- 1Resilient Farm & Food Systems – Climate Adaptation and Mitigation Cluster (CAM), International Crop Research Institute for the Semi-Arid Tropics (ICRISAT), Dakar, Senegal
- 2Sustainable Management of Cropping Systems in Dryland Areas, Centre d'Étude Régional pour l'Amélioration de l'Adaptation à la Sécheresse (CERAAS), Thies, Senegal
- 3WASCAL Graduate Research Program on Climate Change and Agriculture, University of Sciences, Techniques and Technologies of Bamako (USTTB), Bamako, Mali
- 4Sustainable Livestock Systems, International Livestock Research Institute (ILRI), Dar Es Salaam, Tanzania
- 5Sustainable Livestock Systems, International Livestock Research Institute (ILRI), Dakar, Senegal
Understanding and identifying appropriate adaptation optons for cropping systems and management practices at spatial and temporal scales is an important prerequisite for scaling. Pearl millet (Pennisetum glaucum (L) R. Br.) could be regarded as a risk-reducing measure crop under climate change when coupled with tactical agronomic management practices. In this study, we assess the impacts of adaptation strategies such as cultivar type, planting windows, and fertilizer strategies on pearl millet production under rainfed farming systems over Nigeria and Senegal using the Agricultural Production Systems Simulator (APSIM) model. The impact of climate change on millet yield was evaluated using a validated APSIM-millet module that utilized yield data collected through participatory research and extension approach (PREA) in contrasting environments. The climate model projections for the mid-century period (2040–2069) were compared against a baseline period of 1980–2009 for both locations. During the simulation, two millet varieties (improved local and dual-purpose) with two sowing regimes were considered comparing traditional farmers’ sowing window (dry sowing) and agronomic sowing window (planting based on the onset of the rainfall) at three different fertilizer levels [low (23 kg N ha−1), medium (40.5 kg N ha−1), and high (68.5 kg N ha−1) respectively]. The performance of the APSIM-millet module was found to be satisfactory as indicated by the low Root Means Square Error (RMSE) and Normalized Root Mean Square Error (NRMSE) values. The range for grain yield was between 17.7% and 25.8%, while for AGB it was between 18.6% and 21.4%. The results showed that farmers’ sowing window simulated slightly higher grain yield than the agronomic sowing window for improved local millet cultivar indicating yield increased by 8–12%. However, the projected changes in the mid-century (2040–2069) resulted in a decline in yield against baseline climate for both varieties and sowing windows, indicating the negative impact of climate change (CC) on yield productivity. The comparison between dual-purpose millet and improved local millet indicates that disseminating the improved millet variety and implementing early sowing could be an effective adaptation strategy in reducing risks and losses caused by climate change. Similarly, low magnitude impacts simulated on grain yield (< −8% in Nigeria compared to > −8% in Senegal) even though both locations are in the same agroecological zone.
Introduction
Smallholder farmers in the Sub-Saharan African (SSA) region are particularly vulnerable to the adverse effects of climate change on their livelihoods, given their reliance on rain-fed agriculture and limited access to resources and support systems (Lobell et al., 2011; Sultan et al., 2013, 2019). Climate change has been shown to significantly impact traditional farming practices in West Africa, leading to potential crop yield losses and adversely impacting food security in the region (Singh et al., 2017). Studies estimate climate change will lead to a mean yield reduction of −11% in West Africa by the 2050s without adaptation, highlighting the need for agricultural investments to achieve development goals under changing climatic conditions (Sultan et al., 2019). Additionally, the impact of climate change on pearl millet production in West Africa is a growing concern, as changing weather patterns and extreme weather events threaten to reduce yields and increase crop losses (Rhoné et al., 2020). Yield losses in pearl millet production due to climate change-induced droughts are widespread among smallholder farmers (Singh et al., 2017).
Pearl millet (Pennisetum glaucum (L) R. Br.) is a staple food for more than 90 million people in the Sahelian region of Africa and North-western India (Srivastava et al., 2020), and the most important cereal crop for livelihoods mainly for household consumption in the region, particularly in Nigeria and Senegal. Pearl millet is a vital crop in West Africa, where it is primarily grown on marginal lands that are exposed to unpredictable rainfall patterns and environmental stresses (Satyavathi et al., 2021). The major constraints to the production of pearl millet among abiotic and biotic stresses include low and erratic rainfall, high temperatures, poor soil fertility, downy mildew disease, and widespread striga infestation (Singh et al., 2017). Climate change factors such as elevated temperatures and CO2 concentrations can also impact the growth, development, and yield of pearl millet (Singh et al., 2017; Satyavathi et al., 2021). High temperatures can reduce biomass allocation to reproductive organs and shorten the crop life cycle by decreasing seed set and seed growth rate (Rhoné et al., 2020). In tropical regions, including West Africa, a 1.5°C increase in global surface temperature above preindustrial levels is expected to cause a significant reduction in crop yield and an increase in crop failure (Sultan et al., 2013). Thus, global warming is also expected to have adverse effects on crop production, with a mean yield reduction of −8% identified in all of Africa and −11% in West Africa (Sultan et al., 2013, 2019). Unfortunately, even the most optimistic climate change scenarios will not lead to warming below 1.5°C, making crop production losses in West Africa inevitable without adaptation (Sultan et al., 2019). However, pearl millet has natural resilience to higher temperatures and lower rainfall, making it better suited to withstand abiotic stresses such as drought and heat (Satyavathi et al., 2021).
Production systems are associated with many risks especially in areas where land and water resources supporting agricultural production face challenges in meeting food security, owing to increasing population and climate change impacts (Guan et al., 2016; Ajayi et al., 2018). The constraints may be further exacerbated by unsustainable agricultural practices, and social and economic pressures (FAO, 2011; Ajayi et al., 2018). Therefore, knowledge of the most effective adaptation methods becomes critical in countering the adverse effects of climate change on pearl millet production in West Africa (Sultan et al., 2019; Satyavathi et al., 2021). Optimal prioritization of adaptation investments requires the assessment of various possible options both at tactical and strategic levels and their uncertainties (Whitbread et al., 2010; Guan et al., 2016). However, successful adaptations of agriculture to climate change should not only help farmers deal with current production risks but to cope with short-term climate variability as well as reduce the negative (or enhance positive) impacts of climate change in the long term (Lobell, 2014; Saba et al., 2013) using robust climate projections (Sultan et al., 2013; Roudier et al., 2014; Amadou et al., 2022). Therefore, resilient agriculture practices should be formulated to improve productivity and reduce food insecurity under marginal soil resources (Araya et al., 2022). Here in this study, we used the APSIM (Agricultural Production Systems sIMulator) model, which is well-validated to simulate individual farm-level management practices and yields. We further applied downscaled climate data from the Climate Model Intercomparison Project, Phase 5 (CMIP5) to assess suitable adaptation strategies for pearl millet (Pennisetum glaucum (L) R. Br.) production in West Africa. This work expands a previous impact study by Araya et al. (2022), who identified that the millet yield will either improve or unchanged under climate change. The current work takes a further step to assess possible adaptation strategies by switching from the improved local (popularly known as Souna-3) to the dual-purpose cultivars and comparing farmers’ sowing window (“dry sowing”) to agronomic sowing window (which is based on the onset of rainfall). The study hypothesis assessed adaptation management options that can benefit millet farmers under current and future climate conditions, thereby reducing the impacts of climate change. In this research, the objectives are to: (i) Evaluate farm-level millet cultivars yield under organic and inorganic fertilizer or the combination of both; and (ii) Use of sowing window and fertilizer to reduce millet farmers’ risk under mid-century climate change scenario.
Materials and methods
Model calibration and evaluation using farm-level management practices
The model parameterization and evaluation were generated from data collection obtained across the three (Thiel, Méouane, and Daga Birame) selected clusters within the Senegal Sudano-Sahelian zone. Both Méouane and Daga Birame clusters are located within the peanut basin, and Thiel is in the transition zone between the sylvopastoral and peanut basin, and pastoral area (Figure 1). The three clusters spanned across 20 villages in six administrative districts. The variability in annual rainfall is very high in these areas (Araya et al., 2022; Joseph et al., 2023). In Méouane and Thiel clusters, the onset of the rainy season is around late June to late July and the cessation of rainfall is late September to early October. In the Daga Birame cluster, the onset of the rainy season starts in early June while the cessation of the rainy season is late October. The average seasonal rainfall the Méouane cluster community receives is at least 350 mm annually in a good year and less than 300 mm in a below-average year. In the Thiel cluster, the annual average ranges between 300 and 500 mm while in the Daga Birame cluster the average rainfall ranges between 400 and 600 mm (Zagre et al., 2024). Generally, crop simulation models are site and crop-specific in nature and cannot be used in other areas unless validated under local conditions. In this study, the APSIM (Agricultural Production Systems sIMulator) model was parameterized under local conditions across the three selected clusters for pearl millet varieties being the staple cereal crop and widely cultivated by smallholder farmers. The APSIM-millet module was calibrated with yield for two categories of pearl millet (improved local and dual-purpose millet varieties) undertaken through a multi-locations field experiment piloted in the 2022 cropping season within the three clusters namely Méouane, Thiel, and Daga Birame. The multi-locations demonstration plots were part of the intervention of the AICCRA project in Senegal, to evaluate pearl millet varieties’ response to variable climate risks and farm management practices and promote best-bet climate-smart management practices for adoption as well seasonal yield forecasting using DSTs- decision-support tools (Figure 1). Millet is considered as a smart crop and can better reduce risks because it requires less fertilizer, pesticides, and irrigation inputs compared to other cereals (Anuradha et al., 2018). This makes it a more sustainable and environmentally friendly option for cultivation in dry areas. It is further known for its exceptional water use efficiency, making it a suitable crop for regions with limited water availability or under drought conditions. It also utilizes C4 photosynthesis, which has higher water use efficiency due to its specialized carbon fixation mechanism (Uppal et al., 2015; Yadav et al., 2017). It is regarded as a climate-resilient crop since it has special qualities and can adapt to harsh agroclimatic conditions (Ullah et al., 2019). It can withstand high temperatures, low soil fertility, and repeated droughts thanks to its great photosynthetic efficiency and dry matter production (Yadav et al., 2017; Anuradha et al., 2018). To enhance the effectiveness of interventions across clusters, we employ the participatory research and extension approach (PREA). We aim to expedite the adoption of comprehensive Climate-Smart Agriculture (CSA) packages, facilitating their dissemination for enhanced climate risk management. However, the data collection was obtained across the multi-location demonstration plots in 18 villages. In each cluster, six villages were associated, and, in each village, four farmers were selected for production technology using an integrated soil fertilization management (ISFM) approach to produce pearl millet given a total of 72 demonstration plots.
Soils in these zones are mainly sandy of low fertility and seasonally waterlogged or flooded clays (FAO, 1993). They are classified as Lixisol according to FAO (1993) soil classification. Soil-related modules were parameterized mainly with measured data from experiments carried out under optimal growth conditions, and from related literature. Disturbed soil samples were taken from one representative farmer field in each village at soil depths (0–15, 15–30, and 30–60 respectively) before sowing and analyzed for organic carbon (OC %), pH in water, and particle size distribution as described in Hoogenboom et al. (1999). Input data related to soil characteristics include soil texture, number of layers in soil profile, soil layer depth, soil pH for each depth, clay, silt, sand contents, organic matter, cation exchange capacity, etc. Similarly, daily rainfall record within a 2 km radius of the demonstration plots, in each village was observed during the growing season and merged with other parameters including minimum and maximum temperatures, and solar radiation downloaded from the NASA Power database for Climatology Resource for Agroclimatology, National Aeronautics and Space Administration (NASA) (http://power.larc.nasa.gov, assessed on 10 June 2023) for the corresponding farm’s reference coordinates.
The demonstration plots followed the split-plot design having four fertilizer treatments and two millet varieties, farmers within the same village were used as replications, and each plot size was 63m2 (10 m × 6.3 m) with 7 rows, sown at 0.45m within a row. The varieties evaluated at the Thiel cluster were Souna 3, an improved local variety, and Thialack-2(dual-purpose variety), Méouane cluster was Souna 3, an improved local variety, and SL 28 (dual-purpose variety) while the Daga Birame cluster was Souna 3, an improved local variety and SL 423 (dual-purpose variety). The fertilizers treatments used were: T1 – 150 kg of NPK (15:15:15) + 100 kg of urea (46%N), T2 – 150 kg/ha (NPK)+ 100 kg/ha (urea) + 2.5 ton/ha cow manure, T3 – 75 kg/ha (NPK)+ 50 kg/ha (urea) +1.25 ton/ha cow manure, T4 – 75 kg/ha (NPK) + 2.5 ton/ha (cow manure). The quantity of cow manure was determined based on the National recommendation of 5ton/ha for millet and sorghum in Senegal which farmers hardly meet requirements. In this study, we considered 50% and 25% of the recommended rate incorporated with inorganic fertilizer sources (Table 1). In addition, a study by Akinseye et al. (2023) who found a progressive increase in grain yield with an increasing level of cow manure (CM) from 0 to 100 g/hill under sorghum production in Mali. The concentration of NPK in cow manure used as an organic fertilizer treatment is 0.37% nitrogen, 0.41% phosphorus, and 0.44% potassium respectively. Planting dates varied across the clusters which was determined based on the onset date of rainfall. In the Thiel cluster, farmers sown between 14 and 27 July, at the Meouane Cluster farmers sown between 20 and 25 July while farmers in the Daga Birame cluster sown between 13 and 25 June respectively. The phenological data such as days to flowering and physiological maturity were determined following the breeder’s records for each cultivar.
However, for better model calibration some parameters of the species file were adjusted. The trial-and-error method was used to determine genetic coefficients manually (Godwin and Singh, 1998). The parameter value was adjusted to minimize Root Mean Square Error (RMSE) between simulated and measured data. For model evaluation, only the simulated grain yield and above-ground biomass (AGB) were compared with the farm-level observed data. Different statistical indices were employed, including Mean Bias Error (MBE), Root Mean Square Error (RMSE), and Normalized Root Mean Square Error (NRMSE) expressed in percentage (Loague and Green, 1991). Normalized RMSE gives a measure (%) of the relative difference between simulated and observed data. The simulation is considered excellent with a normalized RMSE is less than 10%, good if the normalized RMSE is greater than 10% and less than 20%, fair if the normalized RMSE is greater than 20 and less than 30%, and poor if the normalized RMSE is greater than 30% (Jamieson et al., 1991).
Long-term simulations with different crop management practices for current and future climate
APSIM model simulates the long-term dynamics of soil water, organic matter, nutrients, crop growth, and yield (Nelson et al., 1998; MacCarthy et al., 2010) in response to weather conditions and management practices. For climate change impact assessment, historical daily weather data (1980–2009) represented a baseline climate were derived from the global bias-shifted Modern-Era Retrospective analysis for Research and Applications dataset (MERRA) (Rosenzweig et al., 2013) for two sites selected in two agroecological zones (AEZs). The first site is Nioro du Rip, Senegal which falls within the Sudano-Sahelian zone of Senegal referred to as “Nioro-Senegal” and the second site is Kano, Nigeria – representing the Sudan Savanna zone in Nigeria. Also, 30 years of projected weather data were downscaled from 2040 to 2069 for the same sites. The decision to focus on the time scale of 2040–2069 was influenced by previous research, which indicated that there were no significant differences in simulated cereal crop outcomes between the baseline climate (1980–2009) and the near-term (2010–2039). Additionally, it was deemed inappropriate to rely on the end of the century (2070–2099) for immediate adaptation strategies for seasonal crops, considering the unpredictability and changes in climate patterns. These considerations were based on studies conducted by Akinseye (2015) and Adiku et al. (2015). Both sites have a mono-modal rainfall pattern with a short growing season (May–Oct). On average, more than 60% of rain is recorded in July and August which implies the peak of the rainy season in both locations. However, the inter-annual variability in Nioro-Senegal is lower (CV = 16.8%) than that of Kano (CV = 23%). The estimated onset of the growing season (OGS) and length of the growing season (LGS) followed the findings reported by Akinseye et al. (2016). At both sites, OGS and LGS slightly varied predicted early OGS with an average onset date of May 19th and longer LGS of an average of 138 days for Kano-Nigeria compared to Nioro-Senegal estimated an average onset date of June 25th and LGS of an average of 120 days. In both sites, mean monthly minimum and maximum temperatures show a significant warming trend throughout the growing season. Minimum temperature (Tmin) ranged from 18 to 24.5°C in Nioro and 21 to 25°C in Kano, while maximum temperature (Tmax) varied from 31.8 to 38.7°C in Nioro, 30.9 to 37.8°C in Kano. Furthermore, to downscale future climate scenarios for mid-century (2040–2069), an R script was used to generate a simple delta-based implemented within AgMIP protocol for climate change projections from the Fifth Coupled Model Intercomparison Project (CMIP5; (Taylor et al., 2012; Rosenzweig et al., 2013). These scenarios are based on historical daily weather time series perturbed for future periods following the high representative concentration pathways (RCP8.5; Knutti and Sedláček, 2013). The simulations for the current climate assumed a CO2 concentration of 360 ppm, while the future climate simulations assumed a CO2 concentration of 571 ppm for mid-century (Rosenzweig et al., 2013). Five Global Climate Models (GCM) outputs (CCSM4, GFDL-ESM2M, HadGEM2-ES, MIROC5, and MPI-ESM-MR) were selected due to their long history of development and evaluation, higher resolution, and established performance that captured a profile of the full ensemble of temperature and precipitation changes for the West Africa region (Adiku et al., 2015; Ruane et al., 2017; Akinseye et al., 2020). The seasonal analysis of yields and water use involved a simulation based on 30 years of historical weather data on two types of pearl millet (Improved local and Dual-purpose). The study considered two different planting windows: the traditional “farmer’s method” and the agronomic sowing window. Additionally, three levels of N-fertilization strategies (low, medium, and high) were examined using the integrated soil fertility management approach (ISFM) with fertilizer. The “farmer’s method” involved dry sowing of pearl millet approximately two weeks before the first major rain, following the traditional practice of farmers in both agroecological zones. On the other hand, the agronomic sowing window, also known as the “onset sowing window,” occurred at 20-day intervals after the onset of the rainy season.
ISFM approach involves the combination of inorganic fertilizer with organic fertilizer using micro-dosing technology, which not only increases yield productivity but also serves as an adaptation strategy to mitigate climate-related risks and enhance farmers’ profitability. By combining organic and inorganic inputs, the fasting effects of nutrients are prolonged, and the physical properties of the soil are improved compared to using either source alone. The soil fertility enhancement technologies that have been developed over the years for staple food crops in West Africa, like sorghum and millet, are considered climate-smart practices to mitigate the risks posed by climate change and improve farm-level interventions. During the simulation, fertilizer strategies include three levels of N-fertilizer: Low-N = 23.7 kg/ha (2.5 tons/ha cow manure + NPK 15:15:15), Medium-N = 40.5 kg/ha (1.25 tons/ha cow manure + 75 kg/ha of NPK 15:15:15 + 50 kg/ha of urea 46%N), and High-N = 68.5 kg/ha (150 kg/ha of NPK 15:15:15 + 100 kg/ha of urea 46%N). Cow manure and NPK 15:15:15 were applied at sowing, while urea (46% N) was applied as a top dressing at 30 DAS. The sowing depth was set at 5 cm, with a planting density of 3.7 plants m−2. To simulate the farmers’ practices in both regions, a non-successive simulation (single season, non-rotation mode) was adopted, which involved resetting the water, organic matter, nitrogen, and phosphorus a few weeks before the start of the growing season.
The analysis involved examining the simulated outputs to determine how sowing windows and fertilizer strategies impact millet yield and water use under the current climate. Additionally, the study evaluated inter-annual variability using the coefficient of variation (CV%) across different treatments for both varieties of pearl millet. To measure the influence of climate change on yearly simulated yields, the relative yield change was calculated for each variety and sowing window under future climate in the mid-century against the baseline climate conditions. This calculation was performed for each GCM, and the time horizon determined the effect of the sowing window on each variety based on the collective outputs. Based on the findings, the analysis also provides recommendations for suitable adaptation strategies to be implemented during dry and wet years for both categories of simulated pearl millet.
Results and discussion
Effect of fertilization strategy and millet varieties on yield performance in three clusters
The utilization of farmer-participatory production technology (as shown in Table 2) revealed significant variations in grain and stover yields among pearl millet varieties and different fertilization strategies. Across all clusters, the grain yield of millet exceeded 1000 kg ha−1, which is higher than the national average millet production in Senegal (917 kg ha−1) as reported by FAOSTAT (2021). Notably, the average yields of dual-purpose grain and Stover millets (Thialack-2, SL 423, and SL 28) were slightly greater than those of the local improved varieties (Souna3), except for the Stover yield in the Méouane cluster. The yield differences were 41% (Thiel), 18% (Méouane), and 13% (Daga Birame) higher compared to the improved local variety (Souna3). The significant coefficient of variations (CV > 20%) observed for both grain and Stover yield among pearl millet varieties across the three clusters indicates the influence of varying soil fertility and the spatial distribution of villages within the cluster in terms of rainfall. Nevertheless, the dual-purpose millet varieties are considered potentially adaptable options that can mitigate climate-related risks, and enhance yield potential, even in the face of changing climatic conditions, particularly when rain delays. This finding aligns with the research conducted by Traore et al., 2014, according to a study conducted, it was found that millet varieties with shorter growing seasons are well-suited for cultivation when the onset of rain delays in Mali.
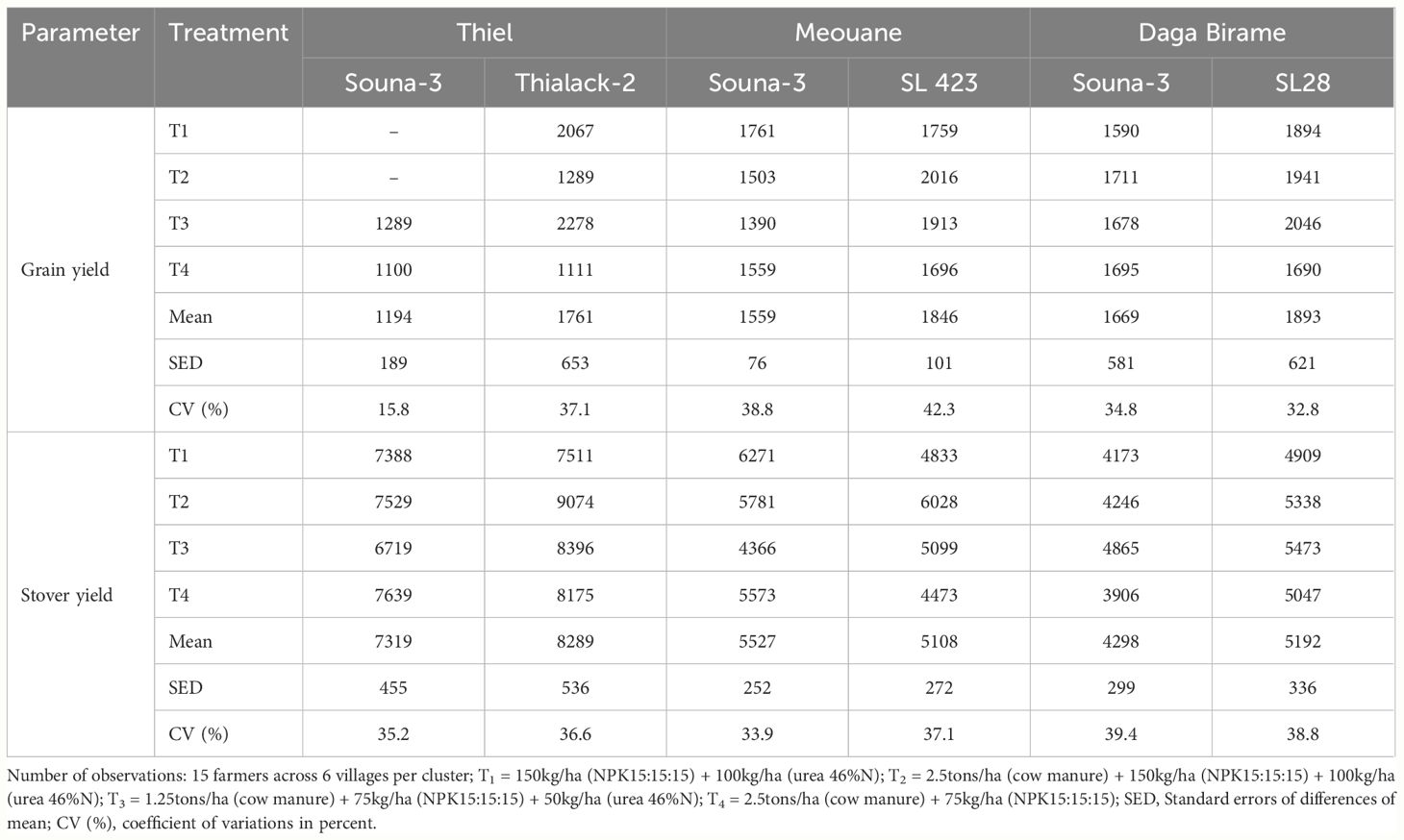
Table 2 Effect of the fertilization strategies on grain and stover yields (kg ha−1) of pearl millet varieties in Thiel, Meouane, and Daga Birame clusters respectively.
Model performance in predicting farm-level yields
Crop productivity in drylands, especially where millets are grown, is strongly affected by rainfall patterns and soil fertility management. To improve the accuracy of crop models for farm-level decision-making, it is important to evaluate models to predict yields and management practices, especially fertilization strategies. Although the results are not presented in this study, the model successfully predicted the number of days to flowering and physiological maturity, aligning with the crop breeder’s declared values for both varieties. Table 3 presents the statistical indicators such as MBE, RMSE, and NRMSE (expressed as percentages) compared simulated and observed values of farm-level grain yield and above-ground biomass (AGB) for model accuracy and reliability. The results showed good agreement between simulated and observed values, with low RMSE and NRMSE values between 17.7% and 25.8% for grain yield and between 18.6% and 21.4% for AGB.
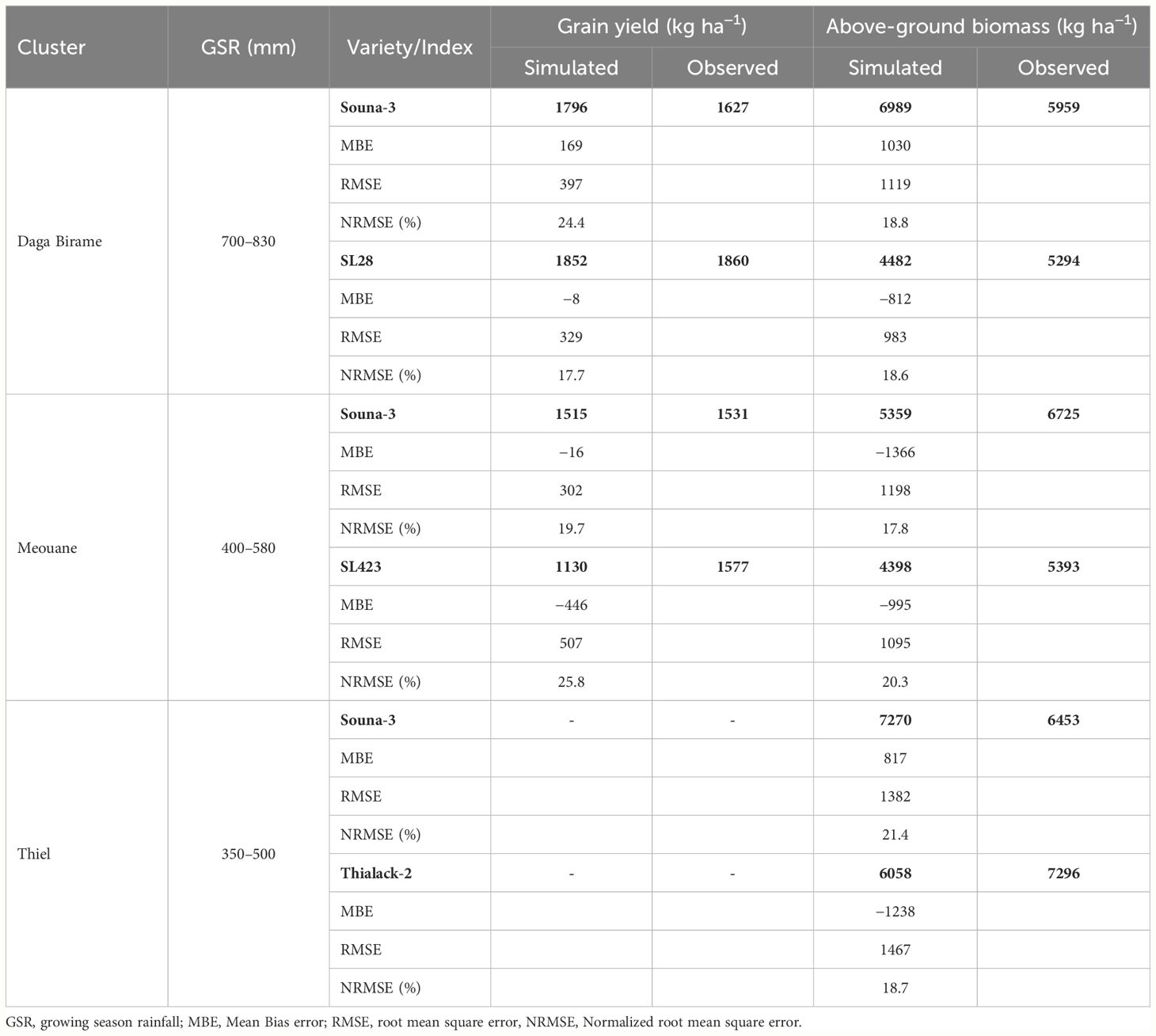
Table 3 Statistical indicator for evaluating the performance of APSIM-millet module in simulating farm-level grain yield and biomass production produced under different fertilizer strategies during the 2022 growing season.
From this study, the APSIM-millet model proved to be a promising tool for examining different farm management options under variable climate conditions and determining the most effective ones for crop production. When analyzing the performance of individual clusters using MBE between the simulated and observed values, the Daga Birame cluster showed that the model overestimated grain yield and AGB for improved local millet (Souna-3), while underestimating both for dual-purpose (SL28). In Méouane, both improved local millet and dual-purpose (SL423) had underestimated grain yield and above-ground biomass. In the Thiel cluster, only AGB was assessed, with the model overestimating improved local millet and underestimating dual-purpose (Thialack-2). Numerous studies have confirmed the accuracy of the APSIM model, highlighting its usefulness in optimizing agricultural practices and mitigating climate-related risks. For example, Adiku et al. (2015) conducted a study in semi-arid regions of Ghana and Senegal (Nioro) where the APSIM model successfully simulated farm-scale maize and peanut yields. They also observed that APSIM tends to overpredict millet yields at low yield ranges. Similarly, MacCarthy et al. (2009) demonstrated the capacity of APSIM model in simulating the effects of inorganic fertilizer application and residue management on sorghum yields in various agricultural management systems in the semi-arid region of Ghana. They emphasized the good response of APSIM to both organic and inorganic fertilizer applications in influencing temporal sorghum grain production. Akinseye et al. (2017) calibrated and evaluated the APSIM model in Mali and showed that the model accurately reproduced the observed yields of four different sorghum varieties at different sowing dates.
Analysis of projected climate in the mid-century (2040–2069) across the study areas
The projected changes in future climate over the two areas recorded show that in Nioro, total seasonal rainfall decreased (−14.1%) compared to baseline while in Kano, there is an increased change (7.2%) (Figure 2). The rainfall decreases over Nioro agreed with earlier projected changes by Adiku et al. (2015) for mid-century under RCP8.5 for median precipitation change ranged between −20 and −5% for Senegal. This projection in West Africa highlighted at the same time, an increase in change in Kano by up to 10%. Regarding temperature, the results revealed increasing in both minimum and maximum temperature throughout the growing season in Nioro and Kano which is consistent with the projected changes in West Africa given by Adiku et al. (Adiku et al., 2015; Akinseye, 2015) for mid-century RCP8.5. Indeed, temperature increases are projected faster in Kano than in Nioro du Rip, however, the minimum is projected faster than the maximum temperature in Kano. Temperature increases during the rainy season can immediately result in decreased yields for most food crops, which will lower food output (IPCC, 2014). Furthermore, LGS and NRD projected decreased change over Nioro but no significant change at Kano. This result emphasized that rainfall variability over West Africa is critical even within the same agro-climatic zone. Ibrahim et al. (2012) projected a decrease in the number of low rainfall events (0.1–5 mm/d) by 3% and an increase in the number of strong rainfall events (>50 mm/d) by 15% on average. They also emphasized that the onset of the rainy season is consistently projected to be delayed by one week on average, and there is a consensus among models regarding the lengthening of dry spells by about 20% for the period 1971–2000 to 2021–2050. On the other hand, Hess et al. (1995) observed a consistent decrease in annual rainfall of 8 mm/year across four stations in Nigeria, with most of the reduction occurring in August or September. They further noted that this reduction in rainfall was primarily due to a decrease of 25 days in the number of rainy days during the rainy season, resulting in longer dry spells of up to 1.5 days.
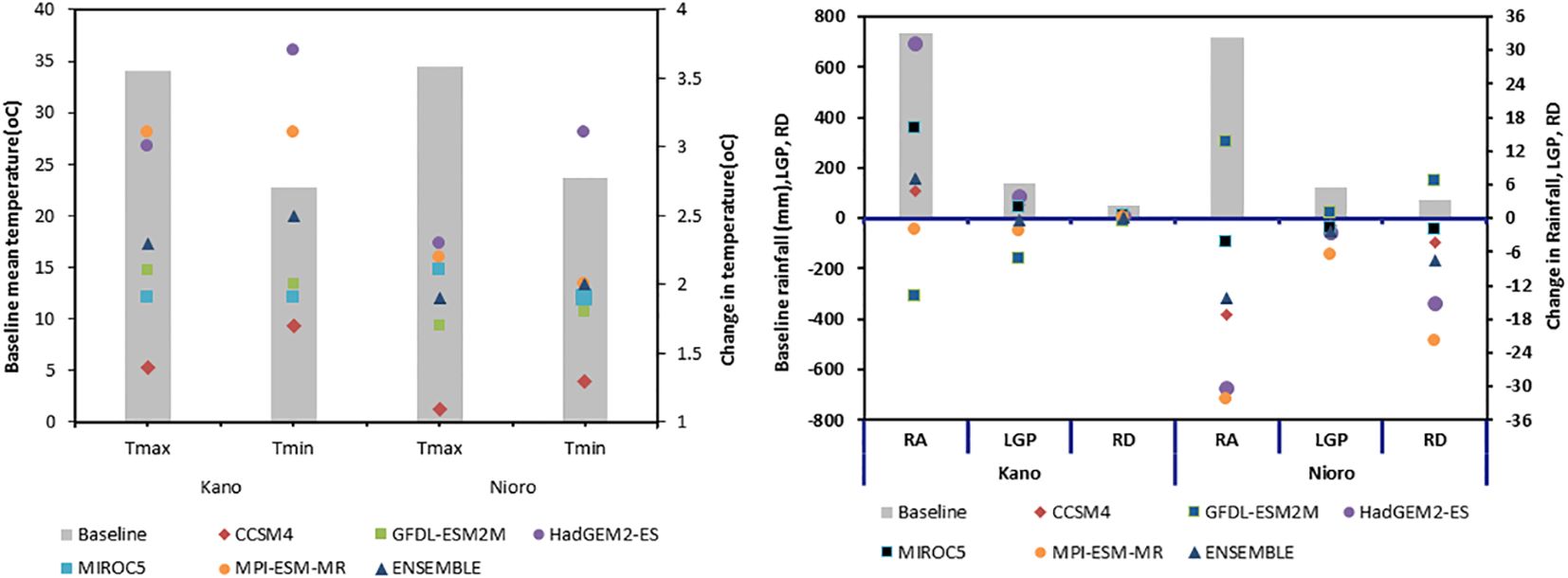
Figure 2 Change in temperatures and moisture parameters between baseline (1980–2009) and projected mid-century (2040–2069) for the selected GCMs under RCP8.5 for two representative sites, Nioro du Rip, Senegal, and Kano, Nigeria. RA, Rainfall amount (May–Oct); LGS, length of the growing season; NRD, number of rainy days (May–Oct).
Impacts of sowing windows and fertilizer strategies on millet yield under the current climate
The impact of the sowing window (SW), fertilization strategy (FS), and cultivar (C) on simulated grain yield was found to be significant in both locations, but FS did not have a significant effect on grain yield in Nioro as shown in Table 4. However, there was a significant interaction between SW and C, but no significant interactions were observed between SW and FS, FS and C, and SW, FS, and C in both locations. It is worth noting that the coefficient of variation (CV%) for simulated grain yield was lower in Kano, Nigeria compared to Nioro, Senegal. The simulated grain yield was influenced by SW and C, with FS having little impact, except in Kano, Nigeria. This can be attributed to the role of water availability in nutrient absorption and movement within the plants. Adequate water is crucial for the absorption and transportation of nutrients from the roots to other parts of the plants (Araya et al., 2022). Crop water stress, caused by high evapotranspiration and increased water demand under high temperatures, can affect the growth season (Fatondji et al., 2012; Amouzou et al., 2019). In addition, a study conducted by Ochieng et al. (2023) found the use of optimized fertilization strategies at a rate of 50 kg N ha−1 in water-scarce agro-ecologies in Sub-Saharan Africa resulted in increased yield stability, rain use efficiency, and gross returns in maize production. Similarly, a long-term field experiment conducted by Ranjan et al. (2023) highlighted the benefits of using a combination of 50% of the recommended dose of fertilizers and 50% nitrogen from farmyard manure. This approach not only increased soil organic carbon by 46.4% but also improved grain yield by 24.7% and enhanced agronomic efficiency. Furthermore, it led to a reduction in greenhouse gas emissions in rice and wheat production.
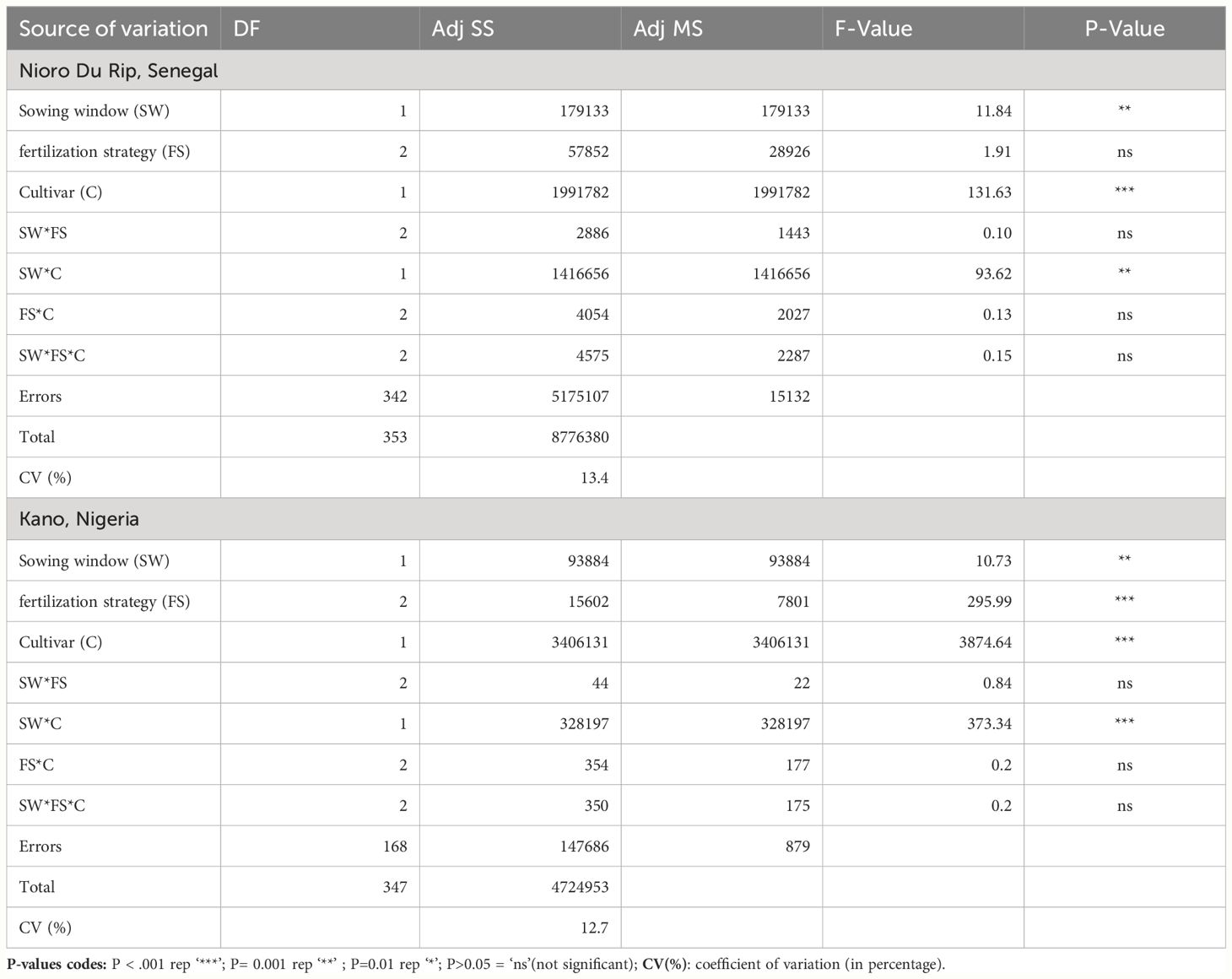
Table 4 p‐value and coefficient of variations (CV%) of ANOVA test of sowing window and fertilizer strategy on millet grain yield simulated between 1990 and 2020 over Nioro du Rip, Senegal and Kano, Nigeria.
Figure 3 further reveals that the farmers’ sowing window resulted in slightly higher simulated yields compared to the Onset sowing window for the improved local millet variety, indicating a yield gain of 8–12%. When comparing dual-purpose millet to improved local millet, it was found that the former yielded slightly higher when sown using the rainfall onset method. This indicates that dry sowing, which is the preferred method for local millet varieties, is not suitable for dual-purpose millet varieties. This finding emphasizes the importance of combining the scaling up of improved dual-purpose varieties with knowledge of rainfall onset to maximize yield gain. Joseph et al. (2023) discussed the impact of rainy season onset on rainfall distribution and maize yield in Senegal, highlighting its variability. Several studies, including Akinseye et al. (2016, 2023), Egbebiyi et al. (2020), and Soler et al. (2007, 2008), have shown that missing the optimal planting window would negatively affect crop yield. Mohamed et al. (2023) predicted that sowing dates could lead to a 50% reduction in millet yield in the Sahel zone. Sultan et al. (2013) further noted that this yield decline due to climate change is expected to be higher for early-duration genotypes that do not adopt climate-smart strategies. The significance of integrating intelligent tactics, such as selecting the ideal time for sowing and utilizing enhanced varieties, was underscored by the findings of this study. This approach effectively enhances the production capacity of farmers. The study further reveals the astuteness of farmers who choose to engage in dry sowing of pearl millet to avoid the negative impact of delayed onset, a fact that has been substantiated by numerous research studies.
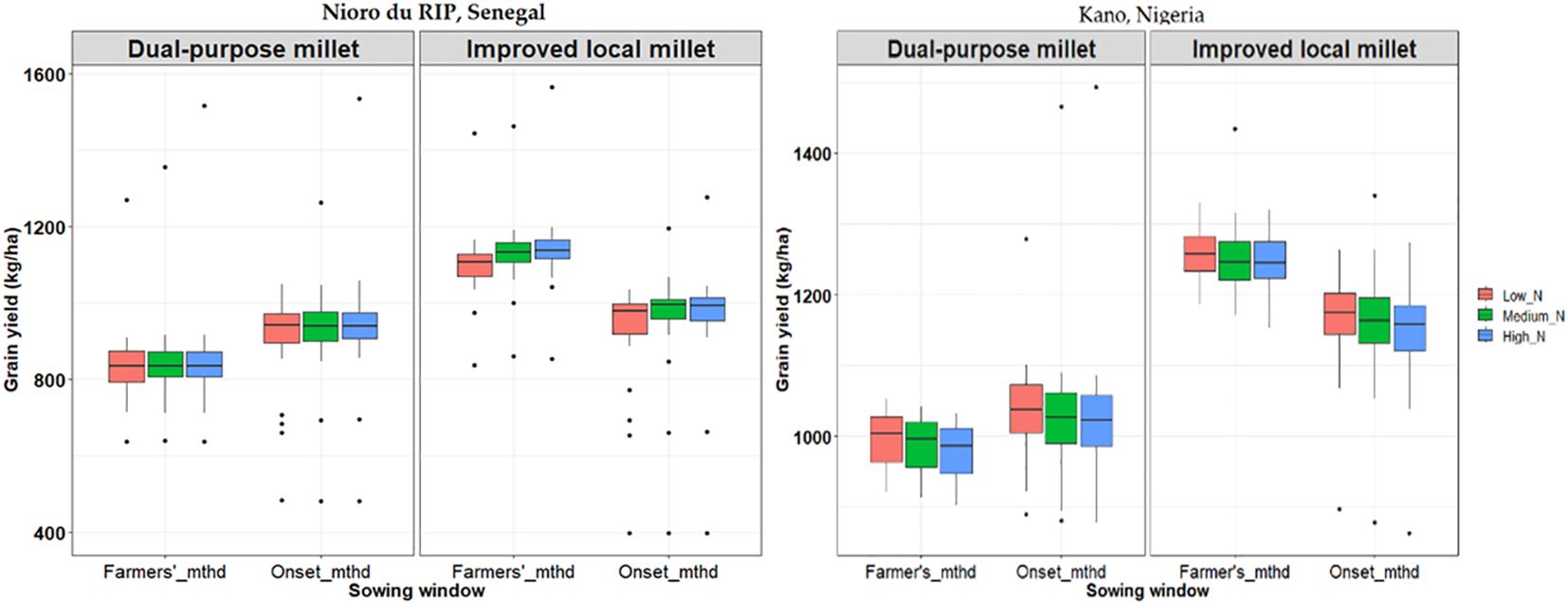
Figure 3 Impacts of sowing window and fertilizer strategies on millet yield under the current climatic conditions.
Impact of future climate change on millet yield
The results recorded that all GCMs simulated decline yield against baseline climate for both cultivars and sowing windows (Figure 4). The negative impact of future CC on yield has a lower magnitude change on dual-purpose millet compared to improved local millet. Several studies (Pilloni et al., 2022; Faye et al., 2023) were done to compare dual-purpose millet and farmers’ local varieties and mentioned that it provides a higher grain-fodder ratio under suitable spacing for human and livestock feeding. This implies improved millet variety promotion and early sowing could serve as a good strategy to minimize risk or losses under CC. These results are consistent with Traore et al. (2014) who mentioned that a short-duration variety of millet was particularly well-suited for a late planting date. This indicates that specific millet varieties have been developed and adapted to maximize their yield potential even under changes in climate variability. Deeply, low magnitude impacts simulated on grain yield in Kano, Nigeria compared to Nioro du Rip, Senegal despite the similarity in agroecology could be associated with inherent soil fertility such as organic carbon than additional inputs applied. It could be also associated with the variability of rainfall even within a similar agroecology zone. Indeed, in Sahelian zones, rainfall is a significant determinant of crop production but unfortunately, rainfall is highly variable in time and space (Aman et al., 2019). On the other hand, Graef and Haigis (2001) conducted research in the Sahel zone and found that rainfall can vary considerably even within a few kilometers and across different time scales.
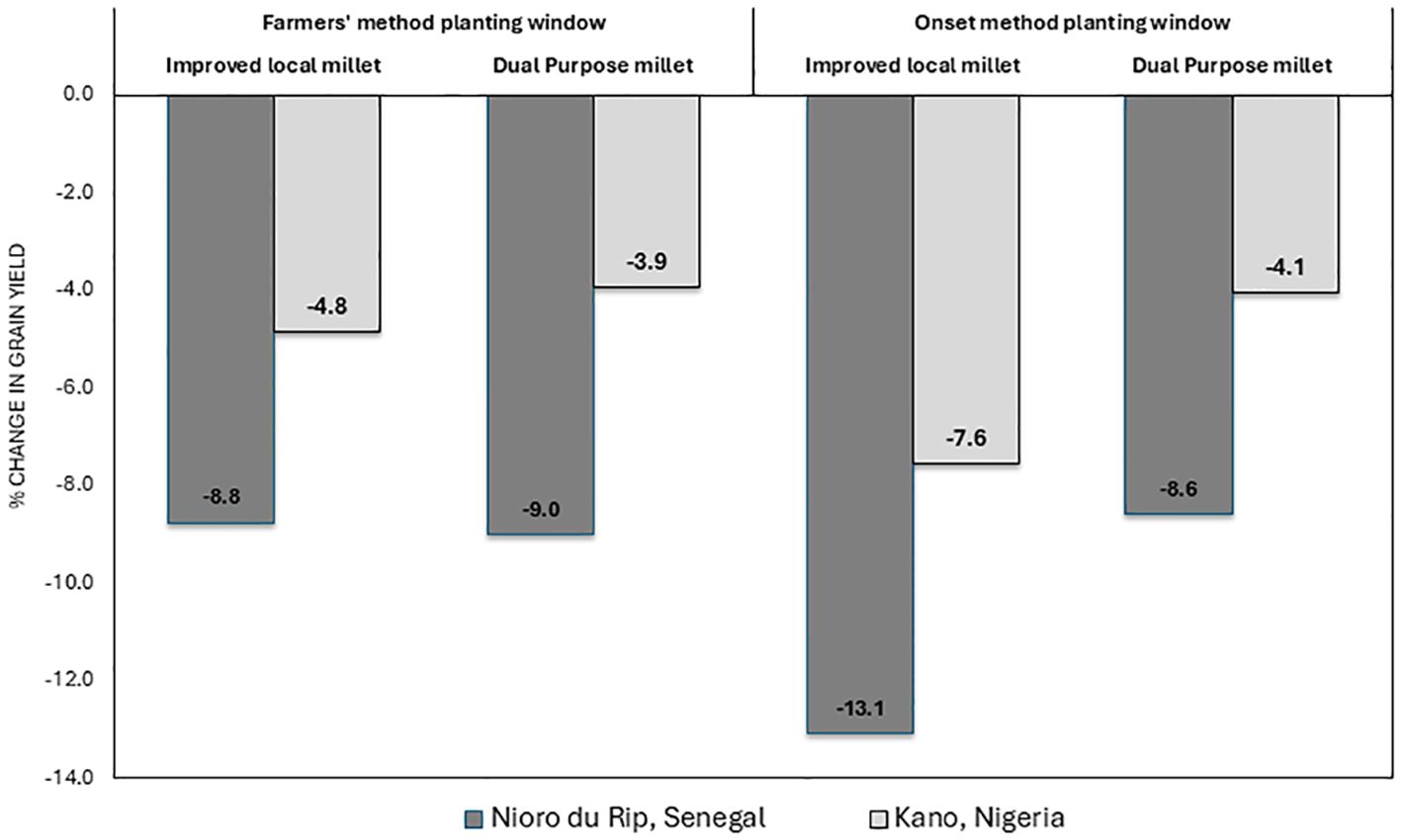
Figure 4 Percentage change in pearl millet yield under climate change scenarios by the mid-century (2040–2069).
Conclusion
The study highlights the significance of integrating indigenous knowledge with innovative agricultural practices to enhance the resilience and productivity of smallholder farmers in dryland farming systems particularly for pearl millet. The emphasis is to provide the urgent need for tailored interventions that address the complex interplay of factors influencing smallholder farmers’ ability to adapt and thrive in the face of climate change, contributing to the adaptation strategies and knowledge in the field of agricultural sustainability. interestingly, there was no significant difference among fertilization strategies, suggesting that a low nitrogen (N) application at 27.4 kgha−1, which combines organic and inorganic fertilizers, is the most suitable to achieve optimal yield without increasing inter-annual variability under baseline climate conditions (1980–2009). On the contrary, the sowing window demonstrated significant yield productivity as farmers’ sowing window resulted in slightly higher grain yield than the onset sowing window for the improved local millet variety, leading to a yield increase of 8–12% compared to the dual-purpose millet. Conversely, the onset sowing window yielded a higher grain yield for the dual-purpose millet than that of the improved local pearl millet variety. Under climate change (CC), millet yield was observed to have a minimal and negative effect, though with varying degrees between locations, indicating that millet is not highly susceptible to climate change and variability. Instead, the management practices and types of cultivars play a more significant role. Interestingly, the impact of climate change on grain yield appears to be more pronounced in Senegal compared to Nigeria. This discrepancy could be attributed to the increasing temperature changes rather than changes in rainfall patterns, as well as the low organic carbon content in the soil and limited additional inputs. Our research has demonstrated that achieving optimal millet yield is closely associated with selecting the appropriate sowing window and cultivar type. This strategic adaptation approach can be effective, especially when minimal nitrogen input (27.4 kg ha−1) is applied. The growth and development of millet are highly influenced by the duration of daylight and the availability of soil moisture, which ultimately determine productivity.
Data availability statement
The original contributions presented in the study are included in the article/supplementary material, further inquiries can be directed to the corresponding author.
Author contributions
FA: Conceptualization, Data curation, Formal analysis, Methodology, Project administration, Writing – original draft, Writing – review & editing. IZ: Data curation, Validation, Writing – review & editing. AF: Project administration, Resources, Writing – review & editing. JJ: Formal analysis, Methodology, Validation, Writing – review & editing. NW: Writing – review & editing. AW: Funding acquisition, Project administration, Validation, Writing – review & editing.
Funding
The author(s) declare financial support was received for the research, authorship, and/or publication of this article. The study was co-funded by AICCRA (Accelerating CGIAR Climate Impact Research in Africa) by the World Bank (Project ID 173398) and One CGIAR Initiative on Climate Resilience (also known as the ClimBeR project) through a collaboration between ICARDA and ICRISAT.
Acknowledgments
The authors thank the International Crops Research Institute for the Semi-Arid Tropics (ICRISAT) and the Centre for Regional Studies (CERAAS) for their institutional support for this study. This research was contributed by the Global Research Program “Resilient Farm and Food Systems” under Climate Adaptation and Mitigation Research Cluster.
Conflict of interest
The authors declare that the research was conducted in the absence of any commercial or financial relationships that could be construed as a potential conflict of interest.
Publisher’s note
All claims expressed in this article are solely those of the authors and do not necessarily represent those of their affiliated organizations, or those of the publisher, the editors and the reviewers. Any product that may be evaluated in this article, or claim that may be made by its manufacturer, is not guaranteed or endorsed by the publisher.
References
Adiku S. G. K., Maccarthy D. S., Hathie I., Diancoumba M., Freduah B. S., Amikuzuno J., et al. (2015). Climate change impacts on West African agriculture: an integrated regional assessment (CIWARA). Handbook of Climate Change and Agroecosystems., 25–73.
Ajayi T., Fatunbi O., Akinbamijo O. (2018). Strategies for Scaling Agricultural Technologies in Africa. Forum for Agricultural Research in Africa (FARA), Accra Ghana. doi: 10.48565/bonndoc-23
Akinseye F. M. (2015). Factoring climate variability and change into crop models for enhancing Sorghum performance in the West African Semi-Arid Tropics. Available online at: http://www.wascal.org/publications/doctoral-theses/.
Akinseye F. M., Adam M., Hoffmann M. P., Traore P. C. S., Agele S. O., Whitbread A. M. (2017). Assessing crop model improvements through comparison of sorghum (Sorghum bicolor l. moench) simulation models: a case study for west african cultivars. Field Crop Res. 201, 19–31. doi: 10.1016/j.fcr.2016.10.015
Akinseye F. M., Agele S. O., Traore P. C. S., Adam M., Whitbread A. M. (2016). Evaluation of the onset and length of the growing season to define planting date–a’ case study for mali (West africa). Theor. Appl. Climatology 124 (3–4), 973–983. doi: 10.1007/s00704-015-1460-8
Akinseye F. M., Ajegbe H. A., Kamara A. Y., Adefisan E. A., Whitbread A. M. (2020). Understanding the response of sorghum cultivars to nitrogen applications in semi-arid Nigeria using the agricultural production systems simulator. J. Plant Nutr. 43, 834–850. doi: 10.1080/01904167.2020.1711943
Akinseye F. M., Birhanu B. Z., Ajeigbe H. A., Diancoumba M., Sanogo K., Tabo R. (2023). Impacts of fertilization management strategies on improved sorghum varieties in smallholder farming systems in Mali: Productivity and profitability differences. Heliyon 9. doi: 10.1016/j.heliyon.2023.e14497
Amadou T., Falconnier G. N., Mamoutou K., Georges S., Alassane B. A., François A., et al. (2022). Farmers’ Perception and adaptation strategies to climate change in central Mali. Weather Climate Soc. 14, 95–112. doi: 10.1175/WCAS-D-21-0003.1
Aman A., Nafogou M., N’Guessan Bi H. V., Kouadio Y. K., Kouadio H. B. (2019). Analysis and forecasting of the impact of climatic parameters on the yield of rain-fed rice cultivation in the office riz mopti in Mali. Atmos. Climate Sci. 09, 479–497. doi: 10.4236/acs.2019.93032
Amouzou K. A., Lamers J. P. A., Naab J. B., Borgemeister C., Vlek P. L. G., Becker M. (2019). Climate change impact on water- and nitrogen-use efficiencies and yields of maize and sorghum in the northern benin dry savanna, west africa. Field Crop Res. 235, 104–117. doi: 10.1016/j.fcr.2019.02.021
Anuradha N., Satyavathi T., Sankar M., Bharadwaj C., Pathy T. L. (2018). Association of agronomic traits and micronutrients in pearl millet. Int. J. Chem. Stud. 6, 181–184.
Araya A., Jha P. K., Zambreski Z., Faye A., Ciampitti I. A., Min D., et al. (2022). Evaluating crop management options for sorghum, pearl millet and peanut to minimize risk under the projected midcentury climate scenario for different locations in Senegal. Climate Risk Manage. 36. doi: 10.1016/j.crm.2022.100436
Egbebiyi T. S., Crespo O., Lennard C., Zaroug M., Nikulin G., Harris I., et al. (2020). Investigating the potential impact of 1.5, 2, and 3 ◦C global warming levels on crop suitability and planting season over west africa. PeerJ. doi: 10.7717/peerj.8851
FAO (1993). FESLM: An international framework for evaluating sustainable land management Table of contents World Soil Resources Report Food and Agriculture Organization of the United Nations 1993 A Discussion Paper. 73, 85.
FAO (2011). The state of the world’s land and water resources for food and agriculture (SOLAW) – Managing systems at risk. Rome and Earthscan, London: Organization of the United Nations.
FAOSTAT (2021). “World food and agriculture – statistical yearbook 2021,” in World food and agriculture – statistical yearbook 2021 (FAO). doi: 10.4060/cb4477en
Fatondji D., Bationo A., Tabo R., Jones J. W., Adamou A., Hassane O. (2012). “Water use and yield of millet under the zai system: Understanding the processes using simulation,” in In improving soil fertility recommendations in africa using the decision support system for agrotechnology transfer (DSSAT) (Netherlands: Springer), 77–100). doi: 10.1007/978-94-007-2960-5_6
Faye A., Akplo T. M., Stewart Z. P., Min D., Obour A. K., Assefa Y., et al. (2023). Increasing millet planting density with appropriate fertilizer to enhance productivity and system resilience in Senegal. Sustainabil. (Switzerland) 15. doi: 10.3390/su15054093
Godwin D. C., Singh U. (1998). “Nitrogen balance and crop response to nitrogen in upland and lowland cropping systems,” in Understanding options for agricultural production. Eds. Tsuji G. Y., Hoogenboom G., Thornton P. K. (Dordrecht / Boston / London: Kluwer Academic Publishers), 55–77. System Approaches for Sustainable Agricultural Development.
Graef F., Haigis J. (2001). Spatial and temporal rainfall variability in the Sahel and its effects on farmers’ management strategies. J. Arid Environ. 48, 221–231. doi: 10.1006/jare.2000.0747
Guan K., Sultan B., Biasutti M., Baron C., Lobell D. B. (2016) Assessing climate adaptation options and uncertainties for cereal 1 systems in West Africa. Available online at: http://www.elsevier.com/open-access/userlicense/1.0/.
Hess T. M., Stephens W., Maryah U. M. (1995). Rainfall trends in the Northeast Arid zone of Nigeria 1961–1990. Agric. For. Meteorol. 74:87-97. doi: 10.1016/0168-1923(94)02179-N
Hoogenboom G., Wilkens P. W., Thornton P. K., Jones J. W., Hunt L. A., Imamura D. T. (1999). “Decision support system for agrotechnology transfer v3.5,” In Hoogenboom G., Wilkens P. W., Tsuji G. Y. (Eds), DSSAT Version 3 (vol. 4). Honolulu, HI: University of Hawaii pp. 1–36.
Ibrahim B., Polcher J., Karambiri H., Rockel B. (2012). Characterization of the rainy season in Burkina Faso and its representation by regional climate models. Climate Dynam. 39, 1287–1302. doi: 10.1007/s00382-011-1276-x
IPCC (2014). Climate Change 2014: Synthesis Report. Contribution of Working Groups I, II and III to the Fifth Assessment Report of the Intergovernmental Panel on Climate Change [Core Writing Team, Pachauri R. K.Meyer L.A. (eds.)]. (Geneva, Switzerland: IPCC) 151.
Jamieson P. D., Porter J. R., Wilson D. R. (1991). A test of the computer simulation model ARC-WHEAT on the wheat crop grown in zealand. Field Crops Res. 27, 337–350.
Joseph J. E., Akinseye F. M., Worou O. N., Faye A., Konte O., Whitbread A. M., et al. (2023). Assessment of the relations between crop yield variability and the onset and intensity of the West African Monsoon. Agric. For. Meteorol. 333, 109431. doi: 10.1016/j.agrformet.2023.109431
Knutti R., Sedláček J. (2013). Robustness and uncertainties in the new CMIP5 climate model projections. Nat. Climate Change 3 (4), 369–373. doi: 10.1038/nclimate1716
Loague K., Green R. E. (1991). Statistics and graphical methods for evaluating solute transport model: overview and application. J. Contam. Hydrol. 7, 51–73.
Lobell D. B. (2014). Climate change adaptation in crop production: Beware of illusions. In Global Food Secur. 3, 72–76. doi: 10.1016/j.gfs.2014.05.002
Lobell D. B., Schlenker W. S., Costa-Roberts J. (2011). Climate trends and global crop production since 1980. Science 333, 616–620.
MacCarthy D. S., Sommer R., Vlek P. L. G. (2009). Modeling the impacts of contrasting nutrient and residue management practices on grain yield of sorghum (Sorghum bicolor (L.) Moench) in a semi-arid region of Ghana using APSIM. Field Crops Res. 113, 105–115. doi: 10.1016/j.fcr.2009.04.006
MacCarthy D. S., Vlek P. L. G., Bationo A., Tabo R., Fosu M. (2010). Modeling nutrient and water productivity of sorghum in smallholder farming systems in a semi-arid region of Ghana. Field Crops Res. 118, 251–258. doi: 10.1016/j.fcr.2010.06.005
Mohamed A. M. L., Jibrin J. M., Auwalu B. M., Garba M., Lawan B. A. (2023). Application of ceres-millet model of DSSAT for simulating millet varieties under different sowing windows in niger. J. Crop Improvement 37 (1), 41–59. doi: 10.1080/15427528.2022.2048764
Nelson R. A., Dimes J. P., Paningbatan E. P., Silburn D. M. (1998). 58(2) Pp, 129–146. doi: 10.1016/S0308-521X(98)00043-2
Ochieng I. O., Ranjan S., Seleiman M. F., Padhan S. R., Psiwa R., Sow S., et al. (2023). Increasing rainwater use efficiency, gross return, and grain protein of rain-fed maize under nitrate and urea nitrogen forms. Notulae Botanicae Horti Agrobotanici Cluj-Napoca 51. doi: 10.15835/nbha51313293
Pilloni R., Faye A., Kakkera A., Kholova J., Badji R., Faye C., et al. (2022). Higher sowing density of pearl millet increases productivity and water use efficiency in high evaporative demand seasons. Front. Plant Sci. 13. doi: 10.3389/fpls.2022.1035181
Ranjan S., Kumar S., Dutta S. K., Padhan S. R., Dayal P., Sow S., et al. (2023). Influence of 36 years of integrated nutrient management on soil carbon sequestration, environmental footprint and agronomic productivity of wheat under rice-wheat cropping system. Front. Environ. Sci. 11. doi: 10.3389/fenvs.2023.1222909
Rhoné B., Defrance D., Berthouly-Salazar C., et al. (2020). Pearl millet genomic vulnerability to climate change in west africa highlights the need for regional collaboration. Nat. Commun. 11, 5274. doi: 10.1038/s41467-020-19066-4
Rosenzweig C., Jones J. W., Hatfield J. L., Ruane A. C., Boote K. J., Thorburn P., et al. (2013). The Agricultural Model Intercomparison and Improvement Project (AgMIP): Protocols and pilot studies. Agric. For. Meteorol. 170, 166–182. doi: 10.1016/j.agrformet.2012.09.011
Roudier P., Muller B., D’Aquino P., Roncoli C., Soumaré M. A., Batté L., et al. (2014). The role of climate forecasts in smallholder agriculture: Lessons from participatory research in two communities in Senegal. Climate Risk Manage. 2, 42–55. doi: 10.1016/j.crm.2014.02.001
Ruane A. C., Rosenzweig C., Asseng S., Boote K. J., Elliott J., Ewert F., et al. (2017). An AgMIP framework for improved agricultural representation in integrated assessment models. Environ. Res. Lett. 12. doi: 10.1088/1748-9326/aa8da6
Saba A., Biasutti M., Gerrard M. B., Lobell D. B. (2013). Getting ahead of the curve: supporting adaptation to long-term climate change and short-term climate variability alike. Carbon Clim. Law Rev. 7, 3–24.
Satyavathi Tara C., Ambawat Supriya A., Vikas K., Srivastava Rakesh K. (2021). Pearl millet: A climate-resilient nutri-cereal for mitigating hidden hunger and provide nutritional security front. Plant Sci. (Sec. Plant Breeding) 12. doi: 10.3389/fpls.2021.659938
Singh P., Boote K. J., Kadiyala M. D. M., Nedumaran S., Gupta S. K., Srinivas K., et al. (2017). “An assessment of yield gains under climate change due to genetic modification of pearl millet,” in The science of the total environment.
Soler C. M. T., Maman N., Zhang X., Mason S. C., Hoogenboom G. (2008). Determining optimum planting dates for pearl millet for two contrasting environments using a modelling approach. J. Agric. Sci. 146 (4), 445–459. doi: 10.1017/S0021859607007617
Soler C. M. T., Sentelhas P. C., Hoogenboom G. (2007). Application of the CSM-CERES-Maize model for planting date evaluation and yield forecasting for maize grown off-season in a subtropical environment. Eur. J. Agron. 27 (2–4), 165–177. doi: 10.1016/j.eja.2007.03.002
Srivastava R. K., Bollam S., Pujarula V., Pusuluri M., Singh R. B., Potupureddi G., et al. (2020). Exploitation of heterosis in pearl millet: a review. Plants 9, 807. doi: 10.3390/plants9070807
Sultan B., Defrance D., Iizum T. (2019). Evidence of crop production losses in West Africa due to historical global warming in two crop models. Scientific Reports, 12834. doi: 10.1038/s41598-019-49167
Sultan B., Roudier P., Quirion P., Alhassane A., Muller B., Dingkuhn M., et al. (2013). Assessing climate change impacts on sorghum and millet yields in the Sudanian and Sahelian savannas of West Africa. Environ. Res. Lett. 8. doi: 10.1088/1748-9326/8/1/014040
Taylor K. E., Stouffer R. J., Meehl G. A. (2012). An overview of CMIP5 and the experiment design. Bull. Am. Meteorol. Soc. 93, 485–498. doi: 10.1175/BAMS-D-11-00094.1
Traore B., Van Wijk M. T., Descheemaeker K., Corbeels M., Rufino M. C., Giller K. E. (2014). Evaluation of climate adaptation options for Sudano-Sahelian cropping systems. Field Crops Res. 156, 63–75. doi: 10.1016/j.fcr.2013.10.014
Ullah A., Ahmad I., Ahmad A., Khaliq T., Saeed U., Habib-ur-Rahman M., et al. (2019). Assessing climate change impacts on pearl millet under arid and semi-arid environments using CSM-CERES-Millet model. Environ. Sci. pollut. Res. 26, 6745–6757. doi: 10.1007/s11356-018-3925-7
Uppal R. K., Wani S. P., Garg K. K., Alagarswamy G. (2015). Balanced nutrition increases yield of pearl millet under drought. Field Crops Res. 177, 86–97. doi: 10.1016/j.fcr.2015.03.006
Whitbread A. M., Robertson M. J., Carberry P. S., Dimes J. P. (2010). How farming systems simulation can aid the development of more sustainable smallholder farming systems in southern Africa. Eur. J. Agron. 32, 51–58. doi: 10.1016/j.eja.2009.05.004
Yadav O. P., Singh D. V., Vadez V., Gupta S. K., Rajpurohit B. S., Shekhawat P. S. (2017). Improving pearl millet for drought tolerance- Retrospect and prospects. Indian J. Genet. Plant Breed. 77, 464–474. doi: 10.5958/0975-6906.2017.00062.1
Keywords: adaptation strategies, climate change, management practices, pearl millet, potential impacts on yields
Citation: Akinseye FM, Zagre I, Faye A, Joseph JE, Worou ON and Whitbread AM (2024) Exploring adaptation strategies for smallholder farmers in dryland farming systems and impact on pearl millet production under climate change in West Africa. Front. Agron. 6:1428630. doi: 10.3389/fagro.2024.1428630
Received: 06 May 2024; Accepted: 12 June 2024;
Published: 08 July 2024.
Edited by:
Hanuman Singh Jatav, Sri Karan Narendra Agriculture University, IndiaReviewed by:
Abd Al Karim Jaafar, Damascus University, SyriaDibyajyoti Nath, Dr. Rajendra Prasad Central Agricultural University, India
Copyright © 2024 Akinseye, Zagre, Faye, Joseph, Worou and Whitbread. This is an open-access article distributed under the terms of the Creative Commons Attribution License (CC BY). The use, distribution or reproduction in other forums is permitted, provided the original author(s) and the copyright owner(s) are credited and that the original publication in this journal is cited, in accordance with accepted academic practice. No use, distribution or reproduction is permitted which does not comply with these terms.
*Correspondence: Folorunso M. Akinseye, b3Npb3llbWkyMDAzQGdtYWlsLmNvbQ==
†ORCID: Folorunso M. Akinseye, orcid.org/0000-0002-8677-6306 Anthony M. Whitbread, orcid.org/0000-0003-4840-7670