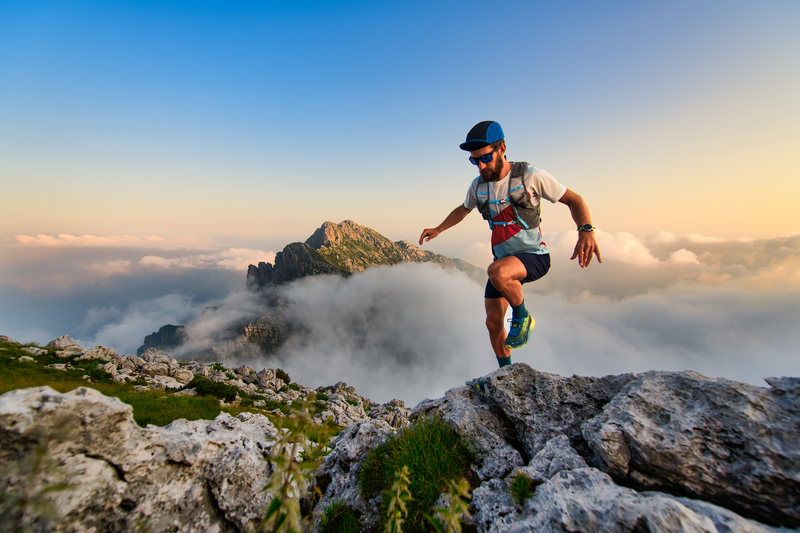
95% of researchers rate our articles as excellent or good
Learn more about the work of our research integrity team to safeguard the quality of each article we publish.
Find out more
ORIGINAL RESEARCH article
Front. Agron. , 07 July 2023
Sec. Agroecological Cropping Systems
Volume 5 - 2023 | https://doi.org/10.3389/fagro.2023.1219490
Introduction: Smallholder farmers in Sub-Saharan Africa (SSA) are increasingly producing soybean for food, feed, cash, and soil fertility improvement. Yet, the difference between the smallholder farmers’ yield and either the attainable in research fields or the potential from crop models is wide. Reasons for the yield gap include low to nonapplication of appropriate fertilizers and inoculants, late planting, low plant populations, recycling seeds, etc.
Methods: Here, we reviewed the literature on the yield gap and the technologies for narrowing it and modelled yields through the right sowing dates and suitable high-yielding varieties in APSIM.
Results and Discussion: Results highlighted that between 2010 and 2020 in SSA, soybean production increased; however, it was through an expansion in the cropped area rather than a yield increase per hectare. Also, the actual smallholder farmers’ yield was 3.8, 2.2, and 2.3 times lower than the attainable yield in Malawi, Zambia, and Mozambique, respectively. Through inoculants, soybean yield increased by 23.8%. Coupling this with either 40 kg ha−1 of P or 60 kg ha−1 of K boosted the yields by 89.1% and 26.0%, respectively. Overall, application of 21–30 kg ha-1 of P to soybean in SSA could increase yields by about 48.2%. Furthermore, sowing at the right time increased soybean yield by 300%. Although these technologies enhance soybean yields, they are not fully embraced by smallholder farmers. Hence, refining and bundling them in a digital advisory tool will enhance the availability of the correct information to smallholder farmers at the right time and improve soybean yields per unit area.
Soybean (Glycine max (L.) Merril) is a legume crop that improves soil fertility (Agomoh et al., 2021), human and animal nutrition (Ali et al., 2020), and income (Tufa et al., 2019). Its demand continuously rises globally, while its production in Southern Africa is low, contributing to 1% of the global output (Murithi et al., 2016). This is against a regional demand of two million metric tonnes (TechnoServe, 2011), leading to significant net importation. Commercial production majorly contributes to 1% of the global output and dominates in the region, with South Africa producing about 65% of total regional produce, followed by Zambia, Malawi, and Zimbabwe (TechnoServe, 2011). Contribution by smallholder farmers (owning farms that are less or equal to 2 ha (AGRA, 2017)) is limited as the crop is marginally profitable due to low yields, in both quality and quantity, resulting from poor agronomic management practices, poor or low input use, poor soil fertility, inappropriate variety choices specific to agroecological zones, and erratic rainfall patterns and amounts (Van Vugt et al., 2017). Further exacerbating these low yields is the focus of most governments in the region on staple food crops like maize (Grant et al., 2012; Jakobsen and Westengen, 2022), leaving minimal support for the soybean value chain.
Soybean production in Sub-Saharan Africa (SSA) is increasing (Figure 1), a fact confirmed also by Khojely et al. (2018), yet this is not due to a yield increase per hectare but to increased land for production, i.e., the hectareage under soybean. Indeed, an increase in land area for soybean will dominate its future production (Foyer et al., 2019). Our analysis here indicated that in the past 10 years, from 2010 to 2020, soybean production increase was parallel to the harvested area, while yield declined in Zambia and Malawi (Figure 1). This was also reported by Meyer et al. (2018). Improved soybean market prices are the drivers of increased production with an increase in land area (Nsomba et al., 2022) due to food and feed demand, especially in the poultry industry (Ncube et al., 2017); the need to diversify from maize (Mhango et al., 2012; Renwick et al., 2021), particularly to combat climate change (Siamabele, 2021) and improve smallholder farmers’ nutrition (Kolapo, 2011); improve soil fertility through biological nitrogen fixation (Ciampitti et al., 2021); break pest and disease cycles—pest control in integrated pest management such as fall armyworm and stemborer (Hailu et al., 2018; Matova et al., 2020) among others. Such land expansion for more production will gradually be unsustainable as the population grows; the land size per household reduces (Headey and Jayne, 2014; Muyanga and Jayne, 2014), and hence yield per unit area requires improvement (Mellor, 2014).
Figure 1 Production, harvested area, and yield of soybean in (A) Malawi, (B) Zambia, and (C) Mozambique for 10 years from 2010 to 2022. Source: FAO (2023).
In 2020, FAOSTAT reported soybean yields in Malawi, Zambia, and Mozambique as 0.95, 1.41, and 1.67 t ha−1, respectively (FAO, 2023). Comparing this with FAOSTAT Africa’s average production in the same year indicates that soybean yield from Malawi is lower by 39.8%, while those of Zambia and Mozambique are above by 6.1% and 25.9%, respectively. This is an indication that the reasons behind the yield gap are country-specific and could further narrow to agroecology, households, and individual farms. In comparison to the global average yield, the yield gap widens in all three countries; the average soybean yield in the world was 195.2%, 99.0%, and 67.8% higher than in Malawi, Zambia, and Mozambique, respectively. This shows that globally, some countries are narrowing their yield gap, inching closer to the potential yield, while most countries in SSA lag. Identifying these countries that are narrowing the yield gap and their strategies and adapting them to Sub-Saharan Africa conditions and site-specifics is important. A further comparison of the FAOSTAT soybean yield in 2020 with the attainable soybean yield under breeding trials at the International Institute of Tropical (IITA) in those three countries indicated a yield gap of 276.3%, 117.1%, and 125.0% in Malawi, Zambia, and Mozambique, respectively. This is an even wider yield gap than a comparison with either Africa’s or the global average yield. To accelerate increased production by smallholder farmers in the region and narrow the yield gap, research in development could play an essential role through the use of decision-support tools (Chivenge et al., 2022) and digital platforms. Ezui (2020) indicated a yield boost to smallholder farmers who used the AKILIMO App (Chernet and Pypers, 2020) in cassava production in Tanzania, Nigeria, and Rwanda. This shows the importance of digital platforms in enhancing crop yields. In soybean, such a digital tool does not exist for smallholder farmers in Sub-Saharan Africa. A tool that could address yield-limiting challenges in soybean and bottlenecks such as site-specific timing of planting, poor soil fertility/site-specific nutrient recommendation, suitable varieties, and disease/pest control (Van Vugt et al., 2017) and provide a well-coordinated and informed agronomy advisory and market services. Furthermore, poor access to and use of improved agronomic strategies that address production constraints is a major challenge among the region’s smallholder farmers. Approaches that take agronomy to scale and provide location- and context-specific decision support to farmers facing poor extension services could provide a viable route to enhancing soybean yields and production in the region. Moreover, improved soybean varieties and yield-enhancing agronomic practices developed and disseminated so far have had less adoption, and their scaling is required (Tufa et al., 2019). Therefore, the study: (i) assessed soybean production and yield gaps in Southern African countries—Malawi, Zambia, and Mozambique; (ii) determined site-specific yield-enhancing practices; and (iii) modeled through APSIM the potential soybean yields for Malawi, Zambia, and Mozambique under the right sowing date, and suitable variety. Later, these objectives will lead to the development of a soybean agronomic advisory tool that can assist smallholder farmers in getting the right information for suitable planting dates, crop variety choice, and site-specific fertilizer recommendations.
The literature search, selection criteria of the articles, and meta-analysis followed the steps described by Hansen et al. (2022). The search engines used were Web of Science, Google Scholar, Google, and Scopus, and the treatments, i.e., management practices are described in Table 1. The search strings included the following keywords: (1) soybean: TOPIC: (soybean OR soybean crop) AND TOPIC: (grain yield) AND TOPIC: (Africa OR Sub-Saharan Africa OR Southern Africa OR Malawi OR Zambia OR Mozambique); (2) for nitrogen application: TOPIC: (nitrogen fertilization OR nitrogen) AND TOPIC: (soybean grain yield OR yield) AND TOPIC: (Africa OR Sub-Saharan Africa OR Southern Africa OR Malawi OR Zambia OR Mozambique); (3) for phosphorus application: TOPIC: (phosphorus fertilization OR phosphorus) AND TOPIC: (soybean grain yield OR yield) AND TOPIC: (Africa OR Sub-Saharan Africa OR Southern Africa OR Malawi OR Zambia OR Mozambique); (4) for potassium: TOPIC: (potassium fertilization OR potassium) AND TOPIC: (soybean grain yield OR yield) AND TOPIC: (Africa OR Sub-Saharan Africa OR Southern Africa OR Malawi OR Zambia OR Mozambique); (5) for inoculation: TOPIC: (biological nitrogen fixation OR BNF OR nitrogen fixation OR fixed nitrogen OR nitrogen derived from atmosphere (ndfa)) AND TOPIC: (soybean grain yield OR yield) AND TOPIC: (Africa OR Sub-Saharan Africa OR Southern Africa OR Malawi OR Zambia OR Mozambique). From these searches, approximately 360 citations were obtained, and only 19 articles aligned with the objectives of this study included a control or a standard practice and provided the required data (Supplementary Table S1). Data from these articles were cleaned, curated, and subjected to different analyses.
Comparisons among the countries were done by finding the averages of production, harvested area, and yield; for Malawi and Zambia, it was from 2011 to 2020, whereas for Mozambique, it was from 2016 to 2020. Comparing the average yields between Malawi and Zambia was done from 2011 to 2020, while between Malawi and Mozambique, for both countries, it was conducted from 2016 to 2020.
A meta-analysis was conducted using Comprehensive Meta-Analysis V3 (Borenstein et al., 2003) on the effects of (1) P or K, (2) inoculants, (3) a combination of either P or K with inoculants, and (4) NPK and inoculants on soybean yield in Malawi, Zambia, Mozambique, and Sub-Saharan Africa. Analysis of the effect size of phosphorus application on soybean grain yield, heterogeneity, and publication bias tests were conducted. Furthermore, using SAS software (SAS, 2002), the means of the nitrogen, phosphorus, potassium, inoculant, and their combinations were compared using Fisher’s least significant difference (LSD) method at a 95% confidence level. These yield data were also subjected to analysis of variance using the Proc Glm statement.
The modeling component of the study was based on the breeding trials that were undertaken at Chipata in Zambia, Chitedze in Malawi, and Angonia in Mozambique. The countries were selected because they have a significant proportion of smallholder soybean-growing farmers. Chipata district is in Eastern Province, Zambia, at an altitude of 1,070 M above sea level (m.a.s.l) at 13° 70′ S and 32° 61′ E. The soils are predominantly clay-loam textured, which are moderately acidic with low phosphorus content. The area receives 850–1,050 mm of rainfall per season (Thierfelder et al., 2013). Angonia (14° 72′ S and 34° 37′ E) is located within the north-western district of Mozambique within Tete province and at an altitude of over 1,000 m.a.s.l. The region receives at least 850 mm of rainfall per season, and the mean annual temperature ranges between 15°C and 23°C. The predominant soils are Lixisols and Luvisols but are nutrient-depleted (Nyagumbo et al., 2016; Inacio et al., 2022). Chitedze agriculture research station (13° 98′ S and 33° 67′ E) is at an altitude of 1,145 m.a.s.l. The predominant soils are ferruginous Latosols, which are deep and freely draining. The pH ranges from 4.5 to 6.0. Chitedze has unimodal rainfall ranging from 700 to 1,100 mm per season. The mean annual temperature ranges from 18°C to 22°C (Ivy et al., 2017).
The APSIM Next-Generation model, known as APSIM NextGen (Holzworth et al., 2014), was calibrated based on the biophysical data from the breeding trials that were undertaken in Malawi, Mozambique, and Zambia. The APSIM NextGen was developed to improve execution speed, cross-platform development, model construction and visualization, and manager script flexibility (Holzworth et al., 2018). This improvement allows model developers to run larger simulations faster on multiple operating systems and simulate complex farming systems on temporal and spatial scales (Holzworth et al., 2018). It uses the APSIM Plant Modelling Framework (PMF) described by Brown et al. (2014), which allows the model developer to choose from a library of commonly used functions and algorithms for plant modeling. These are subsequently configured into a model description using the eXtensible Markup Language (XML). The APSIM Soybean model simulates crop development by pooling management and biophysical modules. The APSIM Soybean modules mimic crop growth upon input of certain data, for instance, crop management data, weather data, and soil data.
The APSIM Soybean model was calibrated based on the biophysical data collected from on-station IITA breeding trials. For effective and reliable simulations, the APSIM crop model needs to be calibrated for leaf area index (LAI), biomass, maturity, anthesis and emergence dates, and grain yield. In Sub-Saharan Africa, there are generally challenges due to inadequate data on soybean field research. The study, therefore, used one season of data but replicated across three sites in Malawi, Mozambique, and Zambia. The model was therefore calibrated based on one season’s data. In this study, most of the parameters needed were not collected, so the APSIM crop model was calibrated only for maturity, anthesis, and grain yield. In addition, in breeding trials, each line only appears once per year per location; it is therefore not possible to have data for at least three seasons for each variety. The model was also calibrated with daily rainfall, solar radiation, minimum temperature, and maximum temperature data which were downloaded from NASA (Supplementary Table S2). The soil data were extracted from the ISRIC global soil database (Table 2) (Batjes, 2022). The datasets were used since there was no weather or soil data of satisfactory quality.
Table 2 Biophysical and chemical characteristics of the soil used for model calibration and validation for Angonia, Mozambique; Chitedze, Malawi; and Good Nature, Zambia.
Crop model performance was evaluated using the root mean square error (RMSE) (Moriasi et al., 2007), which compares measured and observed values of the different parameters. In this study, all the RMSE values for all the parameters, varieties, and locations were less than 30%, which is acceptable (Table 3). Specifically, RMSE values between 20% and 30% are considered “fair,” 10% an 20% are considered “good,” and 0% and 10% are considered “excellent” (Moriasi et al., 2007). The soybean yields were also validated with key informants.
Table 3 Root mean square error (RMSE) values comparing observed and model-simulated parameters across the different varieties and locations in Chitedze, Malawi; Angonia, Mozambique; and Good Nature, Zambia (2018–2020).
The study coupled agronomic scenarios with the calibrated APSIM Soybean model. Soybean yield simulations were undertaken under different varieties, planting dates, and different phosphorus fertilizer application rates. The study evaluated long-duration varieties (grouped as taking > 120 days to physiological maturity) (Sc Serenade, Sc Sentinile, and Sc Safari) and short-duration varieties (days to physiological maturity is < 120) (MRI Dina). The attributes of these varieties are in Table 3. The study also evaluated the effect of different planting dates on yields from 1 November to 28 February, with a single planting every week in a similar fashion as done by Nyagumbo et al. (2017).
Soybean production and harvested area in Malawi, Zambia, and Mozambique have been increasing from 2011 to 2020 (Figure 1). From 2011 to 2020, production in Malawi increased by 137.9%. This was parallel to harvested area, which increased by 150.5% while the yield decreased by 5.0%. In Zambia, the production and the harvested area increased by 154.7% and 252.2%, respectively. Conversely, the yield declined by 27.7%. In Mozambique, even though the data were only available from 2016 to 2020, there was an increase in production, harvested area, and yield per area by 59.6%, 18.4%, and 34.8%, respectively. However, the production and the harvested area were lower than that of Zambia and Malawi; both were highest in Zambia. Considering the available data from Mozambique from 2016 to 2020, despite the average yield declining in Zambia (1,583.3 kg ha−1), it was more than that of Mozambique (1,394.2 kg ha−1) and Malawi (972.6 kg ha−1). Malawi produced less per area, 85.5% and 43.4% lower than Zambia and Mozambique, respectively (Figure 1).
The actual yield of soybean reported by FAOSTATS for 2020 (FAO, 2023) and Solidaridad in 2020 and the attainable yield from a variety of evaluation trials across the three countries (Malawi, Zambia, and Mozambique) by IITA in 2020 were compared. The attainable yield in Malawi (3,565.3 kg ha−1) was 2.6 and 3.8 times more than Solidaridad’s and FAOSTAT’’s (FAO, 2023) yields, respectively (Figure 2). In Zambia, the attainable yield (3,051.3 kg ha−1) was 2.5 and 2.2 times higher than Solidaridad’s and FAOSTAT’s (FAO, 2023) yields, respectively. Whereas in Mozambique, the attainable yield (3,750.7 kg ha−1) was 2.1 and 2.3 times more than the actual yields from Solidaridad’s and FAOSTAT’s (FAO, 2023), respectively (Figure 2). The potential yield calculated by the APSIM model was 3.2% and 19.6% higher than attainable in Malawi and Zambia, respectively. Interestingly, it was 20.0% lower than the attainable yield in Mozambique (Figure 2). Comparing it with the actual FAOSTAT yields in Malawi, Zambia, and Mozambique, it was 288.4%, 195.2%, and 46.7% higher, respectively, and with Solidaridad’s actual yields, it was 171.9%, 159.7%, and 42.4% more in Malawi, Zambia, and Mozambique, respectively (Figure 2).
Figure 2 Actual soybean grain yield according to FAOSTAT 2020 and Solidaridad in 2020, the attainable yield from soybean variety breeding trials at the International Institute Tropical of Agriculture (IITA) in 2020, and the APSIM model’s simulated potential yield.
The mean effect size of the study meta-analysis was 0.363 with a 95% confidence interval of 0.279 to 0.473, and the Z-value was −7.511 with p < 0.001. Both the Tau2 (an estimate of the standard deviation of the distribution of true effect sizes) and I2 (also known as Higgins I2, a statistical method to analyze heterogeneity and a measure for the proportion of observed variance that reflects real differences in effects (Borenstein et al., 2009)) were 0.332% and 0.000%, respectively (Figure 3).
Figure 3 Forest plot of the effect sizes for phosphorus application to soybean. Heterogeneity: TAU2 = 0.332, Q = 14.7%, df = 18 (p = 0.000), I2 = 0.0%. Test for overall effect: Z = −7.511 (p = 0.000).
Phosphorus significantly increased the grain yield of soybeans (Figure 4). Applying 22 kg ha−1 of phosphorus to soybean in Malawi increased yields by 52.4% (Figure 4A), while in Zambia, an application of 20 kg ha−1 led to a 6.6% yield increase (Figure 4B). In Mozambique, 40 kg ha−1 of P improved soybean yield by 42.6% (Figure 4C). Overall, in Sub-Saharan Africa, the application of 21–30 kg ha−1 of P raised soybean yield by 48.2% (Figure 4D). However, there was a negligible decline of 1% if more than 30 kg ha−1 of P.
Figure 4 Effect of phosphorus on soybean grain yield: (A) Malawi, (B) Zambia, (C) Mozambique, and (D) Sub-Saharan Africa. The percentages in (D) are of grain yield (kg ha−1) in comparison to 0–10 kg P ha−1. Multiple sources: Supplementary Table S1. Means with different letters are significantly different at p < 0.05; the error bars are ±SE (standard error).
Inoculating soybeans with rhizobia significantly improved their grain yield. By only inoculating soybean, the yield increased by 23.8% (Figure 5A), whereas, coupling this with either 40 kg ha−1 of P or 60 kg ha−1 of K boosted the yields by 89.1% (Figure 5B) and 26.0% (Figure 5C), respectively. Furthermore, combining the inoculant with N, P, and K improved yield by 32.7% compared to not inoculating but applying P and K (Figure 5D).
Figure 5 (A) Rhizobia inoculant and (B) rhizobia inoculant and phosphorus. (C) Rhizobia inoculant and potassium and (D) rhizobia inoculant and NPK influence on soybean grain yield in Sub-Sahara Africa. Multiple sources: Supplementary Table S1. Means with different letters are significantly different at p < 0.05; the error bars are ±SE (standard error).
Simulations of different soybean varieties and planting dates impacted yields (Figures 6, 7). Variety Sc Serenade had the highest yields regardless of the planting dates over the 30 years across different locations and countries, as well as for the selected season of 2020/2021. However, the pattern was more pronounced in Angonia (Figure 6A). This was similar to Chipata and Chitedze over the 30 years for the first half of the season, where from January till the end of the season there were no notable differences between all the four varieties (Figures 6, 8). The pattern was similar for the selected case study years, where there was a notable yield decline in the two locations after January (Figures 6, 8).
Figure 6 (A) Optimal soybean planting dates of the four varieties (short-duration varieties (Sc Serenade, Sc Sentinile, Sc Safari) and long-duration varieties (MRI Dina)) across 30 years for Angonia, Mozambique. (B) Optimal soybean planting dates of the four varieties (short-duration varieties (Sc Serenade, Sc Sentinile, Sc Safari) and long-duration varieties (MRI Dina) for the 2020/2021 season in Angonia, Mozambique.
Figure 7 (A) Optimal soybean planting dates of the four varieties (short-duration varieties (Sc Serenade, Sc Sentinile, Sc Safari) and long-duration varieties (MRI Dina) across 30 years for Chitedze, Malawi. (B) Optimal soybean planting dates of the for varieties (short-duration varieties (Sc Serenade, Sc Sentinile, Sc Safari) and long-duration varieties (MRI Dina) for the 2020/2021 season in Chitedze, Malawi.
Figure 8 (A) Optimal soybean planting dates of the four varieties (short-duration varieties (Sc Serenade, Sc Sentinile, Sc Safari) and long-duration varieties (MRI Dina) across 30 years for Good Nature, Zambia. (B) Optimal soybean planting dates of the four varieties (short-season varieties (Sc Serenade, Sc Sentinile, Sc Safari) and long-duration varieties (MRI Dina) for the 2020/2021 season in Good Nature, Zambia.
For Angonia, over the 30 years, the optimal planting window was remarkably wide from 12 December to 23 January, with Sc Serenade consistently being the highest-yielding variety (Figure 6A). During the case study year, 2020–2021, the optimal planting date range was 2–23 January across all cultivars. However, for Sc Serenade and Sc Sentinile, the optimal planting date was 9 January, with the highest yield of 3,600 and 2,600 kg ha−1, respectively. Also, the optimal planting date for Safari and MRI Dina was 16 January, achieving the highest yields of 2,600 had 2,500 kg ha−1, respectively (Figure 6B).
At Chipata in Zambia, over the 30 years, the optimal planting period was from 1 November to 31 December, with the variety SC Serenade having high yields of 3,600–4,200 kg ha−1. The yields of all four varieties began to decline, and the yield difference narrows after 2 January, with yields dropping to as low as 400 kg ha−1 when soybean was planted as late as 27 February (Figure 8A). The pattern was similar at Chitedze in Malawi (Figure 7A).
For the season 2020/2021 at Good Nature in Zambia, the optimal planting window was from 28 November to 2 January, which led to the highest yields of 3,990 to 4,050 kg ha−1. After 2 January, yields began to drop until the end of the season, reaching 1,200 kg ha−1 planted on 27 February (Figure 8B). The pattern was similar in Malawi, Chitedze (Figure 7B).
The soybean yield gap in three of the Southern African countries covering an area locally referred to as the Chinyanja Triangle is wide, with an attainable yield of above 3.5 t ha−1 in Malawi and Mozambique, against an actual of 0.9 and 1.7 t ha−1 in those countries, respectively. In Zambia, the attainable is above 3.1 t ha−1, against an actual of 1.4 t ha−1. Also, the world’s average soybean yield is 2.1 times that of Africa’s and 2.8, 1.9, and 1.7 times that of Malawi, Zambia, and Mozambique, respectively (FAO, 2023). This huge difference between the attainable and actual yield, or the actual yields from various agroecologies and regions—the yield gap (Sadras et al., 2015) is attributed to a myriad of factors. These include the use of poor-performing varieties, limited or no fertilizer application, especially phosphorus, and a lack of or poor usage of inoculants in soils without a previous history of soybean production (Khojely et al., 2018). Interestingly, these factors are continuously being addressed through research (Gbegbelegbe et al., 2019), from the release of high-yielding soybean varieties through breeding to proper crop, disease, pest, and soil fertility management, yet the yields of smallholder farmers are still low. The overall significant positive effect, as indicated by the meta-analysis, showed the importance of nutrient application in closing the yield gap in soybean. We revealed that inoculant application improves yields by 23.8%, application of between 20 and 30 kg P ha−1 increases yields by 48.2%, and a combination of P and K elevates the yields by 89.1%. These are also corroborated by some of the studies in Supplementary Table S1. Despite indications that these improved technologies increased soybean yield, most smallholder farmers are yet to adopt them (Tufa et al., 2019). However, there is hope, as early adopters lead the others in cementing that these technologies increase yields (Tufa et al., 2021). There are many reasons cited for low adoption, including lack or low ability to purchase inputs, lack or minimum skills and knowledge, variability in climate, poor access and availability of inputs, especially seeds, performance of the technology, poor soil fertility, gender and cultural practices (Odame et al., 2013), etc. Although poor extension services of the new improved technologies feature prominently too. Therefore, there is a need to improve extension services. In this era of mobile technology, e-extension is becoming popular, for example, the use of the Akilimo App by smallholder farmers producing cassava to enhance yields (Chernet and Pypers, 2020; Ezui, 2020). This can be developed for soybeans too to improve extension, farmers’ skills, management, and production. However, technologies required for yield improvement and sustainability must be gathered, refined, and developed into a one-stop application tool that can be easily used by soybean smallholder farmers in Sub-Saharan Africa, and crop modeling is an essential component of that.
Evaluation of the contribution of different farm management systems to the total yield output can potentially inform farmers on the choice and potential of strategies that can be used to close the yield gap. This also enhances decision-making and resource allocation. The actual average soybean yields are relatively low. The modeling outputs showed that the use of different planting dates notably changed soybean crop yield from the current yield of an average of 1 t ha−1 to as high as 3.3 t ha−1, which is 1.1 t ha−1 lower than the attainable yield. This shows the value of the right timing of planting. Previous studies in maize research showed at least a 5% yield decline for every week’s delay in planting (Shumba et al., 1992; Nyagumbo et al., 2017). This pattern was also realized in this study, where there was at least 6% soybean loss per week’s delay in sowing. Incorporation of other crop management practices such as suitable fertilizer quantity and timing, inoculant, and varieties could possibly further narrow the soybean yield gap. Factors such as fertilizer quantity and timing of planting have been identified as some of the major edaphic and socioeconomic yield gaps explaining factors in smallholder farming systems (Beza et al., 2017).
The use of different varieties significantly increased yields, thus providing further opportunities for narrowing the soybean yield gap. Most smallholder farmers use their own seed (sometimes called recycled seed), which reduces vigor, thus attaining yields of less than 1.5 t ha−1. From the model simulations, the use of certified short- and long-duration varieties will lead to yield increases of up to 300% and 250%, respectively. The use of different varieties to narrow the soybean yield gap is, however, dependent on the right time of planting and location-specific, as the study showed that some varieties consistently performed well in high-rainfall areas such as Angonia, Mozambique. Angonia receives about 800–2,000 mm per season (Nyagumbo et al., 2016; Inacio et al., 2022). In environments such as Chitedze and Chipata in Zambia and Malawi, respectively, the effect of genotype diminishes more as rainfall starts to decrease and planting is late in the season. For example, at Chitedze (Malawi), which receives 700–1,100 mm per season (Ivy et al., 2017), SC Seranade and MRI Dina, whose yields are as high as 3,600–4,200 and 3,500–3,800 kg ha−1, respectively, when planted early on 12 December, both drop to as low as 400 kg ha−1 when planted late on 27 February. Yield gap evaluation and yield decomposition, as in rice (Saito et al., 2017), wheat, and maize (Silva, 2017), are indeed important for soybean production in Sub-Sahara Africa to enhance targeted intervention and technology deployment.
Soybean production increased, although it was through an expansion in the cropped area rather than a yield increase per hectare. The average soybean yield in the world was 195.2%, 99.0%, and 67.8% higher than in Malawi, Zambia, and Mozambique, respectively. Also, the actual smallholder farmers’ yield was 3.8, 2.2, and 2.3 times lower than the attainable yield in Malawi, Zambia, and Mozambique, respectively - a wide yield gap. Some site-specific yield-enhancing practices, including the application of (1) between 20 and 30 kg P ha−1, (2) other nutrients like K and starter N, and (3) inoculants, improved soybean yields in Sub-Saharan Africa. Moreover, improved high-yielding soybean varieties preferred by most farmers in the region have been identified, and suitable management practices are being coupled with them to produce best-fit solutions. Furthermore, as shown by the APSIM model, suitable sowing dates have been identified for specific varieties in respective countries and agroecological zones (12 December to 23 January in Angonia and 1 November to 31 December in Chipata were the optimal sowing windows for SC Serenade). Altogether, proper management, soil fertility improvement strategies, and suitable sowing dates and varieties bundled in a digital tool that is easily available and usable by soybean smallholder farmers could enhance e-extension services and improve soybean yields per unit area.
The original contributions presented in the study are included in the article/Supplementary Materials, further inquiries can be directed to the corresponding author/s.
JO, SM, JM, PM, and IN conceived and designed the manuscript. JO, SM, JM, PM, and IN collected, synthesized, analyzed, and presented the data. JO, SM, JM, and PM drafted the manuscript, which was reviewed by AC, MC, GC, and SK-B. JO edited and revised the manuscript’s final version. All authors contributed to the article and approved the submitted version.
This study was funded under the OneCGIAR initiative, Excellence in Agronomy.
The authors are grateful to colleagues at Solidaridad, IITA, CIMMYT, and ICRAF.
The authors declare that the research was conducted in the absence of any commercial or financial relationships that could be construed as a potential conflict of interest.
All claims expressed in this article are solely those of the authors and do not necessarily represent those of their affiliated organizations, or those of the publisher, the editors and the reviewers. Any product that may be evaluated in this article, or claim that may be made by its manufacturer, is not guaranteed or endorsed by the publisher.
The Supplementary Material for this article can be found online at: https://www.frontiersin.org/articles/10.3389/fagro.2023.1219490/full#supplementary-material
Agomoh I. V., Drury C. F., Yang X., Phillips L. A., Reynolds W. D. (2021). Crop rotation enhances soybean yields and soil health indicators. Soil Sci. Soc Am. J. 85, 1185–1195. doi: 10.1002/saj2.20241
AGRA. (2017). Africa Agriculture status report: the business of smallholder agriculture in Sub-Saharan Africa, alliance for a green revolution in Africa (Nairobi, Kenya: Ecomedia).
Ali W., Ahmad M. M., Iftikhar F., Qureshi M., Ceyhan A. (2020). Nutritive potentials of soybean and its significance for humans health and animal production: a review. Eurasian J. Food Sci. Technol. 4, 41–53.
Batjes N. H. (2022). Basic principles for compiling a profile dataset for consideration in WoSIS (Wageningen: ISRIC - World Soil Information).
Beza E., Silva J. V., Kooistra L., Reidsma P. (2017). Review of yield gap explaining factors and opportunities for alternative data collection approaches. Eur. J. Agron. 82, 206–222. doi: 10.1016/j.eja.2016.06.016
Borenstein M., Hedges L., Higgins J., Rothstein H. (2003). Comprehensive meta-analysis: a computer program for research synthesis. J. Pers. Assess. 80, 321–322.
Borenstein M., Hedges L. V., Higgins J. P. T., Rothstein H. R. (2009). Introduction to meta-analysis. 1st ed (United Kingdom: John Wiley and Sons, Ltd).
Brown H. E., Huth N. I., Holzworth D. P., Teixeira E. I., Zyskowski R. F., Hargreaves J. N. G., et al. (2014). Plant modelling framework: software for building and running crop models on the APSIM platform. Environ. Model. Software 62, 385–398. doi: 10.1016/j.envsoft.2014.09.005
Chernet M., Pypers P. (2020) AKILIMO: smart phone app to deliver tailored agronomic advice for cassava growers. Available at: https://repo.mel.cgiar.org/handle/20.500.11766/67085.
Chivenge P., Zingore S., Ezui K. S., Njoroge S., Bunquin M. A., Dobermann A., et al. (2022). Progress in research on site-specific nutrient management for smallholder farmers in sub-Saharan Africa. F. Crop Res. 281, 108503. doi: 10.1016/j.fcr.2022.108503
Ciampitti I. A., De Borja F. A. R., Córdova S. C., Castellano M. J., Archontoulis S. V., Correndo A. A., et al. (2021). Revisiting biological nitrogen fixation dynamics in soybeans. Front. Plant Sci. 12. doi: 10.3389/fpls.2021.727021
Ezui K. S. (2020)New agronomic advice tool “ AKILIMO “ is showing high returns for cassava farmers. Available at: https://www.apni.net/2020/09/03/new-agronomic-advice-tool-akilimo-is-showing-high-returns-for-cassava-farmers/ (Accessed 1.18.23).
FAO. (2023). Crops and livestock products (Rome, Italy: FAOSTAT). Available at: https://www.fao.org/faostat/en/#data/QCL.
Foyer C. H., Kadambot H. M. S., Tai A. P. K., Anders S., Fodor N., Ferguson B. J., et al. (2019). Modelling predicts that soybean is poised to dominate crop production across Africa. Plant Cell Environ. 42, 373–385. doi: 10.1111/pce.13466
Gbegbelegbe S., Alene A., Kamara A., Wiebe K., Manyong V., Abdoulaye T., et al. (2019). Ex-ante evaluation of promising soybean innovations for sub-Saharan Africa. Food Energy Secur. 8, 1–16. doi: 10.1002/fes3.172
Grant W., Wolfaardt A., Louw A. (2012). Maize value chain in the SADC region (Gaborone, Botswana: USAID Southern Africa Trade Hub).
Hailu G., Niassy S., Zeyaur K. R., Ochatum N., Subramanian S. (2018). Maize–legume intercropping and push–pull for management of fall armyworm, stemborers, and striga in Uganda. Agron. J. 110, 2513–2522. doi: 10.2134/agronj2018.02.0110
Hansen C., Steinmetz H., Block J. (2022). How to conduct a meta-analysis in eight steps: a practical guide. Manage. Rev. Q. 72, 1–19. doi: 10.1007/s11301-021-00247-4
Headey D. D., Jayne T. S. (2014). Adaptation to land constraints: is Africa different? Food Policy 48, 18–33. doi: 10.1016/j.foodpol.2014.05.005
Holzworth D. P., Huth N. I., deVoil P. G., Zurcher E. J., Herrmann N. I., McLean G., et al. (2014). APSIM - evolution towards a new generation of agricultural systems simulation. Environ. Model. Software 62, 327–350. doi: 10.1016/j.envsoft.2014.07.009
Holzworth D., Huth N. I., Fainges J., Brown H., Zurcher E., Cichota R., et al. (2018). APSIM next generation: overcoming challenges in modernising a farming systems model. Environ. Model. Software 103, 43–51. doi: 10.1016/j.envsoft.2018.02.002
Inacio M. C., Didy O. O., Titki D. T., Idris I. A., Bily A. B. (2022). Redefining conservation agriculture through appropriate use of herbicides and fertilisers to improve crop production in Mozambique. Afr. J. Agric. Res. 18, 136–145. doi: 10.5897/ajar2021.15897
Ivy S. L., Patson C. N., Joyce N., Wilkson M., Christian T. (2017). Medium-term effects of conservation agriculture on soil quality. Afr. J. Agric. Res. 12, 2412–2420. doi: 10.5897/ajar2016.11092
Jakobsen J., Westengen O. T. (2022). The imperial maize assemblage: maize dialectics in Malawi and India. J. Peasant Stud. 49, 536–560. doi: 10.1080/03066150.2021.1890042
Khojely D. M., Ibrahim S. E., Sapey E., Han T. (2018). History, current status, and prospects of soybean production and research in sub-Saharan Africa. Crop J. 6, 226–235. doi: 10.1016/j.cj.2018.03.006
Kolapo A. L. (2011). Soybean: africa’s potential Cinderella food crop, in: ng, t.-b. (Ed.), soybean - biochemistry, chemistry and physiology. Intech Rijeka Croatia, 642.
Matova P. M., Kamutando C. N., NKutywayo D., Gutsa F., Labuschagne M. T. (2020). Fall-armyworm invasion , control practices and resistance breeding in Sub-Saharan Africa. Crop Sci. 60, 2951–2970. doi: 10.1002/csc2.20317
Mellor J. W. (2014). High rural population density Africa – what are the growth requirements and who participates? Food Policy 48, 66–75. doi: 10.1016/j.foodpol.2014.03.002
Meyer F., Traub N. L., Davids T., Chisanga B., Kachule R., Tostão E., et al. (2018). Modelling soybean markets in Eastern and southern Africa (Luxembourg: European Union). doi: 10.2760/20598
Mhango W. G., Snapp S. S., Phiri G. Y. K. (2012). Opportunities and constraints to legume diversification for sustainable maize production on smallholder farms in Malawi. Renew. Agric. Food Syst. 28, 234–244. doi: 10.1017/S1742170512000178
Moriasi D. N., Arnold J. G., Van Liew M. W., Bingner R. L., Harmel R. D., Veith T. L. (2007). Model evaluation guidelines for systematic quantification of accuracy in watershed simulations. Trans. ASABE 50, 885–900. doi: 10.13031/2013.23153
Murithi H. M., Beed F., Tukamuhabwa P., Thomma B. P. H. J., Joosten M. H. A. J. (2016). Soybean production in eastern and southern Africa and threat of yield loss due to soybean rust caused by phakopsora pachyrhizi. Plant Pathol. 65, 176–188. doi: 10.1111/ppa.12457
Muyanga M., Jayne T. S. (2014). Effects of rising rural population density on smallholder agriculture in Kenya. Food Policy 48, 98–113. doi: 10.1016/j.foodpol.2014.03.001
Ncube P., Roberts S., Zengeni T., Samboko P. C. (2017). Identifying growth opportunities in the southern African development community through regional value chains: the case of the animal feed to poultry value chain (Helsinki, Finland).
Ngonga E. M. (2020). Soybean phenotyping for rapid variety breeding in Zambia (York, United Kingdom: University of York).
Nsomba G., Roberts S., Tshabalala N., Manjengwa E. (2022). Assessing agriculture & food markets in Eastern and southern Africa: an agenda for regional competition enforcement (South Africa: Johannesburg).
Nyagumbo I., Mkuhlani S., Mupangwa W., Rodriguez D. (2017). Planting date and yield benefits from conservation agriculture practices across southern Africa. Agric. Syst. 150, 21–33. doi: 10.1016/j.agsy.2016.09.016
Nyagumbo I., Mkuhlani S., Pisa C., Kamalongo D., Dias D., Mekuria M. (2016). Maize yield effects of conservation agriculture based maize–legume cropping systems in contrasting agro-ecologies of Malawi and Mozambique. Nutr. Cycl. Agroecosystems 105, 275–290. doi: 10.1007/s10705-015-9733-2
Odame H., Kimenye L., Kabutha C., Alemu D., Oduori L. H. (2013). Why the low adoption of agricultural technologies in Eastern and central Africa? ASARECA (Association for strengthening agricultural research in Eastern and central Africa) (Entebbe, Uganda: Association for Strengthening Agricultural Research in Eastern and Central Africa (ASARECA)).
Renwick L. L. R., Deen W., Silva L., Gilbert M. E., Maxwell T., Bowles T. M., et al. (2021). Long-term crop rotation diversification enhances maize drought resistance through soil organic matter. Environ. Resear 16, 084067. doi: 10.1088/1748-9326/ac1468
Sadras V. O., Cassman K. G., Grassini P., Hall A. J., Bastiaanssen W. G. M., Laborte A. G., et al. (2015). Yield gap analysis of field crops: methods and case studies (Rome: FAO).
Saito K., van Oort P., Dieng I., Johnson J.-M., Niang A., Ahouanton K., et al. (2017). Yield gap analysis towards meeting future rice demand, in: sasaki, t. (Ed.), achieving sustainable cultivation of rice Vol. 2 (United Kingdom: Burleigh Dodds Science Publishing), 157–182. doi: 10.19103/as.2016.0003.26
Seed Co. (2023). Key agronomic attributes of seed Co soyabean varieties soyabean varieties. Available at: https://www.seedcogroup.com/zw/fieldcrops/key-agronomic-attributes-of-seed-co-soyabean-varieties/ (Accessed 6.12.23).
Shumba E. M., Waddington S. R., Rukuni M. (1992). Use of tine-tillage, with atrazine weed control, to permit earlier planting of maize by smallholder farmers in Zimbabwe. Exp. Agric. 28, 443–452. doi: 10.1017/S0014479700020159
Siamabele B. (2021). The significance of soybean production in the face of changing climates in Africa Vol. 7 (United Kingdom: Cogent Food Agric). doi: 10.1080/23311932.2021.1933745
Silva J. V. (2017). Using yield gap analysis to give sustainable intensification local meaning (Wageningen: Wageningen University).
TechnoServe (2011). Southern Africa regional soybean roadmap final report (Arlington: TechnoServe, Inc).
Thierfelder C., Mwila M., Rusinamhodzi L. (2013). Conservation agriculture in eastern and southern provinces of Zambia: long-term effects on soil quality and maize productivity. Soil Tillage Res. 126, 246–258. doi: 10.1016/j.still.2012.09.002
Tufa A. H., Alene A. D., Manda J., Akinwale M. G., Chikoye D., Feleke S., et al. (2019). The productivity and income effects of adoption of improved soybean varieties and agronomic practices in Malawi. World Dev. 124, 104631. doi: 10.1016/j.worlddev.2019.104631
Tufa A. H., Alene A. D., Manda J., Feleke S., Wossen T., Akinwale M. G., et al. (2021). The poverty impacts of improved soybean technologies in Malawi. Agrekon 60, 297–316. doi: 10.1080/03031853.2021.1939075
Keywords: decision support tools, digital tools, legumes, site-specific recommendations, Sub-Sahara Africa
Citation: Omondi JO, Mkuhlani S, Mugo J, Chibeba AM, Chiduwa MS, Chigeza G, Kyei-Boahen S, Masikati P and Nyagumbo I (2023) Closing the yield gap of soybean (Glycine max (L.) Merril) in Southern Africa: a case of Malawi, Zambia, and Mozambique. Front. Agron. 5:1219490. doi: 10.3389/fagro.2023.1219490
Received: 09 May 2023; Accepted: 19 June 2023;
Published: 07 July 2023.
Edited by:
Adornis Dakarai Nciizah, Agricultural Research Council of South Africa (ARC-SA), South AfricaReviewed by:
Cosmas Parwada, Midlands State University, ZimbabweCopyright © 2023 Omondi, Mkuhlani, Mugo, Chibeba, Chiduwa, Chigeza, Kyei-Boahen, Masikati and Nyagumbo. This is an open-access article distributed under the terms of the Creative Commons Attribution License (CC BY). The use, distribution or reproduction in other forums is permitted, provided the original author(s) and the copyright owner(s) are credited and that the original publication in this journal is cited, in accordance with accepted academic practice. No use, distribution or reproduction is permitted which does not comply with these terms.
*Correspondence: John Okoth Omondi, am8ub21vbmRpQGNnaWFyLm9yZw==
Disclaimer: All claims expressed in this article are solely those of the authors and do not necessarily represent those of their affiliated organizations, or those of the publisher, the editors and the reviewers. Any product that may be evaluated in this article or claim that may be made by its manufacturer is not guaranteed or endorsed by the publisher.
Research integrity at Frontiers
Learn more about the work of our research integrity team to safeguard the quality of each article we publish.