- 1Research Institute, Agrarian University of Ecuador, Guayaquil, Ecuador
- 2Computational Sciences, Universidad Agraria del Ecuador, Guayas, Ecuador
- 3Peninsula State University of Santa Elena, Faculty of Agrarian Sciences, Santa Elena, Ecuador
- 4Center of Natural and Exact Sciences (CCNE), Department of Physics, Federal University of Santa Maria (UFSM), Santa Maria, Brazil
- 5Escuela de Posgrado “Ing. Jacobo Bucaram Ortiz, Ph.D”, Universidad Agraria del Ecuador (UAE), Guayas, Ecuador
- 6Colegio de Economia, Universidad San Francisco de Quito (USFQ), Quito, Ecuador
Modeling the intricate interactions between fruit trees, their environments, soils, and economic factors continues to be a significant challenge in agricultural research globally, requiring a multidisciplinary approach. Despite advances in agricultural technology and algorithms, significant knowledge gaps persist in understanding and modeling these interactions. This review explores basic concepts related to modeling for tropical fruit production. It explains modeling development from sensor technologies, image analysis, databases, and algorithms for decision support systems while considering climate changes or edaphoclimatic limitations. We report the current fruit modeling tendencies showing a significant increase in publications on these topics starting in 2021, driven by the need for sustainable solutions and access to large agricultural databases. This study emphasizes inherent challenges in tropical fruit modeling, such as fruit tree cycles, costly and time-consuming experimentation, and the lack of standardized data. These limitations are evident in tropical fruit, where few models have been reported or validated for cocoa, avocado, durian, dragonfruit, banana, mango, or passion fruit. This study analyzes the classification of the algorithms related to tropical fruit into three main categories: supervised, unsupervised, and reinforcement learning, each with specific applications in agricultural management optimization. Crop classification and yield prediction use supervised models like neural networks and decision trees. Unsupervised models, like K-Means clustering, allow pattern identification without prior labels, which is useful for area segmentation and pest detection. Automation of irrigation and fertilization systems employs reinforcement learning algorithms to maximize efficiency. This multidisciplinary review discusses recent approaches to 1) Modeling Soil health and plant-soil interaction, 2) Yield prediction in tropical fruit orchards, 3) Integrating meteorological models for enhanced tropical fruit production, and 4) Economics of tropical fruit business through modeling. Furthermore, this review illustrates the complexity and multidisciplinary research on models for tropical fruit and platforms using agricultural models. Further opportunities to advance fruit modeling frameworks are indicated, requiring technical knowledge about the fruit crop requirements with user-friendly platforms to collect and access fruit tree data and site-specific agroecological conditions.
1 Introduction
The global demand for tropical fruits has experienced an increase in recent years, influenced by a growing consumer preference for healthier, more diverse, and sustainable food options (Mukhametzyanov et al., 2023). Tropical regions of the world, such as parts of North America, South America, Africa, Australia, and Southeast Asia, are home to Tropical fruits renowned for their unique flavors, vibrant colors, and abundant nutritional benefits (Stewart and Ahmed, 2020; Harris et al., 2022). Despite the steady increase in demand for pineapples, mangoes, papayas, and avocados (Altendorf, 2017), tropical fruit production is limited by unpredictable weather patterns (Nath et al., 2019), pest and disease outbreaks (Cilas et al., 2016; Merle et al., 2022), and fluctuating market demands (Mukhametzyanov et al., 2023). These factors can significantly impact yield and fruit quality, making it difficult for farmers to optimize production and ensure consistent income. Then, modeling can work as a powerful tool for simulating the interplay of variables like climate (González-Orozco et al., 2020), soil conditions, and crop management practices; models can help predict potential production bottlenecks and assess the effectiveness of different interventions (González-Orozco et al., 2020; He et al., 2022).
Modeling the complex interactions of fruit trees with biotic and abiotic factors remains a challenge for agricultural research worldwide (Grisafi et al., 2021). Despite coordinated efforts of research institutions, universities, and private companies and advances in the Internet of Things (IoT) or e-farming technology, the knowledge gaps in modeling tropical fruits remain recurrent and challenging for the research community. Fruit modeling can enable producers and consumers to combine satellite data and sensor technologies for decision support systems and mitigate climate changes or edaphoclimatic limitations (Villa-Henriksen et al., 2020; Miranda et al., 2023). Tropical fruit production modeling presents inherent challenges, starting with the fruit tree cycles, costly and time-consuming experimentation, and the lack of standardized data available (Ackerman and Montalvo, 1990; Goldschmidt and Lakso, 2005). These limitations are evident in tropical fruit, where few models are available or validated for cocoa (Tosto et al., 2023), banana (Jayasinghe et al., 2022; Sahu et al., 2022), guava (Bibwe et al., 2022), mango (Boudon et al., 2020), or avocado (Erazo-Mesa et al., 2021; Mokria et al., 2022). Moreover, most tropical fruit crops are evergreen, and evergreen fruit trees are often considered more challenging to study than deciduous trees due to the continuous physiological activity and dynamic role of their leaves (Grisafi et al., 2022). Improved fruit modeling capabilities, from soil health to disease management, fruit development, and forecasting yield and price trends, are needed to provide fruit growers with better tools for sustainable orchards, adapt management tactics, efficiently plan harvests, and foresee probable obstacles, thus establishing a reliable foundation for well-informed fruit-growing decisions (Gallardo et al., 2020; Anderson et al., 2021). Opportunities to advance tropical fruit modeling frameworks require blending technical knowledge about the fruit crop requirements and genetic and environmental limitations with user-friendly platforms to collect and access fruit tree data and site-specific agroecological conditions (Haque et al., 2020).
Modern fruit production is a mechanized multidisciplinary process that enables the regulation, control, and management of the resources required to produce a high-quality product. This modernization enhances the use of sensors, cameras, drones, datasets, and algorithms while incorporating fruit production into the economic analysis and modeling at the domestic and international spheres (Shamshiri et al., 2016). The efficient system of contemporary tropical fruit production is interconnected with all economic facets, and its development is intertwined with the total dynamics (Altendorf, 2017). Forecasting tropical fruit yields poses difficulties, and assessing prediction jobs is crucial in enhancing agricultural yield (Villachica et al., 2020). This predictive methodology adopted in Machine learning tools will enhance operational efficiency and positively impact strategic planning, increasing productivity and sustainability in fruit production (Gómez-Lagos et al., 2023).
Machine Learning is an area of artificial intelligence that empowers a computer to acquire knowledge and skills from data and construct mathematical models to facilitate comprehension of the gathered data, which is of significant importance (Jawade et al., 2020; Chabalala et al., 2022). This analysis of fruit data addresses three primary categories of problems: supervised learning difficulties, unsupervised learning problems, and reinforcement learning problems (Sahu et al., 2022). The utility of machine learning on fruit modeling has been enhanced with the emergence of big data technology, which refers to a substantial volume of information derived from many sources (Khan et al., 2020).
Nowadays, agricultural scientists account for multiple sources of information such as AVHRR (Advanced Very High-Resolution Radiometer) sensor, AgRISTARS (Agriculture and Resource Inventory Surveys Through Aerospace Remote Sensing), MARS (Monitoring Agricultural Resources), GMFS (Global Monitoring of Food Security), and PlantVillage for plant phenotyping, among resources (Atzberger, 2013; Jiang and Li, 2020; Saiz-Rubio and Rovira-Más, 2020). Fruit research and production can obtain information on precipitation, temperature, yield, pesticide use, land use, and other factors influencing fruit production and commercialization. Despite studies describing different AI technology and ML algorithms at a fruit farm level, the majority of available information relates to temperate fruits on developed-country farms (Kamilaris et al., 2017; Wolfert et al., 2017; Ip et al., 2018; Saiz-Rubio and Rovira-Más, 2020).
Despite being in the era of Agriculture 4.0 and 5.0, databases or big data for tropical fruit modeling are still a great challenge across regions, but especially for farmers in least-developed and developing countries (Wolfert et al., 2017; Jones et al., 2017a; Morris et al., 2020). Even within developed countries, studies emphasize that big data are not accessible for everyone but for big agri-business companies (Kamilaris et al., 2017). A source of data in the tropics is the yearly national agricultural surveys. For instance, researchers can access worldwide agricultural statistics based on these surveys through the FAOSTAT repository (FAO, 2018). In some countries, public statistics agencies have gathered information (censuses) on land use, production levels, chemical input use, and labor demand across farm products since early 2000. However, tropical countries have many difficulties in developing these national censuses on time due to a lack of financial resources, struggles in using new technologies to gather information, or inadequate technical staff (Castano and Neciu, 2022). A problem for tropical fruit modeling is that the census databases gather information to diagnose the global situation of agriculture in a country but are not real-time data to accurately model the behavior or tendency of any crop, or particularly, tropical fruit production system (Deere and Twyman, 2014).
Modeling in tropical regions has struggled with a lack of standardized information on agricultural data to understand problems such as deforestation in America, Africa, Asia, and Oceania (Berman et al., 2023), understanding pest affection in extreme temperatures in the tropical Andes (Crespo-Pérez et al., 2013), having real-time pest detection data in banana production (Selvaraj et al., 2019), insufficient environmental and climate change data in Latin America, and African countries (OECD, 2023; Chemura et al., 2024), scarce annotated databases for plant development analysis (Jiang and Li, 2020), lack of information of data inputs of the tropical fruit production. Overall, tropical regions have to work towards the improvement of big data access in terms of specialized human capital, digital infrastructure, data governance, and accurate, standardized information, among others (Atzberger, 2013; Kamilaris et al., 2017; Wolfert et al., 2017; Jones et al., 2017b; Ip et al., 2018; Saiz-Rubio and Rovira-Más, 2020).
This review describes the modeling advances in the multiple disciplines related to the tropical fruit production environment, illustrated in Figure 1. The tendency analysis provides an image of the current interest in the domain and reveals the lack of customized functions for tropical fruits and few language or socioeconomic access considerations (Table 1).
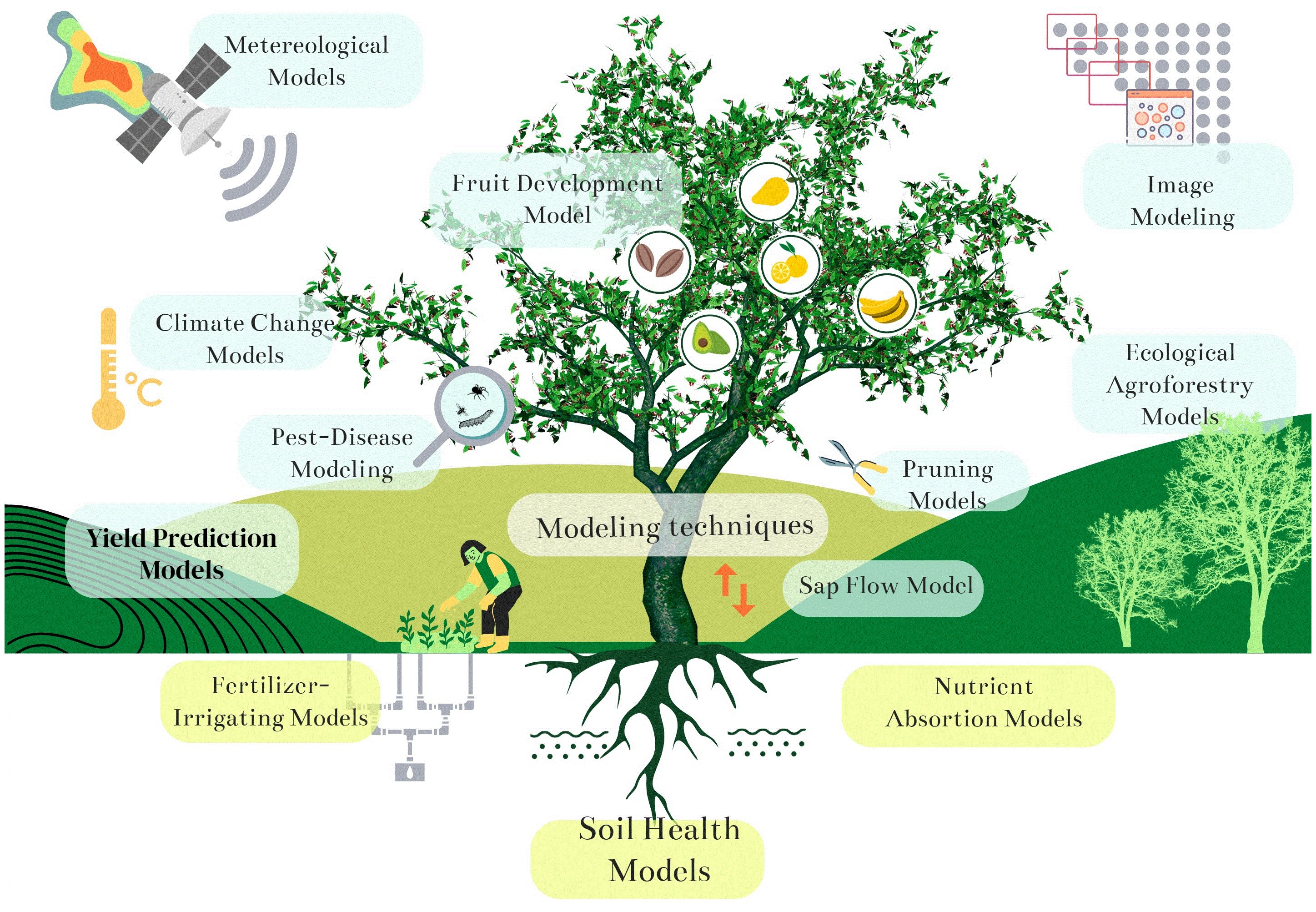
Figure 1. Graphical representation of a multidisciplinary framework for modeling tropical fruit production, highlighting the structures, biological processes, functions, and environmental interactions involved.
2 Models and algorithms in fruticulture
Research in fruit production and technology has experienced remarkable advances in integrating artificial intelligence (AI) and machine learning for modeling, image analysis, and robotics in recent years (Licardo et al., 2024). Predictive models are highlighted as they use climate, soil, and crop data to enable farmers to make informed decisions about irrigation, fertilization, pest control, and management, promoting optimal crop yields and quality (Macharia and Kiage, 2024). Mostly, fruit orchard data inputs are related to weather, soil, management, tree structure and physiology, satellite data, and historical records (Mite-Baidal et al., 2019). Computer vision has also emerged as a promising tool for early disease detection and plant growth monitoring, while agricultural robotics is already automating processes such as harvesting and sowing, improving efficiency, and reducing labor requirements (Anbumozhi and Shanthini, 2023; Varma et al., 2024). Collectively, these innovations are remodeling more precise and productive sustainable agriculture.
Using models and algorithms for fruit production is a trend for improving fruit production through data analysis (Mathiazhagan et al., 2021). Some of the most common algorithms used are related to fruit yield prediction, precision agriculture, fruit pest and disease detection, fruit breeding and genetics, fruit production waste management, and market analysis. Pioneering models for perennial crops began in the 1980s with TREEDYN (Bossel, 1996), predating personal computers, and have since evolved into hybrid AI models on cloud-based platforms. The timeline evolution of modeling in Figure 2 highlights key advancements related to fruit modeling, starting from basic statistical models, moving through mechanistic models, artificial neural networks, and sensor integration, to modern applications of AI and machine learning, such as convolutional neural networks and hybrid AI models. Each decade is marked by significant developments, detailing the specific models and technologies contributing to yield prediction, disease detection, and resource optimization in fruit tree modeling.
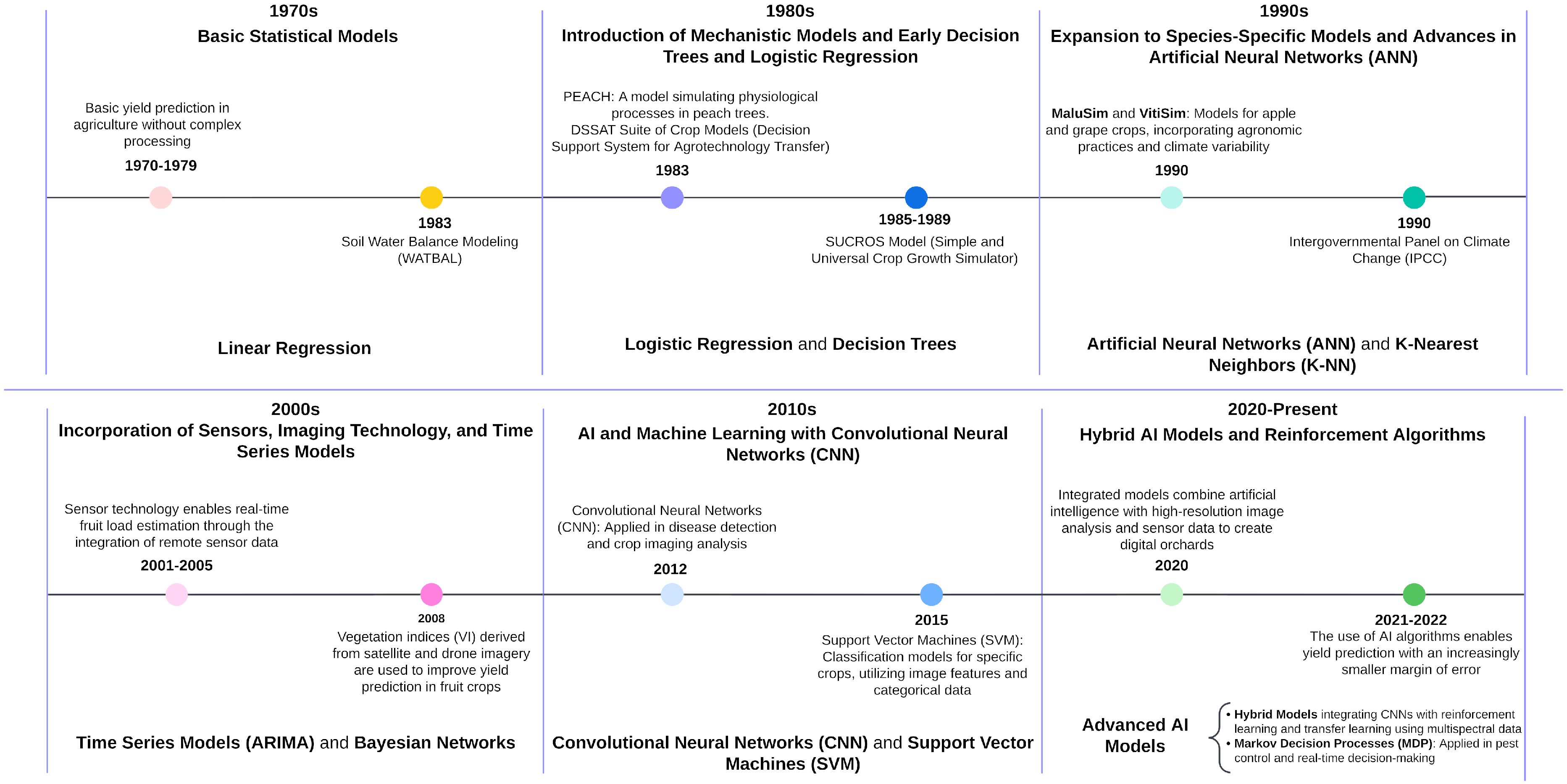
Figure 2. Timeline of modeling techniques and technological advancements in tropical fruit research from the 1970s to present (see Table 1 and subsections for additional details).
The fruit tree models have been mainly categorized based on the structure, process, and function of the tree organs, resulting on Processed-Based models (PBM), Functional structural plant models (FSPM) and a combination of both (Grisafi et al., 2022). In addition, fruit tree models have also been classified based on the data relationship with function or statistics on empirical and mechanistic models (Barbault et al., 2024). In this review, the machine learning algorithms applied to tropical fruits are classified into three categories: supervised, unsupervised, and reinforcement learning algorithms, as shown in Figure 3. Each category has specific applications that enable addressing various aspects related to crop management and optimization. Supervised algorithms include classification and regression models used in crop categorization and yield estimation. The most common machine learning techniques are support vector machines (SVM), decision trees and random forests, and artificial neural networks (ANN). Neural networks are particularly effective tools for representing complex, nonlinear relationships in agricultural datasets and outperform previous techniques based on expert-driven feature engineering (Kraus et al., 2020). Deep learning is a specialized type of neural network that builds models with numerous layers to detect complex and abstract patterns in data (Hasimi et al., 2024). In contrast, decision trees and random forests excel at analyzing heterogeneous and noisy datasets. Currently, a research trend involves using these models to improve fruit production efficiency through the anticipation of specific growth conditions and the categorization of detailed information, such as fruit quality assessment and disease identification. Within unsupervised algorithms, clustering methods such as K-means and hierarchical clustering are more relevant. These algorithms enable the identification of patterns in data without requiring predefined labels. Various applications highlight their utility, including the segmentation of agricultural areas based on soil properties, the classification of production regions, and the detection of pest hotspots. Bayesian networks, which fall within this category, provide sophisticated capabilities for modeling uncertainty and causal relationships. The agricultural sector uses these networks to predict pest outbreaks and manage risk. Agriculture specifically uses reinforcement learning algorithms for automation and long-term decision optimization. Field applications of this technology include managing irrigation and fertilization systems to optimize resource efficiency through reward accumulation. Within reinforcement learning, the use of convolutional neural networks (CNN) for real-time agricultural image evaluation stands out, enabling the identification of potential issues related to crop development or health. Research in this field focuses on enhancing the sustainability of agricultural production through intelligent automation and optimal decision-making, aiming to maximize yields and reduce agricultural input usage.
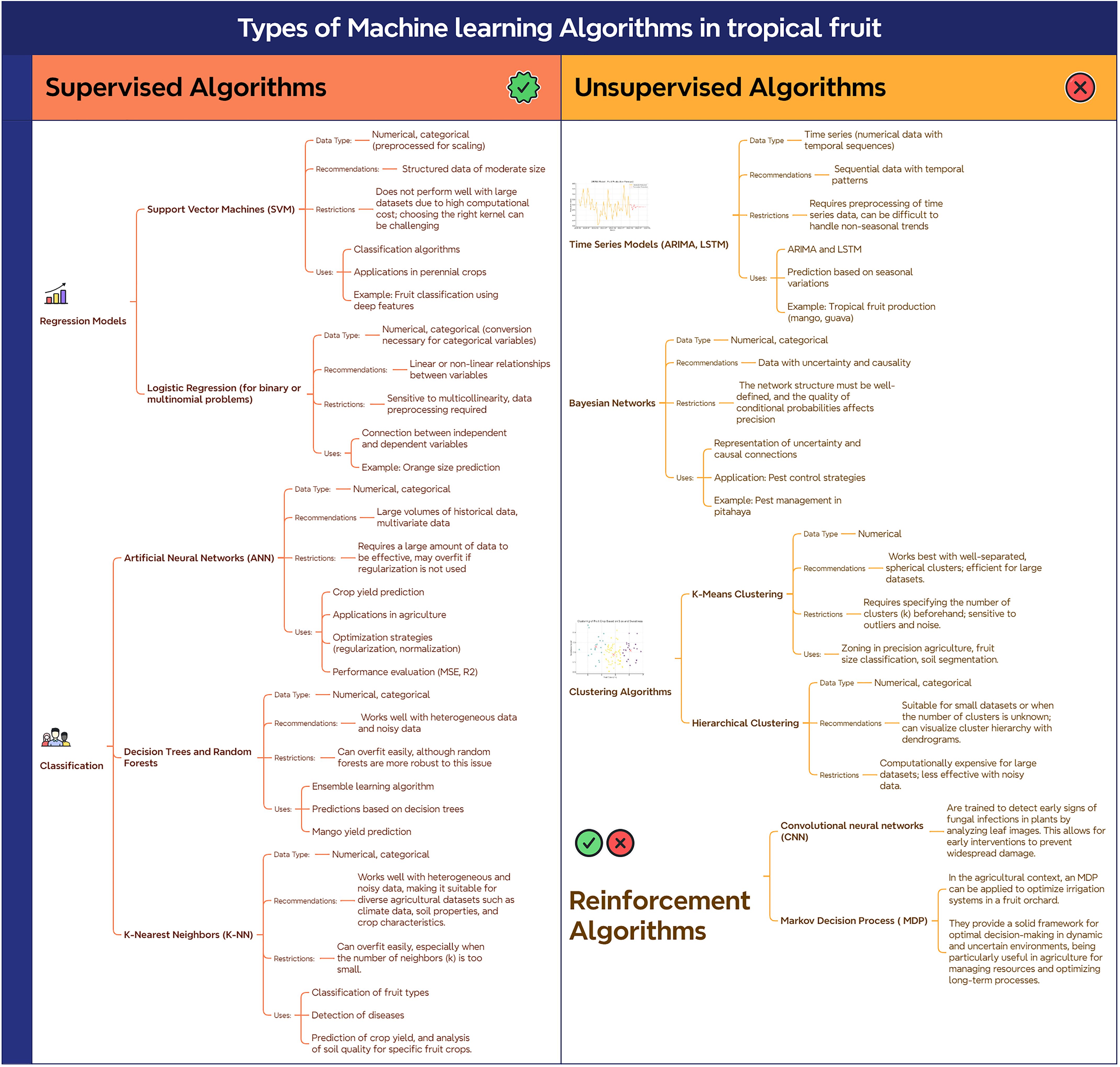
Figure 3. Classification of artificial intelligence algorithms applied to agriculture into three main categories: supervised, unsupervised, and reinforcement learning. Each category details the data type, recommendations, restrictions, and specific applications in the agricultural context.
2.1 Artificial neural networks
These mathematical models, derived from the architecture of the human brain, can acquire knowledge about non-linear connections between variables and are often used to predict fruit yield (Rauber et al., 2017; Aworka et al., 2022). ANNs can comprehend agricultural systems and predict their performance using historical data and climate, soil conditions, and management practices (Heaton et al., 2018). ANNs are a category of deep learning algorithms that comprise interconnected layers of artificial “neurons” that analyze and convert input data into output predictions following a specific structure. Every individual neuron is linked to a specific collection of weights and biases, which undergo modifications as part of the training procedure. The layers are often classified into three categories: Input Layer: Receives input variables, including climatic, soil, and management data (Salari et al., 2023). Produces final predictions, such as the expected crop performance. (Duarte-Carvajalino et al., 2021; Karydas et al., 2023). The network iteratively adapts its weights and biases to minimize the disparity between forecasts and actual performance values. Since the quality and quantity of training data in these models heavily influence the precision of an ANN, validating the significance of data is crucial. The data should accurately reflect the state of crops and encompass a range of elements, including historical weather data, soil information, management strategies, and records of past performance (Salari et al., 2023). Various strategies and factors enhance the prediction of perennial crop yields, including regularization, and normalization, which prevents overfitting, enables input data scaling, accelerates the training process, and enhances network convergence (Bennett et al., 2013; Shahhosseini et al., 2019). Evaluation measures, such as the mean squared error (MSE) or the coefficient of determination (R2), are utilized to quantify the precision of predictions (Humphrey et al., 2017).
2.2 Support vector machines
These supervised learning algorithms identify the most effective decision boundaries separating distinct data classes. In perennial crops, SVMs can categorize various performance degrees by utilizing predictor factors (Berk, 2020). For example, fruit recognition using an SVM based on deep features presents a model that utilizes a classifier connected to the layer of a convolutional neural network model. This model aims to classify 40 different types of Indian fruits (Behera et al., 2020).
2.3 Decision trees and random forests
Random forest is a powerful ensemble learning algorithm that has gained significant attention in predictive modeling (Trieu and Thinh, 2023). This methodology relies on constructing a forest of decision trees, where each tree is trained on a random subset of features and data samples. The resulting ensemble of trees is then used to make predictions, with the final output determined by a majority vote or average of the individual tree predictions (Yaseen, 2023). An RF model from Fukuda et al. (2013) accurately predicted mango fruit yields based on water supply and various irrigation methods in mango research. The RF models provided precise estimations for maximum and average yield values for mango fruit and intermediate accuracy in predicting fruit minimum yields.
2.4 Time series models
Similar to ARIMA (AutoRegressive Integrated Moving Average) and LSTM (Long Short-Term Memory), these models are helpful for fruit crops that experience seasonal and climatic variations. These TSM algorithms prioritize forecasting future values by analyzing previous patterns in the time series (Ali et al., 2024). In their study, Amir-Hamjah (2014) highlighted the performance of the hybrid temporary series model for predicting the production of mango, banana, and guava. If the data set consists of a pattern of linearity and non-linearity, the hybrid model presented better performance compared to any individual time series or machine learning technique.
2.5 Bayesian networks
This statistical model helps represent data uncertainty and causal connections between variables. They are valuable for evaluating the impact of various factors on agricultural productivity. Several studies present a promising approach to assessing pest control strategies using Bayesian nets, given that effective pest management is a crucial component for crop performance, as these can seriously affect yields and crop quality throughout the fruit supply chain (Singh and Gupta, 2017; Holt et al., 2018; Salliou et al., 2019). Then BN have been used to compare pest control interventions such as fruit fly pest in the pitahaya (Holt et al., 2018).
2.6 Regression models
Regression models, such as linear, multiple linear and polynomial regressions, can be employed to identify connections between independent factors (e.g., soil properties, climate, image analysis) and the dependent variable (crop yield). In Rauch et al.‘s (2023) citrus study, a polynomial regression model estimated the size of the oranges. Another RM study on sensors or image analysis; Robson et al. (2017) used high-resolution multispectral satellite imagery (Worldview 2-3) to map yield parameters in avocado crops in Australia.
2.7 Research tendency on fruit modeling
The tropical fruit modeling domain has undergone significant changes in recent years. Our study of literature indicates a large surge in publications starting in 2021, with a particularly rapid acceleration in 2022 and 2023. The co-currency chart in Figure 4 represents the interconnected nature of several research fields within the trends of application of models in fruit crops. This co-currency reveals that the keywords “machine learning,” “forecasting,” and “internet of things” are the most recurring keywords in fruit modeling research studies. Note that other terms like precision farming, which is gaining importance in 2021, are strongly associated with implementing technology in agriculture, keeping a relation previously mentioned by Ruiz-Real et al. (2020). Moreover, trends in fruit crop artificial intelligence techniques have developed around agronomy, agriculture, and sustainability. The analysis of scientific publications from 2018 to 2024 in Figure 5 reveals an upward trajectory in research on Tropical fruit related to agriculture and technology. Between 2018 and 2019, the number of publications remained low, suggesting an emerging field with limited interest. However, a gradual increase was observed between 2019 and 2020, indicating growing interest and development. The year 2021 showed a turning point, with a significant increase in publications, possibly due to methodological advances, new technologies, or increased funding. This growth accelerated further in 2022 and 2023, peaking in the last year, reflecting an active scientific community and a consolidated research field. Although 2024 is at a midpoint, the number of publications remained high, suggesting that the field remains relevant and dynamic (He et al., 2023).
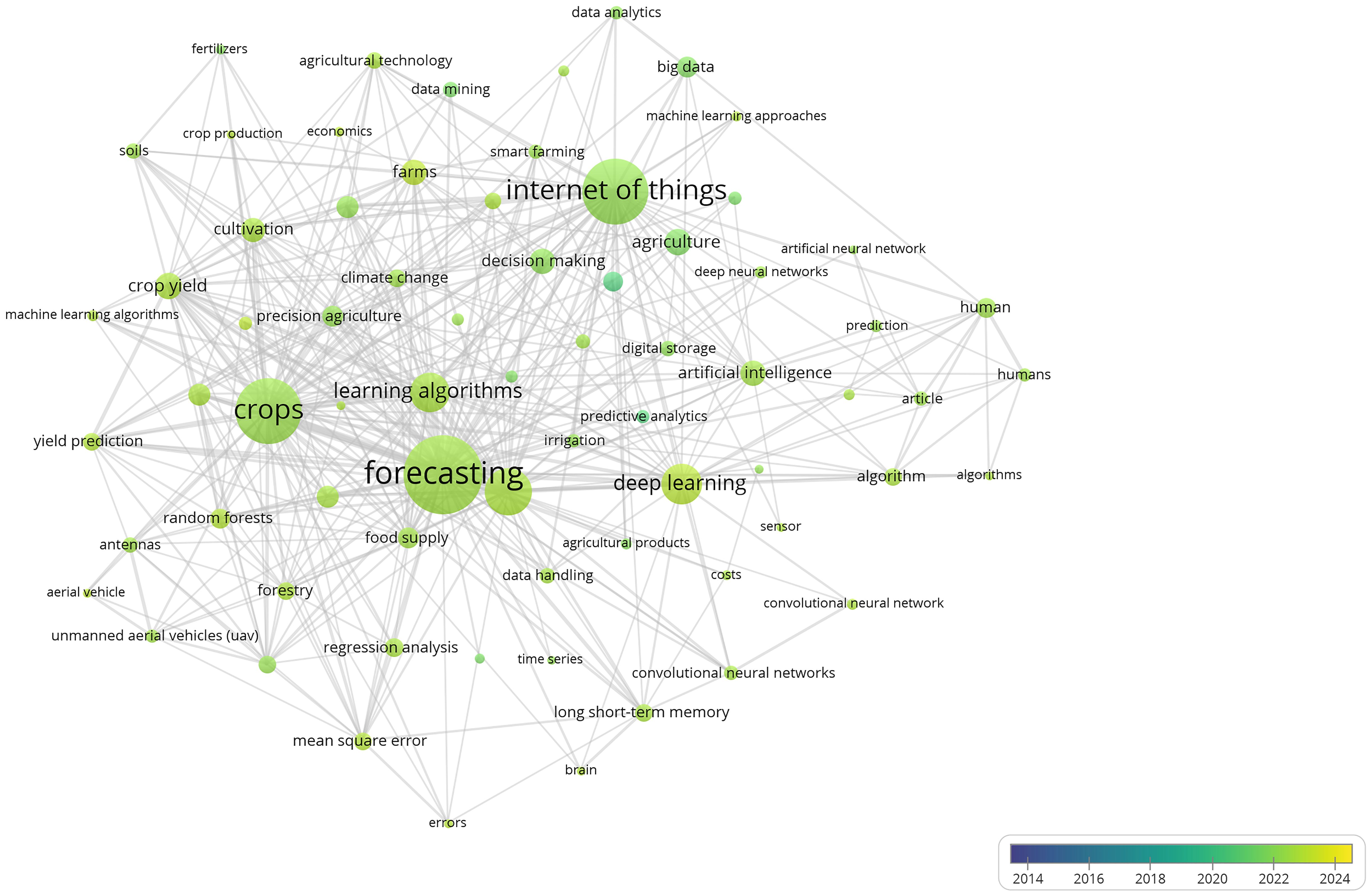
Figure 4. Keyword network analysis and association strength from the research literature associated with tropical fruit modeling. The color gradient indicates the timing from 2014 (blue) to 2024 (yellow).
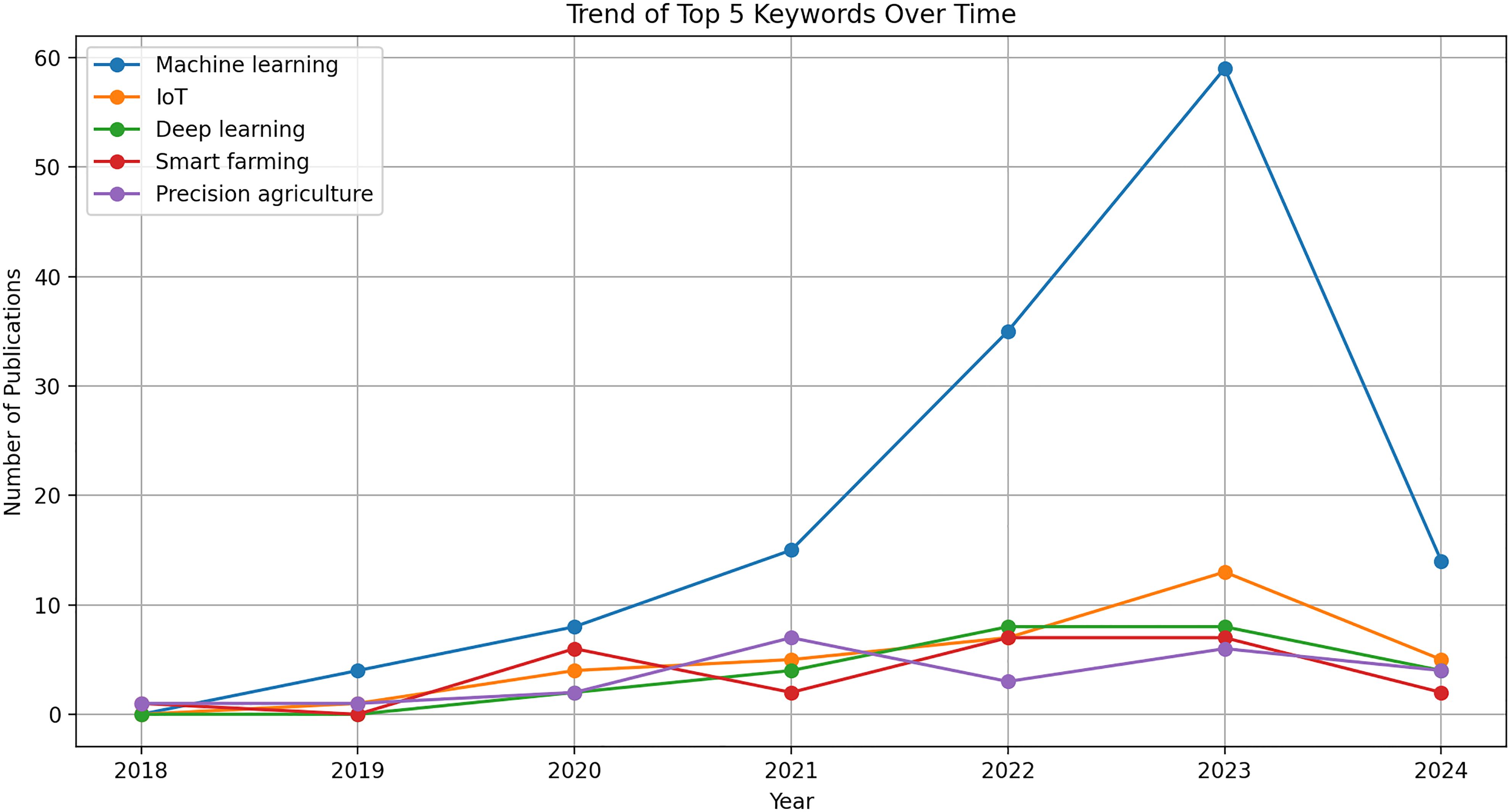
Figure 5. Annual scientific paper publication rate indexed by Scopus records for Machine learning, IoT, Deep learning, Smart farming, and precision agriculture related to fruit production from 2018 to 2024.
The growing interest in tropical fruits highlights the field’s importance to science and society. Advances in technology and methods, like modeling, data collection, and computing power, have enabled researchers to tackle complex issues more accurately. The modeling field is becoming increasingly important because of the rising demand for tropical fruits, which demands sustainable and efficient production strategies (Bhat and Paliyath, 2016). Notably, innovations in remote sensing, weather stations, sensors, drones, and artificial intelligence have driven research forward, sparking broader interest and increasing the dissemination of findings in tropical fruit research (Nath et al., 2019; Md Nor and Ding, 2020). In response, the research community has increased its efforts to understand the dynamics of tropical fruit production, ranging from genetic enhancement to post-harvest handling (Vieira et al., 2024).
The consistently increasing number of publications in 2024 highlights this study topic’s ongoing interest and significance. Tropical fruit modeling has practical consequences for farmers, consumers, and politicians, extending beyond academic research (Aline et al., 2023). Modeling studies contribute to the establishment of resilient and sustainable tropical fruit production systems by providing evidence-based insights into crop management, resource optimization, and climate change adaptation.
3 Modeling of soil health, plant-soil interaction
Soils are a fundamental component of agroecosystems, with their quality playing a crucial role in tropical fruit production. Traditionally, fertility has been assessed based on chemical properties; however, in recent years, this concept has evolved to include physical and biological properties as well (García et al., 2012). This shift in understanding reflects a broader recognition of the diverse factors that contribute to soil health.
Moreover, fluctuations in climatic conditions, such as rainfall, temperature, and air quality, significantly impact soil composition and, consequently, agricultural productivity. Therefore, current efforts focus on finding solutions to mitigate the adverse effects of environmental changes on agricultural yields (Borrelli et al., 2020).
To aid in decision-making, prediction models have become valuable tools as they can forecast variable behaviors and generate patterns or trends for specific situations. These models are particularly useful for analyzing the physical properties of soil, which tend to remain stable over time under natural conditions. For instance, one of the most well-known models for predicting soil erosion is the Universal Soil Loss Equation (USLE) (Alewell et al., 2019). Despite being an empirical model, it has been widely accepted and applied in many fields of soil science due to its reliability.
Advanced methods such as the Deep Learning Regression Network (DNNR) have also shown great promise. As demonstrated by Cai et al. (2019), the DNNR method was used to predict soil moisture content, achieving an R2 value of 0.98. This performance is comparable to the Artificial Neural Network (ANN2) (Adeyemi et al., 2018) and superior to the Support Vector Machine (SVM) (Gill et al., 2006), which had an R2 value of 0.89. Furthermore, the same study analyzed the Multilayer Perceptron (MLP) model, which yielded similar R2 values (0.97) but with a higher Mean Absolute Error (MAE) of up to 70%. In this context, Mallick et al. (2022) used the Nearest-Neighbourhood Autoregressive Moving Average (NN-ARMA) method to predict soil organic matter content from remote sensors. This method was able to explain 96.4% of the total variation in soil organic matter with an RMSE of 0.093.
Additionally, various models have been developed to simulate root-soil interactions. Dunbabin et al. (2013) compared six models (RootTyp, SimRoot, ROOTMAP, SPACSYS, R-SWMS, and RootBox) and evaluated the potential of each model for creating a three-dimensional model root structure and growth dynamics according to intrinsic soil factors. Generally, R-SWMS (Meunier et al., 2022) simulates water uptake by roots and models the root-soil hydrological interaction. SimRoot (Lynch et al., 1997) simulates nutrient uptake dynamics, although it has nitrate issues. ROOTMAP (Diggle, 1988) is more efficient and simulates root proliferation. SPACSYS (Wu et al., 2007) is used for crop modeling and integrates biomass and crop yield predictions with root and soil dynamics. RootTyp (Pagès et al., 2004) simulates root architecture and can be combined with soil models. RootBox (Leitner et al., 2010) uses a Matlab structure to simulate root growth and development systems, an open system that supports expansion.
A primary goal of most agricultural models is to predict yield with high precision and accuracy. Concerning this, Barbosa et al. (2020) evaluated the Convolutional Neural Network (CNN) model using inputs such as nitrogen and seed rates, elevation maps, soil electroconductivity, and satellite imagery. Notably, this study achieved a 68% reduction in RMSE compared to multiple linear regression and a 29% reduction compared to random forests. This approach integrates neural networks into geospatial problems, enabling the creation of models without the need to develop specific features or make assumptions about spatial distribution, which are directly incorporated into the learning process.
In this regard, studies described by Jasoliya et al. (2024) detail a group of models focused on soil water dynamics through the matrix component and other physical properties. These authors note that empirical and analytical models are easy to implement but provide limited information; numerical methods based on finite elements (FE) and particles are preferred for detailed studies. The Arbitrary Lagrangian-Eulerian (ALE) method within the FE method correlates better with the actual soil behavior but is unsuitable for modeling large deformations and discontinuous behaviors. Particle-based methods (SPH) and the Discrete Element Method (DEM) are also used to overcome the limitations of the FE method. Although moderately advanced, these methods require further evaluation and improvement for these specific applications.
Predictive models for tropical fruits are scarce due to the heterogeneity of physiological variables. Leaves, trunks, and roots can have different ages within the same plantation, resulting in varying sizes, shapes, and colors. Additionally, leaf flows are highly sensitive to climatic and soil conditions (Nafees, 2019). A clear example is mango, where water stress triggers the activation of senescence phytohormones (ethylene), halting the emergence of new shoots and preparing the plant for flowering (Valdez-Rivera et al., 2022). This exemplifies one of the many challenges in modeling perennial crops, and it becomes more complex when the interaction with the soil matrix is added.
4 Yield prediction in tropical fruit orchards
Fruit yield is intricately linked to various biotic and abiotic factors such as weather conditions (Haque et al., 2020), soil quality (Srivastava et al., 2021), orchard management practices (Haque and Sakimin, 2022), irrigation (Zuazo et al., 2021; Tong et al., 2022), fertilization (de Mello Prado and Rozane, 2020; Sun et al., 2022), pests and diseases, and physiological tree development (Bons and Kaur, 2020). Weather conditions, including temperature, rainfall, and sunlight, directly influence fruit-bearing plants’ growth and development and indirectly affect disease and pest pressure (Orlandini et al., 2020). Yield prediction starts with understanding and managing the physiological development of fruit crops, including flowering (Gene Albrigo and Galán Saúco, 2004; Mohandass et al., 2018) and fruit setting processes (Agustí and Primo-Millo, 2020; Alcaraz and Hormaza, 2021). The intricate interplay of these factors demands comprehensive models achieving accurate fruit production predictions for maximizing fruit yields. Previous studies have evaluated microsensors, frameworks, and numerous features that could be considered for tropical fruit yield prediction systems, such as genetics (Seyum et al., 2022), NDVI (Mwinuka et al., 2022), photosynthetically active radiation (PAR), biomass, temperature, precipitation, fertilization, irrigation schemes on (Garrido et al., 2023). Therefore, multiple models are under evaluation for climate conditions, management strategies, and plant/fruit growth (He et al., 2022).
By harnessing the power of current modeling techniques and technologies for data acquisition and computing, nations and fruit growers can optimize their practices, reduce environmental impact, and contribute to global food security (van Meijl et al., 2020). Fruit modeling leverages data from various sources, including weather patterns, soil conditions, and historical crop performance (Anderson et al., 2021), to generate precise predictions of fruit yields. This information analysis enables fruit orchards and governments to make informed decisions about agricultural programs and fruit waste management (Abadi et al., 2021; Magalhães et al., 2021), improving resource allocation and increasing orchards’ productivity. In addition, fruit yield models contribute to risk mitigation by allowing fruit industries and growers to anticipate and mitigate the impact of adverse weather conditions. Ultimately, all these benefits enhance the opportunity for breeding more resilient tropical fruits (Sattar et al., 2021). The progression of modeling fruit yield research focuses on revealing trends such as the persistent emphasis on climate-related, the growing importance of modeling techniques, and the shift towards breeding studies in recent years.
Classical Fruit yield prediction models were initiated from traditional calculations using sampling estimation and empirical models. However, these models are limited by variable geographical conditions, complicated natural environments, economic cost, qualified labor, and time (Khan et al., 2020). More recently, automatic monitoring technologies, intelligent equipment, and advanced models have allowed for more comprehensive yield prediction systems. Current yield prediction systems rely heavily on image processing (Wang et al., 2024), weather station data, irrigation sensors, and satellite information to reduce the manual effort of classical models that require counting and weighing fruits. Based on their basic structure, fruit yield prediction models require inputs and provide an output related to fruit quantity and/or quality. Fruit yield data is the ultimate consideration for decision-making in orchard management regarding other labor requirements. Yield data affects post-harvest storage conditions, transport, and marketing logistics. Therefore, fruit yield prediction models are some of the most common models on tropical fruits, as shown in Table 1.
To further illustrate the diversity of modeling approaches applied in tropical fruit production, Table 1 provides a comprehensive summary of different modeling techniques and their respective applications. This table offers a detailed overview of algorithms used to solve specific challenges related to tropical fruit agriculture. These tasks include yield prediction, disease detection, ripeness estimation, pest infestation monitoring, and climate impact modeling. The table highlights the evolution of methodologies, ranging from traditional statistical analyses to sophisticated machine learning algorithms, which reflects the growing complexity of agricultural challenges in tropical environments.
The table is organized to quickly identify which modeling approaches have proven effective for specific tasks across various tropical fruits. By categorizing the models according to their application, the table demonstrates how different modeling tools tackle distinct aspects of fruit production, from growth modeling to optimizing supply chains. The references are an evolution indicator in modeling techniques summarized in the table. For instance, early models employed simpler methods like regression analysis, while more recent studies have adopted machine learning techniques such as Convolutional Neural Networks (CNN) and Decision Tree classifiers.
5 Integrating meteorological models for enhanced tropical fruit production
Tropical fruit production faces increasing challenges due to climate change. Tropical fruit production, essential for economic and nutritional sustenance in many regions, is significantly jeopardized by meteorological variations. These variations include a range of environmental stresses such as temperature fluctuations, drought, and extreme weather events, which directly impact the physiological, anatomical, morphological, and biochemical aspects of fruit crops (Malhotra, 2017; Benkeblia, 2021).
For example, high temperatures can disrupt plant development processes at critical stages—seed germination, plant growth, flower shedding, and fruit setting—thereby affecting fruit weight, size, and overall quality (Pandey et al., 2021). In tropical fruits like mangoes, bananas, and papayas, these climatic conditions may alter flowering patterns, reduce fruit set, and increase susceptibility to pests (Gutierrez et al., 2021; Zhang et al., 2022) and diseases. Additionally, climate-induced drought poses a dual threat: it directly reduces yields by decreasing the number of fruit pods per tree and indirectly affects the essential pollinator activities needed for fruit production (Gupta et al., 2021).
The implications of these climatic challenges are profound, extending beyond agricultural production to broader socio-economic dimensions. In tropical regions, where agriculture is the backbone of economies and communities, the repercussions of climate-induced disruptions in fruit production can be particularly severe, leading to crop losses, economic instability, and labor shortages (Snyder, 2017; Eftekhari, 2022).
Given this scenario, integrating meteorological models into tropical fruit production processes is a crucial strategy (Ramirez-Guerrero et al., 2023). These models offer predictive insights into weather patterns, enabling better preparedness and adaptive measures. The following sections explore the specifics of these meteorological models, their integration with existing agricultural practices, and potential pathways to enhance the resilience and productivity of tropical fruit cultivation in the face of changing climate dynamics (Zscheischler et al., 2020).
5.1 Overview of meteorological models in agriculture
Applying meteorological models in agriculture is pivotal for understanding and managing the impacts of climate variability and extreme weather events on tropical fruit production. These models, which range from empirical statistical analyses to sophisticated dynamic simulations, capture the complex interactions between climatic factors and agricultural outcomes.
Recent trends indicate increasing delays in the onset of the rainy season and more frequent prolonged dry spells, particularly in regions like West and Central Africa (Sylla et al., 2016). Such unpredictability in weather patterns significantly affects rainfed agricultural production, including the cultivation of tropical fruits. The uncertainty surrounding the timing of the first rains has led to variations in crop performance due to delayed planting (Anwar et al., 2020). The Decision Support System for Agricultural Technology Transfer (DSSAT) and the Soil and Water Assessment Tool (SWAT) are prominent models used in agricultural meteorology (Ara et al., 2021). DSSAT, with models like CERES-Maize (Song and Jin, 2020), provides crucial data for recommendations on planting dates and optimizing crop varieties in response to weather variability. The SWAT model, renowned for its effectiveness in hydrological and environmental simulations, assists in assessing water resources and predicting the impacts of land use and management practices on environmental factors. In regions such as Central Queensland, Australia, the impacts of extreme weather events like heatwaves, cyclones, and floods on the production of tropical fruits such as pineapples, mangoes, and lychees are evident (Wheeler and Lobley, 2021). These events can alter flowering and harvesting periods, affecting the fruits’ quality and quantity. Advanced modeling techniques, such as regression models and historical trend analyses, have been instrumental in assessing the relationship between climatic factors and agricultural outputs. For instance, studies using regression models in Central Queensland have revealed moderate correlations between climate variables and crop yields (Jägermeyr et al., 2021).
Despite these advancements, meteorological models in agriculture face challenges such as spatial and temporal variability in weather patterns and the complexity of interactions between multiple climate factors (Parra-Coronado et al., 2016). These challenges can lead to uncertainties in model predictions and affect their accuracy (Tisné et al., 2020). Enhancing the accuracy and reliability of these models is essential, and it can be achieved by incorporating more detailed climatic data, refining model algorithms, and integrating these models with other agricultural data sources. Exploring the use of machine learning and artificial intelligence in meteorological modeling affecting tropical fruits could provide groundbreaking insights and solutions (Chattopadhyay et al., 2020).
5.2 Case studies of model integration in tropical fruit
A thematic mapping (Figure 6), executed using the Bibliometrix package (Aria and Cuccurullo, 2017) in the R environment, allowed for the identification and visualization of key research themes over four distinct periods: 2010-2014, 2015-2020, 2021-2022, and 2023. This analysis was based on co-word analysis, clustering keywords with high co-occurrence to represent prevalent research themes during each period (Lozano et al., 2019). Metrics such as the Weighted Inclusion Index (WII) and stability index were calculated to quantify the significance, continuity, and connection across intervals of these themes (Klarin, 2024). The Sankey diagram effectively represents the flow and transition of research themes across the specified periods (Abdelalim et al., 2017), and the width of the connection indicates their influence and continuity within the research landscape.
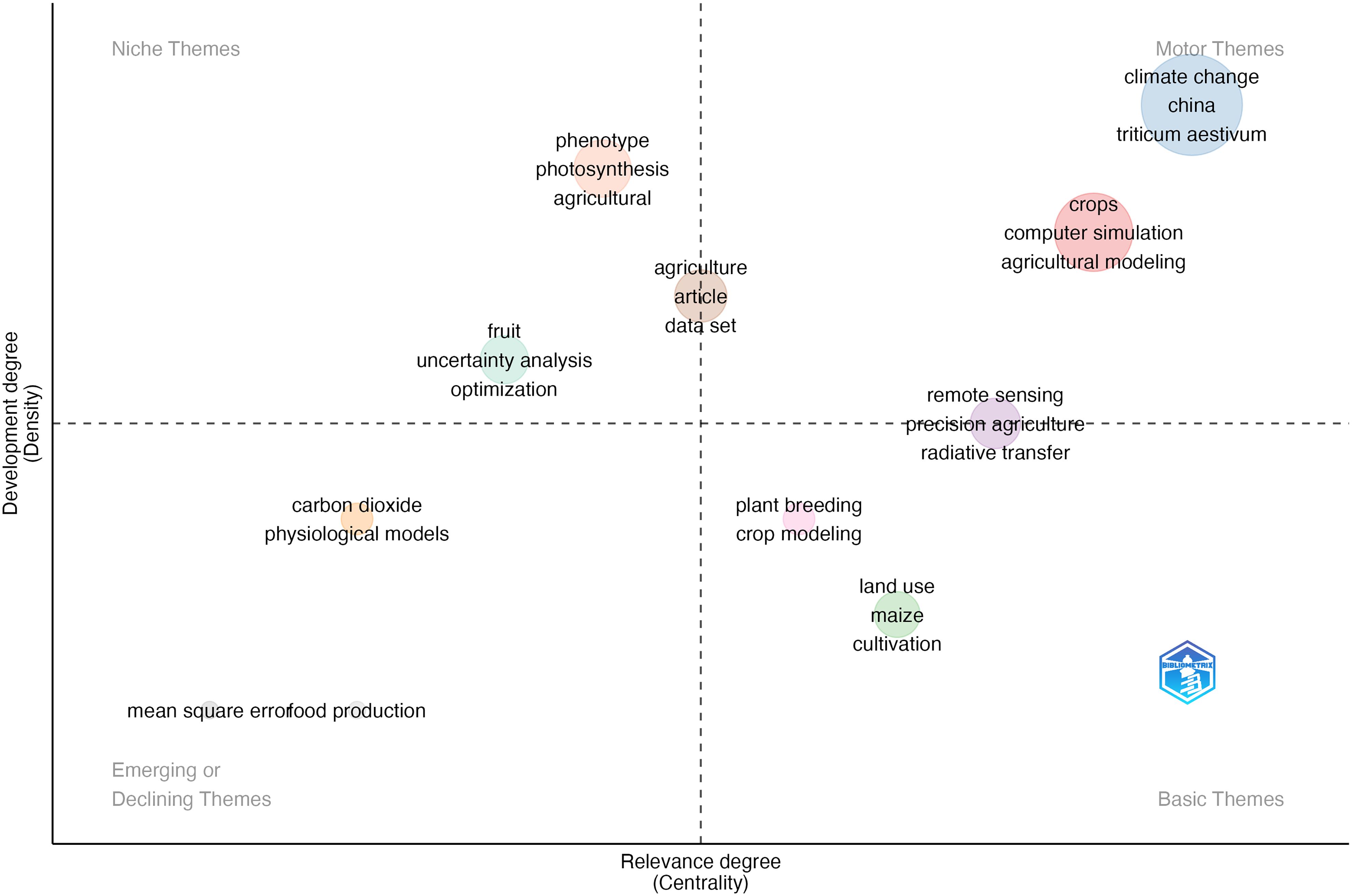
Figure 6. Thematic map of research in tropical fruit production, highlighting the centrality and density of key themes.
The analysis of annual scientific production from 2010 to 2024 reveals a growing interest in studying tropical fruit production. The data shows an initial publication of four articles in 2010, reaching a peak of six in 2014. This trend indicates an increasing academic focus on the effects of climate and weather on fruit production, the application of the DSSAT modeling system, and the broader use of modeling techniques to improve understanding and outcomes in tropical fruit agriculture.
The thematic map analysis (Figure 6) underscores the significant interdisciplinary integration of traditional agricultural techniques with advanced computational models within the research landscape. The term “crops” emerges as a central theme, with 11 occurrences and a high betweenness centrality score of 7175.77, highlighting its pivotal role in connecting various research themes. This centrality suggests that discussions surrounding “crops” serve as a foundational element, effectively bridging practical agricultural concerns with theoretical modeling approaches.
Moreover, the terms “computer simulation” and “agricultural modeling” demonstrate the increasing reliance on computational tools with occurrences. These terms underscore the growing emphasis on simulating complex agricultural ecosystems and predicting outcomes under diverse conditions. Additionally, the themes of “irrigation” and “numerical model” reflect a focused interest in optimizing water usage and enhancing the precision of crop yield forecasts through mathematical models. These metrics quantify the research community’s engagement with these topics, illuminating their strategic importance in advancing our understanding of tropical fruit production. The interconnectedness and influence of these themes are further emphasized by their closeness centrality and PageRank centrality scores, suggesting a cohesive and integrated approach to addressing the challenges of tropical fruit agriculture.
5.3 Challenges and opportunities in model integration
The thematic evolution analysis, as depicted in Figure 7, illustrates the dynamic shifts in research focus within tropical fruit production over time, spanning from 2010 to 2023. The initial period of 2010-2014 primarily centered on themes like “future” and “china,” with a Weighted Inclusion Index (WII) of 0.20 for transitions such as “china–2010-2014” to “climate–2015-2020.” This shift underscores the increasing emphasis on climate-related challenges within the Chinese context. The WII of 0.20, though moderate, highlights the growing importance of climate considerations during this period.
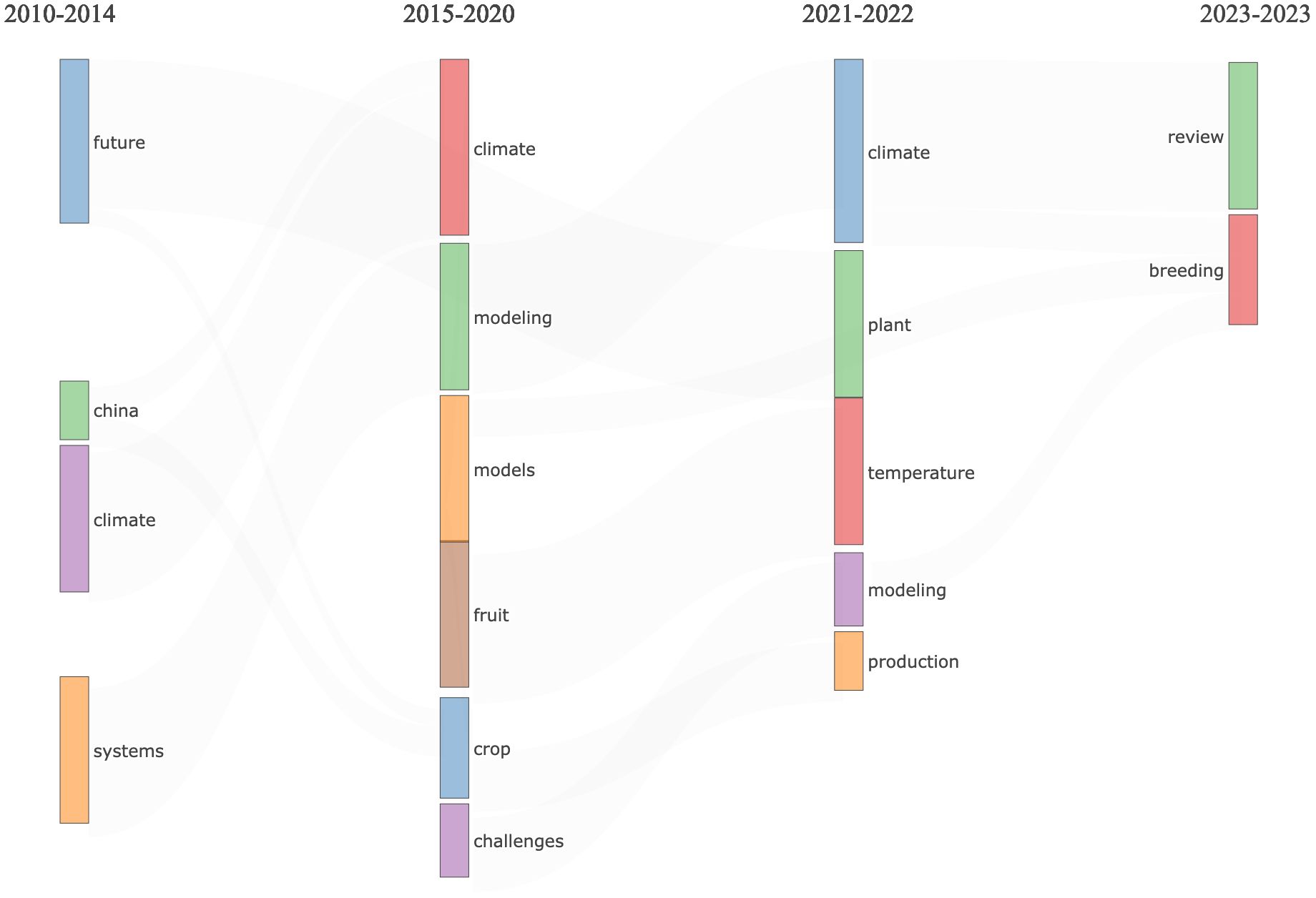
Figure 7. Thematic evolution of key themes research focus in tropical fruit production over four distinct periods: 2010-2014, 2015-2020, 2021-2022, and 2023. The thickness of the connections represents the Weighted Inclusion Index (WII), indicating the relative importance and continuity of each theme over time.
From 2015 to 2020, the research focus evolved towards more specific topics, including “climate”, “crop management”, and “modeling”. Notably, the progression from “climate–2010-2014” to “climate–2015-2020” was marked by a WII of 1.00 and an inclusion index of 0.50, indicating a sustained and deepening focus on climate change within the research community. The stability index of 0.14 for “climate” across these periods further underscores the enduring concern over climate change’s impact on agricultural productivity. The emergence of “models” with a WII of 1.00 in the same period highlights the increasing centrality of predictive modeling techniques in addressing agricultural challenges. By 2023, the thematic focus had shifted to “review” and “breeding”, indicating a move towards consolidating knowledge and advancing breeding techniques to tackle climate-related issues. This stage in the thematic evolution is marked by a WII of 1.00 for “models” and reflects the research community’s commitment to developing practical solutions. Overall, the analysis indicates responsiveness to global challenges and the trajectory towards more integrated, climate-aware, and innovation-driven approaches in tropical fruit production research.
6 Economics of fruit business through modeling
The stochastic frontier and data envelopment analyses are the most applied methods to estimate agricultural product efficiency levels, emphasizing tropical fruits. This review compiled a set of manuscripts using static and dynamic parametric and non-parametric analyses, including information on theoretical and empirical production models, key determinants of efficiency and production levels, sample size, study zones, and types of crops.
6.1 Efficiency models
There is a large body of production economics research estimating the levels of efficiency of different crops and livestock products. Some studies use parametric techniques, while others do not pose any assumption on the distribution of parameters and compute efficiency indexes in a non-parametric fashion. This study describes these alternative approaches and key findings regarding estimated efficiency scores across production systems.
6.1.1 The Stochastic Frontier Model
The Stochastic Frontier Analysis (SFA) (Coelli et al., 2005; Cornwell and Schmidt, 2008) is an economic modeling strategy that consists of two procedures: estimating a production function and the technical inefficiency model. Mathematically, the first stage is as follows:
where qi represents the output level, f(xi;β) is the deterministic part of the model with inputs xi and coefficients β, and exp(ϵi) is the exponential function of a composite error term with noise error (vi) and the inefficiency error (ui). The production frontier is given by f(xi;β)exp(vi), and the inefficiency measure is ui. Here, the researcher must assume a distribution for ϵi such as half normal, exponential, truncated normal, gamma, etc. A Maximum Likelihood (ML) strategy estimates this model, giving its asymptotic properties, and the estimates for the inefficiency scores are obtained by the conditional distribution of ui, giving the estimated ϵi (Greene, 2008; Belotti et al., 2013).
SFA can estimate the production function using different functional forms such as Cobb-Douglas, Translog, or CES (Pascoe et al., 2003); specifically, tropical fruit production studies have implemented Cobb-Douglas function most frequently (Trujillo and Iglesias, 2013; Hossain et al., 2015; Melo-Becerra and Orozco-Gallo, 2017; Roco et al., 2017; Balogun et al., 2018; Kiet et al., 2020; Muhamad et al., 2023). Finally, the SFA provides a model of technical inefficiency scores explained by a set of exogenous variables to find the main determinants of this inefficiency within farms.
Although production economics literature has widely implemented SFA, there are scarce SFA studies related to tropical (fruit) production in the Latin American region. Few Latin American research uses SFA to produce coffee, oil palm, pineapple, and vineyard (Trujillo and Iglesias, 2013; Melo-Becerra and Orozco-Gallo, 2017; Roco et al., 2017; Lizarraga Hernández, 2020). Extending our search of references to other regions, SFA provides evidence of efficiency levels of banana, mango, watermelon, eggplant, groundnut, etc. Frontier models usually estimate the production function using either per-ha output level or per-ha monetary value as a dependent variable, where the covariates are land, labor, capital, fertilizer/pesticide, seeds, irrigation, and weather variables. A common finding in our review is that most farms are functioning below the production frontier for tropical fruits, where we compute an efficiency level of around 70%, on average (i.e., the firm can be fully efficient by reducing inputs by 30%). Such efficiency level is consistent with metanalysis research, which reports an average level of efficiency of 74.2% for agricultural farms, using published manuscripts between 1981 and 2014 (Bravo-Ureta et al., 2017).
6.1.2 The Data Envelopment Analysis
Different studies have applied Data Envelopment Analysis (DEA) in agriculture, banking, education, energy, health, etc (Cooper et al., 2011). However, as in the SFA literature, we do not find much DEA research related to (tropical) fruit production.
As a non-parametric alternative to SFA, DEA is a linear programming, where the decision-making units (DMUs) either minimize the use of input levels given an output level (i.e., input-oriented DEA) or maximize output level given a level of inputs (i.e., output-oriented DEA), with a production technology showing a constant return to scale (CCR DEA model, named after the work of Charnes, Cooper, and Rhodes in the 1970s) or variable return to scale (BCC DEA model, due the study of Banker, Charnes and Cooper) (Cooper et al., 2011). The DEA mathematical model generally provides the technical efficiency score, the maximum production levels possible given a set of inputs, or the minimum uses of inputs given a fixed output level. When using the BCC DEA model or a variable return to scale in the mathematical model, this technical efficiency can be divided into pure technical efficiency (i.e., technical efficiency with a variable return to scale) and scale efficiency (i.e., having the optimal production level by having the ideal scale size) (Boakye et al., 2024). Alternatively, if we count for precise price information, which is not easy to obtain due to market failure, the researcher can estipulate a DEA models that minimize cost or maximize revenue or profits so that cost efficiency (i.e., producing at optimal levels by saving production costs), allocative efficiency (i.e., using optimal levels of inputs given their prices) and profit efficiency (i.e., obtaining the maximum profits from its production levels) can be computed (Fethi and Pasiouras, 2010; Cooper et al., 2011).
Although the efficiency scores are slightly different, a researcher can use either input-oriented or output-oriented DEA depending on what the DMUs have more control over, inputs or outputs. Most studies used input-orientated DEA models in our literature search and showed technical and scale efficiency scores. Studies from Argentina, Brazil, Colombia, Ecuador, Guatemala, and Mexico analyze the efficiency of a variety of crops such as avocado, banana, cocoa, coffee, grapefruit, lemon, orange, tangerine, passion fruit, and plantain (Barreno and Marroquin, 2012; Novo et al., 2013; Guidek et al., 2017; Valencia and Duana, 2019; Barajas and Pabuena, 2023; Varela, 2023). These works use land size, labor, fertilizers, animal traction, and consumed fuel as input variables, and production level in tons (per ha) or the production’s economic (export) value as the output variables. Again, there is space to gain efficiency levels across crops, where the average technical efficiency is 71.79%, meaning that farms can reduce about 29% input levels and still produce the same output levels. Barajas and Pabuena (2023) show the lowest technical efficiency score for tangerine, 17%. Meanwhile, the most efficient crops are avocado, coffee, cocoa, lemon, and orange, with a score of 100% within our literature search (Valencia and Duana, 2019; Barajas and Pabuena, 2023). Finally, some DEA studies also take advantage of econometric models (Tobit models) to explore how weather variables (precipitation), farms, and farmers’ characteristics (e.g., age, education, electric machines), among others, explain these estimated DEA efficiency levels (Barajas and Pabuena, 2023; Varela, 2023).
6.2 Time series models for tropical fruit production
In addition to economic models that estimate efficiency levels, studies often utilize time series data to model dynamic relationships between crop production and key determinants or to Yield prediction levels and crop prices. For fruit production, various manuscripts have estimated multivariate models to measure the effects of socioeconomic variables—such as prices, exchange rates, labor force availability, and production costs—as well as weather and environmental variables, including temperature, precipitation, and CO2 emissions, among others (Gay et al., 2006; Rickard and Pierre, 2008; Howai et al., 2013). Other studies have focused on forecasting production levels and prices, utilizing univariate models for this purpose (Luis-Rojas et al., 2020; Cancino et al., 2022; Pacheco-Sánchez et al., 2023). Furthermore, some authors combine time series models with machine learning techniques (Abdul et al., 2018; Rathod and Mishra, 2018; Khan et al., 2021; Kumari et al., 2023).
The tropical literature on time series models and fruit production presents a broader reach than the efficiency estimation literature. We find references using data from Colombia, Ecuador, Mexico, Trinidad and Tobago, among other countries (Cancino et al., 2021, 2022; Gay et al., 2006; Orozco-Abarca, 2007; Rickard and Pierre, 2008; Kleemann and Effenberger, 2010; Howai et al., 2013; Luis-Rojas et al., 2020; Paniagua-Molina and Solís-Rivera, 2020; Paniagua-Molina and Solórzano-Thompson, 2020; Pacheco-Sánchez et al., 2023). These time series works have examined the production of banana passion fruit, blackberry, cocoa, coffee, limes, oil palm, and vanilla. Some studies apply multivariate time series approaches to understand the impact of key independent variables, using log-log regressions, linear and quadratic functions, or more sophisticated methods such as Vector Autoregressive Models (VARs).
When the purpose is to forecast yields or prices, studies implement the Box-Jenkins method or the Autoregressive Integrated Moving Average (ARIMA). These econometric strategies have allowed researchers to quantify the impact of economic and weather variables on output levels. For instance, temperature and rainfall patterns are key inputs for coffee and cocoa output levels (see Gay et al., 2006; Rickard and Pierre, 2008; Howai et al., 2013). Meanwhile, economic determinants such as income, output and input prices, levels of debt, and labor force availability, among others, explain significant production or profitability of cocoa, coffee, banana passion fruit, and oil palm (Rickard and Pierre, 2008; Howai et al., 2013; Paniagua-Molina and Solórzano-Thompson, 2020; Cancino et al., 2021). Finally, ARIMA models predict the production levels of blackberry and vanilla (Luis-Rojas et al., 2020; Cancino et al., 2022), and even the effect of weevil infestation in oil palm (Pacheco-Sánchez et al., 2023).
Literature search in other regions described the implementation of time series with machine learning methods. These studies have forecasted the production of apples, bananas, citrus, grapes, mango, and pears, using time series techniques such as ARIMA and Seasonal ARIMA (SARIMA), Autoregressive Distributed lag-bound testing (ARDL), Autoregressive Conditional Heteroscedasticity (ARCH), Generalized Autoregressive Conditional Heteroscedasticity (GARCH). At the same time, they have included machine learning methods such as Support Vector Machines (SVM), Logistic regression, K-nearest neighbors classifier, Decision tree classifier, and Random forest classifier, Artificial Neural Network (ANN) and Recurrent Neural Network (RNN) (Abdul et al., 2018; Rathod and Mishra, 2018; Khan et al., 2021; Kumari et al., 2023). These studies claim that the combination of time series and machine learning methods outperforms the predictive power of individual time series estimation.
7 Conclusion
Modeling and artificial intelligence (AI) are revolutionizing fruit orchard management and optimization for fruit growers. This transformation involves advancing from specialized models, which address plant functions, environmental interactions, and pest and disease dynamics, to multidisciplinary research and platforms that adopt a more holistic approach. These comprehensive tools extend beyond basic monitoring, recognizing the vital influence of soil health and weather patterns on tropical fruit yields; incorporating comprehensive soil assessments and precise meteorological data into these advanced models enhances predictive accuracy and supports sustainable orchard management practices. Modeling on “Tropical Fruit Forecasting” requires sophisticated algorithms to examine past data, present weather conditions, and soil characteristics, offering comprehensive predictions for the growth and yield of fruit crops (Gómez-Lagos et al., 2023). Future research is considering large-scale and multi-scale models, improving the representation of physiological processes, tree architecture, physiological responses to climate change and management practices, and enabling the identification of tree-fruit anomalies through image analysis, satellite data, low-cost sensors, and automated recommendations (Grisafi et al., 2022). Moreover, yield data prediction is extremely valuable because it directly influences economic models for sales estimation, which are useful for market pricing (Tanimoto and Yoshida, 2024). Economic models show an opportunity for efficiency improvements across fruit crops. Agricultural sciences have now advanced toward producing detailed data to analyze farming systems and efficiency models more precisely. Unfortunately, such resources are not evenly available worldwide, especially for farmers in the tropics (Wolfert et al., 2017; Jones et al., 2017a). Efforts to access specialized human capital, digital infrastructure, and real-time and field-scale data are all necessary for efficiency gains across tropical regions.
Author contributions
DM-C: Conceptualization, Investigation, Project administration, Supervision, Writing – original draft, Writing – review & editing. YG: Investigation, Validation, Writing – original draft, Writing – review & editing. MA-M: Investigation, Resources, Software, Visualization, Writing – original draft, Writing – review & editing. DL: Investigation, Software, Validation, Writing – original draft, Writing – review & editing. DP: Investigation, Software, Visualization, Writing – original draft, Writing – review & editing. JA-S: Formal analysis, Investigation, Software, Writing – original draft, Writing – review & editing.
Funding
The author(s) declare that financial support was received for the research or authorship of this article. This review of multidisciplinary research was funded thanks to the Agrarian University of Ecuador (Resolution 126-2023).
Acknowledgments
The authors thank the Agrarian University of Ecuador and collaborators for their support and time.
Conflict of interest
The authors declare that the review was conducted in the absence of any commercial or financial relationships that could be construed as a potential conflict of interest.
Generative AI statement
Generative AI was used for the creation of this Manuscript. Authors used ChatGPT4.0 for translation and Grammarly for grammatical corrections.
Publisher’s note
All claims expressed in this article are solely those of the authors and do not necessarily represent those of their affiliated organizations, or those of the publisher, the editors and the reviewers. Any product that may be evaluated in this article, or claim that may be made by its manufacturer, is not guaranteed or endorsed by the publisher.
References
Abadi B., Mahdavian S., Fattahi F. (2021). The waste management of fruit and vegetable in wholesale markets: Intention and behavior analysis using path analysis. J. Clean. Product. 279, 123802. doi: 10.1016/j.jclepro.2020.123802
Abdelalim A., O’Brien W., Shi Z. (2017). Development of sankey diagrams to visualize real hvac performance. Energy Build. 149, 282–297. doi: 10.1016/j.enbuild.2017.05.040
Abdul R., Zhang D., Luan J. (2018). The production and prediction of major Chinese agricultural fruits using an econometric analysis and machine learning technique. Afr. J. Agric. Res. 13, 2134–2145. doi: 10.5897/ajar2018.13491
Ackerman J. D., Montalvo A. M. (1990). Short-and long-term limitations to fruit production in a tropical orchid. Ecology 71, 263–272. doi: 10.2307/1940265
Adeyemi O., Grove I., Peets S., Domun Y., Norton T. (2018). enDynamic neural network modelling of soil moisture content for predictive irrigation scheduling. Sensors 18, 3408. doi: 10.3390/s18103408
Agustí M., Primo-Millo E. (2020). “Flowering and fruit set,” in The Genus Citrus (Cambridge, UK: Woodhead Publishing), 219–244.
Ahuja L., Rojas K. W., Hanson J. D. (2000). Root zone water quality model: modelling management effects on water quality and crop production (St. Joseph, Michigan, USA: Water Resources Publication).
Alcaraz M. L., Hormaza J. I. (2021). Fruit set in avocado: Pollen limitation, pollen load size, and selective fruit abortion. Agronomy 11, 1603. doi: 10.3390/agronomy11081603
Alewell C., Borrelli P., Meusburger K., Panagos P. (2019). Using the USLE: Chances, challenges and limitations of soil erosion modelling. Int. Soil Water Conserv. Res. 7, 203–225. doi: 10.1016/j.iswcr.2019.05.004
Ali A. K., Abdulkadr A. A., Habtie G. M., Asfaw D. M. (2024). Evaluate the current and predict the future real gdp of afar regional state, Ethiopia. Discov. Sustainabil. 5, 9. doi: 10.1007/s43621-024-00181-x
Aline U., Bhattacharya T., Faqeerzada M. A., Kim M. S., Baek I., Cho B.-K. (2023). Advancement of non-destructive spectral measurements for the quality of major tropical fruits and vegetables: a review. Front. Plant Sci. 14. doi: 10.3389/fpls.2023.1240361
Altendorf S. (2017). “Global prospects for major tropical fruits,” in The food outlook: biannual report on global food markets (The Food and Agriculture Organization of the United Nations (FAO, Rome), 68–81.
Amir-Hamjah M. (2014). Forecasting major fruit crops productions in Bangladesh using box-jenkins ARIMA model. J. Econ. Sustain. Dev. 5, 96–108.
Anbumozhi A., Shanthini A. (2023). Adoption of novel technologies to boost precision agriculture (bpa) using internet of things (iot). ITM Web Conf. 56, 5019. doi: 10.1051/itmconf/20235605019
Anderson N. T., Walsh K. B., Wulfsohn D. (2021). Technologies for forecasting tree fruit load and harvest timing—from ground, sky and time. Agronomy 11, 1409. doi: 10.3390/agronomy11071409
Anwar M. R., Wang B., Li Liu D., Waters C. (2020). Late planting has great potential to mitigate the effects of future climate change on Australian rain-fed cotton. Sci. Total Environ. 714, 136806. doi: 10.1016/j.scitotenv.2020.136806
Ara I., Turner L., Harrison M. T., Monjardino M., DeVoil P., Rodriguez D. (2021). Application, adoption and opportunities for improving decision support systems in irrigated agriculture: A review. Agric. Water Manage. 257, 107161. doi: 10.1016/j.agwat.2021.107161
Aria M., Cuccurullo C. (2017). bibliometrix: An r-tool for comprehensive science mapping analysis. J. Inform. 11, 959–975. doi: 10.1016/j.joi.2017.08.007
Atzberger C. (2013). Advances in remote sensing of agriculture: Context description, existing operational monitoring systems and major information needs. Remote Sensing 5, 949–981. doi: 10.3390/rs5020949
Aworka R., Cedric L. S., Adoni W. Y. H., Zoueu J. T., Mutombo F. K., Kimpolo C. L. M., et al. (2022). Agricultural decision system based on advanced machine learning models for yield prediction: Case of East African countries. Smart Agric. Technol. 2, 100048. doi: 10.1016/j.atech.2022.100048
Balogun O. L., Adewuyi S. A., Disu O. R., Afodu J. O., Ayo-Bello T. A. (2018). Profitability and technical efficiency of pineapple production in Ogun state, Nigeria. Int. J. Fruit Sci. 18, 436–444. doi: 10.1080/15538362.2018.1470594
Barajas L., Pabuena J. (2023). Determinantes de la eficiencia de la agricultura del nororiente Colombiano en el año 2019, 949–981.
Barbault N., Dupraz C., Lauri P., Gosme M. (2024). Insights into fruit tree models relevant to simulate fruit tree-based agroforestry systems. Agroforestry Syst. 98, 817–835. doi: 10.1007/s10457-024-00953-4
Barbosa A., Trevisan R., Hovakimyan N., Martin N. F. (2020). Modeling yield response to crop management using convolutional neural networks. Comput. Electron. Agric. 170, 105197. doi: 10.1016/j.compag.2019.105197
Barman U., Pathak C., Mazumder N. K. (2023). Comparative assessment of pest damage identification of coconut plant using damage texture and color analysis. Multimed. Tools Appl. 82, 25083–25105. doi: 10.1007/s11042-023-14369-2
Barreno A. E. F., Marroquin C. A. (2012). Caracterización económica de la producción comercial del cultivo de papaya (carica papaya) en el departamento de petén, Guatemala.
Behera S. K., Rath A. K., Sethy P. K. (2020). Fruit recognition using support vector machine based on deep features. Karbala Int. J. Modern Sci. 6, 235–245. doi: 10.33640/2405-609X.1675
Belotti F., Daidone S., Ilardi G., Atella V. (2013). Stochastic frontier analysis using stata. Stata J. 13, 719–758. doi: 10.1177/1536867X1301300404
Benkeblia N. (2021). Physiological and biochemical response of tropical fruits to hypoxia/anoxia. Front. Plant Sci. 12, 670803. doi: 10.3389/fpls.2021.670803
Bennett N. D., Croke B. F. W., Guariso G., Guillaume J. H. A., Hamilton S. H., Jakeman A. J., et al. (2013). Characterising performance of environmental models. Environ. Model. Softw. 40, 1–20. doi: 10.1016/j.envsoft.2012.09.011
Berk R. A. (2020). “Neural Networks,” in Statistical Learning from a Regression Perspective (Springer International Publishing, Cham), 361–399. doi: 10.1007/978-3-030-40189-4{\-}8
Berman N., Couttenier M., Leblois A., Soubeyran R. (2023). Crop prices and deforestation in the tropics. J. Environ. Econ. Manage. 119, 1–12. doi: 10.5281/zenodo.6078914
Bezabh Y. A., Ayalew A. M., Abuhayi B. M., Demlie T. N., Awoke E. A., Mengistu T. E. (2024). Classification of mango disease using ensemble convolutional neural network. Smart Agric. Technol. 8. doi: 10.1016/j.atech.2024.100476
Bezerra A. D. M., Pacheco Filho A. J., Bomfim I. G., Smagghe G., Freitas B. M. (2019). Data relating to threats to passion fruit production in the neotropics due to agricultural area loss and pollinator mismatch as consequence of climate changes. Data Brief. 169, 49–57. doi: 10.1016/j.dib.2019.103802
Bhat R., Paliyath G. (2016). “Fruits of Tropical Climates: Biodiversity and Dietary Importance,” in Encyclopedia of Food and Health. Eds. Caballero B., Finglas P. M., Toldrá F. (Academic Press, Oxford), 138–143. doi: 10.1016/B978-0-12-384947-2.00337-8
Bibwe B., Mahawar M. K., Jalgaonkar K., Meena V. S., Kadam D. M. (2022). Mass modeling of guava (cv. allahabad safeda) fruit with selected dimensional attributes: regression analysis approach. J. Food Process Eng. 45, e13978. doi: 10.1111/jfpe.13978
Boakye K., Lee Y.-F., Annor F. F., Dadzie S. K. N., Salifu I. (2024). Data envelopment analysis (dea) to estimate technical and scale efficiencies of smallholder pineapple farmers in Ghana. Agriculture 14, 1032. doi: 10.3390/agriculture14071032
Bons H. K., Kaur M. (2020). Role of plant growth regulators in improving fruit set, quality and yield of fruit crops: a review. J. Hortic. Sci. Biotechnol. 95, 137–146. doi: 10.1080/14620316.2019.1660591
Borrelli P., Robinson D. A., Panagos P., Lugato E., Yang J. E., Alewell C., et al. (2020). Land use and climate change impacts on global soil erosion by water, (2015-2070). Proc. Natl. Acad. Sci. 117, 21994–22001. doi: 10.1073/pnas.2001403117
Bossel H. (1996). Treedyn3 forest simulation model. Ecol. Model. 90, 187–227. doi: 10.1016/0304-3800(95)00139-5
Boudon F., Persello S., Jestin A., Briand A.-S., Grechi I., Fernique P., et al. (2020). V-mango: a functional–structural model of mango tree growth, development and fruit production. Ann. Bot. 126, 745–763. doi: 10.1093/aob/mcaa089
Bravo-Ureta B. E., Jara-Rojas R., Lachaud M. A., Moreira V. H. (2017). A meta analysis of farm efficiency: evidence from the production frontier literature. Research Report. 5, 1–66. doi: 10.22004/ag.econ.290067
Brisson N., Mary B., Ripoche D., Jeuffroy M. H., Ruget F., Nicoullaud B., et al. (1998). Stics: a generic model for the simulation of crops and their water and nitrogen balances. i. theory and parameterization applied to wheat and corn. Agronomie 18, 311–346. doi: 10.1051/agro:19980501
Cai Y., Zheng W., Zhang X., Zhangzhong L., Xue X. (2019). enResearch on soil moisture prediction model based on deep learning. PloS One 14, e0214508. doi: 10.1371/journal.pone.0214508
Cancino S., Escalante G. O. C., Ricketts D. F. C. (2021). Regression analysis of the factors affecting economic profitability of banana passion fruit production. Dictamen Libre, 69–82. doi: 10.18041/2619-4244/dl.29.7861
Cancino S. E., Escalante G. O. C., Ricketts D. F. C. (2022). Un modelo box jenkins arima para modelar y pronosticar la producción de mora de castilla en Colombia. ECONÓMICAS CUC 44, 129-139. doi: 10.17981/econcuc.44.1.2023.econ.4
Castano J., Neciu A. (2022). Midterm review of the 2020 round of censuses of agriculture. Stat. J. IAOS 38, 201–209. doi: 10.3233/SJI-210884
Chabalala Y., Adam E., Ali K. A. (2022). Machine learning classification of fused sentinel-1 and sentinel-2 image data towards mapping fruit plantations in highly heterogenous landscapes. Remote Sens. 14, 2621. doi: 10.3390/rs14112621
Chattopadhyay A., Hassanzadeh P., Pasha S. (2020). Predicting clustered weather patterns: A test case for applications of convolutional neural networks to spatio-temporal climate data. Sci. Rep. 10, 1317. doi: 10.1038/s41598-020-57897-9
Chemura A., Gleixner S., Gornott C. (2024). Dataset of the suitability of major food crops in Africa under climate change. Sci. Data 11, 1–13. doi: 10.1038/s41597-024-03118-1
Cilas C., Goebel F.-R., Babin R., Avelino J. (2016). "Tropical crop pests and diseases in a climate change setting—a few examples". In: Torquebiau E. (eds) Climate Change and Agriculture Worldwide. (Dordrecht: Springer), pp. 73-82. doi: 10.1007/978-94-017-7462-8_6
Cillis D., Maestrini B., Pezzuolo A., Marinello F., Sartori L. (2018). Modeling soil organic carbon and carbon dioxide emissions in different tillage systems supported by precision agriculture technologies under current climatic conditions. Soil Tillage Res. 183, 51–59. doi: 10.1016/j.still.2018.06.001
Coelli T. J., Rao D. P., O’Donnell C. J., Battese G. E. (2005). Chapter 9. Stochastic Frontier Analysis. 2nd ed. (New York, NY, USA: Springer Science+Business Media, Inc), 241–261. doi: 10.2307/2531310
Cooper W., Seiford L., Zhu J. (2011). Handbook on data envelopment analysis. 2nd ed. Vol. 164 (New York, NY, USA: Springer US). doi: 10.1007/978-1-4419-6151-8
Cornwell C., Schmidt P. (2008). Stochastic Frontier Analysis and Efficiency Estimation (Berlin, Heidelberg: Springer), Vol. 46, 697–123.
Crespo-Pérez V., Dangles O., Régnierè J., Chuine I. (2013). Modeling temperature-dependent survival with small datasets: Insights from tropical mountain agricultural pests. Bull. Entomol. Res. 103, 336–343. doi: 10.1017/S0007485312000776
Deere C. D., Twyman J. (2014). ¿quién toma las decisiones agrícolas? mujeres propietarias en el Ecuador. Agricult. Sociedad y Desarrollo 11, 425–440. doi: 10.22231/asyd.v11i3.94
de Mello Prado R., Rozane D. E. (2020). Leaf analysis as diagnostic tool for balanced fertilization in tropical fruits. Fruit Crops, 131–143. doi: 10.1016/B978-0-12-818732-6.00011-3
Diggle A. J. (1988). enROOTMAP—a model in three-dimensional coordinates of the growth and structure of fibrous root systems. Plant Soil 105, 169–178. doi: 10.1007/BF02376780
Duarte-Carvajalino J. M., Paramo-Alvarez M., Ramos-Calderón P. F., González-Orozco C. E. (2021). Estimation of canopy attributes of wild cacao trees using digital cover photography and machine learning algorithms. IForest 14, 517–521. doi: 10.3832/ifor3936-014
Dunbabin V. M., Postma J. A., Schnepf A., Pagès L., Javaux M., Wu L., et al. (2013). enModelling root–soil interactions using three–dimensional models of root growth, architecture and function. Plant Soil 372, 93–124. doi: 10.1007/s11104-013-1769-y
Eftekhari M. S. (2022). “Impacts of Climate Change on Agriculture and Horticulture,” in Climate Change: The Social and Scientific Construct. (Cham, Switzerland: Springer). doi: 10.1007/978-3-030-86290-98
Erazo-Mesa E., Ramírez-Gil J. G., Sánchez A. E. (2021). Avocado cv. hass needs water irrigation in tropical precipitation regime: Evidence from Colombia. Water 13, 1–17. doi: 10.3390/w13141942
Fethi M. D., Pasiouras F. (2010). Assessing bank efficiency and performance with operational research and artificial intelligence techniques: A survey. European Journal of Operational Research. 204, 189–198. doi: 10.1016/j.ejor.2009.08.003
Fukuda S., Spreer W., Yasunaga E., Yuge K., Sardsud V., Müller J. (2013). Random Forests modelling for the estimation of mango (Mangifera indica L. cv. Chok Anan) fruit yields under different irrigation regimes. Agric. Water Manage. 116, 142–150. doi: 10.1016/j.agwat.2012.07.003
Gallardo M., Elia A., Thompson R. B. (2020). Decision support systems and models for aiding irrigation and nutrient management of vegetable crops. Agric. Water Manage. 240, 106209. doi: 10.1016/j.agwat.2020.106209
García Y., Ramírez W., Sánchez S. (2012). Indicadores de la calidad de los suelos: una nueva manera de evaluar este recurso. Pastos y Forrajes 35, 125–138.
Garrido A., Conde A., Serôdio J., De Vos R. C., Cunha A. (2023). Fruit photosynthesis: more to know about where, how and why. Plants 12, 2393. doi: 10.3390/plants12132393
Gay C., Estrada F., Conde C., Eakin H., Villers L. (2006). Potential impacts of climate change on agriculture: A case of study of coffee production in Veracruz, Mexico. Climatic Change. 79, 259–288. doi: 10.1007/s10584-006-9066-x
Gene Albrigo L., Galán Saúco V. (2004). Flower bud induction, flowering and fruit-set of some tropical and subtropical fruit tree crops with special reference to citrus. Acta Hortic. 632, 81–90. doi: 10.17660/ActaHortic.2004.632.10
Gill M. K., Asefa T., Kemblowski M. W., McKee M. (2006). enSoil moisture prediction using support vector machines1. JAWRA J. Am. Water Resour. Assoc. 42, 1033–1046. doi: 10.1111/j.1752-1688.2006.tb04512.x
Goldschmidt E. E., Lakso A. N. (2005). “Fruit tree models: scope and limitations,” in Information and Communication Technology (ICT) Development and Adoption: Perspectives of Technological Innovation (European Federation for Information Technologies in Agriculture, Food and the Environment), 1–19.
Gómez-Lagos J. E., González-Araya M. C., Ortega Blu R., Acosta Espejo L. G. (2023). A new method based on machine learning to forecast fruit yield using spectrometric data: analysis in a fruit supply chain context. Precis. Agric. 24, 326–352. doi: 10.1007/s11119-022-09947-7
González-Orozco C. E., Porcel M., Velásquez D. F. A., Orduz-Rodríguez J. O. (2020). Extreme climate variability weakens a major tropical agricultural hub. Ecol. Indic. 111, 106015. doi: 10.1016/j.ecolind.2019.106015
Greene W. H. (2008). Maximum Likelihood Estimation, sixth edn. (Upper Saddle River, New Jersey: Pearson Education, Inc.), 770–859.
Grisafi F., DeJong T. M., Tombesi S. (2021). Fruit tree crop models: an update. Tree Physiol. 42, 441–457. doi: 10.1093/treephys/tpab126
Grisafi F., DeJong T. M., Tombesi S. (2022). Fruit tree crop models: an update. Tree Physiol. 42, 441–457. doi: 10.1093/treephys/tpab126
Guidek R., Adams J., Dominguez G., Marcos B., Luca J. D. (2017). Análisis de sensibilidad mediante propiedades de envolvente de datos. Investigación Operativa 25, 23–41.
Gupta D. K., Keerthika A., Gupta C. K., Shukla A. K., Mohamed M. N., Jangid B., et al. (2021). “Climate Change and Its Impact on Fruit Crops,” in Horticulture Based Integrated Farming Systems (CRC Press, London), 223–234. doi: 10.1201/9781003245810-19
Gutierrez A. P., Ponti L., Neteler M., Suckling D. M., Cure J. R. (2021). Invasive potential of tropical fruit flies in temperate regions under climate change. Commun. Biol. 4, 1141. doi: 10.1038/s42003-021-02599-9
Guzmán-Armenteros T. M., Ramos-Guerrero L. A., Guerra L. S., Ruales J. (2023). Optimization of cacao beans fermentation by native species and electromagnetic fields. Heliyon. 9, e15065. doi: 10.1016/j.heliyon.2023.e15065
Haque S., Akbar D., Kinnear S. (2020). The variable impacts of extreme weather events on fruit production in subtropical Australia. Scientia Hortic. 262, 109050. doi: 10.1016/j.scienta.2019.109050
Haque M. A., Sakimin S. Z. (2022). Planting arrangement and effects of planting density on tropical fruit crops—a review. Horticulturae 8, 485. doi: 10.3390/horticulturae8060485
Harris J., van Zonneveld M., Achigan-Dako E. G., Bajwa B., Brouwer I. D., Choudhury D., et al. (2022). Fruit and vegetable biodiversity for nutritionally diverse diets: Challenges, opportunities, and knowledge gaps. Global Food Secur. 33, 100618. doi: 10.1016/j.gfs.2022.100618
Hasimi L., Zavantis D., Shakshuki E., Yasar A. (2024). Cloud computing security and deep learning: An ann approach. Proc. Comput. Sci. 231, 40–47. doi: 10.1016/j.procs.2023.12.155
He L., Fang W., Zhao G., Wu Z., Fu L., Li R., et al. (2022). Fruit yield prediction and estimation in orchards: A state-of-the-art comprehensive review for both direct and indirect methods. Comput. Electron. Agric. 195, 106812. doi: 10.1016/j.compag.2022.106812
He G., Sun A., Lu W. (2023). Research explosion: More effort to climb onto shoulders of the giant. Comput. Electron. Agric. 195, 106812.
Heaton J., Goodfellow I., Bengio Y., Courville A. (2018). Deep learning. Genet. Program. Evolvable. Mach. 19, 305–307. doi: 10.1007/s10710-017-9314-z
Heuvelink E. (1999). Evaluation of a dynamic simulation model for tomato crop growth and development. Ann. Bot. 83, 413–422. doi: 10.1006/anbo.1998.0832
Ho P. L., Tran D. T., Hertog M. L., Nicolaï B. M. (2020). Modelling respiration rate of dragon fruit as a function of gas composition and temperature. Scientia Hortic. 263, 109138. doi: 10.1016/j.scienta.2019.109138
Holt J., Leach A., Johnson S., Tu D., Nhu D., Anh N., et al. (2018). Bayesian networks to compare pest control interventions on commodities along agricultural production chains. Risk Anal. 38, 297–310. doi: 10.1111/risa.2018.38.issue-2
Hoogenboom G., White J. W., Jones J. W., Boote K. J. (2003). Beangro V1.01 dry bean crop growth simulation model: user’s guide. (Florence, Italy). Available online at: https://www.sciencedirect.com/journal/european-journal-of-agronomy
Hossain M. M., Alam M. A., Alam M. A., Uddin M. K. (2015). Application of stochastic frontier production function on small banana growers of kushtia district in Bangladesh. J. Stat Appl. Probabil. Int. J. 4, 337–342. doi: 10.12785/jsap/040218
Howai N., Pemberton C., Patterson-Andrews H. (2013). The supply response of cocoa farmers to economic, social and other variables in Trinidad and Tobago. Trop. Agric. 90, 223–234.
Humphrey G. B., Maier H. R., Wu W., Mount N. J., Dandy G. C., Abrahart R. J., et al. (2017). Improved validation framework and R-package for artificial neural network models. Environ. Model. Softw. 92, 82–106. doi: 10.1016/j.envsoft.2017.01.023
Ip R. H., Ang L. M., Seng K. P., Broster J. C., Pratley J. E. (2018). Big data and machine learning for crop protection. Comput. Electron. Agric. 151, 376–383. doi: 10.1016/j.compag.2018.06.008
Jägermeyr J., Müller C., Ruane A. C., Elliott J., Balkovic J., Castillo O., et al. (2021). Climate impacts on global agriculture emerge earlier in new generation of climate and crop models. Nat. Food 2, 873–885. doi: 10.1038/s43016-021-00400-y
Jasoliya D., Untaroiu A., Untaroiu C. (2024). A review of soil modeling for numerical simulations of soil-tire/agricultural tools interaction. J. Terramech. 111, 41–64. doi: 10.1016/j.jterra.2023.09.003
Jawade P. B., Chaugule D., Patil D., Shinde H. (2020). "Disease prediction of mango crop using machine learning and ioT". In Advances in decision sciences, image processing, security and computer vision: international conference on emerging trends in engineering (ICETE). Springer International Publishing. Vol. 1, 254–260. doi: 10.1007/978-3-030-24322-7{\}33
Jayasinghe S. L., Ranawana C. J. K., Liyanage I. C., Kaliyadasa P. E. (2022). Growth and yield estimation of banana through mathematical modelling: A systematic review. J. Agric. Sci. 160, 152–167. doi: 10.1017/S0021859622000259
Jiang Y., Li C. (2020). Convolutional neural networks for image-based high-throughput plant phenotyping: A review. Plant Phenomics. 2020, 1–22. doi: 10.34133/2020/4152816
Jones J. W., Antle J. M., Basso B., Boote K. J., Conant R. T., Foster I., et al. (2017a). Brief history of agricultural systems modeling. Agric. Syst. 155, 240–254. doi: 10.1016/j.agsy.2016.05.014
Jones J. W., Antle J. M., Basso B., Boote K. J., Conant R. T., Foster I., et al. (2017b). Toward a new generation of agricultural system data, models, and knowledge products: State of agricultural systems science. Agric. Syst. 155, 269–288. doi: 10.1016/j.agsy.2016.09.021
Jones J. W., Hoogenboom G., Porter C. H., Boote K. J., Batchelor W. D., Hunt L. A., et al. (2003). The dssat cropping system model. Eur. J. Agron. 18, 235–265. doi: 10.1016/S1161-0301(02)00107-7
Kamilaris A., Kartakoullis A., Prenafeta-Boldú F. X. (2017). A review on the practice of big data analysis in agriculture. Comput. Electron. Agric. 143, 23–37. doi: 10.1016/j.compag.2017.09.037
Karydas C., Chatziantoniou M., Tremma O., Milios A., Stamkopoulos K., Vassiliadis V., et al. (2023). Profitability assessment of precision agriculture applications—A step forward in farm management. Appl. Sci. (Switzerland) 13, 9640. doi: 10.3390/app13179640
Khan T., Qiu J., Banjar A., Alharbey R., Alzahrani A. O., Mehmood R. (2021). Effect of climate change on fruit by co-integration and machine learning. Int. J. Climate Change Strat. Manage. 13, 208–226. doi: 10.1108/IJCCSM-09-2020-0097
Khan T., Qiu J., Qureshi M. A. A., Iqbal M. S., Mehmood R., Hussain W. (2020). Agricultural fruit prediction using deep neural networks. Proc. Comput. Sci. 174, 72–78. doi: 10.1016/j.procs.2020.06.058
Kiet T. H. V. T., Hau T. V., KimThoa N. T., Nguyen P. T. (2020). Profit efficiency of mango growers in the Mekong delta, Vietnam. Solid State Technol. 63, 367–377.
Klarin A. (2024). How to conduct a bibliometric content analysis: Guidelines and contributions of content co-occurrence or co-word literature reviews. Int. J. Consumer Stud. 48, e13031. doi: 10.1111/ijcs.13031
Kleemann L., Effenberger A. (2010). Price transmission in the pineapple market: What role for organic fruit? pp. 1–25.
Koul B., Taak P. (2017). “Lychee (Litchi chinensis Sonn.): Pre- and Post-harvest Disease Management,” in Lychee Disease Management (Springer Singapore, Singapore), 1–26. doi: 10.1007/978-981-10-4247-81
Kraus M., Feuerriegel S., Oztekin A. (2020). Deep learning in business analytics and operations research: Models, applications and managerial implications. Eur. J. Operational Res. 281, 628–641. doi: 10.1016/j.ejor.2019.09.018
Kumari P., Goswami V., Harshith N., Pundir R. S. (2023). Recurrent neural network architecture for forecasting banana prices in Gujarat, India. PloS One 18, 1–17. doi: 10.1371/journal.pone.0275702
Leitner D., Klepsch S., Knieß A., Schnepf A. (2010). The algorithmic beauty of plant roots – an L-System model for dynamic root growth simulation. Math. Comput. Model. Dynam. Syst. 16, 575–587. doi: 10.1080/13873954.2010.491360
Licardo J. T., Domjan M., Orehovački T. (2024). Intelligent robotics—A systematic review of emerging technologies and trends. Electronics 13, 542. doi: 10.3390/electronics13030542
Lizarraga Hernández R. R. (2020). Relationship between farm size and technical efficiency: An empirical analysis of the oil palm sector in Colombia, 1–55.
Lozano S., Calzada-Infante L., Adenso-Díaz B., García S. (2019). Complex network analysis of keywords co-occurrence in the recent efficiency analysis literature. Scientometrics 120, 609–629. doi: 10.1007/s11192-019-03132-w
Luis-Rojas S., Ramírez-Valverde B., Díaz-Bautista M., Pizano-Calderón J., Rodríguez-López C. (2020). Vanilla (vanilla planifolia) production in Mexico: analysis and forecast. Rev. Mexicana Cienc. Agrícolas 11, 175–187. doi: 10.29312/remexca.v11i1.2065
Lynch J. P., Nielsen K. L., Davis R. D., Jablokow A. G. (1997). enSimRoot: Modelling and visualization of root systems. Plant Soil 188, 139–151. doi: 10.1023/A:1004276724310
Macharia C. W., Kiage L. M. (2024). Conceptualizing heat vulnerability: equity-centered approaches for comprehensive resilience in a changing climate. Natural Hazards 120, 6923–6941. doi: 10.1007/s11069-024-06440-4
Magalhães V. S., Ferreira L. M. D., Silva C. (2021). Using a methodological approach to model causes of food loss and waste in fruit and vegetable supply chains. J. clean. product. 283, 124574. doi: 10.1016/j.jclepro.2020.124574
Malhotra S. (2017). Horticultural crops and climate change: A review. Indian J. Agric. Sci. 87, 12–22. doi: 10.56093/ijas.v87i1.67138
Mallick J., Ahmed M., Alqadhi S. D., Falqi I. I., Parayangat M., Singh C. K., et al. (2022). Spatial stochastic model for predicting soil organic matter using remote sensing data. Geocarto Int. 37, 413–444. doi: 10.1080/10106049.2020.1720314
Mamoudan M. M., Jafari A., Mohammadnazari Z., Nasiri M. M., Yazdani M. (2023). Hybrid machine learning-metaheuristic model for sustainable agri-food production and supply chain planning under water scarcity. Res. Environ. Sustainabil. 14, 100133. doi: 10.1016/j.resenv.2023.100133
Martínez-Ruiz A., Ruiz-García A., Prado-Hernández J. V., López-Cruz I. L., Valencia-Islas J. O., Pineda-Pineda J. (2021). Global sensitivity analysis and calibration by differential evolution algorithm of hortsyst crop model for fertigation management. Water 13, 610. doi: 10.3390/w13050610
Mathiazhagan M., Chidambara B., Hunashikatti L. R., Ravishankar K. V. (2021). Genomic approaches for improvement of tropical fruits: fruit quality, shelf life and nutrient content. Genes 12, 1881. doi: 10.3390/genes12121881
Md Nor S., Ding P. (2020). Trends and advances in edible biopolymer coating for tropical fruit: A review. Food Res. Int. 134, 109208. doi: 10.1016/j.foodres.2020.109208
Melo-Becerra L. A., Orozco-Gallo A. J. (2017). Technical efficiency for Colombian small crop and livestock farmers: A stochastic metafrontier approach for different production systems. J. Product. Anal. 47, 1–16. doi: 10.1007/s11123-016-0487-x
Merle I., Hipólito J., Requier F. (2022). Towards integrated pest and pollinator management in tropical crops. Curr. Opin. Insect Sci. 50, 100866. doi: 10.1016/j.cois.2021.12.006
Meunier F., Couvreur V., Draye X., Lobet G., Huber K., Schroeder N., et al. (2022). “enInvestigating Soil–Root Interactions with the Numerical Model R-SWMS,” in Plant Systems Biology: Methods and Protocols. Ed. Lucas M. (Springer, New York, NY), 259–283. doi: 10.1007/978-1-0716-1816-513
Miranda J. C., Gené-Mola J., Zude-Sasse M., Tsoulias N., Escolà A., Arnó J., et al. (2023). Fruit sizing using ai: A review of methods and challenges. Postharvest Biol. Technol. 206, 112587. doi: 10.1016/j.postharvbio.2023.112587
Mite-Baidal K., Delgado-Vera C., Aguirre-Munizaga M., Calle-Romero K. (2019). “Prototype of an Embedded System for Irrigation and Fertilization in Greenhouses,” in Communications in Computer and Information Science. Eds. Valencia-García R., Alcaraz-Mármol G., Cioppo-Morstadt J.D., Lucio N.V.-., Bucaram-Leverone M. (Springer International Publishing, Cham), 30–40. doi: 10.1007/978-3-030-34989-9_3
Mohandass D., Campbell M. J., Chen X.-S., Li Q.-J. (2018). Flowering and fruiting phenology of woody trees in the tropical-seasonal rainforest, southwestern China. Curr. Sci. 114, 2313–2322. doi: 10.18520/cs/v114/i11/2313-2322
Mokria M., Gebrekirstos A., Said H., Hadgu K., Hagazi N., Dubale W., et al. (2022). Volume estimation models for avocado fruit. PloS One 17, e0263564. doi: 10.1371/journal.pone.0263564
Morris M., Sebastian A. R., Perego V. M. E. (2020). Future foodscapes re-imagining agriculture in Latin America and the Caribbean (Washington DC: International Bank for Reconstruction and Development/The World Bank).
Muhamad M. Z., Kamarulzaman N. H., Nawi N. M., Shamsudin M. N., Laham J. (2023). Assessing technical efficiency in Malaysian pineapple farms: A stochastic frontier analysis approach. Emirates J. Food Agric. 35, 1–9. doi: 10.9755/ejfa.2023.3201
Muhammed A. P., Shemeera S. C. J., Muralidharan A. P., Viswam V. K. (2020). AGRIO APP: an advanced android application for farmers. Int. J. Creative Res. Thoughts 8, 2320–28820.
Mukhametzyanov R. R., Ostapchuk T. V., Dzhancharov T. M., Ivantsova N. N., Vasileva E. N. (2023). “Changes in global production and trade of major tropical fruits,” in Digital agriculture for food security and sustainable development of the agro-industrial complex (Cham, Switzerland: Springer), 147–153.
Mwinuka P. R., Mourice S. K., Mbungu W. B., Mbilinyi B. P., Tumbo S. D., Schmitter P. (2022). Uav-based multispectral vegetation indices for assessing the interactive effects of water and nitrogen in irrigated horticultural crops production under tropical sub-humid conditions: A case of African eggplant. Agric. Water Manage. 266, 107516. doi: 10.1016/j.agwat.2022.107516
Nafees M. (2019). enTree modeling in horticultural crops: a review. J. Global Innov. Agric. Soc. Sci. 7, 87–97. doi: 10.22194/JGIASS/7.870
Nath V., Kumar G., Pandey S., Pandey S. (2019). Impact of climate change on tropical fruit production systems and its mitigation strategies. Climate Change Agric. India: Impact adapt., 129–146. doi: 10.1007/978-3-319-90086-5_11
Naudts K., Ryder J., McGrath M. J., Otto J., Chen Y., Valade A., et al. (2015). A vertically discretised canopy description for orchidee (svn r2290) and the modifications to the energy, water and carbon fluxes. Geosci. Model. Dev. 8, 2035–2065. doi: 10.5194/gmd-8-2035-2015
Nguyen T.-D., Venkatadri U., Nguyen-Quang T., Diallo C., Pham D.-H., Phan H.-T., et al. (2024). Stochastic modelling frameworks for dragon fruit supply chains in Vietnam under uncertain factors. Sustainability 16, 2423. doi: 10.3390/su16062423
Novo A. M., Slingerland M., Jansen K., Kanellopoulos A., Giller K. E. (2013). Feasibility and competitiveness of intensive smallholder dairy farming in Brazil in comparison with soya and sugarcane: Case study of the balde cheio programme. Agric. Syst. 121, 63–72. doi: 10.1016/j.agsy.2013.06.007
OECD. (2023). Environment at a glance in Latin America and the Caribbean: Spotlight on climate change (Paris, France: OECD). doi: 10.1787/2431bd6c-en
Orlandini S., Magarey R. D., Park E. W., Sporleder M., Kroschel J. (2020). Methods of agroclimatology: modeling approaches for pests and diseases. Agroclimatol.: linking Agric. to Climate 60, 453–488. doi: 10.2134/agronmonogr60.2016.0027
Orozco-Abarca J. S. (2007). Factors affecting the supply and demand for limes and lime oil in the U.S.: Development implications for Veracruz State, Mexico., 1–56.
Pacheco-Sánchez E. L., Guamani-Quimis L. A., da Rosa C. E., Portalanza D., Mieles A. E., Garcés-Fiallos F. R. (2023). Forecasting occurrence of palm weevil rhynchophorus palmarum l. (coleoptera, curculionidae) using autoregressive integrated moving average modeling. Scientia Agropecuaria 14, 171–178. doi: 10.17268/sci.agropecu.2023.015
Pagès L., Vercambre G., Drouet J.-L., Lecompte F., Collet C., Le Bot J. (2004). enRoot Typ: a generic model to depict and analyse the root system architecture. Plant Soil 258, 103–119. doi: 10.1023/B:PLSO.0000016540.47134.03
Pandey H., Singh D., Pandey A. K., Suthar K. P., Mehta R., Pandey D. (2021). “Current approaches in horticultural crops to mitigate the effect of cold stress,” in Stress Tolerance in Horticultural Crops (Abingdon, Cambridge, United Kingdom: Woodhead Publishing), 241–257. doi: 10.1016/B978-0-12-822849-4.00010-3
Paniagua-Molina J., Solís-Rivera L. R. (2020). Effect of “golden pineapple innovation” on Costa Rica’s pineapple exports to u.s. market: An econometric approach. Int. J. Food Agric. Econ. 8, 219–231. doi: 10.22004/ag.econ.305325
Paniagua-Molina J., Solórzano-Thompson J. (2020). Improving strategic management through risk analysis: Small palm (elaeis guineensis) oil industrializers, Central America. Agro. Mesoamericana 31, 619–633. doi: 10.15517/AM.V31I3.40349
Parra-Coronado A., Fischer G., Camacho-Tamayo J. H. (2016). Growth model of the pineapple guava fruit as a function of thermal time and altitude. Ingeniería e Investigación 36, 6–14. doi: 10.15446/ing.investig.v36n3.52336
Pascoe S., Kirkley J., D. G., Morrison-Paul C. (2003). Stochastic production frontiers (Rome, Italy: FAO).
Patrick S., Mirau S., Mbalawata I., Leo J. (2023). Time series and ensemble models to forecast banana crop yield in Tanzania, considering the effects of climate change. Res. Environ. Sustainabil. 14, 100138. doi: 10.1016/j.resenv.2023.100138
Pokhrel D. R., Sirisomboon P., Khurnpoon L., Posom J., Saechua W. (2023). Comparing machine learning and plsda algorithms for durian pulp classification using inline nir spectra. Sensors. 23, 5327. doi: 10.3390/s23115327
Qiu G., Lu H., Wang X., Wang C., Xu S., Liang X., et al. (2023). Nondestructive detecting maturity of pineapples based on visible and near-infrared transmittance spectroscopy coupled with machine learning methodologies. Horticulturae 9, 889. doi: 10.3390/horticulturae9080889
Ramirez-Guerrero T., Hernandez-Perez M. I., Tabares M. S., Marulanda-Tobon A., Villanueva E., Peña A. (2023). Agroclimatic and phytosanitary events and emerging technologies for their identification in avocado crops: A systematic literature review. Agronomy. 13, 1976. doi: 10.3390/agronomy13081976
Rathod S., Mishra G. C. (2018). Statistical models for forecasting mango and banana yield of Karnataka, India. J. Agr. Sci. Tech. 20, 803–816.
Rauber P. E., Fadel S. G., Falcão A. X., Telea A. C. (2017). Visualizing the hidden activity of artificial neural networks. IEEE Trans. Visualization Comput. Graphics 23, 101–110. doi: 10.1109/TVCG.2016.2598838
Rauch E., Tippayawong N., Tippayawong K. Y. (2023). Forecasting arabica coffee yields by auto-regressive integrated moving average and machine learning approaches. AIMS Agric. Food. 8, 1052–1070. doi: 10.3934/agrfood.2023057
Rickard B., Pierre C. (2008). “Examining trends and drivers of production and import demand for selected agricultural commodities,” in International Food and Agricultural Trade Policy Council and the session titled Changes in the structure of world agricultural production and demand, Barcelona.
Ritchie J. T. (1998). Soil water balance and plant water stress. Understand. options Agric. product. 7, 41–54.
Robson A., Rahman M. M., Muir J. (2017). Using worldview satellite imagery to map yield in avocado (Persea Americana): A case study in Bundaberg, Australia. Remote Sens. 9, 1223. doi: 10.3390/rs9121223
Roco L., Bravo-Ureta B., Engler A., Jara-Rojas R. (2017). The impact of climatic change adaptation on agricultural productivity in Central Chile: A stochastic production frontier approach. Sustainabil. (Switzerland) 9, 1–16. doi: 10.3390/su9091648
Romero A., Camargo A., Ramirez O., Arenas P., Gallego A. (2022). A crop modelling strategy to improve cacao quality and productivity. Plants 11, 1–16. doi: 10.3390/plants11020157
Ruiz-Real J. L., Uribe-Toril J., Torres Arriaza J. A., de Pablo Valenciano J. (2020). A look at the past, present and future research trends of artificial intelligence in agriculture. Agronomy 10, 1839. doi: 10.3390/agronomy10111839
Sahu P., Singh A. P., Chug A., Singh D. (2022). A systematic literature review of machine learning techniques deployed in agriculture: A case study of banana crop. IEEE Access 10, 87333–87360. doi: 10.1109/ACCESS.2022.3199926
Saiz-Rubio V., Rovira-Más F. (2020). From smart farming towards agriculture 5.0: A review on crop data management. Agronomy. 10, 1–21. doi: 10.3390/agronomy10020207
Salari A., Shakibi H., Soleimanzade M. A., Sadrzadeh M., Hakkaki-Fard A. (2023). Application of machine learning in evaluating and optimizing the hydrogen production performance of a solar-based electrolyzer system. Renewable Energy 220, 119626. doi: 10.1016/j.renene.2023.119626
Salinas I., Hueso J. J., Cuevas J. (2019). Fruit growth model, thermal requirements and fruit size determinants in papaya cultivars grown under subtropical conditions. Scientia Hortic. 246, 1022–1027. doi: 10.1016/j.scienta.2018.11.056
Salliou N., Vialatte A., Monteil C., Barnaud C. (2019). First use of participatory bayesian modeling to study habitat management at multiple scales for biological pest control. Agron. Sustain. Dev. 39, 7. doi: 10.1007/s13593-018-0553-z
Sattar M. N., Iqbal Z., Al-Khayri J. M., Jain S. M. (2021). Induced genetic variations in fruit trees using new breeding tools: Food security and climate resilience. Plants 10, 1347. doi: 10.3390/plants10071347
Selvaraj M. G., Vergara A., Ruiz H., Safari N., Elayabalan S., Ocimati W., et al. (2019). Ai-powered banana diseases and pest detection. Plant Methods 15, 1–11. doi: 10.1186/s13007-019-0475-z
Seyum E. G., Bille N. H., Abtew W. G., Munyengwa N., Bell J. M., Cros D. (2022). Genomic selection in tropical perennial crops and plantation trees: a review. Mol. Breed. 42, 58. doi: 10.1007/s11032-022-01326-4
Shahhosseini M., Martinez-Feria R. A., Hu G., Archontoulis S. V. (2019). Maize yield and nitrate loss prediction with machine learning algorithms. Environ. Res. Lett. 14. doi: 10.1088/1748-9326/ab5268
Shamshiri R. R., Sturm B., Weltzien C., Fulton J., Khosla R., Schirrmann M., et al (2024). Digitalization of agriculture for sustainable crop production: a use-case review. Front. Environ. Sci. 12. doi: 10.3389/fenvs.2024.1375193
Singh N., Gupta N. (2017). Decision-making in integrated pest management and bayesian network. Int. J. Comput. Sci. Inf. Technol. 9, 31–37. doi: 10.5121/ijcsit.2017.9203
Snyder R. (2017). Climate change impacts on water use in horticulture. Horticulturae 3, 27. doi: 10.3390/horticulturae3020027
Song L., Jin J. (2020). Improving ceres-maize for simulating maize growth and yield under water stress conditions. Eur. J. Agron. 117, 126072. doi: 10.1016/j.eja.2020.126072
Srivastava A., Wu Q.-S., Mousavi S. M., Hota D. (2021). Integrated soil fertility management in fruit crops: An overview. Int. J. Fruit Sci. 21, 413–439. doi: 10.1080/15538362.2021.1895034
Steduto P., Hsiao T. C., Raes D., Fereres E. (2009). Aquacrop—the fao crop model to simulate yield response to water: I. concepts and underlying principles. Agron. J. 101, 426–437. doi: 10.2134/agronj2008.0139s
Stewart A. L., Ahmed S. (2020). “Chapter 7 - effects of climate change on fruit nutrition,” in Fruit Crops. Eds. Srivastava A., Hu C. (Amsterdam, The Netherlands: Elsevier), 77–93. doi: 10.1016/B978-0-12-818732-6.00007-1
Stöckle C. O., Donatelli M., Nelson R. (2003). Cropsyst, a cropping systems simulation model. Eur. J. Agron. 18, 289–307. doi: 10.1016/S1161-0301(02)00109-0
Sun G., Hu T., Liu X., Peng Y., Leng X., Li Y., et al. (2022). Optimizing irrigation and fertilization at various growth stages to improve mango yield, fruit quality and water-fertilizer use efficiency in xerothermic regions. Agric. Water Manage. 260, 107296. doi: 10.1016/j.agwat.2021.107296
Supit I. (1994). System description of the WOFOST 6.0 crop simulation model implemented in CGMS. Theory algorithms 1, 146.
Sylla M. B., Nikiema P. M., Gibba P., Kebe I., Klutse N. A. B. (2016). Climate change over West Africa: Recent trends and future projections. Adapt. to Climate Change variability Rural West Afr., 25–40. doi: 10.1007/978-3-319-31499-0_3
Tanimoto Y., Yoshida S. (2024). A method of constructing models for estimating proportions of citrus fruit size grade using polynomial regression. Agronomy 14, 174. doi: 10.3390/agronomy14010174
Tisné S., Denis M., Domonhédo H., Pallas B., Cazemajor M., Tranbarger T. J., et al. (2020). Environmental and trophic determinism of fruit abscission and outlook with climate change in tropical regions. Plant-Environ. Interact. 1, 17–28. doi: 10.1002/pei3.10011
Tong X., Wu P., Liu X., Zhang L., Zhou W., Wang Z. (2022). A global meta-analysis of fruit tree yield and water use efficiency under deficit irrigation. Agric. Water Manage. 260, 107321. doi: 10.1016/j.agwat.2021.107321
Tosto A., Morales A., Rahn E., Evers J. B., Zuidema P. A., Anten N. P. (2023). Simulating cocoa production: A review of modelling approaches and gaps. Agric. Syst. 206, 103614. doi: 10.1016/j.agsy.2023.103614
Trieu N. M., Thinh N. T. (2023). Using random forest algorithm to grading mango’s quality based on external features extracted from captured images. J. Image Graphics(United Kingdom) 11, 391–396. doi: 10.18178/joig.11.4.391-396
Trujillo J. C., Iglesias W. J. (2013). Measurement of the technical efficiency of small pineapple farmers in santander, Colombia: A stochastic frontier approach. Rev. Economia e Sociol. Rural 51, S049–S062. doi: 10.1590/S0103-20032013000600003
Valdez-Rivera D., Garcia-Ortega Y., Ulloa-Bucaram D., Hammer-Juan J. (2022). enTechniques to advance flowering in export mangoes. Centrosur Agraria 1, 99–111.
Valencia K., Duana D. (2019). Los cítricos en méxico: análisis de eficiencia técnica. Análisis Económico XXXIV, 269–283.
Van Laar H. H., Goudriaan J., Van Keulen H. (1992). Simulation of crop growth for potential and water-limited production situations: as applied to spring wheat Vol. 27 (Wageningen, The Netherlands: CABO-DLO).
van Meijl H., Shutes L., Valin H., Stehfest E., van Dijk M., Kuiper M., et al. (2020). Modelling alternative futures of global food security: Insights from foodsecure. Global Food Secur. 25, 100358. doi: 10.1016/j.gfs.2020.100358
Varela D. M. (2023). Efectos de variables climáticas sobre la eficiencia agrícola: El caso de la producción del cacao fino de aroma en la provincia de manabí-Ecuador en el período 2016-2019, 1–30.
Varma T., Mate P., Azeem N. A., Sharma S., Singh B. (2024). Automatic mango leaf disease detection using different transfer learning models. Multimed. Tools Appl. 67. doi: 10.1007/s11042-024-19265-x
Vieira M. S., Cabral R. L. R., Favaratto L., Maciel L. S., Xavier A., d. S., et al. (2024). Tropical fruit virus resistance in the era of next-generation plant breeding. SynBio 2, 267–284. doi: 10.3390/synbio2030016
Villachica H., Silva J. E., Peres J. R., da Rocha C. M. C. (2020). “Sustainable agricultural systems in the humid tropics of South America,” in Sustainable agricultural systems (Ankeny, Iowa: CRC Press), 391–437.
Villa-Henriksen A., Edwards G. T., Pesonen L. A., Green O., Sørensen C. A. G. (2020). Internet of things in arable farming: Implementation, applications, challenges and potential. Biosyst. Eng. 191, 60–84. doi: 10.1016/j.biosystemseng.2019.12.013
Wang M., White N., Grimm V., Hofman H., Doley D., Thorp G., et al. (2018). Pattern-oriented modelling as a novel way to verify and validate functional–structural plant models: a demonstration with the annual growth module of avocado. Ann. Bot. 121, 941–959. doi: 10.1093/aob/mcx187
Wang J., Xie Z., Mao P., Sun M., Guo J. (2024). Fruit modeling and application based on 3d imaging technology: a review. J. Food Measure. Character. 18, 1–17. doi: 10.1007/s11694-024-02480-3
Wheeler R., Lobley M. (2021). Managing extreme weather and climate change in uk agriculture: Impacts, attitudes and action among farmers and stakeholders. Climate Risk Manage. 32, 100313. doi: 10.1016/j.crm.2021.100313
Wolfert S., Ge L., Verdouw C., Bogaardt M. J. (2017). Big data in smart farming – a review. Agricultural Systems. 153, 69–80. doi: 10.1016/j.agsy.2017.01.023
Wu L., McGechan M. B., McRoberts N., Baddeley J. A., Watson C. A. (2007). SPACSYS: Integration of a 3D root architecture component to carbon, nitrogen and water cycling—Model description. Ecol. Model. 200, 343–359. doi: 10.1016/j.ecolmodel.2006.08.010
Yaseen Z. M. (2023). Machine learning models development for shear strength prediction of reinforced concrete beam: a comparative study. Sci. Rep. 13, 1723. doi: 10.1038/s41598-023-27613-4
Zhang Y., Hughes A. C., Zhao Z., Li Z., Qin Y. (2022). Including climate change to predict the global suitable area of an invasive pest: Bactrocera correcta (diptera: Tephritidae). Global Ecol. Conserv. 34, e02021. doi: 10.1016/j.gecco.2022.e02021
Zscheischler J., Martius O., Westra S., Bevacqua E., Raymond C., Horton R. M., et al. (2020). A typology of compound weather and climate events. Nat. Rev. Earth Environ. 1, 333–347. doi: 10.1038/s43017-020-0060-z
Keywords: fruit modeling, crop modeling, agricultural data, tropical climate, tropical agriculture
Citation: Mancero-Castillo D, Garcia Y, Aguirre-Munizaga M, Ponce de Leon D, Portalanza D and Avila-Santamaria J (2024) Dynamic perspectives into tropical fruit production: a review of modeling techniques. Front. Agron. 6:1482893. doi: 10.3389/fagro.2024.1482893
Received: 19 August 2024; Accepted: 26 November 2024;
Published: 20 December 2024.
Edited by:
Pratap Bhattacharyya, ICAR-NRRI, IndiaReviewed by:
Saadat Sarikhani, University of Tehran, IranMuhammad Ather Nadeem, University of Sargodha, Pakistan
Copyright © 2024 Mancero-Castillo, Garcia, Aguirre-Munizaga, Ponce de Leon, Portalanza and Avila-Santamaria. This is an open-access article distributed under the terms of the Creative Commons Attribution License (CC BY). The use, distribution or reproduction in other forums is permitted, provided the original author(s) and the copyright owner(s) are credited and that the original publication in this journal is cited, in accordance with accepted academic practice. No use, distribution or reproduction is permitted which does not comply with these terms.
*Correspondence: Daniel Mancero-Castillo, danielmanceroc@gmail.com