- 1UFR des Sciences Sociales, Université Peleforo Gon Coulibaly (UPGC), Korhogo, Côte d'Ivoire
- 2Regional Center of Excellence for Transboundary Plant Pathogens, Central and West African Virus Epidemiology (WAVE), Pôle Scientifique et d’innovation, Université Félix Houphouët-Boigny (UFHB), Abidjan, Côte d'Ivoire
- 3Centre Ivoirien des Recherches Economiques et Sociales (CIRES), Abidjan, Côte d'Ivoire
- 4Institut de l’Environnement et de Recherches Agricoles (INERA), Ouagadougou, Burkina Faso
- 5UFR Biosciences, Université Félix Houphouët-Boigny (UFHB), Abidjan, Côte d'Ivoire
In Côte d’Ivoire, cassava is the source of calories for about 26 million people and generates significant income for stakeholders in the value chain. However, its production is threatened by Cassava Mosaic Disease (CMD), which causes yield losses of up to 70%. This disease remains poorly known among farmers, who often adopt poor cultivation practices that serve to amplify its spread. To address this issue, the Regional Center of Excellence for transboundary plant pathogens Central and West African Virus Epidemiology (WAVE) introduced an innovate android based plant disease detection smart application called “PlantVillage Nuru” to help farmers recognize the disease through real-time diagnosis in cassava fields. The present study analyzes the perceptions and adoption rate of this application by farmers in the diagnosis of CMD within three departments of Côte d’Ivoire. Quantitative and qualitative data were collected from 152 farmers and agricultural technicians, using a questionnaire and interview guide in Dabou, Bouaké and Man. Data obtained were analyzed through descriptive statistics on SPSS and content analysis. A binary logit regression model was then used to identify the determinants of Nuru application adoption with Stata version 12.1. The study revealed an adoption rate of 45%, focused mainly on monitoring the phytosanitary status of cassava fields (98%), selecting healthy cuttings as planting materials (52%), and accessing agricultural advice (21%). The use of the Nuru application was strongly influenced (P<0.001) by the size of cassava field owned, respondents’ participation in WAVE cassava disease awareness activities, the use of family labor, and whether the respondents own or received a smartphone from WAVE. Gender, level of education, the contact with agricultural extension service and internet access, were also significant determinants (P<0.01) in the use of the application. Despite the constraints of smartphone unavailability (65%) and the complexity of using the application (41%) observed, Nuru was considered by farmers (100%) to be an essential and reliable training tool for detecting and managing CMD but did not appear to be needed on a sustained and regular basis. These findings suggest the need for categorization, follow-up, and sustained support to farmers to improve the adoption of agricultural technologies.
1 Introduction
Cassava (Manihot esculenta Crantz) is the main source of food for around 500 million people in Africa (Vernier et al., 2018). Côte d’Ivoire, with an annual production of 6.9 million tons, is the third-largest producer of cassava in the West African sub-region, following Nigeria and Ghana (FAOSTAT, 2021). Cassava is the second most important food crop produced and consumed in Côte d’Ivoire after yams, providing the main source of calories for almost 26 million people (FAOSTAT, 2020). In the Ivorian context, cassava is an essential food security commodity, providing jobs and significant income for all stakeholders in the value chain (Mendez del Villar et al., 2017).
However, the yield remains low compared to Ghana where yields are three times greater (24.4 tons/hectare) than in Côte d’Ivoire (5.6 tons/hectare) (FAOSTAT, 2021). Indeed, cassava cultivation in Côte d’Ivoire is subject to numerous biotic and abiotic constraints that affect productivity, including Cassava Mosaic Disease (CMD), which is one of the major threats (Brou et al., 2015). CMD is a viral disease transmitted by the whitefly (Bemisia tabaci) and is mainly spread through the use of infected cuttings (Njoroge et al., 2017; Soro et al., 2021). CMD which can cause significant yield losses ranging from 40% to 70%, does not only reduce farmers’ incomes (Patil and Fauquet, 2009), but also hinders their ability to meet the increasing demand for cassava and its derivative products (Soro et al., 2021). The use of healthy planting material and resistant varieties has emerged as an alternative that could help reduce the incidence of the disease in fields and its impact on yields and farmers’ incomes (Rabbi et al., 2014). Over the years, the International Institute of Tropical Agriculture (IITA) and the Centre National de Recherches en Agronomie (CNRA) have been disseminating improved cassava varieties like Bocou 5, Yavo, Olekanga, and TMS30572 to farmers (ANADER, 2017; Vernier et al., 2018) to mitigate the impact of CMD.
However, the lack of healthy planting material and poor farming practices have hampered this approach. Indeed, despite their high susceptibility to CMD, local cultivars remain popular among farmers due to their appreciated organoleptic properties (Kouassi et al., 2018). Moreover, farmers primarily reuse their old cuttings and/or those of neighboring farmers to establish new fields. And they do not engage in any sorting operations when selecting cuttings, as they lack the knowledge and expertise to distinguish between CMD-infected and healthy cuttings (Djaha et al., 2018). The incidence of CMD therefore remained preponderant in Côte d’Ivoire, with an average incidence of 49.21%1 according to data from surveys carried out by WAVE (https://wave-center.org/) in 2016, 2017 and 2020. Furthermore, Kouakou et al. (2024) have shown that the main mode of transmission of CMD in Côte d’Ivoire is through the use of infected cassava cuttings for establishing new farms.
Therefore, to reduce the burden of CMD in Côte d’Ivoire, an innovative approach is necessary for better management of the disease. This involves enhancing the ability of farmers to identify CMD, and to select and use symptom-free planting material. In this regard, WAVE has been disseminating a plant disease detection smart application, “PlantVillage Nuru” to farmers since 2020. Also known as Cassava Disease Detection Application (CDD-APP), this application is based on artificial intelligence and uses image recognition to identify different types of disease, including CMD. It enables farmers to detect the presence of diseases symptoms or pest on the crop, give them feed-back in real time, plays the role of teaching tool; by showing to farmers the symptoms of the disease it has recognized and provide advice on how to manage or control the disease or pest identified. Nuru is a public good resource built at Penn State University and is accessible for free and gives farmers a choice between 23 languages, including English, French and Swahili (Mbilinyi and Mrisho, 2019).
However, its use requires the farmers to register (Country, city, number, status in cassava cultivation, password) in order to access the Nuru digital platform and remain connected with other members of the community (agricultural experts, farmers, seed suppliers, etc.). The farmer is then presented with four main options. The first one (Proceed) enables him to analyze deeply the phytosanitary status of one plant. In this case, he is invited by the AI to expose six different leaves of the cassava plant (3 on the upper part and 3 on the lower part) to the smartphone’s camera, to obtain in vocal and written form, on the phone’s screen, the name of the disease concerned. The second option (scout) allows the farmer to inspect the field by diagnosing series of ten plants, before receiving the results of the analysis in written and vocal form too. In this instance, the application presents the infection rate of the ten diagnostic plants via an image on the screen, with infected plants displayed in black and uninfected plants in green. A third option (Real-time diagnosis) enables the farmer to present to the camera a cassava plant exhibiting anomalies to know its phytosanitary status on the smartphone screen. The last option (Disease comparison) enables farmers to capture and transfer an image of a plant exhibiting unusual symptoms to the platform’s agricultural experts. Following a comparison of the observed symptoms with those of other similar diseases, the farmer will receive a response indicating the condition of the plant in question. The plant diagnostics can be performed offline, and the images captured by the farmer are then systematically transferred to the PlanVillage database, for expert advice and suggestions, when he is again connected to the Internet. Apart from the last two options (Real-time diagnosis and Disease comparison) when the application is used in practice, the farmer must first enter information about the field (geographical position, date of cassava sowing) so that agricultural experts may be able to monitor the phytosanitary status of the field and provide the required advice in accordance with the field characteristics. Initial experience with this application showed that Nuru is a reliable tool for detecting CMD. It is able to recognize CMD symptoms as well as researchers trained in recognizing cassava pests and diseases. It is 1.5 times better than agricultural agents and twice as good as farmers (Mrisho et al., 2020).
As with PlantVillage, which hosts the Nuru application, other digital platforms also make use of AI for the purposes of crop monitoring and management. In this regard, Samuels (2024) demonstrated the effectiveness of platforms such as FarmLogs and Climate FieldView that enable farmers to analyze vegetation indices, detect anomalies and remotely monitor field conditions. Thanks, to satellite imagery, drones and data analysis, farmers could take timely action to reduce risks and optimize crop production. In addition to AI, remote sensing technologies are also employed in the field of plant disease management. Satellite images and drones help farmers understand their crops. They can see if pests are damaging crops, if crops are growing well, and if their farming practices are working. The detailed information captured through high-resolution imagery enables farmers to make data-driven decisions and implement targeted interventions with the objective of maximizing productivity (Raza et al., 2023; Samuels, 2024). Automation and robotics are also used in plant diseases management. The use of autonomous vehicles equipped with GPS and sensors by farmers enables the implementation of precise navigation, field mapping, and optimized crop and pest management practices. In this system, farmers are more able to achieve greater precision in pest management and to enhance productivity through the optimized utilization of resources. In a World Bank case study for instance, Maddison (2007) demonstrated that autonomous agricultural machines could that way increase the speed of agricultural plantations by 15%, as well as avoiding the various precautions farmers need to take against plant diseases. Others study also in the same way, proved that using computerized agriculture for irrigation and precise fertilizer application increased crop yields respectively by 20% and 10% (García-Vila and Fereres, 2012; Kaiser, 2023). Considering the literature, the use of digital technology in plant disease management allows for the following: improved resource efficiency, enhanced crop yields and quality, sustainable farming practices and economic benefits for farmers.
However, beyond the benefits mentioned in the literature above, the effective use of digital agricultural technologies requires specialized skills and knowledge from farmers and agricultural stakeholders. As part of the deployment of the Nuru application in Côte d’Ivoire, farmers in the departments of Dabou, Bouaké and Man were gathered in the main cassava-producing villages. They were given smartphones after receiving training on how to use the application. Beneficiary were then monitored and assisted by extension officers and the WAVE project team in the use of the application for the duration of the project. However, accessibility, ease of use, initial equipment costs and connectivity constraints can be major barriers, particularly for small-scale farmers in digital agriculture technology adoption (Aubert et al., 2012; Paustian and Theuvsen, 2017; Samuels, 2024).
Thus, three years after the diffusion of Nuru in Côte d’Ivoire as a pilot initiative, what is the extent of the adoption of this technology and how do farmers perceive its utilization in the identification and management of CMD within beneficiary farming communities? Specifically, the study aims to:
● Assess the acceptance and utilization level of Nuru by farmers.
● Identify the barriers to the widespread uptake of the application.
● Analyze the perceptions of farmers regarding the use of the Nuru application in identifying and managing CMD.
The different sections of our study will be articulated around the presentation of the methodology of the study, the main results, the discussion, a conclusion and recommendations.
2 Methodology of the study
2.1 Presentation of the study sites
The study was conducted in three departments of Côte d’Ivoire: Dabou in the south, Bouaké in the center, and Man in the west of the country. These departments were selected based on the importance of cassava cultivation, and the incidence of CMD. Data was collected in 18 villages across the three departments. Table 1 presents the villages surveyed by department. It is worth noting that these three departments selected for this study, benefited from the “the Fonds Compétitif pour l’Innovation Agricole Durable (FCIAD) project” entitled “Use of a smart application for participatory diagnosis and monitoring of cassava diseases in Cote d’Ivoire” implemented by WAVE between January 2020 and June 2021. The general aim of this project was “to help improve cassava productivity in Côte d’Ivoire, by monitoring and protecting the cassava crop against viral diseases”. The selected departments are also among the main cassava production areas in Côte d’Ivoire (Kouassi et al., 2018) and have a high incidence of CMD2 (Figure 1).
2.2 Population and sampling
The “PlantVillage Nuru” digital platform tracks and records its users. We used this platform to determine the sampling frame for this study. Indeed, based on the list of users (farmers), we used the simple random sampling technique to determine the number of participants in our study in each of the department. A total of 146 farmers were contacted, including 44 in Dabou, 46 in Bouaké and 56 in Man. The selection of farmers was based on their availability and willingness to participate in the study. Additionally, we interviewed three (3) extension officers, one (1) IT technician, and two (2) “FCIAD project” coordinators. This mixed method used for this study enabled a combined analysis of quantitative and qualitative data and allowed for a better understanding of farmers’ behavior regarding the adoption of Nuru.
2.3 Data collection and analysis
2.3.1 Data collection
Data collection was conducted in two steps. The first consisted of re-establishing contact with the beneficiaries of the “FCIAD project”, to inform them of this study and its objectives and to obtain their buy-in and their consent to participate. This step took place between June 15th to July 13th, 2023. The second step involved data collection in the selected areas which was conducted between the 1st and 26th of August 2023. During this step, we developed a digital questionnaire using the Kobocollect software to survey the farmers selected for the study. The questionnaire was structured around the following points: socio-demographic characteristics of the farmers and their household, agronomic parameters of cassava fields, interaction with agricultural services, experience with the Nuru application, determinants of its use, and level of appreciation of the application by farmers. Additionally, data on frequency of the use of Nuru by the respondents and the number of Nuru’s users over time were collected from the “PlantVillage Nuru” application’s digital platform database. Two focus groups discussions, consisting of 8 to 12 farmers each, were conducted in each department. Furthermore, individual interviews were conducted with three (3) agricultural supervisors responsible for monitoring beneficiary farmers, two (2) “FCIAD project” coordinators, and one (1) WAVE IT technician. These interviews provided insight into farmers’ perceptions of the application, the conditions and constraints associated with its use, and the various forms of assistance received by users.
2.3.2 Data analysis
The quantitative data were analyzed using descriptive statistics, including frequency, mean, and other measures. To identify the determinants of Nuru application use, we used the binary logit regression model. This is a model that estimates the strength of association between a dichotomous qualitative dependent variable and both qualitative and quantitative explanatory variables (Gillet et al., 2011). In this study, we chose this model because our dependent variable, the use of the Nuru application, is qualitative and dichotomous. It can therefore take the form yes (1) when the farmer uses the application or no (0) when the farmer does not. The logit model can be expressed in linear form as follows: Y is a binary variable (yes/no) and X is an independent variable that explains Y. Y can take the value 1 with probability P (Y=1/X) and the value 0 with probability (1−P (Y=1/X)). The model is expressed as:
Given that π (X) represents a probability, it must lie within the interval [0,1]. The logit function is defined as follows: .
If we apply the logit function to π (X), the expression becomes: .
The range of variation of G (π (X)) is between −∞ and +∞, while π(X) varies between 0 and 1. Consequently, regression can be implemented. The parameters β0 and β1 are estimated using the maximum likelihood method. Given that the estimation of our logit model is based on the maximum likelihood method, let be the probability that the logit associates with the survey unit:
In this context, represents a vector that encompasses the characteristics of the survey unit, its environment, and the object of its choice. represents the coefficients of the explanatory variables, while represents the explanatory variables, and represents the error term. The independent variables were identified through an analysis of empirical literature and available data. The following variables were identified: farmer characteristics (gender, marital status, education level, age, main activity, smartphone ownership, internet access) (Chirwa, 2005; Alene and Manyong, 2006; Derra, 2014); field characteristics (farm type, labor type, distance from home to field, cultivated area) (Hailu et al., 2014); and interactions with an agricultural service (contact with a supervisory service, membership of a cassava association, participation in cassava disease awareness campaigns, plot visit for application testing, type of trainer, receipt of a smartphone from the project team) (Lambrecht et al., 2014). Data were then analyzed using SPSS and Stata version 12.1 software.
Qualitative data was processed using content analysis, as recommended by Combessie (2007). The study results were interpreted based on Rogers’ (1995) theory of the diffusion of innovation. This theory provided a framework for analyzing the relationship between the type, cost, and accessibility of the smartphone required to use the “PlantVillage Nuru” application, the conditions of use, and the actual use or non-use of this technology by farmers.
3 Results
3.1 Socio-demographic characteristics of farmers
The data collected showed that out of the 146 farmers surveyed, 30% were from Dabou, 32% from Bouaké and 38% from Man. The respondents were mostly men (59%), and most were between 41 and 64 years old (61%). Additionally, 73% of the farmers interviewed had received formal education at various levels, including primary, secondary, and higher education. However, secondary education was the most common level of education among the respondents (40%). Most farmers reported being in a relationship (65%), with cohabitation being their most common living arrangement (49%) compared to those who were married (16%). Moreover, 67% of those surveyed were heads of households, with farming being their primary occupation (80%). The respondents had an average of over 11 years of experience in agriculture in general (74%) and in cassava growing (57%) in particular. Out of all farmers surveyed, 40% had a smartphone and the majority (60%) had access to the Internet. Table 2 describes the characteristics of the groups selected for this study.
3.2 Characteristics of farmers’ fields
To establish a correlation between farmers’ fields, their interactions with agricultural structures, and the use of the application, we considered additional parameters. Our observations revealed that cassava was typically grown on small superficies of 0.94 ha in average across all three departments surveyed. These fields were generally personal (69%) and mainly worked by hired labor (86%). Farmers had to travel an average distance of 3.12 km to access these plots, whose production represented on average 55.57% of the overall farm income (Table 3). Regarding farmers’ interactions with agricultural structures, data analysis revealed that 55% of farmers who benefited from the “PlantVillage Nuru” application were not affiliated with any agricultural organization involved in cassava-related activities. However, 65% reported being in contact with an agricultural service related to cassava cultivation and had participated in WAVE’s main training activities. These activities involved awareness-raising, targeted training, and plot visits. Targeted training had a higher number of participants (87%). Farmers received training on how to use the application either directly from the WAVE team or indirectly from an extension officer, or fellow farmer previously trained by the WAVE team. Among the users surveyed, 77% had not received a smartphone during the training sessions (Table 4).
3.3 Acceptance and level of use of the application by farmers
Data collected from the “PlantVillage Nuru” platform shows that farmers have used the application at least once in cassava fields since its installation. However, only 45% of beneficiaries across the three departments reported continuing to use the application to diagnose CMD in cassava fields, after the “FCIAD project” has ended, while 56% stated that they had stopped using it. The analysis also revealed a significant difference in the use of the application between departments (P<0.01). The application was more widely used in Dabou (61%) and Bouaké (54%), while its use remained low in the Man area (23%), as shown in Figures 2 and 3.
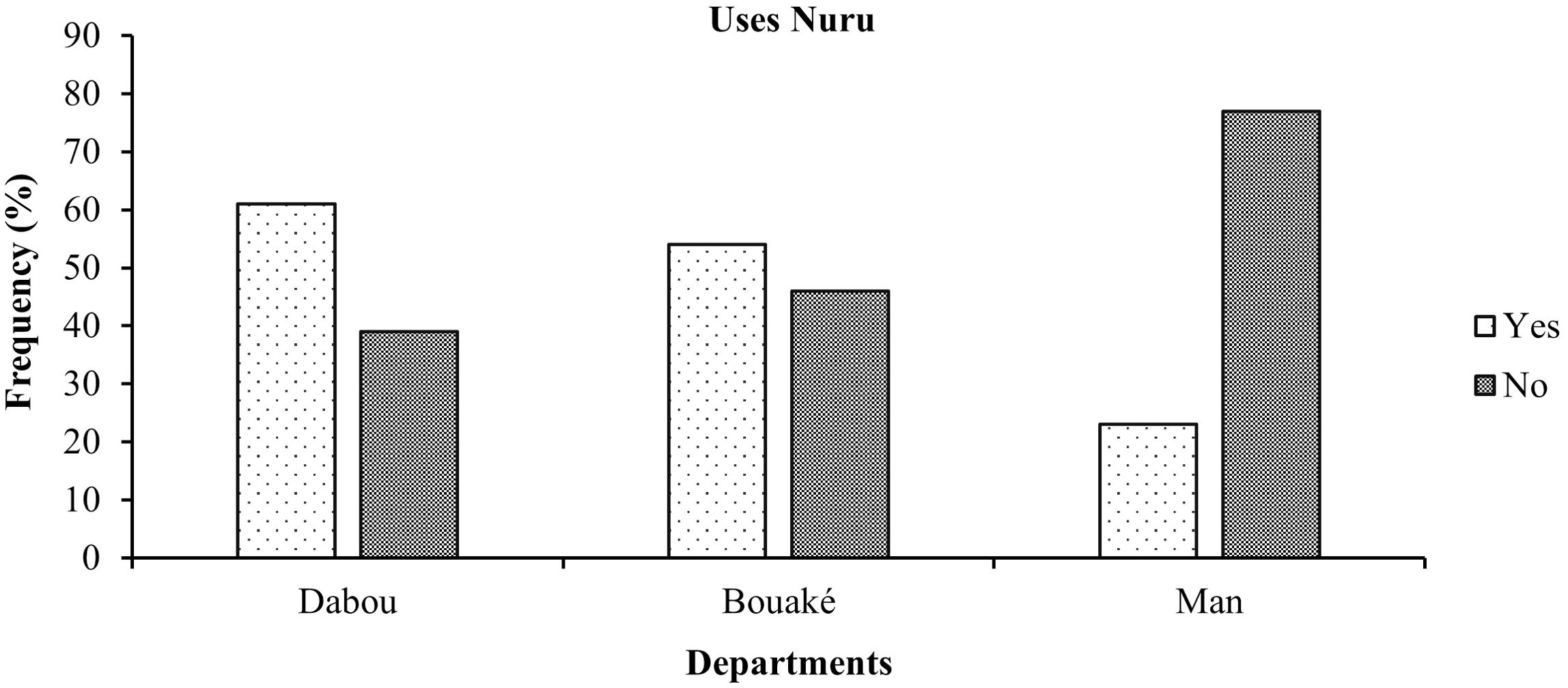
Figure 2. Use of the application by department. Data analyzed with Chi2 test of independence at 5% threshold.
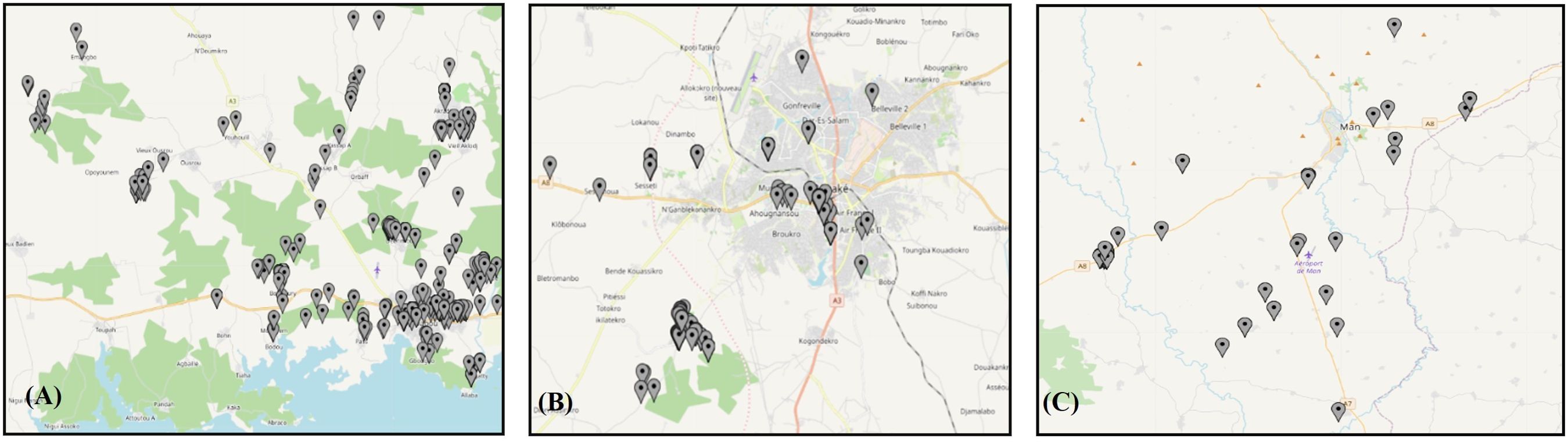
Figure 3. Geographical distribution of “PlantVillage Nuru” users by department. (A) Dabou, (B) Bouaké, (C) Man.
3.4 Mode and period of use of the application in cassava fields
The “PlantVillage Nuru” application was used by farmers to perform three main functions, including regular monitoring of the phytosanitary status of fields (98%), selecting symptom-free cuttings (52%), and obtaining agricultural advice on cassava cultivation and CMD management methods (21%). These different functions varied according to the different stages of cultivation. At the start of production, the application is used to monitor the phytosanitary status of the growing plants, with the objective of progressively replacing those which were infected (61%). This method gradually reduces the incidence of disease in the field and improves yield. During the whole production cycle (from plant growth to maturity), Nuru is used as a tool for monitoring the field (77%), and at the end of the campaign, particularly at harvest time, the application is used to select symptom-free cuttings for the coming planting season (31%). These ways of using the application are well illustrated in the words of one farmer:
« Even when the cassava plants get older and taller, I see abnormal signs on the leaves, I use the app to check. If it’s mosaic, I tie the affected plant with a distinctive colored bag and don’t use its cuttings after harvesting »3 (Figure 4).Figure 4
3.5 Trend in the number of users and frequency of use of the application
To assess the level of adoption of the “PlantVillage Nuru” application among farmers, a diachronic monitoring of the dynamics of the number of users and the frequency of use of the application was carried out in the three study areas. The monitoring was conducted over six semesters in Dabou and Bouaké, and over three semesters in Man, depending on the application’s diffusion period in the areas. The data indicated a significant difference (P<0.01) among all three study areas for these two parameters. Similar patterns were observed when analyzed by department, with significant differences (P<0.05). The number of users increased in Dabou from 5 to 47 between the first and third semesters, and in Bouaké from 10 to 25 during the same period. In Man, the number of users increased from 6 to 14 between the first and second semesters. However, there has been a stagnation in the number of users in Dabou (54 to 57), Bouaké (25 to 27), and Man (14 to 16) during the last three semesters. The frequency of use was also characterized by two dynamics. The first to the second semester was marked by an increase of the frequency in Dabou (7 to 36), Bouaké (14 to 17) and Man (8 to 47). This was followed by a gradual decline in frequency of use over the last four semesters in Dabou (32 to 9), Bouaké (6 to 1) and from the second to the third semester in Man (47 to 5). According to the farmers, this is due to the fact that:
« At the beginning, when they introduced the app, we were getting some money to cover costs and the extension officers were there to help us when we were having difficulties, and that motivated us. But then they left, and all that stopped, so some used the app less and others stopped »4.
Another farmer said:
« I don’t use the app too much anymore because over time, I can recognize on my own the cassava plants that are infected by the mosaic »5.
However, the largest number of users of the application was registered in the department of Dabou (57), while the highest average frequency of use of the application per beneficiary was registered in Man (3.75) (Figure 5).
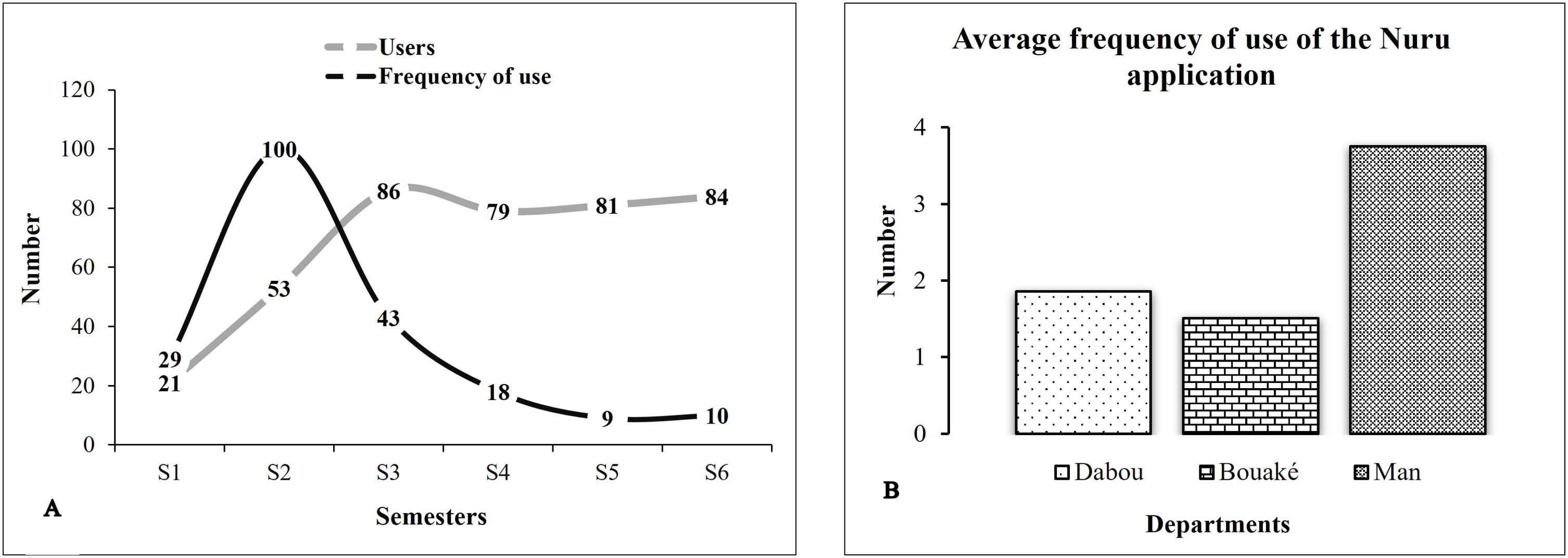
Figure 5. Trend in number of users, frequency of use and average frequency of application use by department. Data analyzed with a generalized linear model, adapted to count data with a threshold of 5%. (A) Number of users and frequency of application use. (B) Average frequency of use of the application in the surveyed departments. S, semester.
3.6 Determinants of use of the “PlantVillage Nuru” application
To determine the factors influencing the use of the Nuru application, correlation tests were first carried out to avoid biases in the estimation of the model results. Then, the binary logit regression model was performed. The results of the regression analysis indicate that the econometric model, which includes variables related to farmers’ characteristics, agronomic parameters of the fields, and farmers’ interactions with an agricultural service, is a significant predictor of the use of the Nuru application. The pseudo R2 value is 0.832, which falls within the 1% threshold. This value indicates that 83.2% of the variability in farmers’ adoption of the application is explained by the variables included in the model. In fact, the results of the marginal effects calculation indicate that nine variables or factors positively influence farmers’ use of the application.
In terms of farmer characteristics, holding all variables constant, the probability of a male farmer using the application (compared to a female farmer) is around 13%. Similarly, being educated increases the probability of using the application by 11%. Consequently, the greater the educational attainment of the farmer, the greater the probability of his or her use of the application. Furthermore, smartphone ownership and internet access also demonstrate positive associations, increasing the probability of app use by approximately 15% and 12%, respectively. In contrast, marital status exerts a negative influence on the app use. The estimator for this variable is negative, indicating that being single reduces the likelihood of using the application by approximately 4%.
Regarding the characteristics of the field, the utilization of family labor is assigned a positive estimator of 0.339. This value indicates a 34% increase in the probability of the farmer using the application if the labor used is family labor. Moreover, an expansion in the area cultivated by the farmer to produce cassava is associated with an increase in the probability of the farmer’s use of the application, with an estimated increase of approximately 25%. Consequently, the larger the field, the more likely the farmer is to use the application. Conversely, the use of hired labor and the distance from the house to the field were found to reduce the probability of farmers using the application by 9.3% and 0.3%, respectively. In other words, the probability of the farmer using the application is reduced when the farmer and/or his family members are not involved in field activities and the field is further from the home.
Concerning the interaction with agricultural services, the results indicate that the probability of farmers using the application is 17% higher among those who are monitored and supervised by an agricultural service compared to those who are not. Furthermore, the probability of using the application is increased by 18% in instances where farmers have participated in awareness campaigns and training on cassava diseases. Moreover, the provision of a smartphone by the project team has a positive impact, increasing the likelihood of using the application by 15%. Conversely, belonging to an association and having received training from a fellow farmer in the use of the application decreased the probability of using the Nuru application by 2.5% and approximately 6%, respectively (Table 5).
3.7 Main constraints associated to the use of the “PlantVillage Nuru” application
The farmers were interviewed to identify the constraints they encountered in using the application. After the interviews, it was found that the lack of personal smartphones among the farmers (65%) and the perceived complexity of the Nuru application (41%), particularly the various steps that the user needs to follow, hindered its usage. The statements collected from a farmer reveal more information, as follows:
« Here in the village, we use basic mobile phones since smartphones are too expensive for us. Even those who have them often struggle to fill in the required fields correctly before reaching the plant diagnostic phase »6.
According to other farmers, this situation is caused by the failure to install the application (33%), mainly due to poor quality of internet service in their locality, loss, or withdrawal of the smartphone by the project team (11%) for non-compliance with obligations, and some observed malfunctions (6%) during the use of the application. The latter case is illustrated by the statement of one respondent below:
« At the beginning of its release, the application encountered some technical issues, including incompatibility with certain smartphone brands, the failure to implement updates, and the mishandling of the application by some users. The users were slightly disrupted, but with the instructions we provided, the situation improved over time »7 (Figure 6).Figure 6
3.8 Perceptions of farmers regarding the use of Nuru in the diagnosis of CMD
Based on the data collected, it appeared that the “PlantVillage Nuru” application was positively appreciated by farmers. The majority (59%) found it to be very reliable or reliable (41%) as it consistently recognizes Cassava Mosaic Disease during diagnoses (93%). To this end, 46% of the users were satisfied, and 54% were very satisfied with the application’s performance. Most farmers (66%) stated that the application is necessary for the cultivation and management of cassava-related diseases, with 34% even considering it indispensable. However, the great majority (97%) of these farmers considered that further training was necessary for better use of the application.
4 Discussion
The aim of this study was to analyze the perceptions and the level of adoption the Nuru application by farmers in the diagnosis of CMD within the study sites. The analysis of the characteristics of the cassava farmers and plots, their interaction with agricultural services, and determinants of the use of the application, including the difficulties encountered by the farmers with this technology and its appreciation, allowed us to arrive at the following interpretations.
4.1 Characteristics of cassava farmers and plots
The study results suggested that cassava cultivation in our study areas was dominated by men. This situation can be justified by customary law, which makes the son or nephew the heir and legitimate landowner (Mendez del Villar et al., 2017), particularly in our study sites. In addition, the high market value acquired by cassava in recent years in Côte d’Ivoire could also contribute to this situation. However, there is a low interest in this culture among the younger population (30%), which is mostly controlled by relatively older individuals (61%). Despite their age, farmers have a high level of education, which could be explained by the beneficiaries’ selection process which targeted literate farmers to ensure the successful use of the application. Most farmers were heads of households and lived as a couple (married or cohabiting). This allows the sharing and reduction of workload according to the activities to be carried out along the production chain. In the specific case of this study, the activities include ridging, planting, weeding, regular monitoring of the phytosanitary status of the cassava plants, uprooting, destruction, replacement of infected plants, harvesting, and selection of cuttings for the new crop year. Additionally, it was observed that most of the respondents had over ten years cassava cultivation experience. These socio-demographic characteristics were previously observed by Houngue et al. (2018), Kouassi et al. (2018), and Frimpong et al. (2021) with cassava farmers in Côte d’Ivoire, Benin, and Ghana, respectively. However, in the Beninese context, a difference was identified regarding the level of education, with the majority (74,9%) of farmers who were illiterate.
In addition to these aspects, it appeared that farmers are not very organized in associations, especially in the southern (Dabou) and central (Bouaké) departments where cassava is grown individually or within the family. Similar results were observed by Yabi et al. (2016) on the study of the adoption of agricultural technologies in Benin, and Issoufou et al. (2017) with millet farmers in Niger. While in the case of Ghana, Frimpong et al. (2021) showed in their results that 77.7% of the surveyed cassava farmers belonged to an agricultural organization. Our results also showed that most farmers (65%) remained in contact with an agricultural service because of the various projects initiated in the agricultural sector in general and in cassava cultivation in particular. This rate remains significantly higher than those observed by Awotide et al. (2012) with rice farmers in Nigeria (13%) and Issoufou et al. (2017) in Niger (31.48%). Cassava acreage remain small (0.2 to 5 ha), with a mean cultivated area of 0.94 ha. These results confirm those of Djaha et al. (2018) and revealed a slight decrease compared to Kouassi et al. (2018), who observed cassava cultivated areas ranging from 0.33 to 8 hectares with an average of 1.45 hectares in the main cassava production areas of Côte d’Ivoire.
4.2 Level and determinants of adoption of the “PlantVillage Nuru” application
Our results showed a 45% adoption rate of the Nuru application across the study sites. The highest adoption rates were recorded in Dabou (61%) and Bouaké (54%) while Man recorded the lowest rate (23%). This difference could be explained by the fact that the application was more recently introduced in the Man area (18 months before the survey) compared to Dabou and Bouaké where it was deployed much earlier (36 months before the survey). It also appeared that the number of users and the frequency of application usage have been consistently increasing during the initial stages of dissemination of the application (semesters 1, 2, and 3). This evolution can be attributed to the continuous presence, monitoring, and support provided by extension officers. Then, from semesters 3 to 6, a stagnation in the number of users and a significant and continuous decrease in usage frequency were observed across all study areas due to the withdrawal of extension officers and the end of user support and assistance. These results confirm those of Aitchedji et al. (2010) and Tesfaye et al. (2016), who have already shown in Nigeria and in Ethiopia that the frequency of contacts between farmers, extension services, and the project team has an impact on the adoption of agricultural technologies. In the case of Nuru, the decline observed in the use the technology, could also be explained by the fact that, with some farmers the application strengthened the ability to recognize CMD autonomously, so it was no longer needed to identify diseased plants. In this context, the application would be more suited to periodic use. It could be used as an aid for early identification of CMD at the start of cassava plant growth, and for disease prevention when selecting cuttings for harvest. This approach could help overcome the constraints associated with permanent adoption, and the withdrawal of the project team, which puts an end to assistance to farmers.
The analysis of socioeconomic factors indicates that gender, level of education, smartphone ownership, and internet access were determinants in the use of the application, with highly significant (P<0.01) differences. Men who were more educated, owned smartphone and have internet access were the ones who used the application the most. Indeed, the application’s functionality requires a fundamental level of literacy among farmers to ensure comprehension of the instructions provided by the device. Similarly, Nuru’s efficacy hinges on the quality of the phone’s camera resolution and the necessity of internet access to transfer images captured during diagnosis. For farmers in general and women in particular, this renders Nuru a complex and costly tool to use. These results corroborate those of Tesfaye et al. (2016), who previously revealed that men are more favorable in adopting innovations than women, who remain slower in deciding to adopt new technologies and remained less able to support the cost related to its use.
Regarding agronomic parameters, the use of the application by farmers was determined by the cultivated area and the type of labor (family) used, with highly significant differences (P<0.001) respectively. Therefore, the larger the cultivated area, the more farmers use the application. Aitchedji et al. (2010) arrived at the same conclusion, indicating that the greater the space allocated to agricultural production, the more likely innovations in disease management are to be adopted. In the case of Ethiopian farmers, this variable increased the intensity of adoption of the Xanthomonas Wilt disease control strategy for enset (Ensete ventricosum). Similarly, family labor encourages the use of the application, which is a personal choice of the farmer, and their willingness to monitor the phytosanitary status of their field. This contrasts with community and salaried labor, who have no interest in it (Jogo et al., 2013).
Farmers’ interactions with agricultural structures were just as determining in the adoption of Nuru. The use of the application was indeed significantly associated with participation in WAVE’s cassava disease awareness and training campaigns, receiving a smartphone from the project team (P<0.001), and having contact with an agricultural service (P<0.01). These data showed the influence of agricultural structures in the adoption of innovations, through the knowledge disseminated to farmers during training sessions, the discovery through learning that they generate (Mfitumukiza et al., 2017).
However, the application’s characteristics and the cost of use (purchase of a smartphone and internet credits) have had a negative impact on it use. 41% of non-users found the application complex (downloading, installing, registering on the Nuru platform, correctly filling in the required fields before use) and the majority (76%) did not have a smartphone for this purpose. These results support those of Sennuga et al. (2020) on the impact of Information and Communication Technologies (ICT) on small-scale farmers in northern Nigeria. The authors demonstrated that the unavailability (79.6%) and high cost of ICT devices (72.9%) coupled with the extremely low incomes of farmers constituted a major constraint to the adoption of this technology. However, the “PlantVillage Nuru” application remained positively perceived and highly appreciated by users (100%) for the reliability of its diagnostic results, as previously stated by Mrisho et al. (2020). They indicated that Nuru’s accuracy in recognizing CMD symptoms is like that of researchers, but 1.5 times higher than that of extension officers and two times higher than that of farmers.
The results of this study showed that the “PlantVillage Nuru” application is an important technological innovation to improve cassava production in Côte d’Ivoire. It strengthens farmers skills in CMD identification and management. However, its adoption rate remains moderate overall in the dissemination areas, where it is mainly used by farmers for monitoring the phytosanitary status of fields and managing Cassava Mosaic Disease. Its use is influenced by some characteristics of the farmers, the agronomic parameters of the cassava fields and farmers’ interactions with agricultural structures. Our study shows the need to categorize the farmers by taking in account the characteristics above, to optimize their use of this new technology. Over time, the number of users and frequency of use of the application decreased in all study sites after the project team’s withdrawal. Similarly, the characteristics of the application, as well as the conditions and costs associated with its use, constitute a major obstacle to its use and widespread adoption. Prolonged follow-up, characterized by regular assistance and the establishment of a continuous exchange platform between beneficiaries and the project team, is necessary to ensure the sustainability of the application’s use and to promote its definitive adoption by farmers. Despite these constraints, farmers have a positive perception of this technology, considering it as an essential tool in managing Cassava Mosaic Disease due to its reliable diagnostics and agricultural advice. However, the application is only an aid to disease identification, and while it certainly indicates the methods to be followed, it is no substitute for human intervention. It’s up to the farmer to decide whether to follow the application’s advice. That’s why, in addition to training on the disease and disseminating the application, it’s necessary to provide farmers with healthy planting material and develop cassava varieties that are resistant to the disease. Moreover, comparative study between beneficiary and non-beneficiary areas, focusing on parameters such as the incidence of CMD, yield, and income, could help to assess the impact of the use of this application on the well-being of cassava farmers.
Data availability statement
The original contributions presented in the study are included in the article/supplementary material. Further inquiries can be directed to the corresponding author.
Ethics statement
The studies involving humans were approved by UFR Sciences sociales, Department of sociology, Peleforo Gon Coulibaly of Korhogo University. The studies were conducted in accordance with the local legislation and institutional requirements. Written informed consent for participation was not required from the participants or the participants’ legal guardians/next of kin because the participants in this study are farmers monitored and supervised by the WAVE center since 2020, as part of the WAVE-FCIAD project entitled ‘‘Use of a smart application for the diagnosis and participatory monitoring of cassava diseases in Côte d’Ivoire’’. They were informed of this study from the outset, and gave their consent. It was therefore with full knowledge of the facts that they gave us their verbal consent to participate in the study.
Author contributions
EA: Formal analysis, Investigation, Methodology, Software, Validation, Visualization, Writing – original draft, Data curation, Writing – review & editing. KT: Conceptualization, Methodology, Supervision, Validation, Visualization, Writing – review & editing. ES: Conceptualization, Methodology, Validation, Visualization, Writing – review & editing. WA: Investigation, Validation, Visualization, Formal analysis, Writing – review & editing. NK: Conceptualization, Validation, Visualization, Writing – review & editing. MK: Conceptualization, Validation, Visualization, Writing – review & editing. JP: Conceptualization, Funding acquisition, Project administration, Resources, Supervision, Writing – review & editing, Validation, Visualization.
Funding
The author(s) declare financial support was received for the research, authorship, and/or publication of this article. This study was funded by the European Union (EU) through the Biorisks project executed by the Regional Center of Excellence for Transboundary Plant Pathogens, Central and West African Virus Epidemiology (WAVE) and the Conseil Ouest et Centre Africain pour la Recherche et le Développement Agricoles (CORAF), grant number: CORAF N°: SC001_MC001 _UE-WAVE CRIS n°FOOD 2019/411-531 and the Bill and Melinda Gates Foundation and the United Kingdom Foreign, Commonwealth, and Development Office (FCDO; INV-002969; grant no. OPP1212988) to the Central and West African Virus Epidemiology (WAVE) Program for root and tuber crops, Université Félix Houphouët-Boigny (UFHB). Under the grant conditions of the Foundation, a Creative Commons Attribution 4.0 Generic License has already been assigned to the author-accepted manuscript version that might arise from this submission.
Acknowledgments
The authors would like to thank the Fonds Interprofessionnel pour la Recherche et le Conseil Agricoles (FIRCA) / Fonds Compétitif pour l’Innovation Agricole Durable (FCIAD) and the Pennsylvania State University (USA) for the technical support, the University Peleforo GON COULIBALY (UPGC) of Korhogo (Côte d’Ivoire) for the academic support, farmers, and extension officers of Dabou, Bouaké and Man, for their availability and commitment during data collection, Fidèle Tiendrébéogo, Eni Angela Obiageli, Dèwanou Kant David Ahoya, for improving the manuscript.
Conflict of interest
The authors declare that the research was conducted in the absence of any commercial or financial relationships that could be construed as a potential conflict of interest.
Publisher’s note
All claims expressed in this article are solely those of the authors and do not necessarily represent those of their affiliated organizations, or those of the publisher, the editors and the reviewers. Any product that may be evaluated in this article, or claim that may be made by its manufacturer, is not guaranteed or endorsed by the publisher.
Footnotes
- ^ The percentage was calculated by averaging the surveys conducted in 2016 (45.95%), 2017 (50.32%), and 2020 (51.37%).
- ^ The incidence of Cassava Mosaic Disease was determined in Dabou (73.33%), Bouaké (53.66%), and Man (71.11%) in Côte d'Ivoire by WAVE in 2020.
- ^ Comments collected with a farmer during the focus group held on 11/08/2023 with the farmers of Douagouin (Man).
- ^ Comments collected with a farmer during the focus group held on 02/08/2023 with the farmers of Débrimou (Dabou).
- ^ Comments collected with a farmer during the focus held on 22/08/2023 with the farmers of Koffikoffikro (Bouaké).
- ^ Comments collected with a farmer during the focus group held on 03/08/2023 with the farmers of Bouboury (Dabou)
- ^ Comments collected with a WAVE trainer during the interview conducted on 12/10/2023.
References
Agence Nationale d’Appui au Développement Rural (ANADER) (2017). Fiche technico-économique du manioc. Available online at: https://fr.scribd.com/document/457624958/fiche-technico-economique-du-manioc (Accessed April 22, 2022).
Aitchedji C. C., Tenkouano A., Coulibaly O. (2010). Factors affecting the adoption of disease-resistant plantain and banana (MUSA SPP.) hybrids in Nigeria. Acta Hhorticult. 879, 741−748. doi: 10.17660/actahortic.2010.879.80
Alene A. D., Manyong V. ,. M. (2006). Farmer-to-farmer technology diffusion and yield variation among adopters: the case of improved cowpea in northern Nigeria. Agric. Econ. 35, 203–211. doi: 10.1111/j.1574-0862.2006.00153.x
Aubert B. A., Schroeder A., Grimaudo J. (2012). IT as enabler of sustainable farming: An empirical analysis of farmers’ adoption decision of precision agriculture technology. Decision Support Syst. 54, 510–520. doi: 10.1016/j.dss.2012.07.002
Awotide B. A., Diagne A., Omonona B. T. (2012). “Impact of improved agricultural technology adoption on sustainable rice productivity and rural farmers’ Welfare in Nigeria: A local average treatment effect (LATE) technique,” in African Economic Conference. Kigali, Rwanda.
Brou G., Zohouri G., N’zué B., Kouadio Z., N’guessan A., Dibi K., et al. (2015). Reconnaître les principales maladies fongiques, bactériennes et virales pour mieux protéger la culture du manioc en Côte d’Ivoire. Guide technique. doi: 10.13140/RG.2.2.29375.15527
Chirwa E. W. (2005). Adoption of fertiliser and hybrid seeds by smallholder maize farmers in southern Malawi. Dev. South. Afr. 22, 1–12. doi: 10.1080/03768350500044065
Combessie J. C. (2007). La méthode en sociologie, collection Repères. Edition la découverte. doi: 10.3917/dec.combe.2007.01
Derra S. (2014). Déterminants de l’innovation technologique sur la biomasse agricole: cas du Jatropha Curcas au Burkina Faso. (Doctoral dissertation, Montpellier: EDEG).
Djaha K. E., Abo K., Koné T., Koné D., Koné M. (2018). Analysis of the population structure of cassava farmers, production systems, and plots’ sanitary state in Côte d’Ivoire. J. Anim. Plant Sci. 36, 5833–5843. doi: 10.4314/ijbcs.v11i1.14
FAOSTAT. (2020). Available online at: http://www.fao.org/faostat/fr/#data/QC (Accessed December 11, 2022).
FAOSTAT. (2021). Available online at: https://www.fao.org/faostat/fr/#data/QCL (Accessed November 30, 2023).
Frimpong B. N., Oppong A., Prempeh R., Appiah-Kubi Z., Abrokwah L. A., Mochiah M. B., et al. (2021). Farmers’ knowledge, attitudes and practices towards management of Cassava pests and diseases in Forest Transition and Guinea Savannah Agro-Ecological Zones of Ghana. Gates Open Res. 4, 101. doi: 10.12688/gatesopenres.13114.2
García-Vila M., Fereres E. (2012). Combining the simulation crop model AquaCrop with an economic model for the optimization of irrigation management at farm level. Eur. J. Agron. 36, 21–31. doi: 10.1016/j.eja.2011.08.003
Gillet A., Brostaux Y., Palm R. (2011). Principaux modèles utilisés en régression logistique. Biotechnol. Agro. Société Environ. 15, 425–433. Available online at: https://hdl.handle.net/2268/112603.
Hailu B. K., Abrha B. K., Weldegiorgis K. A. (2014). Adoption and impact of agricultural technologies on farm income: Evidence from Southern Tigray, Northern Ethiopia. Int. J. Food Agric. Econ. (IJFAEC) 2, 91–106. doi: 10.22004/ag.econ.190816
Houngue J. A., Pita J. S., Cacaï G. H. T., Zandjanakou-Tachin M., Abidjo E. A. E., Ahanhanzo C. (2018). Survey of farmers’ knowledge of cassava mosaic disease and their preferences for cassava cultivars in three agro-ecological zones in Benin. J. Ethnobiol. Ethnomed. 14, 1–9. doi: 10.1186/s13002-018-0228-5
Issoufou O. H., Boubacar S., Adam T., Yamba B. (2017). Déterminants de l’adoption et impact des variétés améliorées sur la productivité du Mil au Niger. Afr. Crop Sci. J. 25, 207. doi: 10.4314/acsj.v25i2.6
Jogo W., Karamura E., Tinzaara W., Kubiriba J., Rietveld A. M. (2013). Determinants of Farm-Level adoption of cultural practices for banana xanthomonas wilt control in Uganda. J. Agric. sci. 5, 70–81. doi: 10.5539/jas.v5n7p70
Kaiser D. E. (2023). Fertilizer Guidelines for Agronomic Crops in Minnesota (Minneapolis, MN, USA: University of Minnesota).
Kouakou B. S. M., Yoboué A. A. N., Pita J. S., Mutuku J. M., Otron D. H., Kouassi N., et al. (2024). Gradual Emergence of East African cassava mosaic Cameroon virus in Cassava Farms in Côte d’Ivoire. Agronomy 14, 418. doi: 10.3390/agronomy14030418
Kouassi K. M., Mahyao A., N’zue B., Koffi E., Koffi C. (2018). Status of Cassava (Manihot Esculenta Crantz) in Côte d’Ivoire: From production to consumption and evaluation of technology adoption. Eur. Sci. J. 14 (9), 285. doi: 10.19044/esj.2018.v14n9p285
Lambrecht I., Vanlauwe B., Merckx R., Maertens M. (2014). Understanding the process of agricultural technology adoption: mineral fertilizer in eastern DR Congo. World Dev. 59, 132–146. doi: 10.1016/j.worlddev.2014.01.024
Maddison D. (2007). The Perception of and Adaptation to Climate Change in Africa Vol. 4308 (Washington, DC, USA: World Bank Publications).
Mbilinyi N., Mrisho L. (2019). Introduction to PlantVillage Nuru. Available online at: https://www.docslides.com/harper/introduction-to-1631161577 (Accessed June 10, 2024).
Mendez Del Villar P., Adayé A., Tran T., Allagba K., Bancal V. (2017). Analyse de la chaîne de Manioc en Cô̂te d’Ivoire. Rapport pour l’Union Europé́enne, DG-DEVCO. Value Chain Analysis for Development Project (VCA4D CTR 2016/375-804), 157p + annexes. Available at: https://agritrop.cirad.fr/588006/.
Mfitumukiza D., Barasa B., Nankya A. M., Dorothy N., Owasa A. H., Siraj B., et al. (2017). Assessing the farmer Field schools diffusion of knowledge and adaptation to climate change by smallholder farmers in Kiboga District, Uganda. J. Agric. Extens. Rural Dev. 9, 74−83. doi: 10.5897/jaerd2016.0832
Mrisho L., Mbilinyi N., Ndalahwa M., Ramcharan A., Kehs A., McCloskey P., et al. (2020). Evaluation of the accuracy of a smartphone-based artificial intelligence system, PlantVillage NURU, in diagnosing of the viral diseases of Cassava. bioRxiv. doi: 10.1101/2020.01.26.919449
Njoroge M. K., Mutisya D. L., Miano D. W., Kilalo D. (2017). Whitefly species efficiency in transmitting Cassava mosaic and brown streak virus diseases. Cogent Biol. 3, 1311499. doi: 10.1080/23312025.2017.1311499
Patil B. L., Fauquet C. (2009). Cassava mosaic geminiviruses: actual knowledge and perspectives. Mol. Plant Pathol. 10, 685−701. doi: 10.1111/j.1364-3703.2009.00559.x
Paustian M., Theuvsen L. (2017). Adoption of precision agriculture technologies by German crop farmers. Precis. Agric. 18, 701–716. doi: 10.1007/s11119-016-9482-5
Rabbi I., Hamblin M. T., Kumar P. L., Gedil M., Ikpan A., Jannink J., et al. (2014). High-resolution mapping of resistance to Cassava mosaic geminiviruses in Cassava using genotyping-by-sequencing and its implications for breeding. Virus Res. 186, 87−96. doi: 10.1016/j.virusres.2013.12.028
Raza A., Shahid M. A., Safdar M., Tariq M. A. R., Zaman M., Hassan M. U. (2023). Exploring the impact of digital farming on agricultural engineering practices. Biol. Life Sci. Forum 27, 10. doi: 10.3390/IECAG2023-15386
Samuels J. A. (2024). Digital agriculture: revolutionizing farming practices through technology integration. ResearchGate. doi: 10.13140/RG.2.2.17600.51204
Sennuga O., Baines R. N., Conway J. S., Naylor R. K. (2020). Role of Information and Communication Technologies (ICTs) in enhancing food utilization among smallholder farmers’ households in northern Nigeria. Int. J. Inf. Knowledge Manage. 10 (1), 24–32. doi: 10.7176/ikm/10-1-05
Soro M., Tiendrébéogo F., Pita J. S., Traoré E. T., Somé K., Tibiri E. B., et al. (2021). Epidemiological assessment of Cassava mosaic disease in Burkina Faso. Plant Pathol. 70, 2207−2216. doi: 10.1111/ppa.13459
Tesfaye S., Bedada B., Mesay Y. (2016). Impact of improved wheat technology adoption on productivity and income in Ethiopia. Afr. Crop Sci. J. 24, 127. doi: 10.4314/acsj.v24i1.14s
Vernier P., N’zué B., Zakhia-Rozis N. (2018). Le manioc, entre culture alimentaire et filière agro-industrielle. Éditions Quae, 232.
Keywords: farmers, cassava mosaic disease, disease management, PlantVillage Nuru, Côte d’Ivoire
Citation: Adjéi EA, Traoré K, Sawadogo/Compaoré EMFW, Amoakon WJ-L, Kouassi NK, Kouassi MK and Pita JS (2024) Perception and adoption by cassava farmers of the PlantVillage Nuru application disseminated in the agricultural environment of Côte d’Ivoire: a case study in the departments of Dabou, Bouaké, and Man. Front. Agron. 6:1433204. doi: 10.3389/fagro.2024.1433204
Received: 15 May 2024; Accepted: 26 August 2024;
Published: 13 September 2024.
Edited by:
M. Leonor Faleiro, University of Algarve, PortugalReviewed by:
Syam Viswanath, Kerala Forest Research Institute, IndiaJustice Gameli Djokoto, Dominion University College, Ghana
Charles P. Staver, Independent researcher, Xalapa, Mexico
Copyright © 2024 Adjéi, Traoré, Sawadogo/Compaoré, Amoakon, Kouassi, Kouassi and Pita. This is an open-access article distributed under the terms of the Creative Commons Attribution License (CC BY). The use, distribution or reproduction in other forums is permitted, provided the original author(s) and the copyright owner(s) are credited and that the original publication in this journal is cited, in accordance with accepted academic practice. No use, distribution or reproduction is permitted which does not comply with these terms.
*Correspondence: Ettien A. Adjéi, ZXR0aWVuYWRqZWkzMUBnbWFpbC5jb20=