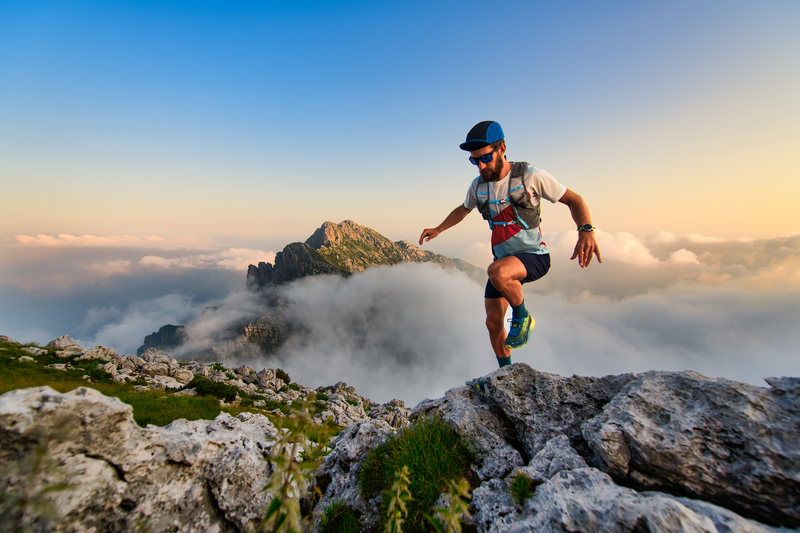
94% of researchers rate our articles as excellent or good
Learn more about the work of our research integrity team to safeguard the quality of each article we publish.
Find out more
ORIGINAL RESEARCH article
Front. Agron. , 13 May 2022
Sec. Disease Management
Volume 4 - 2022 | https://doi.org/10.3389/fagro.2022.881591
Pesticides are critical tools for golf course managers to maintain healthy and economically profitable golf course playing surfaces. However, the intensity and types of pesticides used on golf courses can be harmful to human and environmental health. Two separate studies were conducted at two locations in Wisconsin, USA between 2014 and 2020 to test the ability of reduced risk fungicide programs to control dollar spot (Clarireedia spp.) on golf course fairways and putting greens. Risk of the pesticide application programs was quantified in both studies using the active ingredient application rate, the Environmental Impact Quotient (EIQ), hazard quotient (HQ), and the Pesticide Risk Tool (PRT). The first study found that using the Smith-Kerns Dollar Spot Prediction Model to schedule fungicide applications did not reduce pesticide risk on its own, but that a pesticide program utilizing reduced risk products was just as effective in controlling dollar spot as a conventional program while reducing pesticide risk by ~50–80% depending on the pesticide risk indicator used. The second study established an average pesticide risk using HQ based on the pesticide records of 23 randomly selected Wisconsin golf courses. This statewide average was then used to test pesticide programs at 100, 75, 50, and 25% of the average risk for their efficacy in controlling dollar spot over a 4-year period. In the 4 years of the study, dollar spot severity of the 25% risk treatment was statistically indistinguishable from the other three programs. Taken together, these results indicate that pesticide risk can be significantly reduced on golf courses in the US Midwest without sacrificing dollar spot control.
There are ~25 million golfers in the United States who play on 16,752 golf courses (Royal Ancient (R&A), 2019; National Golf Federation (NGF), 2021). According to the US Bureau of Labor Statistics, golf is the second most popular participation sport in the US behind basketball (Woods, 2017). However, managing golf course turfgrass often requires repeated use of fungicides o provide acceptable control of damaging plant diseases. Without pesticide applications, golf course greens are often not able to survive in US climates (Rossi and Grant, 2009). Dollar spot (Clarireedia spp.) is the most common golf course turfgrass disease in temperate climates around the world, and on cool-season turfgrass in North America dollar spot is caused by C. jacksonii (Smiley et al., 2005; Salgado-Salazar et al., 2018; Sapkota et al., 2022). Optimal conditions for dollar spot symptom development include temperatures between 15 and 30°C and relative humidity in excess of 85% (Smiley et al., 2005). Left uncontrolled, tan-colored foci 2–5 cm in diameter can interrupt the playability and the aesthetics of the turfgrass playing surfaces (Smiley et al., 2005). The primary fungicides used to control dollar spot in the US include chlorothalonil, fluazinam, and multiple fungicides from the demethylation inhibitor (DMI) and the succinate dehydrogenase inhibitor (SDHI) classes of fungicides (Latin, 2021). In most temperate US climates fungicide applications targeting dollar spot begin in May and end in September or October (Smiley et al., 2005). Controlling dollar spot on golf course greens and fairways is generally in the best economic interest of those in the golf business to retain clientele. Indeed, more money is spent on fungicides to suppress dollar spot than any other turfgrass pest, and a single golf course can easily exceed $10,000 USD annually targeting dollar spot (Vargas, 2005; Golf Course Industry, 2015).
The high cost of fungicides to suppress dollar spot has led to the development of multiple dollar spot forecasting models to help optimize fungicide application timing (Sapkota et al., 2022). Models developed by Mills and Rothwell (1982); Hall (1984); Ryan et al. (2012) have been developed to predict dollar spot symptom development, however they have not been widely implemented by the golf course management industry due to poor performance. More recently, the Smith-Kerns Dollar Spot Model (SKM) was developed that uses a 5-day moving average of average daily air temperature and average daily relative humidity to produce a probability that dollar spot will develop on any given day (Smith et al., 2018). The authors were involved in the development of the SKM and found that a model probability of 20% acted as an acceptable threshold to time preventative fungicide applications for dollar spot control (Smith et al., 2018). The SKM helps reduce the number of overall fungicide applications targeting dollar spot by eliminating applications when there isn't a threat of symptom development, and it has become widely utilized by the golf course industry (Smith et al., 2018; Sapkota et al., 2022).
The repeated use of fungicides to control dollar spot has led to widespread documentation of C. jacksonii populations that are resistant to a variety of fungicide classes including the demethylation inhibitor (DMI), dicarboximide, succinate dehydrogenase inhibitor (SDHI), and benzimidazole fungicide classes (Detweiler et al., 1983; Golembiewski et al., 1995; Burpee, 1997; Sang et al., 2015; Sapkota et al., 2022). In addition, the frequent application of pesticides to prevent turfgrass diseases can also present a variety of possible health risks to golf course workers, the golfing public, and the environment. Previous research has indicated that golf course pesticide use had quantifiable human health effects on golf course superintendents (Kross et al., 1996; Knopper and Lean, 2010), but that the chronic inhalation and dermal risk of pesticides to golfers was relatively low (Murphy and Haith, 2007; Wong and Haith, 2014). Ecologically, Haith and Rossi (2003) found that runoff of chlorothalonil, iprodione, and pentachloronitrobenzene (PCNB) from fairways may routinely affect aquatic health in surrounding water bodies. Metcalfe et al. (2008) found concentrations of PCNB in golf course streams, a byproduct of the fungicide quintozene, at concentrations toxic to developing fish. Multiple studies have detected high levels of arsenic in soils and groundwater of golf courses from the use of arsenic containing pesticides (Cai et al., 2002; Feng et al., 2005; Pichler et al., 2008). In addition, Gunstone et al. (2021) reviewed 400 studies of soil invertebrates and 2,800 tested parameters (the effect of a specific pesticide on a specific soil invertebrate) and found that 70.5% of tested parameters showed negative effects, 1.4% showed positive effects, and 28.1% showed no effect. Soil invertebrates, critical to nutrient cycling, carbon sequestration, and soil morphology are often overlooked in pesticide risk assessment.
Reducing the risk of injury associated with pesticide use on golf courses has the potential to benefit golf course superintendents, local environmental health, and the golfing public. Bekken et al. (2021) found that pesticide risk on golf courses in the northern US was primarily caused by fungicide use, and the majority of golf course fungicide use targets dollar spot (Vargas, 2005). Pesticide applications to fairways (average size of 11.4 ha), though more infrequent than applications to greens (average area of 1.3 ha), had significantly higher total pesticide risk because their larger area increased potential non-target pesticide exposure (Bekken et al., 2021). Thus, reducing the risk of fairway dollar spot applications is one of the most effective means to reduce overall golf course pesticide risk.
The US Environmental Protection Agency (EPA) Risk Assessment Program reviews the ecological and human health risks of all new and existing pesticides before they can be registered or re-registered for use in the US (US EPA, 2021). The goal of the program is to ensure that the use of pesticides poses no “unreasonable risks” to humans, plants, wildlife, and the environment. However, EPA risk assessments only consider individual pesticide products and therefore do not quantify the risk of pest management programs that commonly contain many different pesticides. One method to estimate pesticide risk of pesticide application programs is to sum the annual weight of pesticide active ingredient applied (Kerns and Tredway, 2013). However, such metrics consider only pesticide exposure and fail to consider differences in toxicity between pesticides (Barnard et al., 1997). To account for this, many pesticide risk indicators have been developed to account for both exposure and toxicity for use in agricultural situations (Greitens and Day, 2006; Oliver et al., 2016; Kniss, 2017; Schulz et al., 2021). Pesticide risk indicators have the added benefit of being able to quickly assess risk of a high number of sites regardless of geographic location at a lower cost than field-based sampling and ecological risk assessment methods. Pesticide risk indicators that consider both toxicity and exposure and that are utilized in this study include: Environmental Impact Quotient (EIQ) (Kovach et al., 1992), hazard quotient (HQ) (Kniss, 2017), and the Pesticide Risk Tool (PRT) (IPM Institute of North America, 2021). Indicators of pesticide risk are not widely used in the golf course management industry despite the heavy reliance on chemical fungicides to provide acceptable conditions.
The objective of this study was to test the ability of the SKM and reduced risk pesticide products to both reduce golf course pesticide risk and control dollar spot infection on fairways and greens in comparison to conventional pesticide programs. We define reduced risk pesticide products as those either labeled as reduced risk by the Reduced Risk Program (EPA Reduced Risk Program, 2021) or products with similar chemistries and toxicity profiles as fungicides included in the Reduced Risk Program. In addition, the study benchmarks average pesticide risk for 23 golf courses in Wisconsin, USA, and tests application programs of lower risk for their efficacy in controlling dollar spot.
The first method utilized, though technically not a measure of pesticide risk, was to calculate the active ingredient (AI) application rate (kg AI ha−1) of each treatment. This method only considers the exposure level of the pesticide and does not consider the second component of pesticide risk, toxicity. However, many previous studies use this method as an indicator of general environmental pesticide risk and thus we include it here, so our results are comparable to previous work, such as Kearns and Prior (2013).
The second risk indicator used was the area normalized hazard quotient (HQ), calculated consistent with the methods of Bekken et al. (2021) (Equation 1).
Where n equals the total number of pesticide applications each year; W is the weight of pesticide product (mg); A is the area of application (ha); and Rfdp is the reference dose associated with the pesticide product (mg pesticide product/kg rat). Rat (Rattus spp) acute oral median lethal dose to control 50% of the control group (LD50) was used to approximate the acute mammalian toxicity and was chosen as the reference dose for the hazard quotient formula. HQ was used because it is a strictly quantitative framework that, as applied in this study, only measures the pesticide risk to mammals. Mammals were chosen as the endpoint for the HQ model because mammalian toxicity has important implications for human health.
Next, the Field Use Environmental Impact Quotient (FUEIQ) was calculated consistent with the methods of Kovach et al. (1992) (Equation 2). The model uses a qualitative framework to weigh risk for eleven environmental endpoints and subjectively combines these risk factors into a single score, meant to be representative of overall environmental risk. For a detailed discussion of the advantages and disadvantages of the EIQ model see Kniss (2017) and Bekken et al. (2021). Base EIQ values were obtained from the EIQ Calculator website (https://nysipm.cornell.edu/eiq/calculator-field-use-eiq/).
The fourth method used was the Pesticide Risk Tool (PRT). Similar to EIQ, the PRT estimates pesticide risk to a variety of environmental endpoints and weighs them to produce a score of overall environmental pesticide risk. The PRT estimates pesticide risk across 13 different categories: Avian Acute, Avian Reproductive, Small Mammal Acute, Earthworm, Fish Chronic, Aquatic Algae, Aquatic Invertebrate, Inhalation, Worker Dermal, Dermal Cancer, Pollinator in Bloom, Pollinator No Bloom, and Pollinator off Crop. Similar to EIQ and hazard quotient, the PRT estimates pesticide risk based on the two elements of risk: toxicity and exposure. However, the PRT incorporates results from in-field toxicity and exposure studies wherever possible with the goal of making risk estimates more realistic to in-field conditions. Two pesticide risk indicators from the PRT were utilized in this study: (1) high risks per application, and (2) risk points per application. The high risks per application indicator is a summation of the number of high risks across the 13 categories included in the PRT for each application made. High risks are defined by the IPM Institute as those with a risk probability <50%. This indicator has the advantage of flagging high risk applications but the disadvantage that it misses moderate risks. The risk points per application metric captures both high, moderate, and low risk by converting the different numerical scales across 13 categories to a common 0 Bekken et al. (2021) 10 scale. The number of risk points in each category for an application is then averaged across all categories to produce the risk points per application metric.
In summary, our study utilizes four models to estimate of pesticide risk, three of which are meant to represent pesticide risk to the environment generally, and the fourth which is meant to estimate pesticide risk to mammals specifically. Because we utilize two pesticide risk indicators from the PRT, there are a total of five pesticide risk indicators used in the study, which are derived from four pesticide risk models.
Two consecutive field experiments were conducted to determine the efficacy of lower risk fungicide programs to suppress dollar spot development. The first experiment ran for three growing seasons (2014 through 2016) and is referred to as the Smith-Kerns Model Reduced Risk Study (SKM Reduced Risk). The second experiment ran for four growing seasons (2017 through 2020) and is referred to as the “State Risk Comparison Study.”
The SKM Reduced Risk field experiment was conducted at the O. J. Noer Turfgrass Research and Education Facility on two separate plots (14th and 18th holes) on the adjacent University Ridge Golf Course in Verona, Wisconsin, USA. Each experiment was conducted on “Penncross” creeping bentgrass maintained as either a golf course fairway or golf course putting green (Table 1). Individual plot area measured 1.8 by 3.0 m and the four treatments were arranged in a randomized complete block design with four replications. The study was conducted in 2014, 2015, and 2016 on approximately the same area in each year. Pesticide applications were made using a CO2-pressurized boom sprayer (R&D Sprayers, Opelousas, Louisiana) equipped with XR Teejet AI8004 nozzles pressurized to 275.8 kPa. All treatments were agitated by hand and applied in the equivalent of 814 L water ha−1.
Table 1. Mowing height during each year at both the O. J. Noer Turfgrass Research Facility and University Ridge Golf course for the Smith-Kerns Model (SKM) reduced risk study and the state risk comparison study.
The State Risk Comparison field experiment was conducted at the O. J. Noer Turfgrass Research Facility on ‘Focus' creeping bentgrass maintained as a golf course fairway. Individual plot area measured 1.8 by 3.0 m and the four treatments were arranged in a randomized complete block design with four replications. The study was conducted in 2017, 2018, 2019, and 2020 on approximately the same location each year. Pesticide applications were made using the same method as described for the SKM Reduced Risk study.
The two goals of this study were to: (1) determine if the utilization of the Smith-Kerns Dollar Spot Prediction Model (SKM) in timing fungicide applications contributed to reducing pesticide risk and (2) assess whether reduced risk pesticides were effective in controlling dollar spot on putting green and fairway height turf. In this field experiment, three fungicide programs were tested in addition to a non-treated control. The first fungicide program was titled the Conventional Program (CP) and was based on the fungicide program of a public golf course in southern Wisconsin, USA where pesticides were applied on a preventative calendar schedule. The second fungicide program was titled the Conventional Program Smith-Kerns Model (CP-SKM) and used similar fungicides as the CP but based the application timing on the SKM. An additional application of chlorothalonil was added to this treatment compared to the CP because of concerns with overall efficacy of the program and development of fungicide resistant organisms. The third fungicide program, titled Reduced Risk Smith-Kerns Model (RR-SKM), also based application timing on the SKM but only used fungicides labeled as reduced risk by the Reduced Risk Program (EPA Reduced Risk Program, 2021) or products with similar chemistries and toxicity profiles as fungicides included in the Reduced Risk Program. For the CP-SKM and RR-SKM treatments the SKM threshold was set at 20% based on separate research the authors had conducted with the SKM in 2012 through 2014 (Smith et al., 2018). Once a pesticide was applied, the recommended reapplication interval from that pesticide label was observed, and thereafter the next application of fungicide(s) was applied when the model again exceeded 20%. The products used in each treatment, the associated pesticide risk scores, and the dates of application can be found in Table 2.
Table 2. SKM reduced risk study treatment program, date, trade name, active ingredient, rate of application, and pesticide risk as quantified by active ingredient application rate, HQ, hazard quotient; EIQ, Environmental Impact Quotient; PRT, Pesticide Risk Tool for each product applied in the study.
The two goals of this study were to: (1) determine the average fungicide risk on a Wisconsin golf course and (2) assess whether fungicide programs of decreasing risk compared to the statewide average would provide adequate dollar spot suppression. To calculate the statewide average, 50 golf courses in Wisconsin, USA were selected at random out of the nearly 500 golf courses in the state. The random selection occurred by assigning a number to every golf course in the Wisconsin Golf Course Superintendents Association directory and using a random number generator to select 50 numbers. Selected golf courses were contacted via email and asked to share their pesticide application records over a 2-year period within a three-year window, from 2014 through 2016. Twenty-three golf courses responded to the request for pesticide records and provided a broad representation of golf courses in the state, both geographically and economically. Pesticide risk for each golf course was quantified using the hazard quotient (HQ) model (Equation 1).
Our focus was on reducing the risk of fairway dollar spot management programs, so the risk scores from the fairway pesticide programs of the 23 golf courses were averaged to obtain an approximate statewide average HQ pesticide risk score for a Wisconsin golf course fairway. The fungicide program submitted by one of the 23 golf courses referenced above that had an HQ score closest to the statewide average was chosen as the baseline for use in this study and is referred to as the 100% state average (SA) risk program. Additional fungicide programs with an HQ value that equaled 75, 50, and 25% of the statewide average were developed by substituting individual products with lower HQ scores and are referred to as the 75%-SA, 50%-SA, and 25%-SA programs. The products used in each treatment, the associated pesticide risk scores, and the dates of application can be found in Table 3.
Table 3. State risk comparison study treatment program, date, trade name, active ingredient, rate of application, and pesticide risk as quantified by active ingredient application rate, HQ, hazard quotient; EIQ, Environmental Impact Quotient; PRT, Pesticide Risk Tool for each product applied in the state risk comparison study.
Data collection was the same for both studies. Dollar spot severity was assessed by counting dollar spot infection centers approximately every 14 days from May through October depending on how long the dollar spot epidemic persisted in each year. Turfgrass quality was also rated visually every 14 days using the National Turfgrass Evaluation Program (NTEP) 1 to 9 rating scale, where 1 is dead/necrotic, 6 is minimally acceptable, and 9 is excellent (Lee et al., 2011). Turfgrass quality ratings included combinations of disease, color, density, and uniformity and were always conducted by the same person to avoid interpersonal variation. In 2014 and 2015, normalized difference vegetation index (NDVI) measurements were collected on a 14-day interval to estimate turfgrass color and was measured using a FieldScout TCM 500 NDVI Turf Color Meter (Spectrum Technologies Inc., Plainfield, Illinois). Beginning in 2016, chlorophyll index was used to estimate turfgrass color and was assessed using a FieldScout CM 1000 Chlorophyll Meter (Spectrum Technologies Inc., Plainfield, Illinois). The cost of each fungicide treatment was estimated from cost information obtained from a pesticide distributor in the Midwest US and is broadly representative of prices in the region.
The area under the disease progress curve (AUDPC) was calculated using the trapezoidal method (Campbell and Madden, 1990) and standardized based on the length of the epidemic in each year and at each location. For the SKM Reduced Risk study the length of the epidemic at both University Ridge and the O. J. Noer Research Facility were 143 days in 2014 and 158 days in 2015. In 2016 the length of the epidemic was 133 days at University Ridge and 154 days at O. J. Noer. For the State Average Comparison study conducted at the O. J. Noer the length of the epidemic was 122 days in 2017, 116 days in 2018, 119 days in 2019, and 113 days in 2020. AUDPC data were subjected to analysis of variance (ANOVA) and means separated using Fishers least significant difference (P ≤ 0.05) using the MIXED procedure in SAS (Version 9.4, Cary, North Carolina). Dollar spot severity on individual dates was analyzed by repeated measures analysis using the GLIMMX procedure in SAS. The turf color (NDVI and chlorophyll meter) data were also subjected to analysis of variance (ANOVA) and means separated using Fishers least significant difference (P ≤ 0.05) in SAS. Turfgrass quality results were analyzed using the Kruskal Bekken et al. (2021) Wallis test via the “kruskal” function of the agricolae package in R (v.3.6.2), and the kruskal function also separated out treatment differences in turfgrass quality using a Fishers least significant difference test (P ≤ 0.05).
Dollar spot AUDPC values were significantly different across sites (P < 0.001) and years (P < 0.001) (Supplementary Tables S1–S4). All treatments reduced disease compared to the non-treated control in each of the 9 site-years (Table 4). The CP-SKM treatment had the same or lower disease compared to the CP in all site-years except for University Ridge Hole #18 in 2015 and the OJ Noer in 2014. The RR-SKM treatment had the same or lower disease compared to the non-treated control in all nine site-years (Table 4). Supplementary Table S5 indicates dollar spot severity at each rating date at each study location across the 3 years of the study.
Table 4. Area under the disease progress curve (AUDPC), turfgrass color, and turfgrass quality (TQ) for the Smith-Kerns model reduced risk study conducted at University Ridge Golf Course and the O. J. Noer Turfgrass Research Facility in Verona, Wisconsin, USA between 2014 and 2016.
According to all five pesticide risk indicators utilized in this study, the CP-SKM had the highest pesticide risk, the CP had similar but slightly lower risk, and the RR-SKM had far lower risk than the other two programs (Figure 1). When comparing RR-SKM to CP, pesticide risk as quantified by the AI application rate, HQ and FUEIQ were reduced by 76, 72, and 81%, respectively. When comparing the RR-SKM to CP-SKM pesticide risk as quantified by the AI application rate, HQ and FUEIQ were reduced by 77, 78, and 83%, respectively. The PRT also estimated large reductions in risk for RR-SKM in comparison to CP and CP-SKM. The CP had 0.64 high risks per application, the CP-SKM had 0.78 high risks per application, while the RR-SKM had zero high risks per application. Pesticide risk as quantified by the PRT Risk Points per application was reduced by 51% between the RR-SKM and the CP program, and 56% between the RR-SKM and the CP-SKM program. Thus, according to the five different indicators utilized in this study to quantify pesticide risk, the RR-SKM program reduced pesticide risk by at least 50% (PRT Risk Points) and by up to 100% (PRT High Risk). The other three risk indicators predicted risk reductions within this range.
Figure 1. Pesticide risk and price of each treatment program in the Smith Kerns Model Reduced Risk Study according to the five pesticide risk indices used to quantify pesticide risk: HQ, Hazard Quotient; AI App Rate, Active Ingredient Application Rate; FUEIQ, Field Use Environmental Impact Quotient; PRT-Risk Points per App, Pesticide Risk Tool- Risk Points per Application; PRT-High Risks per App, Pesticide Risk Tool- High Risks per Application. The treatment programs were the control, Conventional Program (CP), Conventional Program Smith-Kerns Model (CP-SKM), and Reduced Risk Smith-Kerns Model (RR-SKM).
While the RR-SKM program had significantly lower pesticide risk than the other two pesticide application programs in the study, the estimated cost of the program was $5,049 USD per hectare. This was more than two times greater than the cost of the CP-SKM program ($2,446 USD per hectare) and 1.6 times the cost of CP program ($3,172 USD per hectare).
Across all years and locations of the study, turf color, as quantified by NDVI (2014 and 2015) and CI (2016), was significantly higher for the treatment programs (CP, CP-SKM, RR-SKM) compared to the control (Table 4). However, which treatment program had the highest turf color value varied by year and location. The turf quality of the treatment programs across all years and locations were significantly higher than in the control. No differences in turf quality were observed among the three treatment programs in 2014. In 2015 turf quality of CP-SKM was lower than CP and RR-SKM at all study locations. In 2016, RR-SKM had the highest turf quality at University Ridge hole 14 and O. J. Noer and was tied for the highest turf quality at University Ridge hole 7 and 18.
Dollar spot AUDPC values were significantly different across years (P = 0.0029) (Supplementary Table S6). Each of the four fungicide programs suppressed dollar spot relative to the non-treated control in each year of the study (Table 5). With one exception, there was no significant difference in dollar spot AUDPC values among the fungicide programs in 3 of 4 years despite the programs varying widely in their pesticide risk profile. Only in 2018 was there a treatment difference in dollar spot AUDPC, during which the 100%-SA treatment actually had more dollar spot relative to the 75%-SA, 50%-SA, and 25%-SA treatments. Supplementary Table S7 indicates dollar spot severity at each rating date across the 4 years of the study.
Table 5. Area under the disease progress curve (AUDPC), turfgrass color, and turfgrass quality (TQ) for the State Risk Comparison Study conducted at the O. J. Noer Turfgrass Research Facility in Verona, Wisconsin USA between 2017 and 2020.
The HQ model was used to establish the four treatment programs of the State Risk Comparison Study (100%-SA, 75%-SA, 50%-SA, 25%-SA). However, the three other methods used to estimate risk, the AI App Rate method, FUEIQ, and PRT (Risk Points per Application, High Risks per Application) estimated that the 50%-SA treatment had the highest risk of the four treatments (Figure 2). This does not mean that the HQ model is faulty or incorrect, but rather is an indication that the various models are measuring pesticide risk of different environmental endpoints.
Figure 2. Pesticide risk and price of each treatment program in the State Risk Comparison Study according to the five pesticide risk indices used to quantify pesticide risk: HQ, Hazard Quotient; AI App Rate, Active Ingredient Application Rate; FUEIQ, Field Use Environmental Impact Quotient; PRT-Risk Points per App, Pesticide Risk Tool- Risk Points per Application; PRT-High Risks per App, Pesticide Risk Tool- High Risks per Application. The study included a control and four programs that were 100%, 75%, 50%, and 25% the pesticide risk of the statewide average (SA) as quantified by hazard quotient (HQ).
Turf color as quantified by chlorophyll index (CI) was significantly different between the control and the four treatment programs in 3 of the 4 years of the study. However, the four treatment programs were statistically indistinguishable in color across all 4 years of the study (Table 5). Except for the 100%-SA treatment in 2018, turf quality of the control was significantly lower than the four treatment groups. In 2019, all four treatment groups had significantly higher turf quality than the control but were not statistically distinguishable from one another. In the remaining 3 years of the study, however, the four treatment groups were statistically distinguishable. Surprisingly of the four treatment groups, the 100%-SA treatment had the lowest turf quality. The 50%-SA and 25%-SA had the highest turf quality in all years except 2019 (Table 5).
Both the SKM Reduced Risk and State Risk Comparison studies indicate that pesticide risk, as measured by a wide variety of pesticide risk indicators, associated with dollar spot management of cool season turfgrass can be reduced by more than 50% while maintaining high levels of dollar spot control. Reductions in pesticide risk in both studies were achieved through both changes to product selection and by applying pesticides less frequently, but product selection generally had a greater effect on reducing pesticide risk. Bekken et al. (2021) also found product selection to be an important determinant of pesticide risk on golf courses in the northern US. One of the central goals of the SKM Reduced Risk study was to determine if the utilization of the Smith-Kerns Model (SKM) would lead to a reduction in pesticide risk. Using the SKM model in the CP-SKM treatment to time fungicide applications led to two fewer fungicides applications compared to the CP, however overall risk was higher than the CP. The CP-SKM treatment, despite two fewer fungicide applications, had higher risk because the amount of chlorothalonil applied in this treatment compared to the CP was greater. Chlorothalonil is often repeatedly applied during the summer months in Midwestern climates to manage a broad range of fungal diseases and to suppress fungicide-resistant dollar spot populations. The CP-SKM treatment had three applications of chlorothalonil compared to two applications in the CP to help improve overall efficacy and resistance management of the CP-SKM treatment. These results make clear, however, that product selection plays a larger role in risk reduction than the overall number of fungicide applications because of the large differences in risk between products.
Another central goal of both studies was to determine the ability of reduced risk pesticide programs to control dollar spot. The lowest risk program in both studies, the reduced risk treatment and the 25%-SA treatment, actually had the lowest mean AUDPC dollar spot values across all years and study locations (Tables 4, 5). In addition, these two programs had turf quality and color values that were statistically indistinguishable from the conventional programs. Thus, a reduced risk program was just as effective or more effective in controlling dollar spot and yielding a high-quality turf surface than conventional programs. Altering product selection in favor of lower risk products, pesticide risk for the control of dollar spot on golf course fairways can be reduced sharply without compromising dollar spot control.
The fungicide chlorothalonil had the single biggest impact on risk in both studies. This was evident when comparing the CP and CP-SKM programs in the SKM Reduced Risk study but was also evident in the State Risk Comparison study. Chlorothalonil has long been used in golf course management because of its favorable characteristics as a low-cost, broad-spectrum fungicide that is not susceptible to issues of fungal resistance (Latin, 2021). Despite golf courses only covering less than a half percent the land area as US agriculture, US golf courses account for 10% of total chlorothalonil use (Van Scoy and Tjeerdema, 2014). All of the 23 golf courses that submitted pesticide records to calculate an average pesticide risk for Wisconsin used chlorothalonil in their pesticide application programs. Chlorothalonil, as measured by HQ, is not a highly toxic pesticide because it has modest mammalian acute toxicity. The HQ model was used to defined risk levels of the State Risk Comparison study, and thus the 50%-SA treatment could include chlorothalonil. However, EIQ and PRT assign chlorothalonil a much higher risk value, which is why the 50%-SA treatment was the highest risk treatment, as quantified by PRT and EIQ, in the State Risk Comparison Study. In the PRT, chlorothalonil has a “high risk” score in the aquatic invertebrates and (human) dermal cancer categories, and a “moderate risk” score in the avian reproductive and aquatic algae categories. The EIQ value of chlorothalonil is 37, which is considered “high.” Indeed, recent toxicology studies indicate that chlorothalonil, while not acutely toxic to mammals, is an endocrine distributor in mice, and secondary metabolites of chlorothalonil can be particularly toxic to fish (Zhang et al., 2016; Hao et al., 2019). Our research indicates that reducing or removing chlorothalonil from a pest management program is the single most effective way to reduce pesticide risk on golf courses.
In the 25% treatment in the State Risk Comparison Study and the RR-SKM treatment in the SKM Reduced Risk study, the fungicides in the program are primarily lower risk pesticides such as boscalid, fluxapyroxad, and penthiopyrad from the SDHI fungicide class. Unfortunately, dollar spot populations can quickly develop resistance to SDHI fungicides and practitioners must use them wisely or risk developing SDHI resistant strains on their golf course (Sang et al., 2015; Popko et al., 2018). As such, pesticides like fluazinam were incorporated into the lower risk programs beginning in 2015 as a broad-spectrum, resistance-management fungicide with a lower risk profile than the traditional broad-spectrum fungicides that have higher risk profiles, such as chlorothalonil. Building a pesticide application program around lower risk fungicides while still incorporating lower risk resistance management chemistries, such as fluazinam, is a way to both reduce risk but decrease the likelihood of resistance development.
Trends in pesticide risk over time on US golf courses are unknown due to a lack of nationwide data, but multiple analyses have been conducted in US agriculture. Kniss (2017) found that herbicide use in US agriculture increased from 1990 to 2015 but that acute and chronic mammalian risk was mostly either declining or stable. However, Kniss (2017) only measured the chronic and acute risk trends to mammals. Schulz et al. (2021) found that despite large reductions in pesticide risk to fish, mammals, and birds, pesticide risk to aquatic invertebrates and pollinators has increased significantly over the past 25 years in US agriculture. Increased risk to aquatic invertebrates and pollinators was primarily a result of pyrethroid and neonicotinoid insecticides and came as insecticide use rates declined. These results highlight the importance both of calculating pesticide risk instead of weight of pesticide applied as a measure of ecological impact and estimating pesticide risk to a wide variety of non-target organisms.
Golf courses in Wisconsin spend on average $42,000 on pesticides annually (Bekken et al., 2021). Bekken et al. (2021) found that golf courses with lower maintenance budgets have lower pesticide risk than golf courses with higher maintenance budgets, suggesting that money is a limiting factor in pesticide use for lower maintenance budget golf courses. In the State Risk Comparison Study, the 25%-SA treatment reduced risk by 75% compared to the Wisconsin statewide average but only reduced costs by 10%. Thus, for practical purposes the 25%-SA program is cost neutral. In the SKM Reduced Risk study, the reduced risk treatment (RR-SKM) was nearly double the cost of the conventional program (CP). When calculating treatment costs from the provided distributor price list it was apparent the increased cost of the reduced risk programs was largely due to the increased cost of these products compared to older, more highly toxic compounds. For the average US golf course with 11.4 ha of fairways, the annual cost of the RR-SKM program would be ~$58,000 USD, compared to $36,200 for the CP. Given these significant cost differences, golf course superintendents are often not willing or not able to voluntarily pay higher prices for lower risk pesticides. The incentive for golf course superintendents to adopt reduced risk strategies is nuanced and will differ based on numerous situations. There is some scientific evidence that golf course superintendents have worse health outcomes which may be linked to on-the-job pesticide exposure (Kross et al., 1996; Knopper and Lean, 2010). Despite such evidence, Arcury-Quandt et al. (2011) found that most US golf course superintendents interviewed did not think that pesticides present a personal health risk. The authors are aware of no regulations in the US that require golf courses to track pesticide risk, or that set an upper limit on golf course pesticide risk. Even voluntary sustainability certification programs for golf courses, such as Audubon International and GEO-Certified, do not require golf courses to track pesticide risk. Superintendents in the US often feel pressure from golfers to maintain high quality pest-free playing surfaces, and therefore are incentivized to use more pesticide rather than less.
Denmark is a case study of how pesticide risk can be monitored across the golf industry. In Denmark, all golf courses are required to use an online pesticide application software [Scandinavian Turfgrass Environmental Research Foundation (STERF), 2019]. The program calculates pesticide risk using a pesticide risk indicator similar to EIQ. Under an agreement between the Danish Golf Union (DGU) and Danish Government, pesticide risk for each golf course component (greens, tees, fairways, and roughs) is capped every year [Scandinavian Turfgrass Environmental Research Foundation (STERF), 2019]. The amount of pesticide risk allowed on each component is being slowly reduced each year until a minimum sustainable level is reached, though what this level will be is currently a point of debate between the DGU and Danish Government. The Danish model of pesticide risk reduction has been effective in reducing pesticide risk on golf courses in the country and could serve as an example for reducing pesticide risk on golf courses around the world [Scandinavian Turfgrass Environmental Research Foundation (STERF), 2019]. Short of regulation, concepts of pesticide risk and how pesticide risk is calculated and tracked should be part of both the undergraduate turfgrass science curriculum and continuing education programs offered by superintendents' associations. In addition, voluntary sustainability standards in golf such as Audubon International and GEO-Certified could require their golf courses to track pesticide risk through software such as Playbooks for Golf ®, which can calculate a golf course's EIQ score. Other software programs used by superintendents to track pesticide applications should also consider integrating Pesticide risk indicators, such as EIQ, HQ, or PRT.
Dollar spot is the most common and costly disease for golf course superintendents in temperate US climates to control, but the repeated pesticide applications to control dollar spot can increase fungal resistance and risk both human and environmental health. This study found that lower risk fungicides can reduce pesticide risk by over 50% without sacrificing disease control. While such high levels of risk reduction may not be possible on all golf courses given the constraints of maintenance budgets and the development of fungal resistance, significant reductions in golf course pesticide risk to control dollar spot are achievable simply by making changes in product selection. Future advancements in disease resistant grasses, precision application technology, and plant-based fungicides may bring further reductions in pesticide risk and increase the sustainability of US golf courses.
The original contributions presented in the study are included in the article/Supplementary Material, further inquiries can be directed to the corresponding author/s.
KH and PK contributed to conceptualization, design of the study, field work, and editing paper drafts. MB and PK contributed to the data and statistical analyses. MB organized the database, produced the figures and tables, and wrote the first draft of the manuscript. All authors contributed to the manuscript revision and approved the submitted version.
The authors declare that the research was conducted in the absence of any commercial or financial relationships that could be construed as a potential conflict of interest.
All claims expressed in this article are solely those of the authors and do not necessarily represent those of their affiliated organizations, or those of the publisher, the editors and the reviewers. Any product that may be evaluated in this article, or claim that may be made by its manufacturer, is not guaranteed or endorsed by the publisher.
The authors would like to thank University Ridge Golf Course Superintendent Phil Davidson for allowing on-site research from 2014 through 2016. In addition, thanks to BASF Turf and Ornamental, Bayer CropScience, NuFarm, and Syngenta Professional Products for donating the products used in these studies. We would also like to thank Ariel Larson and the IPM Institute of North America for their assistance in calculating the Pesticide Risk Tool values in our studies. Last, we would like to thank the Univ. of Wisconsin-Madison College of Agricultural and Life Sciences Agricultural Research Stations for their support in maintaining the research plots at the O. J. Noer Turfgrass Research and Education Facility.
The Supplementary Material for this article can be found online at: https://www.frontiersin.org/articles/10.3389/fagro.2022.881591/full#supplementary-material
Arcury-Quandt, A. E., Gentry, A. L., and Marin, A. J. (2011). Hazardous materials on golf courses: Experience and knowledge of golf course superintendents and grounds maintenance workers from seven states. Am. J. Indust. Med. 54, 474–485. doi: 10.1002/ajim.20942
Barnard, C., Daberkow, S., Padgitt, M., Smith, M. E., and Uri, N. D. (1997). Alternative measure of pesticide use. Sci. Total Environ. 203, 229–244. doi: 10.1016/S0048-9697(97)00151-4
Bekken, M. A. H., Schimenti, C. S., Soldat, D. J., and Rossi, F. S. (2021). A novel framework for estimating and analyzing pesticide risk on golf courses. Sci. Total Environ. 783, 146840. doi: 10.1016/j.scitotenv.2021.146840
Burpee, L. L (1997). Control of dollar spot of creeping bentgrass caused by an isolate of Sclerotinia homoeocarpa resistant to benzimidazole and demethylation-inhibitor fungicides. Plant Dis. 81, 1259–1263. doi: 10.1094/PDIS.1997.81.11.1259
Cai, Y., Cabrera, J. C., Georgiadis, M., and Jayachandran, K. (2002). Assessment of arsenic mobility in the soils of some golf courses in South Florida. Sci. Total Environ. 291, 123–134. doi: 10.1016/S0048-9697(01)01081-6
Campbell, C. L., and Madden, L. V. (1990). Introduction to Plant Disease Epidemiology. New York, NY: Wiley.
Detweiler, A., Vargas, J., and Danneberger, T. (1983). Resistance of Sclerotinia homoeocarpa to iprodione and benomyl. Plant Dis. 67, 627–630. doi: 10.1094/P.D.-67-627
EPA Reduced Risk Program (2021). Available online at: https://www.epa.gov/pesticide-registration/conventional-reduced-risk-pesticide-program (accessed April 6, 2022).
Feng, M., Schrlau, J. E., Snyder, R., Snyder, G. H., Chen, M., Cisar, J. L., et al. (2005). Arsenic transport and transformation associated with MSMA application on a golf course green. J. Agricult. Food Chem. 53, 3556–3562. doi: 10.1021/jf047908j
Golembiewski, R., Vargas, J., Jones, A. L., and Detweiler, A. R. (1995). Detection of demethylation inhibitor (DMI) resistance in Sclerotinia homoeocarpa populations. Plant Dis. 79, 491–493. doi: 10.1094/PD-79-0491
Golf Course Industry (2015). State of the Industry Report. Available online at: http://www.golfcourseindustry.com/article/gci0115-golf-state-industry-report-2015/ (accessed April 6, 2022).
Greitens, T. J., and Day, E. (2006). An alternative way to evaluate the environmental effects of integrated pest management: pesticide risk indicators. Renew. Agricult. Food Syst. 22, 213–222. doi: 10.1017/S1742170507001755
Gunstone, T., Cornelisse, T., Klein, K., Dubey, A., and Donley, N. (2021). Pesticides and soil invertebrates: A hazard assessment. Front. Environ. Sci. 9, 643847. doi: 10.3389/fenvs.2021.643847
Haith, D. A., and Rossi, F. S. (2003). Risk assessment of pesticide runoff from turf. J. Environ. Qual. 32, 447–455. doi: 10.2134/jeq2003.4470
Hall, R (1984). Relationship between weather factors and dollar spot of creeping bentgrass. Can. J. Plant Sci. 64:167–174. doi: 10.4141/CJPS84-021
Hao, Y., Zhang, H., Zhang, P., Yu, S., Ma, D., Li, L., et al. (2019). Chlorothalonil inhibits mouse ovarian development through endocrine disruption. Toxicol. Lett. 303, 38–27. doi: 10.1016/j.toxlet,0.2018.12.011
IPM Institute of North America (2021). Available online at: https://pesticiderisk.org (accessed April 6, 2022).
Kearns, C. A., and Prior, L. (2013). Toxic greens: a preliminary study on pesticide usage on golf courses in Northern Ireland and potential risks to golfers and the environment. Safety Security Eng. 134, 173–182. doi: 10.2495/SAFE130171
Kerns, J. P., and Tredway, L. P. (2013). “Advances in turfgrass pathology since 1990,” in Turfgrass: Biology, Use, and Management, eds J. C. Stier, B. P. Horgan, and S. A. Bonos, S.A (Madison, WI: American Society of Agronomy, Crop Science Society of America, Soil Science Society of America), 733–776. doi: 10.2134/agronmonogr56.c21
Kniss, A. R (2017). Long-term trends in the intensity and relative toxicity of herbicide use. Nat. Commun. 8 ,14865. doi: 10.1038/ncomms14865
Knopper, L. D., and Lean, D. R. S. (2010). Carcinogenic and genotoxic potential of turf pesticides commonly used on golf courses. J. Toxicol. Environ. Health Part B 7, 267–279. doi: 10.1080/10937400490452697
Kovach, J., Petzoldt, C., Degni, J., and Tette, J. (1992). A method to measure the environmental impact of pesticides. N. Y. Food Life Sci. Bull. 139, 1–8.
Kross, B. C., Burmeister, L. F., Ogilvie, L. K., Fuortes, L. J., and Fu, C. M. (1996). Proportionate mortality study of golf course superintendents. Am. J. Indus. Med. 29, 501–506.
Latin, R (2021). A Practical Guide to Turfgrass Fungicides, 2nd Ed. St. Paul, MN: APS Publications. doi: 10.1094/9780890546741
Lee, H., Bremer, D., Su, K., and Keeley, S. (2011). Relationships between normalized difference vegetation index and visual quality in turfgrasses: effects of mowing height. Crop Sci. 51, 323–332. doi: 10.2135/cropsci2010.05.0296
Metcalfe, T. L., Dillion, P. J., and Metcalfe, C. D. (2008). Detecting the transport of toxic pesticides from golf courses into watersheds in the Precambrian shield Region of Ontario, Canada. Environ. Toxicol. Chem. 27, 811–818. doi: 10.1897/07-216.1
Mills, G., and Rothwell, J. D. (1982). Predicting diseases—the hygrothermograph. Greenmaster 8, 14–15.
Murphy, R. R., and Haith, D. A. (2007). Inhalation health risk to golfers from turfgrass pesticides at three Northeastern U.S. sites. Environ. Sci. Technol. 41, 1038–1043. doi: 10.1021/es060964b
National Golf Federation (NGF) (2021). Golf Industry Facts. Available online at: https://www.ngf.org/golf-industry-research/ (accessed April 6, 2022).
Oliver, D. P., Kookana, R. S., Miller, R. B., and Correll, R. L. (2016). Comparative environmental impact assessment of herbicides used on genetically modified and non-genetically modified herbicide-tolerant canola crops using two risk indicators. Sci. Total Environ. 557–558, 754–763. doi: 10.1016/j.scitotenv.2016.03.106
Pichler, T., Brinkmann, R., and Scarzella, G. I. (2008). Arsenic abundance and variation in golf course lakes. Sci. Total Environ. 394, 313–320. doi: 10.1016/j.scitotenv.2008.01.046
Popko, J. T., Sang, H., Lee, J., Yamada, T., Hoshino, Y., and Jung, G. (2018). Resistance of Sclerotinia homeocarpa field isolates to succinate dehydrogenase inhibitor fungicides. Plant Dis. 102, 2625–2631. doi: 10.1094/PDIS-12-17-2025-RE
Rossi, F. S., and Grant, J. A. (2009). Long term evaluation of reduced chemical pesticide management of golf course putting turf. Int. Turfgrass Soc. 11, 77–90. Available online at: https://hdl.handle.net/1813/43858
Royal Ancient (R&A) (2019). Golf Around the World. Available online at: https://www.randa.org/TheRandA/AboutTheRandA/DownloadsAndPublications (accessed April 6, 2022).
Ryan, C. P., Dernoeden, P. H., and Grybauskas, A. P. (2012). Seasonal development of dollar spot epidemics in six creeping bentgrass cultivars in Maryland. Hortscience 47, 422–426. doi: 10.21273/HORTSCI.47.3.422
Salgado-Salazar, C., Beirn, L. A., Ismaiel, A., Boehm, M. J., Carbone, I., Putman, A. I., et al. (2018). Clarireedia: a new fungal genus comprising four pathogenic species responsible for dollar spot disease of turfgrass. Fungal Biol. 122, 761–773. doi: 10.1016/j.funbio.2018.04.004
Sang, H., Hulvey, J., Popko, J. T., Lopes, J., Swaminathan, A., Chang, T., et al. (2015). A pleiotropic drug resistance transporter is involved in reduced sensitivity to multiple fungicide classes in Sclerotinia homoeocarpa (F.T. Bennett). Mole. Plant Pathol. 16, 251–261. doi: 10.1111/mpp.12174
Sapkota, S., Catching, K. E., Raymer, P. L., Martinez-Espinoza, A. D., and Bahri, B. A. (2022). New approaches to an old problem: dollar spot of turfgrass. Phytopathology 112, 469–480. doi: 10.1094/PHYTO-11-20-0505-RVW
Scandinavian Turfgrass Environmental Research Foundation (STERF) (2019). Experience With an Upper Limit for Total Pesticide Use on Danish Golf Courses. Available online at: http://www.sterf.org/Media/Get/3162/fjeldsted-sterf-pesticide-seminar-7march-2019-danish-epa-experiences.pdf (accessed April 6, 2022).
Schulz, R., Bub, S., Petschick, L. L., Stehle, S., and Wolfram, J. (2021). Applied pesticide shifts towards plants and invertebrates, even in GM crops. Science 372, 81–84. doi: 10.1126/science.abe1148
Smiley, R. W., Dernoeden, P. H., and Clarke, B. B. (2005). Compendium of Turfgrass Diseases, 3rd Edn. St. Paul, MN: APS Press. doi: 10.1094/9780890546154
Smith, D. L., Kerns, J. P., Walker, N. R., Payne, A. F., Horvath, B., Inguagiato, J. C., et al. (2018). Development and validation of a weather-based warning system to advise fungicide applications to control dollar spot on turfgrass. PLoSONE 13, e0194216. doi: 10.1371/journal.pone.0194216
US EPA (2021). Pesticide Science and Assessing Pesticide Risks. Available online at: https://www.epa.gov/pesticide-science-and-assessing-pesticide-risks (accessed April 6, 2022).
Van Scoy, A. R., and Tjeerdema, R. S. (2014). “Environmental fate and toxicology of chlorothalonil,” in Reviews of Environmental Contamination and Toxicology, Vol. 232, ed D. Whitacre (Heidelberg; Dordrecht; London; New York, NY: Springer), 89–105. doi: 10.1007/978-3-319-06746-9_4
Wong, H., and Haith, D. A. (2014). Volatilization of pesticides from golf courses in the United States: mass fluxes and inhalation health risks. J. Environ. Qual. 42, 1615–1622. doi: 10.2134/jeq2013.01.0017
Woods, R. A (2017). Spotlight on Statistics: Sport and Exercise. U.S. Bureau of Labor Statistics. Avaialble online at: https://www.bls.gov/spotlight/2017/sports-and-exercise/pdf/sports-and-exercise.pdf (accessed April 06, 2022).
Keywords: turfgrass, hazard quotient (HQ), Environmental Impact Quotient (EIQ), ecological risk assessment (ERA), Pesticide Risk Tool, fungi, plant disease, fungicide
Citation: Bekken MAH, Hockemeyer KR, Soldat DJ and Koch PL (2022) Reducing Pesticide Risk Associated With Dollar Spot Management on Golf Course Turfgrass. Front. Agron. 4:881591. doi: 10.3389/fagro.2022.881591
Received: 22 February 2022; Accepted: 20 April 2022;
Published: 13 May 2022.
Edited by:
Jonathan Spencer West, Rothamsted Research, United KingdomReviewed by:
Benjamin Richard, University of Hertfordshire, United KingdomCopyright © 2022 Bekken, Hockemeyer, Soldat and Koch. This is an open-access article distributed under the terms of the Creative Commons Attribution License (CC BY). The use, distribution or reproduction in other forums is permitted, provided the original author(s) and the copyright owner(s) are credited and that the original publication in this journal is cited, in accordance with accepted academic practice. No use, distribution or reproduction is permitted which does not comply with these terms.
*Correspondence: Paul L. Koch, cGxrb2NoQHdpc2MuZWR1
Disclaimer: All claims expressed in this article are solely those of the authors and do not necessarily represent those of their affiliated organizations, or those of the publisher, the editors and the reviewers. Any product that may be evaluated in this article or claim that may be made by its manufacturer is not guaranteed or endorsed by the publisher.
Research integrity at Frontiers
Learn more about the work of our research integrity team to safeguard the quality of each article we publish.