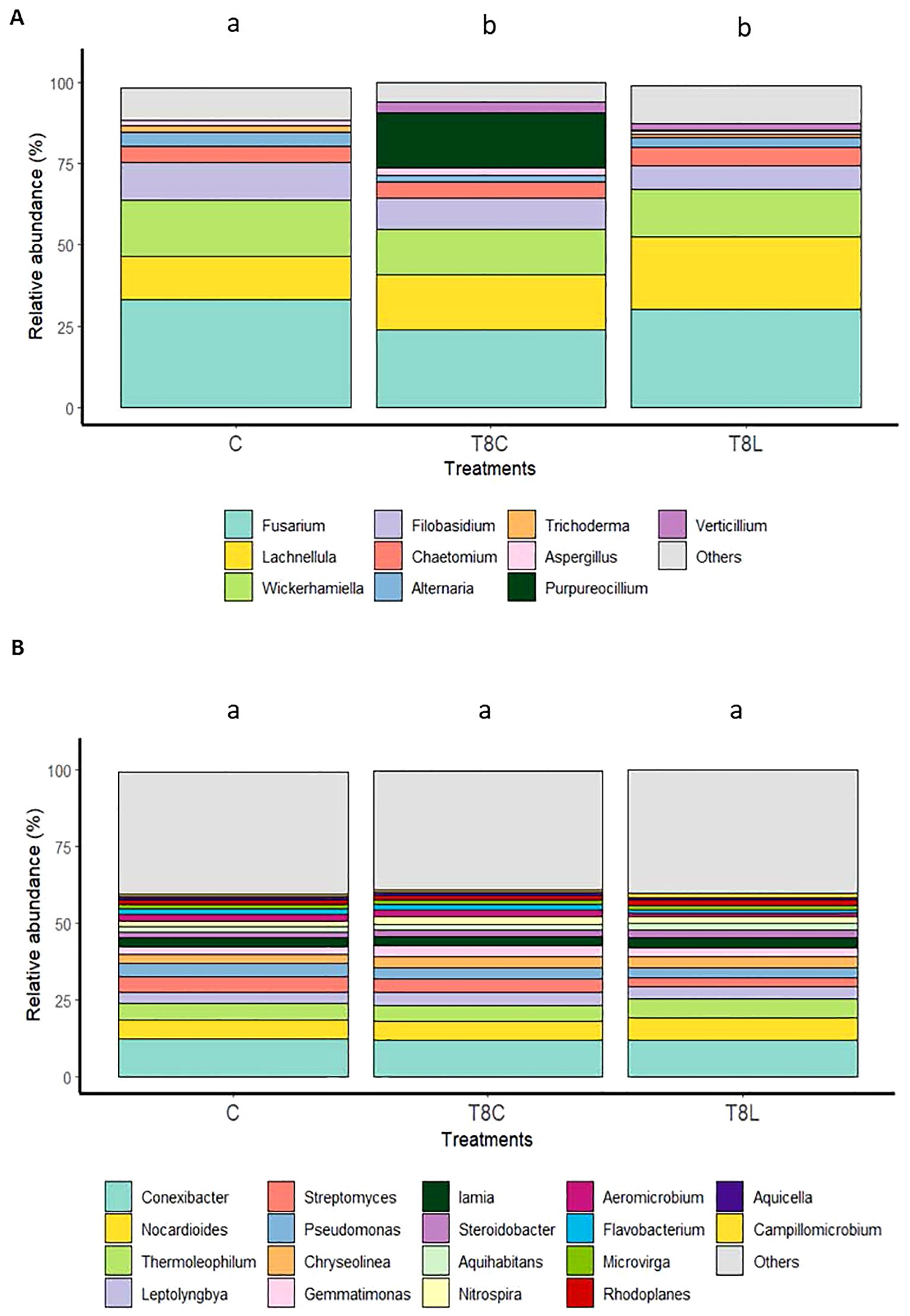
Figure 5. Effect of chitosan on field soil microbiota. (A) Fungal genera (ITS primers) and (B) bacterial genera (V1–V2 primers). Treatments: field (C, untreated), chitosan coacervates (T8C), and chitosan solution (T8L). Different letters indicate significant differences (p-value < 0.05).
3.4 Chitosan coacervates increased naturally occurring nematophagous fungus Purpureocillium in soil under field conditions
Chitosan coacervates significantly (univariant GLM, p value = 0.006, Supplementary Table S12) increased (ca. 50-fold) naturally occurring nematophagous fungus Purpureocillium in field soil (Figure 5A). Significant differences were found for the variable fungal species relative abundance (multivariate GLM, p value = 0.044; Supplementary Figure S5, Supplementary Table S13) between control and chitosan coacervate treatments. Chitosan coacervates significantly increase (ca. 3,500%) the presence of the invertebrate pathogen Purpureocillium takamizusanense in soil (univariate GLM, p = 0.006, Figure 5B; Supplementary Table S11).
3.5 Nematophagous fungi and structure of soil microbiota
The use of ITS2+V1–V2 and ITS2+V3–V4 regions revealed variations in the co-occurrence network outcomes (Figure 6; Supplementary Tables S14, S15). However, we showed that the weighted degree centrality (WDC) parameter could not allow to detect contrasting influence of microbial groups within the network of each amplified region in the ITS2+V3–V4 subset, only marginally detected in bacteria that belonged to Acidimicrobiales (Figure 6A).
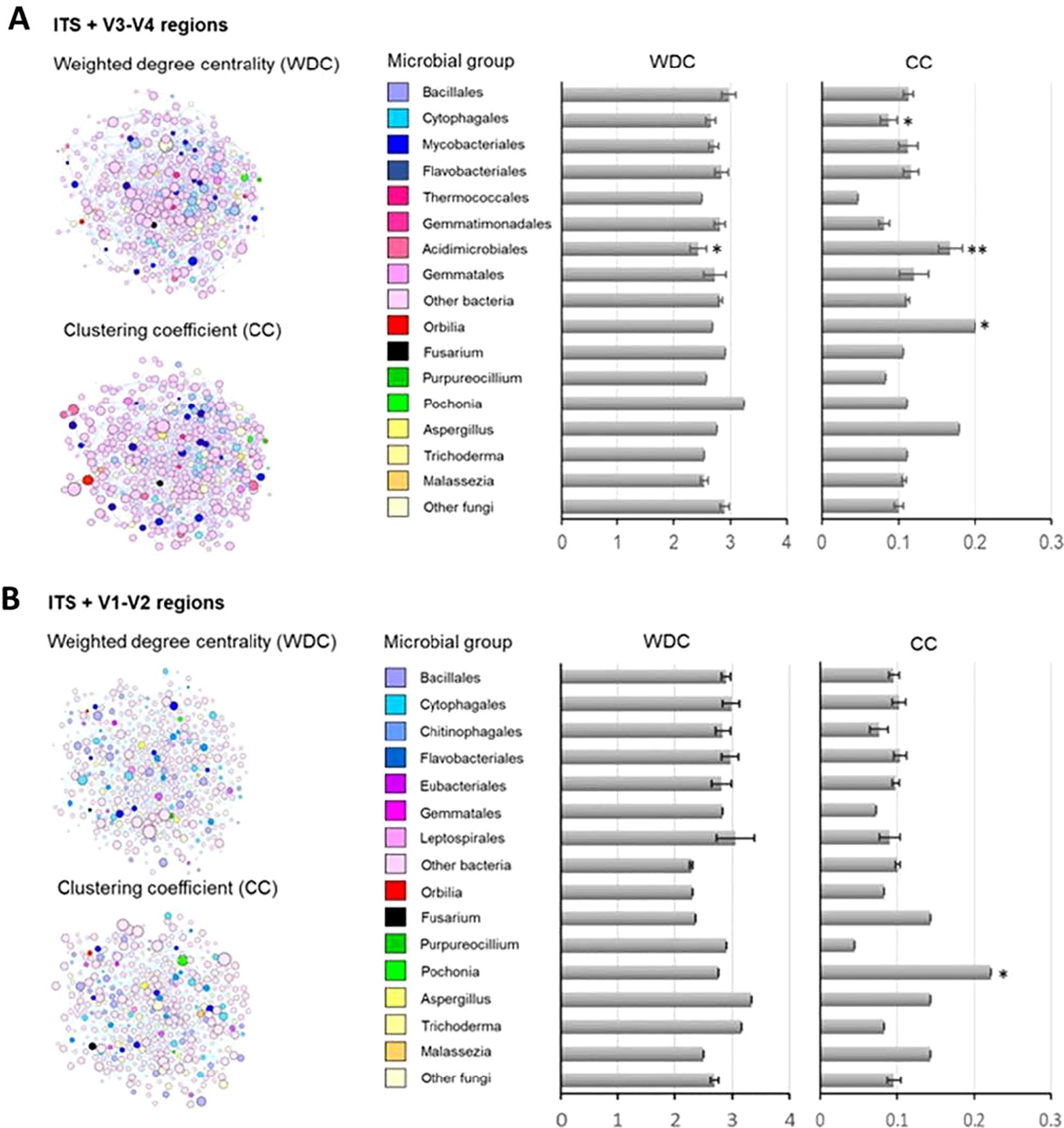
Figure 6. Microbial co-occurrence networks by amplified region pairs, ITS (fungi) and V1–V2 (A) / V3–V4 (B) (bacteria) in soils. Networks were inferred from SPIEC.EASI R package. Graphs and network metric-weighted degree centrality (WDC), i.e., the interaction frequency between OTU pairs, and clustering coefficient (CC), i.e., the extent of an OTU to cluster with others into modules, were determined in Gephi software. WDC and CC values are also shown in bar graphs by microbial group, bacteria, and fungi at order and genus taxonomic levels, respectively. The effects of microbial taxa identity on the network metrics were evaluated by linear models and t-tested, and those significantly explaining WDC and CC are marked by the significance level: **p < 0.01, *p < 0.05.
The clustering coefficient (CC) parameter for ITS2+V1–V2 regions showed that the nematode egg-parasitic fungi Pochonia (CC = 0.22) promoting network clustering into modules (n = 15 modules) (Figure 6B; Supplementary Table S16). By evaluating the co-occurrence results, we detected Pochonia chlamydosporia with a positive interaction to xylan-degrading (Humisphaera), N-fixing (Leptolyngbya), and sulphate-reducing bacteria (Rubrobacter) (Supplementary Table S18). Furthermore, we detected antagonistic interactions with soil bacteria such as Aquihabitans spp., a Gram-negative bacteria, Leptolyngbya spp., a worldwide distributed cyanobacteria, and Proteatibacter spp., a widely distributed soil bacteria (Supplementary Table S18). The ITS2+V3–V4 regions showed that the nematode-trapping fungi Orbilia (CC = 0.20) and the Order Acidimicrobiales (CC = 0.17 ± 0.02) and Cytophagales (CC = 0.04 ± 0.01) significantly contributed to network clustering into modules (n = 29) (Figure 5B; Supplementary Table S17). Orbilia oligospora showed synergistic co-occurrence with a wide group of soil bacteria (Nakamurella spp., Nocardioides spp., or Vulgatibacter spp.). By the other side, O. oligospora showed a competitive behaviour with important soil borne fungal pathogens like Talaromyces spp. and Aspergillus spp. species (Supplementary Table S19).
Acidimicrobium ferrooxidans, an extremophile bacteria able to grow under extremely low-pH conditions (pH <2), showed positive interactions with soil-living bacteria such as Massilia spp., Nitrospira spp., or Stella spp. However, this bacterium had an antagonistic effect on Jiangella spp., Hymenobacter spp., and Limnoglobus spp. bacteria present in crop soils. Inside of the Cytophagales, the species Cytophaga hutchinsonii showed positive interactions with many soil-born bacteria (Calothrix spp., Chitinophaga spp., or Lysobacter spp.). Furthermore, C. hutchinsonii revealed negative associations with important soil fungal pathogens like Fusarium oxysporum and Verticillium dahliae.
4 Discussion
Chitosan applied maintaining soil water content at field capacity in pots for a month significantly reduced soil pH, CE, and CEC. The slight reduction of pH in the soil induced by chitosan could be simply due to the weak acidity of chitosan solutions. This effect was not found under field conditions. This was perhaps by the lower volumes of chitosan solutions applied monthly. The high calcium carbonate content of both soils could neutralise the chitosan solutions. The reduction of soil CE by chitosan in pots could be associated with the mopping capacity of chitosan (polycation) of ions present in the soil solution (Kamari et al., 2011). Chitosan solutions and coacervates reduced soil cation exchange capacity (CEC) with respect to controls for potted soils. For example, when applied to sodium montmorillonite, chitosan intercalates in the layers of the clay (Darder et al., 2003), both reducing the negative charges for cation exchange and immobilising chitosan in soils. In our pot study, applying this chitosan may have displaced exchangeable cations from the clay complex, thus reducing CEC. However, this was not found when chitosan was applied monthly in the field. The regime of chitosan irrigation (field capacity vs. monthly applications) could account for a lower chitosan presence in field soil than in the pots. This may have made the chitosan displacement of cations of the clay complex in field soil less efficient than in pots. Taken together, our results suggest that chitosan can be applied to agricultural fields without affecting CEC, a key parameter for soil fertility (Anderson et al., 2023).
Undissolved chitosan added to soil (5% w/w) caused N increase (ammonium and nitrogen), with respect to untreated controls in previous microcosm experiments (Sawaguchi et al., 2015). In our study, chitosan application to field soil had no significant effect on soil mineral nitrogen content due mainly to the high mobility of mineral in soils. Our treatments also involved less chitosan applied to soil than in the microcosm. This, and the time lapse (9 months) for N soil content testing, may explain our results. In soil incubation experiments with chitosan, soil respiration was found to increase with chitosan concentration (Nkoh et al., 2024). In our field study, chitosan treatments resulted in increases in soil respiration, especially during spring–midsummer. This effect, although not significant, could be related to eventual organic N input when chitosan was added to our microplots.
Our metabarcoding study shows that chitosan significantly modifies fungal genera composition of ecological field soil. Chitosan coacervates increase naturally occurring nematophagous fungus Purpureocillium in soil with respect to chitosan solution treated soil and untreated controls. Chitosan increases by ca. 6,000% conidiation of Purpureocillium (Palma-Guerrero et al., 2010c) cultures with respect to control media with no chitosan. The similar increase (ca. 50-fold) in the relative abundance of Purpureocillium spp. found in this work could be due to chitosan induction for conidiation of the fungi naturally occurring in soil. The highly sporulating capacity of this chitosan-tolerant fungal genus could explain our results (Gortari and Hour, 2016). Indeed, Purpureocillium lilacinum was previously applied combined with chitosan promoting managing effects on root knot nematodes (Giannakou et al., 2020; Zhan et al., 2021). In our study, we find the species Purpureocillium takamizusanense, which has been also isolated as an entomopathogenic fungus (Nguyen et al., 2022). Future studies should evaluate the effect of chitosan on the performance of this fungus in the field for insect/nematode pest biomanagement. These studies should include augmentative natural biocontrol and enhanced biocontrol with inundative or sustained additions of inoculum of the fungus. Furthermore, chitosan particles should be used in these studies, since chitosan solutions did not enhance naturally occurring Purpureocillium on soil.
We also found that the abundance of Alternaria atra and Fusarium falciforme decreased in soil treated with a chitosan solution with respect to control soil. Chitosan accumulates in the cell wall of non-chitinolytic fungi, thus preventing their growth (Muzzarelli et al., 1986). However, the plasma membrane is the main target of chitosan (Lopez-Moya et al., 2019). Chitosan-sensitive fungi, e.g., Fusarium, have fluid membranes with respect to chitosan-resistant fungi such as Purpureocillium. These two fungal species cause diseases in several crops worldwide (Bonthala et al., 2021; Trolinger et al., 2017). Therefore, soil treatment with chitosan could be a sustainable alternative for managing these fungal plant pathogens. Furthermore, our co-occurrence network analyses show that Purpureocillium spp. negatively related to Alternaria atra and A. rosae (Supplementary Table S18). Purpureocillium spp. are fungi well known to produce antimicrobial secondary metabolites (Chen and Hu, 2021). Future studies should investigate the mechanisms involved in the antagonism of Purpureocillium spp. to Alternaria spp. in soil.
Metagenomics on soil exposed to chitin-rich exoskeletons has been a source of gene sequences encoding chitin–chitosan-degrading enzymes (Li et al., 2015; Stöveken et al., 2015). Most of these sequences were of bacterial origin. Our metabarcoding analysis shows that chitosan application during 9 months to field soil did not change bacterial taxa profiles. Perhaps time of exposure to chitin/chitosan could account for these differences.
We have carried out a microbial diversity and ecological network analysis (Barberán et al., 2012). Our results show that the two main ecological groups of nematode-destroying fungi (Barron, 1997), nematode trapping (Orbilia spp.) and egg parasites (Pochonia spp.), promote soil microbe network clustering into modules. Nematophagous fungi interact with nematodes, the most abundant animal taxon in soil (Dervash et al., 2018). Since most soil nematodes are bacterivorous (De Mesel et al., 2004), it was expected that nematophagous fungi were also related to soil bacteria. Indeed, our co-occurrence network analyses show that the nematode egg parasite fungus Pochonia positively related to xylan-degrading (Humisphaera), N-fixing (Leptolyngbya), and sulphate-reducing bacteria (Rubrobacter). These soil prokaryotes could help with nutrient acquisition by the fungus. Nocardioides, a hydrocarbon degrader, antibiofilm and antibiotic producer filamentous bacterium, is negatively correlated with Pochonia and positively with Orbilia. This and other bacteria (Paraflavitalea, Chitinophagaceae), also positively related with Orbilia, can degrade chitin in soil. Root nodule bacteria (Microvirga and Botea) are positively correlated with the nematode trapping fungus. Pochonia can show endophytic lifestyle in crop plants and can be beneficial for plant defence against soil-borne pathogens (Manzanilla-Lopez et al., 2013). Nematode egg fungal parasites are multitrophic organisms than can be enhanced by chitosan (Escudero et al., 2016; 2017). In this work, we find that chitosan application in soil enhances P. lilacinum recruitment and the promotion of P. chlamydosporia as key fungi to structure microbial communities in soil. Bacteria belonging to Acidimicrobiales and Cytophagales also significantly contributed to network clustering in field soil. These groups are documented to act on iron redox-related processes (Garber et al., 2021) and carbohydrate polymer (chitin, pectin, cellulose) turnover (Mohapatra et al., 2022) in soil, respectively. They play a key role in recruiting soil-borne bacteria essential to maintaining soil health. In addition, we show that C. hutchinsonii is an antagonistic microorganism against two important plant pathogenic fungi such as V. dahliae and F. oxysporum (Kausar et al., 2021).
In conclusion, this work has shown that chitosan in the form of coacervates increases the abundance of Purpureocillium in soil (ca. 50-fold). Nematophagous fungi, both egg parasites (Pochonia) and predatory (Orbilia), promoted soil microbiota network clustering.
Future studies could combine the use of these fungi with chitosan to treat diseases in various agricultural crops. Our work opens new and promising possibilities to develop integrated strategies based on the use of chitosan formulations to improve soil health and for managing important plant diseases caused by plant parasitic nematodes.
Data availability statement
The datasets presented in this study can be found in online repositories. The data presented in the study are deposited in the NCBI repository, accession number PRJNA1164777 (https://www.ncbi.nlm.nih.gov/sra/PRJNA1164777). The names of the repository/repositories and accession number(s) can be found in the article/Supplementary Material.
Author contributions
RL-N: Formal analysis, Investigation, Methodology, Writing – original draft, Writing – review & editing. JP-R: Data curation, Formal analysis, Investigation, Software, Writing – original draft, Writing – review & editing. IB: Conceptualization, Formal analysis, Investigation, Methodology, Validation, Writing – original draft, Writing – review & editing. AL: Conceptualization, Investigation, Methodology, Supervision, Visualization, Writing – original draft, Writing – review & editing. MVU: Data curation, Formal analysis, Methodology, Software, Validation, Writing – original draft, Writing – review & editing. FL-M: Conceptualization, Funding acquisition, Investigation, Methodology, Project administration, Resources, Supervision, Validation, Visualization, Writing – original draft, Writing – review & editing. LL-L: Conceptualization, Funding acquisition, Investigation, Methodology, Project administration, Resources, Supervision, Validation, Visualization, Writing – original draft, Writing – review & editing.
Funding
The author(s) declare financial support was received for the research, authorship, and/or publication of this article. This research was funded by the PID2020-119734RB-I00 Project from the Spanish Ministry of Science.
Acknowledgments
We would like to thank C.R.A, Agustin Chumillas Roldán, from the Plant Pathology Laboratory (University of Alicante) for his technical support.
Conflict of interest
The authors declare that the research was conducted in the absence of any commercial or financial relationships that could be construed as a potential conflict of interest.
The funders had no role in the design of the study; in the collection, analyses, or interpretation of data; in the writing of the manuscript; or in the decision to publish the results.
Generative AI statement
The author(s) declare that no Generative AI was used in the creation of this manuscript.
Publisher’s note
All claims expressed in this article are solely those of the authors and do not necessarily represent those of their affiliated organizations, or those of the publisher, the editors and the reviewers. Any product that may be evaluated in this article, or claim that may be made by its manufacturer, is not guaranteed or endorsed by the publisher.
Supplementary material
The Supplementary Material for this article can be found online at: https://www.frontiersin.org/articles/10.3389/fagro.2024.1502402/full#supplementary-material
References
Anderson A., Khaleel A., Dutter C., Blauwet M., Flores A., Miller B., et al. (2023). Introduction to soil science (Iowa State University Digital Press Ames, Iowa Consulted). Available at: https://iastate.pressbooks.pub/introsoilscience/chapter/cec-aec/.
Barberán A., Bates S. T., Casamayor E. O., Fierer N. (2012). Using network analysis to explore co-occurrence patterns in soil microbial communities. ISME J. 6, 343–351. doi: 10.1038/ismej.2011.119
Bastian M., Heymann S., Jacomy M. (2009). “Gephi: an open source software for exploring and manipulating networks,” in Proceedings of the international AAAI conference on web and social media, Vol. 3. 361–362. doi: 10.1609/icwsm.v3i1.13937
Bautista-Baños S., Hernández-Lauzardo A. N., del Valle M. V., Bosquez-Molina E., Sánchez-Domínguez D. (2005). Quitosano: una alternativa natural para reducir microorganismos postcosecha y mantener la vida de anaquel de productos hortofrutícolas. Rev. Iberoamericana tecnología postcosecha 7, 1–6.
Bonthala B., Small C. S., Lutz M. A., Graf A., Krebs S., Sepúlveda G., et al. (2021). ONT-based draft genome assembly and annotation of Alternaria atra. Mol. Plant-Microbe Interact. 34, 870–873. doi: 10.1094/MPMI-01-21-0016-A
Bouyoucos G. J. (1927). The hydrometer as a new method for the mechanical analysis of soils. Soil Sci. 23, 343–354. doi: 10.1097/00010694-192705000-00002
Chen J., Zou X., Liu Q., Wang F., Feng W., Wan N. (2014). Combination effect of chitosan and methyl jasmonate on controlling Alternaria alternata and enhancing activity of cherry tomato fruit defense mechanisms. Crop Prot. 56, 31–36. doi: 10.1016/j.cropro.2013.10.007
Chen W., Hu Q. (2021). Secondary metabolites of Purpureocillium lilacinum. Molecules 27, 18. doi: 10.3390/molecules27010018
Darder M., Colilla M., Ruiz-Hitzky E. (2003). Biopolymer– clay nanocomposites based on chitosan intercalated in montmorillonite. Chem. Materials 15, 3774–3780. doi: 10.3390/molecules27010018
De Mesel I., Derycke S., Moens T., van der Gucht K., Vincx M., Swings J. (2004). Top-down impact of bacterivorous nematodes on the bacterial community structure: a microcosm study. Environ. Microbiol. 6, 733–744. doi: 10.1111/j.1462-2920.2004.00610.x
Dervash M., Bhat R., Mushtaq N., Singh V. (2018). Dynamics and importance of soil mesofauna. Int. J. Advance Res. Sci. Eng. 7, 2010–2019.
Escudero N., Ferreira S. R., Lopez-Moya F., Naranjo-Ortiz M. A., Marin-Ortiz A. I., Thornton C. R., et al. (2016). Chitosan enhances parasitism of Meloidogyne javanica eggs by the nematophagous fungus Pochonia chlamydosporia. Fungal Biol. 120, 572–585. doi: 10.1016/j.funbio.2015.12.005
Escudero N., Lopez-Moya F., Ghahremani Z., Zavala-Gonzalez E. A., Alaguero-Cordovilla A., Ros-Ibañez C., et al. (2017). Chitosan increases tomato root colonization by Pochonia chlamydosporia and their combination reduces root-knot nematode damage. Front. Plant Sci. 8. doi: 10.3389/fpls.2017.01415
Garber A. I., Cohen A. B., Nealson K. H., Ramírez G. A., Barco R. A., Enzingmüller-Bleyl T. C., et al. (2021). Metagenomic insights into the microbial iron cycle of subseafloor habitats. Front. Microbiol. 12. doi: 10.3389/fmicb.2021.667944
Giannakou I. O., Tasoula V., Tsafara P., Varimpopi M., Antoniou P. (2020). Efficacy of Purpureocillium lilacinum in combination with chitosan for the control of Meloidogyne javanica. Biocontrol Sci. Technol. 30, 671–684. doi: 10.1080/09583157.2020.1756227
Gortari M. C., Hour R. A. (2016). Purpureocillium lilacinum LPSC876: Producción de conidias en cultivos sobre sustratos sólidos y evaluación de su actividad sobre Nacobbus aberrans en plantas de tomate. Rev. la Facultad Agronomía La Plata 115, 239–249.
Gouveia C., Móréh Á, Jordán F. (2021). Combining centrality indices: Maximizing the predictability of keystone species in food webs. Ecol. Indic. 126, 107617. doi: 10.1016/j.ecolind.2021.107617
Hartig F. (2022). DHARMa: residual diagnostics for hierarchical (Multi-level/mixed) regression models. Available at: https://cran.r-project.org/web/packages/DHARMa/vignettes/DHARMa.html.
Hataf N., Ghadir P., Ranjbar N. (2018). Investigation of soil stabilization using chitosan biopolymer. J. Cleaner Production 170, 1493–1500. doi: 10.1016/j.jclepro.2017.09.256
Heidari J., Amooaghaie R., Kiani S. (2020). Impact of chitosan on nickel bioavailability in soil, the accumulation and tolerance of nickel in Calendula tripterocarpa. Int. J. Phytoremediation 22, 1175–1184. doi: 10.1080/15226514.2020.1748564
Herlemann D. P., Labrenz M., Jürgens K., Bertilsson S., Waniek J. J., Andersson A. F. (2011). Transitions in bacterial communities along the 2000 km salinity gradient of the Baltic Sea. ISME J. 5, 1571–1579. doi: 10.1038/ismej.2011.41
Iriti M., Varoni E. M. (2015). Chitosan-induced antiviral activity and innate immunity in plants. Environ. Sci. pollut. Res. 22, 2935–2944. doi: 10.1007/s11356-014-3571-7
Kamari A., Pulford I. D., Hargreaves J. S. J. (2011). Chitosan as a potential amendment to remediate metal contaminated soil—A characterisation study. Colloids Surfaces B: Biointerfaces 82, 71–80. doi: 10.1016/j.colsurfb.2010.08.019
Kausar R., Iram S., Ahmad K. S., Jaffri S. B. (2021). Molecular characterization of Fusarium solani and Fusarium oxysporum phyto-pathogens causing mango maturity malconformation. Arch. Phytopathol. Plant Prot. 54, 1372–1390. doi: 10.1080/03235408.2021.1910417
Khalil M. S., Badawy M. E. (2012). Nematicidal activity of a biopolymer chitosan at different molecular weights against root-knot nematode, Meloidogyne incognita. Plant Prot. Sci. 48, 170–178. doi: 10.17221/46/2011-pps
Kurtz Z. D., Müller C. L., Miraldi E. R., Littman D. R., Blaser M. J., Bonneau R. A. (2015). Sparse and compositionally robust inference of microbial ecological networks. PloS Comput. Biol. 11, e1004226. doi: 10.1371/journal.pcbi.1004226
Latapy M. (2008). Main-memory triangle computations for very large (sparse (power-law)) graphs. Theor. Comput. Sci. 407, 458–473. doi: 10.1016/j.tcs.2008.07.017
Lenth R. V., Buerkner P., Herve M. J., Jung M., Love J., Miguez F., et al. (2023). emmeans: Estimated Marginal Means, aka Least-Squares Means. Version 1.9.0. Available online at: https://cran.r-project.org/package=emmeans/ (Accessed May, 2023).
Li H., Fei Z., Gong J., Yang T., Xu Z., Shi J. (2015). Screening and characterization of a highly active chitosanase based on metagenomic technology. J. Mol. Catalysis B: Enzymatic 111, 29–35. doi: 10.1016/j.molcatb.2014.11.005
Lopez-Moya F., Colom-Valiente M. F., Martinez-Peinado P., Martinez-Lopez J. E., Puelles E., Sempere-Ortells J. M., et al. (2015). Carbon and nitrogen limitation increase chitosan antifungal activity in Neurospora crassa and fungal human pathogens. Fungal Biol. 119, 154–169. doi: 10.1111/aab.12199
Lopez-Moya F., Suarez-Fernandez M., Lopez-Llorca L. (2019). Molecular mechanisms of chitosan interactions with fungi and plants. Int. J. Mol. Sci. 20, 332. doi: 10.3390/ijms20020332
Lopez-Nuñez R., Suarez-Fernandez M., Lopez-Moya F., Lopez-Llorca L. V. (2022). Chitosan and nematophagous fungi for sustainable management of nematode pests. Front. Fungal Biol. 3. doi: 10.3389/ffunb.2022.980341
Lull C., Bautista I., Segarra R., Monzó J. M., Seguí J. V., Lidón A. (2021). Efecto de una cubierta de paja de arroz sobre la respiración del suelo (IX Simposio Nacional sobre control de la degradación y recuperación del suelo).
Malerba M., Cerana R. (2016). Chitosan effects on plant systems. Int. J. Mol. Sci. 17, 996. doi: 10.3390/ijms17070996
Mamgain A., Roychowdhury R., Tah J. (2013). Alternaria pathogenicity and its strategic controls. Res. J. Biol. 1, 1–9.
Manter D. K., Vivanco J. M. (2007). Use of the ITS primers, ITS1F and ITS4, to characterize fungal abundance and diversity in mixed-template samples by qPCR and length heterogeneity analysis. J. Microbiological Methods 71, 7–14. doi: 10.1016/j.mimet.2007.06.016
Manzanilla-Lopez R. H., Esteves I., Finetti-Sialer M. M., Hirsch P. R., Ward E., Devonshire J., et al. (2013). Pochonia chlamydosporia: Advances and challenges to improve its performance as a biological control agent of sedentary endo-parasitic nematodes. J. Nematol. 45, 1–7.
Mohapatra M., Manu S., Dash S. P., Rastogi G. (2022). Seagrasses and local environment control the bacterial community structure and carbon substrate utilization in brackish sediments. J. Environ. Manage. 314, 115013. doi: 10.1016/j.jenvman.2022.115013
Muzzarelli R. A., Jeuniaux C., Gooday G. W. (Eds.) (1986). Chitin in nature and technology (New York: Plenum Press).
Najafi Z., Golchin A., Alamdari P. (2021). Comparison of the efficiency of different chitosan composites in immobilisation of chromium in contaminated soils. Arch. Agron. Soil Sci., 1–14. doi: 10.1080/03650340.2021.1909720
Nguyen N. H., Tamura T., Shimizu K. (2022). Draft genome sequence of purpureocillium takamizusanense, a potential bioinsecticide. Microbiol. Resource Announcements 11, e00268–e00222. doi: 10.1128/mra.00268-22
Nkoh J. N., Guan P., Li J. Y., Xu R. K. (2024). Effect of carbon and nitrogen mineralization of chitosan and its composites with hematite/gibbsite on soil acidification of an Ultisol induced by urea. Chemosphere 349, 140896. doi: 10.1016/j.chemosphere.2023.140896
Orts W. J., Sojka R. E., Glenn G. M. (2000). Biopolymer additives to reduce erosion-induced soil losses during irrigation. Ind. Crops Products 11, 19–29. doi: 10.1016/S0926-6690(99)00030-8
Palma-Guerrero J., Gómez-Vidal S., Tikhonov V. E., Salinas J., Jansson H. B., Lopez-Llorca L. V. (2010b). Comparative analysis of extracellular proteins from Pochonia chlamydosporia grown with chitosan or chitin as main carbon and nitrogen sources. Enzyme Microbial Technol. 46, 568–574. doi: 10.1016/j.enzmictec.2010.02.009
Palma-Guerrero J., Huang I.-C., Jansson H.-B., Salinas J., Lopez-Llorca L. V., Read N. D. (2009). Chitosan permeabilizes the plasma membrane and kills cells of Neurospora crassa in an energy dependent manner. Fungal Genet. Biol. 46, 585–594. doi: 10.1016/j.fgb.2009.02.010
Palma-Guerrero J., Larriba E., Güerri-Agulló B., Jansson H.-B., Salinas J., Lopez-Llorca L. V. (2010c). Chitosan increases conidiation in fungal pathogens of invertebrates. Appl. Microbiol. Biotechnol. 87, 2237–2245. doi: 10.1007/s00253-010-2693-1
Palma-Guerrero J., Lopez-Jimenez J. A., Pérez-Berná A. J., Huang I.-C., Jansson H.-B., Salinas J., et al. (2010a). Membrane fluidity determines sensitivity of filamentous fungi to chitosan. Mol. Microbiol. 75, 1021–1032. doi: 10.1111/j.1365-2958.2009.07039.x
Pichyangkura R., Chadchawan S. (2015). Biostimulant activity of chitosan in horticulture. Scientia Hortic. 196, 49–65. doi: 10.1016/j.scienta.2015.09.031
R Core Team. (2023). _R: A language and environment for statistical computing_ (Vienna, Austria: R Foundation for Statistical Computing). Available at: https://www.R-project.org/ (Accessed May, 2023).
Reddy D. V. S. S., Kowshik K., Kishor M. J., Chittaranajan M., Sravani E. (2018). “Investigation of chitosan bio-polymer effect on the geotechnical properties of an expansive soil,” in Proceedings of international conference on recent trends in engineering materials, management and sciences, ICRTEMMS-2018, 25–27.
Ren J., Tong J., Li P., Huang X., Dong P., Ren M. (2021). Chitosan is an effective inhibitor against potato dry rot caused by Fusarium oxysporum. Physiol. Mol. Plant Pathol. 113, 101601. doi: 10.1016/j.pmpp.2021.101601
Rhoades J. D. (1982). Cation exchange capacity. Methods Soil analysis: Part 2 Chem. microbiological properties 9, 149–157. doi: 10.2134/agronmonogr9.2.2ed.c8
Sawaguchi A., Ono S., Oomura M., Inami K., Kumeta Y., Honda K., et al. (2015). Chitosan degradation and associated changes in bacterial community structures in two contrasting soils. Soil Sci. Plant Nutr. 61, 471–480. doi: 10.1080/00380768.2014.1003965
Stöveken J., Singh R., Kolkenbrock S., Zakrzewski M., Wibberg D., Eikmeyer F. G., et al. (2015). Successful heterologous expression of a novel chitinase identified by sequence analyses of the metagenome from a chitin-enriched soil sample. J. Biotechnol. 201, 60–68. doi: 10.1016/j.jbiotec.2014.09.010
Suarez-Fernandez M., Marhuenda-Egea F. C., Lopez-Moya F., Arnao M. B., Cabrera-Escribano F., Nueda M. J., et al. (2020). Chitosan induces plant hormones and defenses in tomato root exudates. Front. Plant Sci. 11. doi: 10.3389/fpls.2020.572087
Torres G. A. (2000). Algunos aspectos de los hongos del género Fusarium y de la especie Fusarium oxysporum. Agronomía Colombiana 17, 11–16.
Trolinger J. C., McGovern R. J., Elmer W. H., Rechcigl N. A., Shoemaker C. M. (2017). “Diseases of chrysanthemum,” in Handbook of florists’ Crops diseases. Handbook of plant disease management. Eds. McGovern R., Elmer W. (Springer, Cham, Switzerland), 439–491. doi: 10.1002/ndr2.12013
Turan V. (2019). Confident performance of chitosan and pistachio shell biochar on reducing Ni bioavailability in soil and plant plus improved the soil enzymatic activities, antioxidant defence system and nutritional quality of lettuce. Ecotoxicology Environ. Saf. 183, 109594. doi: 10.1016/j.ecoenv.2019.109594
Turner S., Pryer K. M., Miao V. P., Palmer J. D (1999). Investigating deep phylogenetic relationships among cyanobacteria and plastids by small subunit rRNA sequence analysis. The Journal of eukaryotic microbiology 46 (4), 327–338. doi: 10.1111/j.1550-7408.1999.tb04612.x
Wagg C., Schlaeppi K., Banerjee S., Kuramae E. E., van der Heijden M. G. (2019). Fungal-bacterial diversity and microbiome complexity predict ecosystem functioning. Nat. Commun. 10, 4841. doi: 10.1038/s41467-019-12798-y
Walkley A., Black I. A. (1934). An examination of the Degtjareff method for determining soil organic matter, and a proposed modification of the chromic acid titration method. Soil Sci. 37, 29–38. doi: 10.1097/00010694-193401000-00003
Wood D. E., Lu J., Langmead B. (2019). Improved metagenomic analysis with Kraken 2. Genome Biol. 20, 257. doi: 10.1186/s13059-019-1891-0
Wood D. E., Salzberg S. L. (2014). Kraken: ultrafast metagenomic sequence classification using exact alignments. Genome Biol. 15, R46. doi: 10.1186/gb-2014-15-3-r46
Zavala-González E. A., Lopez-Moya F., Aranda-Martinez A., Cruz-Valerio M., Lopez-Llorca L. V., Ramírez-Lepe M. (2016). Tolerance to chitosan by Trichoderma species is associated with low membrane fluidity. J. Basic Microbiol. 56, 792–800. doi: 10.1002/jobm.201500758
Keywords: chitosan, metabarcoding, nematophagous fungi, plant pathogenic fungi, co-occurrence networks, coacervates
Citation: Lopez-Nuñez R, Prieto-Rubio J, Bautista I, Lidón-Cerezuela AL, Valverde-Urrea M, Lopez-Moya F and Lopez-Llorca LV (2025) Chitosan reduces naturally occurring plant pathogenic fungi and increases nematophagous fungus Purpureocillium in soil under field conditions. Front. Agron. 6:1502402. doi: 10.3389/fagro.2024.1502402
Received: 26 September 2024; Accepted: 16 December 2024;
Published: 22 January 2025.
Edited by:
Danilo Scordia, University of Messina, ItalyReviewed by:
Waheed Ullah Khan, University of the Punjab, PakistanNazia Manzar, National Bureau of Agriculturally Important Microorganisms (ICAR), India
Copyright © 2025 Lopez-Nuñez, Prieto-Rubio, Bautista, Lidón-Cerezuela, Valverde-Urrea, Lopez-Moya and Lopez-Llorca. This is an open-access article distributed under the terms of the Creative Commons Attribution License (CC BY). The use, distribution or reproduction in other forums is permitted, provided the original author(s) and the copyright owner(s) are credited and that the original publication in this journal is cited, in accordance with accepted academic practice. No use, distribution or reproduction is permitted which does not comply with these terms.
*Correspondence: Federico Lopez-Moya, ZmVkZXJpY28ubG9wZXpAdWEuZXM=