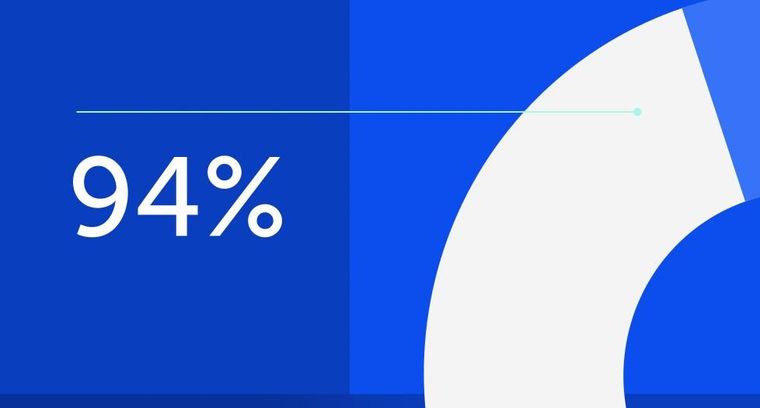
94% of researchers rate our articles as excellent or good
Learn more about the work of our research integrity team to safeguard the quality of each article we publish.
Find out more
ORIGINAL RESEARCH article
Front. Agron., 10 May 2022
Sec. Agroecological Cropping Systems
Volume 4 - 2022 | https://doi.org/10.3389/fagro.2022.823069
This article is part of the Research TopicConservation Agriculture: Knowledge Frontiers Around the WorldView all 11 articles
Conservation agriculture (CA) allows farmers to reduce costs and enhance soil health, but tends to increase weed infestation and associated crop yield loss, and/or herbicide use. We investigated how much tillage reduces weed infestation and yield loss, and which systems and weed species are the most affected by tillage suppression. We collected farming practices on 395 arable cropping systems mainly from France, and simulated them over 30 years and with 10 weather repetitions, using FLORSYS. This process-based model simulates daily multi-species weed floras and crop canopies from cropping systems and pedoclimate over the years. Three series were simulated, (1) using the recorded systems, (2) eliminating tillage without any other changes, (3) eliminating herbicides without any other changes. Each series was run twice, once with a regional weed-flora pool to simulate weed dynamics and their impact on crop production and biodiversity, and once without weeds to predict potential crop yield. Among the recorded systems, herbicide treatment frequency index (HTFI) averaged over rotation increased when tillage frequency decreased. No recorded no-till system was herbicide-free. The untilled crops with the lowest HTFI (0.16) were unusual crops, i.e., relay grass-crops or multi-annual crops. Simulations of the recorded systems showed no correlation between tillage-frequency, and weed biomass or weed-caused yield loss. When tillage was deleted without any other change, yield loss almost doubled. We identified (1) which weed species and traits (e.g., spring annuals) increased after tillage suppression and in which cropping systems, (2) which recorded systems were robust to tillage suppression in terms of yield loss (with cover crops, summer crops, etc.), (3) which no-till systems (recorded or obtained after deleting tillage) limited yield loss (e.g., with frequent and/or efficient herbicides), (4) which management techniques were associated with a reduction in tillage, in herbicides, and in yield loss (long and diverse rotations, cover crops, etc.). No tested system achieved all three objectives simultaneously. The simulations indicated that two CA pillars (diverse crop rotations, cover cropping) were essential to manage weeds while reducing (or eliminating) both tillage and herbicide use. More no-till cropping systems must be investigated to determine whether sustainable no-till herbicide-free systems are possible.
Conservation agriculture relies on three fundamental pillars: minimum soil disturbance (i.e., only the soil disturbance required to introduce the crop seeds into the soil is tolerated), permanent soil cover with crop residues and living mulches, and diversified crop rotation in space and time (Reicosky, 2015). Initiated primarily by farmers to reduce soil degradation (Sun et al., 2015) and production costs (Hobbs et al., 2008), this crop management system is challenged by increased weed infestation (Derrouch et al., 2020) and yield loss (Pittelkow et al., 2015). Indeed, implementing no-till systems deprives farmers of key non-chemical weed management levels (Cordeau et al., 2020). As weeds are the most damaging pest for crop production (Oerke, 2006) and their threat to crop production is even increasing because of agricultural intensification and climate change (Storkey et al., 2021), conservation agriculture is frequently accompanied by an increase in herbicide use intensity (Friedrich, 2005). Moreover, national and European legislation increasingly call for a drastic reduction in pesticide use, particularly herbicide use (Kudsk and Mathiassen, 2020). Simultaneously, weed diversity is known to be higher in conservation agriculture than in tillage-based systems (Adeux et al., 2019; Derrouch et al., 2021), and weeds support ecosystem services provided by crop auxiliaries or pollinators (Blaix et al., 2018; Barbercheck and Wallace, 2021). So, today's weed management in conservation agriculture must shift from short-term weed control aiming at total eradication to long-term weed management aiming to favor beneficial weed species to the detriment of those damaging crop production, while reducing herbicide use.
To achieve this major break, we need to understand how much tillage contributes to controlling weeds and crop yield loss as well as which cropping systems and weed species would be most affected if tillage were deleted. Field studies would be too slow and expensive to answer these questions, they often only monitor a small number of cropping systems, weed floras and pedoclimates, and disregard long-term effects (Ramsdale et al., 2006; Hiltbrunner et al., 2008; Adeux et al., 2019). Surveying farm-field networks would allow extending the range of investigated systems and conditions, but makes it more difficult to analyze biophysical processes and risk confounding effects, particularly the effect of abandoning tillage with effects of compensatory measures taken by farmers (Derrouch et al., 2020). The same pitfall occurs when investigating the relationships between herbicide use intensity and weed infestation/damages (Colbach et al., 2020b). The latter critical review proposed combining surveys of farming practices with simulation models as a way out of the trap. The feasibility of this approach was demonstrated in a previous study, concluding that reduced herbicide use does not increase crop yield loss if it is compensated by alternative preventive and curative measures (Colbach and Cordeau, 2018).
The objective of the present paper was to identify technical and biological drivers to achieve the triple target of reducing tillage and herbicide use as well as yield loss due to weeds. To reach this target, we recorded cropping systems from farming practices and combined them with simulations with a process-based weed dynamics model in order to determine (1) which weed species and traits were increased after tillage suppression and in which cropping systems, (2) which cropping systems were the most robust to tillage suppression in terms of weed infestation and the resulting crop yield loss, (3) which no-till cropping systems limited weed-caused yield loss the most, (4) which cropping techniques were associated with reduced tillage, herbicide use and yield loss. The simulation model used was FLORSYS (Colbach et al., 2021) which is to date the most complete in terms of effects of management techniques and weeds (see Chantre et al., 2020 for examples of other models).
The principle was developed by Colbach and Cordeau (2018) to investigate the contribution of herbicides to weed management. It was adapted here to focus on tillage vs. no-till systems. The first step consisted in collecting cropping system data from diverse regions and production situations, from farmers' interviews, agricultural statistics, regional expertise and cropping-system design.
The cropping systems were then simulated with a virtual-field model. The chosen model had to simulate multi-species and multi-cohort weed dynamics and their impact on crop production as a function of cropping systems and pedoclimate, at a daily scale over several years or decades. FLORSYS (Gardarin et al., 2012; Munier-Jolain et al., 2013, 2014; Colbach et al., 2014a,b, 2017c, 2021; Mézière et al., 2015) is the only model answering to these requirements. In addition to direct weed harmfulness (yield loss, harvest contamination), FLORSYS predicts indicators of technical (harvesting problems), sociological (loss of face due to field infestation) and indirect harmfulness (promotion of weed-borne crop diseases and parasites) (Mézière et al., 2015; Colbach et al., 2017a).
The simulation plan was designed to disentangle confounding effects. All cropping systems were simulated in pairs, with and without weeds, to estimate the impact of weeds on crop yields in each system. Three sets of paired simulations were run. (1) One set consisted of the recorded systems. (2) A second set took the recorded systems and removed all tillage operations without any other changes. (3) The third set took the recorded systems and removed all herbicide applications, again without any additional changes. This design allowed estimation of the effects of tillage and herbicides on weeds without the confounding effects of altered cultural practices that normally accompany such changes.
FLORSYS is a virtual field on which cropping systems can be experimented and a large range of crop, weed and environmental measures estimated (Gardarin et al., 2012; Munier-Jolain et al., 2013, 2014; Colbach et al., 2014a,b, 2017c, 2021; Mézière et al., 2015). Further information on the model can be found in Section A in Supplementary Material.
The input variables of FLORSYS consist of (1) a description of the simulated field (daily weather, latitude and soil characteristics); (2) all the simulated crops and management operations in the field, with dates, tools and options; and (3) the initial weed seed bank which is either measured on soil samples or, more feasible, estimated from regional flora assessments (Colbach et al., 2016). These input variables influence the annual life cycle which applies to annual weeds and crops, with a daily time-step. Pre-emergent stages (surviving, dormant and germinating seeds, emerging seedlings) are driven by soil structure, temperature and water potential. Post-emergent processes (e.g., photosynthesis, respiration, growth, and etiolation) are driven by light availability and air temperature. At plant maturity, weed seeds are added to the soil seed bank; crop seeds are harvested to determine crop yield (in t/ha and in MJ/ha). In case of multi-annual crops (e.g., lucerne, ryegrass), seedlings can also be the offspring of vegetative older plants. The FLORSYS version used here was parameterized for 30 frequent and contrasting annual weed species (see list in Table A1).
Life cycle processes also depend on the dates, options and tools of management practices (tillage, sowing, herbicides, mechanical weeding, mowing, harvesting), in interaction with weather and soil conditions on the day the operations are carried out (Section A.5 in Supplementary Material). For instance, weed plant survival probabilities are calculated deterministically depending on management operations (tillage, herbicides, mechanical weeding, mowing, harvesting), biophysical environment as well as weed morphology and stage; the actual survival of each plant is determined stochastically by comparing this probability to a random probability.
FLORSYS simulates crop yield (in t/ha and MJ/ha) as well as a set of indicators assessing weed impact on crop production (Mézière et al., 2015; Colbach et al., 2020a) (see Section A.6 in Supplementary Material). These indicators consider direct harmfulness for crop production (crop yield loss, harvest pollution by weed debris), technical harmfulness (harvesting problems due to green weed biomass blocking the harvest combine), and sociological harmfulness (field infestation by weed biomass during crop growth) which reflects the farmer's worry of being thought incompetent by their peers even if there is no effect on yield loss. Weed benefits include wild plant biodiversity and contribution to feeding fauna (birds, pollinators, carabids).
FLORSYS was evaluated with independent field data, showing that crop yields, daily weed species densities and, particularly, densities averaged over the years were generally well predicted and ranked as long as a corrective function was added to keep weeds from flowering during winter at more southern latitudes (Colbach et al., 2016). Higher crop yield losses than those reported in previous field studies mostly resulted from the simulation plan. This does not adapt practices to simulated weed floras and interannual weather variability (as farmers or trial managers would do), in order to discriminate the effect of crop species and management practices on weeds from the effect of weeds on the choice of crops and practices (Colbach and Cordeau, 2018).
A total of 395 arable cropping systems were recorded for this study (Table 1). They originated from detailed descriptions of systems existing in cropping system trials and in farms, the Biovigilance-Flore network collecting regional practices (Fried et al., 2008), expert opinion on existing and prospective practices, participatory workshops with farmers aiming to reduce herbicide use, or were designed with the help of decision trees aiming to reconcile reduced weed harmfulness, reduced herbicide use and biodiversity conservation. These systems covered one Spanish and 14 French territories or locations from ten nine regions. They included both conventional and organic systems, with a tillage intensity varying from no-till to annual mouldboard plowing. Crop diversity varied from monocultures (usually maize, Zea mays) to highly diverse rotations, including intercropping and cover crops. Rotations were mainly based on cereals (wheat, Triticum aestivum; barley, Hordeum vulgare; maize) and oilseed rape (Brassica napus), with a smaller proportion of legumes (lucerne, Medicago sativa; faba bean, Vicia faba; etc.), non-legume broadleaved crops (sunflower, Helianthus annuus; flax, Linum usitatissimum etc.) and temporary grassland, with proportions and crop species depending on regions.
Table 1. Summary of the 395 cropping systems used in the simulation study (further details in Section B.1 in Supplementary Material).
Several hundred descriptors (see examples in Table A2) were calculated from the detailed operations to describe each cropping system, both at annual scale (e.g., previous crop species, current crop, number of tillage operations prior to sowing, days from last tillage to cash-crop sowing…) and at rotation scale (e.g., proportion of spring crops in the rotation, proportion of wheat crops preceded by legumes, number of tillage operations per year averaged over the rotation, days from last tillage to cash crop sowing averaged over the rotation…). The rotation-scale descriptors also discriminated practices per crop (e.g., number of tillage operations per year before wheat averaged over all wheat crops in the rotation) and assessed inter-annual variability in operation dates (e.g., variability in cash-crop sowing dates as the median over the rotation of the differences in dates of year N vs. year N-1) and frequency (e.g., variability in number of tillage operations).
Three series of simulations were run. The first (hence “recorded”) simulated the cropping systems starting with a typical regional weed seed bank consisting of the 30 weed species currently included in FLORSYS (Table A1). The second series (“tillage-free”) eliminated all tillage operations from the recorded systems, without any other change in management practices. The third (“herbicide-free”) eliminated all herbicides from the recorded systems without changing anything else. These series provided output variables such as weed and crop state variables and all weed-impact indicators (see Section Effect of Weeds on Crop Production and Biodiversity), except crop yield loss. To calculate the latter, the three simulation series were run again, this time without any weeds (i.e., without an initial seedbank), to predict potential crop yield as the yield difference between weedy and weed-free simulations.
In each series, each cropping system was simulated over 30 years, repeating the basic rotational pattern (e.g., oilseed rape/wheat/barley) over time. For each region, a typical soil (texture etc.) was based on soil analyses from the cropping system trials or from locations inside the simulated regions (Section B.2 in Supplementary Material). Daily weather variables were recorded by INRAE weather stations in the different regions (INRAE Climatik platform, https://intranet.inrae.fr/climatik). Each system was run 10 times with 10 different weather series to assess the systems's response to different weather conditions. Each series consisted of 30+1 randomly chosen weather years (+1 because cropping years run from summer to summer in the Northern hemisphere) from its region of origin (e.g., repetition 1 = 1998, 2013, 1983, ….; repetition 2 = 2000, 2002, 1987,…). The systems of a given region were tested with the same 10 weather series (hence, weather repetitions), which resulted in a randomized block design, with cropping systems as a factor and 10 blocks. The dates and options of the simulated management operations remained unchanged in the different weather repetitions, regardless of the simulated conditions and weed floras. This allowed us to discriminate the impact of cultural practices on weeds from its reciprocal, i.e., the way farmers adapt their practices to weather and weeds, depending on their mental model.
In total, 395 systems × 10 weather repetitions were run over 30 years for each of the three series, with and without weeds, resulting in a total of 711,000 data lines.
The analyzed outputs included the weed-impact indicators described in Section Effect of Weeds on Crop Production and Biodiversity and plant density during seasonal fallow (i.e., the period between the previous cash-crop harvest and the next cash-crop sowing) and during cash crop (average over all days, maximum) for each weed species. Crop yield loss was calculated as 100 × (yield from weedy simulations – yield from weed-free simulations)/yield from weed-free simulations. Yield could be either grain yield (t/ha) or above-ground biomass production (t/ha) in fodder crops (e.g., lucerne, grassland) though we mostly focused on grain yield loss. These outputs were analyzed both at annual (118,500 data points for a given simulation series = 395 systems × 30 years × 10 weather repetitions) and multi-annual scale by averaging indicator values over the 30 simulated years (3,950 data points = 395 systems × 10 weather repetitions).
The recorded cropping-system data set (395 systems) was characterized by analyzing tillage frequency (number of all tillage operations), mouldboard plowing frequency (absence or presence of plowing) and herbicide use intensity (treatment frequency index, with 1 = one product sprayed at full dosage over the whole field) in a given year of the rotation as a function of the crop species grown that year, the region, and the origin of the cropping system (e.g., farm survey, crop advisor), using the lm() and Anova() functions of R (R Core Team, 2017) to determine Type-III sum of squares and partial R2 per factor. This was followed by a comparison of means for each factor, using the lsmean() function.
To identify the determinants of tillage intensity, a classification and regression tree (CART) (Breiman et al., 1984) was used to analyze the number of tillage operations per year, first at annual (i.e., in a given year) and then at rotation scale (i.e., averaged over the rotation) as a function of all other cropping-system variables, excluding any tillage-related variables. The annual-scale analysis identifies the cropping-system characteristics associated with an untilled crops, the rotation-scale analysis those linked with a no-till cropping system.
A CART predicts a continuous response variable (here, tillage frequency) from a set of discrete or continuous predictors (here, the other cropping-system variables). The data set is recursively split into two subsets along a threshold value of the predictor in order to maximize the difference between subsets. When predictors are partially correlated, as is the case in logically constructed cropping systems, several predictors (surrogates) can split the set exactly in the same way. This reduces the risk of confounding effects. Branches are combinations of predictor values that lead to predictions contained in leaf nodes. Variable importance of predictors (VIP) are used to rank predictors (including surrogates) based on the contribution predictors make to the construction of the tree, i.e., according to their impact on the response variable. Partial R2 were calculated for each type of management techniques (e.g., herbicide, rotation) by summing the VIP of all variables of a given technique-type, multiplying it by the R2 of the CART and then dividing by the total VIP of all predictors (see Section D in Supplementary Material). The trees were computed, using the package mvpart (De'ath et al., 2014) of R (R Core Team, 2017). The optimal tree size was internally identified using a 10-fold cross validation procedure to avoid overfitting. In the following R2 refers to the cross-validated R2 of the resulting tree.
The analysis carried out at the annual scale (395 systems × rotation length of a given system) used both annual and rotation-scale descriptors (see Section Recorded cropping systems) as predictors. The rotation-scale analysis (395 systems) only worked with rotation-scale descriptors. The annual CART rules were then applied to the “recorded” data set (395 systems × 30 years × 10 weather repetitions) to distribute all data points into the tree branches (with the rpart.predict.leaves() function) and calculate average herbicide use intensity and crop yield loss due to weeds for each branch.
To assess the role of tillage and herbicides for weed control, the data of the three series were analyzed in a common analysis of variance, analyzing grain yield loss as a function of simulation factors, i.e., series, region, cropping system, and weather repetition (lm() and Anova() functions) followed by a comparison of means for series (lsmeans() function).
To determine which weed-impact indicators (e.g., weed species richness, yield loss…) were the most affected by tillage suppression, the difference in indicator values in the “tillage-free” minus those in the “recorded” simulation series were calculated for each year and weather repetition, and averaged over all 395 cropping systems, 30 years and 10 weather repetitions.
To assess the correlation between initial tillage frequency and variation in weed-impact after tillage suppression, linear regressions were fitted to the variation in grain yield loss (values of “tillage-free” minus “recorded” series) vs. tillage frequency or plowing frequency, using the lm() function. The analysis was carried out at the rotation scale (395 systems × 10 weather repetitions).
To identify how weed species reacted to the suppression of tillage and which species traits were selected by this suppression we ran an RLQ analysis, using the ade4 package (Chessel et al., 2004) of R (R Core Team, 2016). The RLQ analysis was initially developed to investigate correlations between cultural techniques (R matrix) and species traits (Q matrix) via weed species densities (L matrix). Here, we used the cropping techniques of the recorded cropping systems for the R matrix. The Q matrix consisted of 142 parameters for the 30 weed species in FLORSYS (see examples in Section A.4 in Supplementary Material). The L matrix comprised the differences in log10-transformed plant density of each weed species (after adding 0.01 to account for zero values) from simulations of tillage-free systems vs. recorded ones, for each of the 27 years and the 10 repetitions, using the maximums of the daily weed species densities between previous and current crop harvests. Only technique-parameter relationships significant at p = 0.05 and Pearson correlation coefficients > 0.025 after a 4th corner analysis were considered, using the fourthcorner() function of R. This analysis tests whether species are distributed independently of their response to techniques and of their traits, retaining for each technique × trait combination the highest p-values of models permuting either techniques or traits. To check whether weed species could be aggregated into functional groups in terms of response to cropping techniques related to species characteristics, species were grouped based on a Ward ascendant hierarchy classification using the hclust() function of R according to the Euclidian distances separating coordinates of species in the RLQ multidimensional space.
To identify the determinants that increase cropping-system robustness to tillage suppression, the variation in grain yield loss due to tillage suppression (values of “tillage-free” minus “recorded” series) was analyzed as a function of cropping-system variables, excluding all tillage-related variables, using a stepwise linear regression with PROC GLMSELECT of SAS (version 9.4). This approach was developed to select from a very large number of effects (Cohen, 2006) and has been successfully used in various disciplines (e.g., Van der Borght et al., 2011). Cropping-system variables were introduced sequentially (stepwise selection), by adding effects that at each step produce the smallest value of the Schwarz Bayesian information criterion (SBC) statistic and stopping when adding any effect increased the SBC statistic again. The final model was chosen among the successive models as the one that yielded the lowest predicted residual sum of square with cross validation. Using a method including cross-validation leads to more robust relationships and avoids over-fitting.
The 395 recorded cropping systems differed in terms of tillage frequency, mouldboard plowing frequency herbicide use intensity. These frequencies and intensities depended on the crops (partial R2 = 0.12, 0.07, and 0.11 for tillage frequency, plowing frequency and herbicide use intensity, respectively, details in Section B.4 in Supplementary Material), regions (partial R2 = 0.08, 0.05, and 0.11) and, to a lesser degree, cropping-system origin (e.g., farm survey, expert opinion, partial R2 = 0.01, 0.08, and 0.02). Tillage (particularly mouldboard plowing) was the most frequent before spring crops (e.g., pea, soya, sugar beet, sunflower, flax, 3.21–4.44 operations per year). It was the lowest before fodder crops (~0) and oilseed-legume intercropping (1.03). Similar tendencies were seen for herbicide use intensity, with the highest herbicide treatment frequency index in sugar beet (HTFI = 2.61) and the lowest in fodder crops (0.52).
Interestingly, cropping-system origin also influenced tillage and herbicide practices. Tillage was the most frequent in cropping systems recorded during farm surveys (3.29 tillage operations/year) or designed during participatory workshops with farmers (3.16) and similar for other origins (2.61–2.76 for systems provided by advisors, experimenters, regional statistics or designed with a decision tree). Conversely, mouldboard plowing was, by far, the most frequent in the systems designed with decision trees (frequency = 0.83/year), and the lowest in those proposed by crop advisors (0.10). Finally, herbicide use intensity was the highest in systems recorded in the Biovigilance network (HFTI = 1.84) or during farm surveys (1.70). It was the lowest in innovative cropping systems designed by farmer workshops (1.47), trial managers (1.33), and scientists using decision trees (1.40).
Generally, herbicide use intensity was negatively correlated with tillage frequency in the recorded cropping systems, and vice-versa (Figure 1). No-till systems differed greatly in terms of herbicide use intensity which varied more or less continuously from a treatment frequency index of 1 to more than 3 on average over the rotation, just as most of tilled systems. Conversely, herbicide-free systems were characterized by a tillage frequency that was higher than for herbicide-inclusive systems, with either ~5 or 10 tillage operations per year on average over the rotation. There were no systems that were both tillage-free and herbicide-free. The herbicide-free system with the lowest tillage frequency presented 2.8 operations per year averaged over the rotation.
Figure 1. Herbicide use intensity vs. tillage intensity in the 395 recorded cropping systems (the blue line shows the fitted regression y = 2.24–0.217 x, with the confidence interval in gray). The color and size of the data points illustrate the simulated grain yield loss averaged over 30 simulated years, ranging from zero loss (green, smallest symbol) to total loss (magenta, largest symbol). Symbols are partially transparent to take account of overlaying. HTFI, herbicide treatment frequency index (unitless), i.e., average number of doses at the recommended dose per ha per year (Colbach, 2022 ).
The typology of cropping systems according to their tillage frequency (regardless of type of operation) discriminated only six types according to their average herbicide efficiency on monocot weeds, the frequency of herbicides moderately efficient on dicot weeds, the frequency of mechanical weeding with a harrow as well as the frequency of maize and winter crops in the rotation (Figure 2). The lowest tillage frequencies were associated with the most frequent and efficient herbicides. The typology at the annual scale, using both annual and cropping-system variables, distinguished 45 types whose tillage frequency varied from 0 operations per year to 9. Tillage frequency was mostly linked to different herbicide strategies (partial R2 = 0.32, details in Section B.6 in Supplementary Material), followed by descriptors of rotation (0.19) and (weed-free) manure spreading (0.07). The presence of a cover crop during the fallow period and non-chemical destructive operations (mechanical weeding, mowing, shredding, topping) was little or not at all correlated to tillage frequency (partial R2 < 0.01 and 0.02).
Figure 2. Typology of cropping systems with different tillage frequencies (indicated in nodes and leaves, colored from = lowest to = highest value, with % data included in node shown below) in the data set consisting of 395 cropping systems. Classification and regression tree explaining the number of tillage operations per year on average over the rotation from cropping-system variables other than tillage-related variables. Percentages below nodes and leaves indicate the percentage of systems among the total 395 systems. Total R2 is 0.59, partial R2 are 0.38, 0.11, and 0.10 for impacts of herbicides, mechanical weeding, and rotation, respectively (Colbach, 2022 ).
The most frequent cropping-system type (type C, 6.6% of all analyzed years, Table 2) without tillage before crop sowing was thus based on:
- herbicides targeting dicot weeds frequent in the rotation (more than 1.33 applications per year on average over the rotation), resulting in a HTFI exceeding 1.97,
- rare crop-residue shredding during fallow (<0.49 operations per year on average over the rotation),
- few or no rolling operations, particularly before summer crops like sunflower,
- wheat sown in rows and not on soil surface (i.e., at least 1 cm deep).
Table 2. Typology of tillage-free crops among the 395 recorded cropping systems, based on a classification and regression tree. Discriminating practices are ranked from the most influential at the top to the least influential at the bottom. Cell colors distinguish variables in relation to rotation (green), herbicides (red), sowing (purple), crop-residue shredding, mowing and topping (blue), and others (white). Italics show practices averaged over rotation. TFI, Treatment Frequency Index (1=one commercial product applied at the recommended dose over the whole field a given year).
The combination of these practices resulted in a high herbicide use intensity (HTFI = 2.38 averaged over the rotation) but crop yield loss (20% averaged over rotation) was lower than the average of the dataset (27%, Figure 3). The second most frequent strategy (type B, 2.5% of analyzed years, Table 2) was very different and did not need herbicides, but consisted of unusual crops, either relay crops (e.g., lucerne sown during a previous sunflower) or multiple years of multi-annual crops (e.g., temporary grassland or lucerne). As a consequence, herbicide use intensity was close to zero (HTFI = 0.16 averaged over the rotation). Yield loss was lower than in the type-C strategy, whether for grain or biomass production.
Figure 3. Effect of deleting tillage or herbicides without any compensation in cropping systems on grain yield loss due to weeds, averaged over all simulated years. The analyzed data set consisted of the same 395 cropping systems × 30 years × 10 weather repetitions, simulated without modification (Standard), after deleting all tillage operations (NoTill) or after deleting all herbicide operations (NoHerbicides). Blue diamonds show means, letters indicate differences significant at p = 0.05 (Colbach, 2022 ).
Among the recorded cropping systems, there was a significant but negligible negative correlation at the rotation scale between grain yield loss due to weeds on one hand, tillage frequency (p = 0.01) and herbicide use intensity (p = 0.001) on the other hand (%yield loss = 38.5 – 0.88 × tillage frequency – 4.59 × herbicide treatment frequency index). As this correlation explained only 1% of the variability in yield loss (R2 = 0.01), both high and low yield loss values could occur for any tillage and/or herbicide intensity (Figure 1). The same tendencies were seen at the annual scale and/or for weed biomass (Section C.1 in Supplementary Material). The same applied when looking at the frequency of superficial tillage operations only or at mouldboard plowing only.
When tillage was deleted from cropping systems without any compensatory practices, field infestation (i.e., weed biomass in cash crop) more than tripled (from 0.51 to 1.8 t/ha), whether looking at annual values or averages over the rotation (Figure 3, details in Section C.2 in Supplementary Material). Grain yield loss due to weeds increased less, but still significantly (p = 0.05) from an average of 27–45%, regardless of the analyzed scale. The increase in weed biomass and yield loss were slightly larger when herbicides were deleted without compensation (to 2.1 t/ha weed biomass and 49% yield loss).
Tillage deletion similarly increased most of the weed impacts, i.e., harvest pollution, harvesting difficulty, or weed-based carabid-food offer (Table 3). Weed offer for birds and pollinators increased approximately twice as much. Conversely, species richness, field infestation and potential yield (in the absence of any weeds) varied little on average.
Table 3. Which weed impacts were impacted most when tillage was deleted without any other changes in management practices? Average difference in weed impact indicators from tillage-free simulations—values from unmodified cropping systems (395 systems × 30 years × 10 weather repetitions) (percentages show variation relatively to mean indicator values from unmodified cropping systems). Cells of relative variation were colored in green for beneficial effects (increased biodiversity) and red for detrimental effects (decreased yield, increased weed harmfulness), and the stronger the relative variation was, the darker the color.
The increase in weeds and the resulting yield loss depended very much on the tillage frequency in the unmodified systems. The difference in yield loss between the tillage-free and the unmodified systems increased significantly with the total number of tillage operations per year in the unmodified system on average over the rotation (y = 5.61 + 5.12 x, R2 = 0.10, p < 0.001, details in Section C.3 in Supplementary Material). The correlation with the number of superficial operations was almost identical. Conversely, the correlation with the mouldboard plowing frequency in the unmodified system was negligible (R2 = 0.003), indicating that the frequency rather than the type of disturbance was important to control weeds. There was, though, a huge variability in yield-loss variation for a given initial tillage frequency (as shown by the low R2, even for the total number of tillage operations).
The impact of tillage suppression on weed densities depended on the weed species (Figure 4). Approximately half of the species, like Amaranthus retroflexus (AMARE) or Sonchus asper (SONAS) saw their plant densities increase in the majority of situations (i.e., year × cropping system × weather repetition). Six out of the 30 species decreased in the majority of situations (e.g., AVEFA: Avena fatua) whereas no tendency was visible for the remaining species.
Figure 4. Variation in weed plant densities when deleting tillage without compensation in the 395 systems × 30 years × 10 weather repetitions. Weed species are ordered according to decreasing change in densities and named with their EPPO codes, for full names see Table A1. Boxes show 25, 50 (median), and 75-percentiles of distribution, whiskers extend from the box to, respectively, the lowest and largest values no further from the box than 1.5 × the height of the box, blue diamonds are mean values. Boxes were colored in for species whose mean variation was significantly > 1 and whose value-distribution was skewed toward values > 1 (and vice-versa for boxes colored in ). Letters on top of the plot refer to species clusters established during the RLQ analysis of Figure 5. The letters at the bottom show monocot species (Nathalie Colbach, 2022
).
Weed species could be clustered into five groups in terms of their response to tillage suppression (Figure 5B). Group A (in the lower right quadrant of Figure 5B) only consisted of species whose densities increased in the majority of situations (Figure 4): Amaranthus retroflexus (AMARE), Chenopodium album (CHEAL), Digitaria sanguinalis (DIGSA), Solanum nigrum (SOLNI), and Sonchus asper (SONAS). This group became more abundant when tillage was deleted if the recorded cropping systems presented frequent summer tillage (SummerTillage_R in the lower right quadrant of Figure 5A), particularly when implemented with power harrow (nbHROT_R), if mean and maximum tillage depths averaged over the rotation were high (MaxTillageDepth_R, MeanTillageDepth_R) and in systems with few or no efficient large-spectrum herbicides (propSpeciesMortSup80 and propSpeciesMortSup90 in upper left quadrant of Figure 5A). When comparing Figures 5A–C, we could draw generic conclusions on which species features were selected when tillage was deleted. The species of group A emerged during May and June (emergenceMay and emergenceJune in lower right-hand quadrant of Figure 5C) and needed warmer conditions to start photosynthesis. These features are typical of summer annuals.
Figure 5. The weed species (named with EPPO codes) and species traits that are selected when tillage is deleted from recorded cropping systems without compensating practices, depending on the characteristics of the recorded systems. Synthetic representation of the RLQ results with cropping techniques of the recorded cropping systems as matrix R, differences in weed plant densities in tillage-free vs. recorded systems as matrix L, and FLORSYS species parameters as matrix Q. (A) The cropping practices (list in Table A2) of the recorded cropping systems, with the most correlated to the difference in weed densities highlighted in blue based on fourth-corner analysis (green: rotation, brown: tillage, red: herbicides, black: others). (B) Weed species (list in Table A1), clustered into groups, following a Ward ascendant hierarchy classification, based on the difference in tillage-free vs. recorded systems; species labels are colored in red or green if their densities increased or decreased in the majority of situations when tillage was deleted (based on Figure 4). (C) Species parameters (list in Table A3), with the most correlated ones shown in blue (green: morphology and shading response, red: seasonality, brown: seed traits, black: others), based on fourth-corner analysis. Hidden labels: IVdaysBetweenSowing-Harvest_R behind propWinterCrops_R, nbIrrigationObs_R behind TimeHarvest1stTill_R, mu_SLAlate behind mu_WMmid (Colbach, 2022 ).
The species of group C (upper right quadrant of Figure 5B) included dicot weeds such as Polygonum species (POLPE, POLLA, POLAV), which generally became more abundant in case of tillage suppression, as well as grass weeds such as Echinochloa crus-galli (ECHCG) and Panicum miliaceum (PANMI) whose reaction to tillage suppression depended much more on the cropping system (Figure 4). The cropping systems and species traits that favored these species were different from group A in case of tillage suppression. These species increased in rotations with frequent winter crops (propWinterCrops_R and DurationCropCover_R in upper right quadrant of Figure 5A) and rare spring crops (propSpringCrops_R in lower left-hand quadrant), except if wheat was preceded by a spring crop (previousSpringCrop_BLE in the lower left quadrant). These species were also favored if the first tillage of the fallow period and/or mouldboard plowing of the recorded systems occurred soon after the harvest of the previous crop (TimeHarvest1stTill_R and TimeHarvestPlough_R) and summer plowing before wheat was frequent (summerPlough_Wheat), when irrigation was rare and/or rate was low (nbIrrigationOps_R and IrrigationAmount_R), with frequent pseudo-root herbicides (nbPseudoRootHerbicides_R) and in systems frequently switching between years with and without manure spreading (IVnbManureOps_R). In terms of species traits, group C consisted of thick seed coats (seedCoatThickness in the right upper quadrant of Figure 5C) and thus persistent and dormant seeds, as well as plant heights strongly increasing with plant biomass (b_HMlate) and leaves tending to be concentrated toward the top of the plant (b_RLHlate). These species also tended to be spring annuals (emergenceApril).
Group E (lower left quadrant of Figure 5B) was opposed to group C, both in terms of the cropping systems (e.g., late first tillage and/or plow) where these species became more abundant after tillage suppression (Figure 5A), and in terms of species traits (e.g., thin seed coats) that favored this increase in plant densities (Figure 5C). Group E also included a species that increased after tillage suppression in the majority of situations, i.e., Poa annua (POAAN). This species saw its density decrease less in the type of cropping systems associated to group E. But most of its species were favored by tillage suppression, such as Capsella bursa-pastoris (CAPBP), Matricaria perforata (MATIN), and Senecio vulgaris (SENVU).
Group B (on the left-hand side of the first axis of Figure 5B) included both increasing (e.g., GERDI: Geranium dissectum) and decreasing species (e.g., STEME: Stellaria media, Figure 4). Their increase was stronger (or their decease lesser) when the recorded systems included many spring crops (Years Between Spring Crops on the right-hand side of Figure 5A), particularly before wheat (previousSpringCrop_Wheat) and with rare summer plowing before wheat (summerPlough_Wheat). These were winter annuals (Winter Annual of left-hand side of Figure 5C) with a high seed biomass ratio (BMseed_BMair.1.) and a high specific root length (srlMax.mg.g.), but without shading response in terms of specific leaf area and plant width biomass ratio (mu_SLAlate and mu_WMmid on the right-hand side of Figure 5C).
Finally, group D (upper left quadrant of Figure 5B) was opposed to group A, both in terms of systems and trait that favored them when tillage was deleted. These species, e.g., Avena fatua (AVEFA) or Galium aparine (GALAP) generally became less abundant after tillage suppression (Figure 4). Their emergence period did not include May and June, and their photosynthesis started at lower temperatures.
To assess the sensitivity of crop yields to tillage suppression, we analyzed the increase in yield loss in simulations without vs. with tillage as a function of management practices (Table 4). Rotation was crucial. The most sensitive systems were characterized by long-duration crops (e.g., winter crops) or early sown spring crops (combination of lines 4 and 5 with early sowing in Table 4) whereas systems with frequent cover crops (lines 2 and 3) or summer crops (lines 4 and 5 with late sowing) were less sensitive to tillage suppression. The same was true for systems with efficient herbicide programs, e.g., programs varying between years (line 6). However, non-systemic herbicides (whose efficiency decreases with weed density) work better in conjunction with other weed-control options such as tillage. Conversely, persistent herbicides, such as pseudo-root herbicides work better if they are not followed by tillage (line 8) as the latter dilutes their concentration in the soil. These herbicides are also applied before or at crop sowing, often replacing pre-sowing tillage, and the systems that include these herbicides are thus less sensitive to tillage suppression. Similarly, the beneficial effect of (weed-free) manure spreading (which indirectly increases soil contact of surface seeds when organic matter is spread on soil surface and thus weed seedbank depletion during fallow) lasts longer when tillage is deleted (line 9). The same applies to a lesser degree to irrigation (line 10).
Table 4. Effect of management practices on the resilience (variation in yield loss) of cropping systems to tillage suppression.
In the recorded no-till systems and the tillage-free systems (resulting from deleting tillage in the recorded systems), grain yield loss depended the most on herbicides (partial R2 = 0.39, details in Section C.4 in Supplementary Material) as well as rotation (R2 = 0.12), including cover crops during seasonal fallow (R2 = 0.14). Mechanical weeding (R2 = 0.06), sowing (R2 = 0.07), harvest (R2 = 0.04) and mowing/shredding/topping operations (0.06) had a much smaller effect whereas the effect of manure (R2 = 0.01), rolling operations (R2 = 0) and irrigation (R2 = 0.03) was nil or negligible. The three best types of systems, i.e., the three types with the lowest grain yield loss due to weeds on average over the rotation, all relied on herbicides, but to a varying degree (Table 5).
Table 5. The three best strategies for limiting grain yield loss due to weed at the rotation scale (i.e., yield loss averaged over 30 simulated years) in no-till systems, based on a classification and regression tree on 466 systems × 10 weather repetitions (395 systems from the “No till” series, 2 × 36 no-till systems from the “recorded” and “herbicide-free” series).
Among these, strategy A consisted of only recorded cropping systems. It presented the highest herbicide use intensity (HTFI = 2.49 on average over rotation), requiring herbicides targeting monocot weeds, and preferring a high efficiency over frequency of application and over high dosage, particularly in wheat (where HTFI could be low). The other weed control lever was crop-residue shredding during fallow, which needed to be implemented a few days after harvest at the earliest and cut as low as possible. A high crop sowing density was also helpful. All these rules were particularly important in wheat. The rotation included winter crops and/or spring-crops preceded by cover crops.
Strategy B was very different, comprising only systems from the “No-till” series. It consisted of a summer-crop monoculture, mainly late-sown and late-harvested maize (with glyphosate-tolerant maize in 50% of the systems) preceded by a cover crop during fallow. Frequency of herbicide applications and HTFI were lower but spraying needed to be during the first month after sowing. A very high irrigation was essential, starting during the first 6 weeks of the crop, and occasional crop-residue shredding during fallow. This strategy was mostly found in South-Western France.
Strategy C also only comprised systems from the “No-till” series. It differed from the other two, using few and less efficient herbicides, except for pseudo-root herbicides at crop sowing. Cover crops (lasting at least for 2 months), well timed residue shredding before sunflower and frequency manure spreading were also essential. The latter added a small layer of organic matter on top of weed seeds located on the soil surface, thus improving the latter's imbibition and germination during fallow, which resulted in a smaller weed seed bank at cash crop sowing.
As the previous no-till systems often relied on intense herbicide use, our last analysis aimed to identify management techniques and combinations thereof that reduce all three indicators, i.e., grain yield loss due to weeds, herbicide use intensity and tillage frequency. In the 3 simulation series ×395 investigated systems × 10 weather repetitions, rotation was the most important factor (Table 6). The larger the number of different crops and varieties grown over time, the more our three target indicators were reduced (line [1] of Table 6) and if the crop cover (resulting from both cover and cash crops) lasted long [4] (particularly in maize [26]) and this each year (i.e., cover duration should vary little from year to year, [5]). More in detail, this meant (i) frequent spring crops, particularly maize [7], (ii) cover crops, particularly long-lasting ones [11], which required early-harvested winter crops [3] except in wheat [18]; and/or (iii) double crops in the rotation [9] and therefore few winter crops [10]. Long rotations [15] alternating winter, spring and/or multiannual crops [20] were the best. In a rotation, wheat should follow cereals or oilseed crops [23] rather than legumes and other crop species. Sowing strategies were also important. Early sowing of both winter [2] (particularly in wheat) and spring crops [6] (particularly in maize) was best. Moreover, wheat should be sown in narrow rows [13] and [25].
Table 6. The main cropping-system components (other than those related to tillage and herbicide strategies) that are associated with a reduction in tillage, in herbicide use intensity and/or in grain yield loss due to weeds. Results from multi-variate classification and regression tree analyzing grain yield loss, number of tillage operations per year and herbicide treatment frequency as a function of cropping system characteristics using all three simulation series (with recorded systems, after tillage deletion, after herbicide deletion), with partial R2 calculated from Variable Importance Predictors and showing the probability that an increase in a cropping-system variable is associated to an increase in the mean of the three response variables [normed to (0 = lowest value in data set, 1 = highest value in data set)]. Cropping-system variables with a higher-than-average probability of increased response variable are shaded. The lower the partial R2 was, the more the effect of a variable was local (i.e., it depended on other cropping system variables). Variable colors distinguish rotation (green), mechanical weeding (brown), sowing (purple), crop-residue shredding, mowing and topping (blue), and other (black). The complete table can be found in Section The cropping practices that drive grain yield loss in no-till systems in Supplementary Material.
Weed destroying operations (other than tillage or herbicides) were crucial. The target indicators were reduced if a large field proportion was mechanically weeded [12] (i.e., tools weeding the whole field rather than just the interrow are better) though frequent mechanical weeding had the opposite effect [22] as it can stimulate new weed emergence flushes. Similarly, delaying mowing and topping (i.e., cutting weed plants approximately at the height of the crop canopy to avoid weed seed production) date to leave enough time to the weeds to be grow above the cutting height (i.e., operating close to harvest) was much more important [24] than to take action in each crop (i.e., the frequency of crops with mowing or topping increased the triple target [17]). Conversely, frequent crop-residue shredding during the fallow period was helpful [19] though in no-till, this technique would necessarily be limited to a single operation aiming to destroy the cover-crop canopy. Frequent rolling was also a good idea [8]. Two other management techniques influenced our target. Irrigation, particularly in maize [14] and [27], was counterproductive and was best carried out as late as possible after sowing [16] to limit weed emergence. Similarly, (weed-free) manure spreading increased our target indicators, particularly before wheat [21]. This was not because manure boosted weed infestation, on the contrary as shown in Tables 4, 5. This was because in recorded systems, manure spreading is usually followed sooner or later by tillage.
Among the techniques that are impossible to implement in no-till herbicide-free systems, but still possible in reduced-tillage and reduced-herbicide systems we found that some features (other than number of operations and dosage) of herbicide programs also contributed to reducing the three target indicators (e.g., broad-spectrum herbicides, highly efficient herbicides, early spraying after sowing, Section C4 in Supplementary Material). Similarly, well-chosen tillage options helped to reduce the target indicators (e.g., till as close as possible to crop sowing).
The effects of changing cropping-system variables were not always straightforward, i.e., increasing a given variable could lead to both an increase and a decrease in the target indicators, depending on other cropping-system variables (Table 6). For instance, increasing the number of irrigations per year on average over the rotation increased the target indicators in 40% of situations and decreased it in 60% of situations (line [14] in Table 6). To further pinpoint “winning” cropping systems, we analyzed the four strategies that presented both the lowest tillage and herbicide intensities (Table 7). The resulting yield losses due to weeds (27–59%) were not low in absolute values but much lower than most no-till systems and still below average of no-herbicide systems (Figure 3). Note that absolute yield-loss values can be higher than what is commonly reported in fields because management operations were not adapted to simulated weed flora and weather in the simulations (Sections Domain of Validity and Simulation Plan).
Table 7. How to reconcile reduced tillage, reduced herbicide use intensity, and reduced grain yield loss due to weeds? The four strategies with herbicide use intensity < 0.15 and tillage frequency < 0.2 operations per year. Results from multi-variate classification and regression tree analyzing grain yield loss, number of tillage operations per year and herbicide treatment frequency as a function of cropping-system characteristics, with partial R2 calculated from Variable Importance Predictors and showing the probability that an increase in a cropping-system variable leads to an increase in the mean of the three response variables [normed to (0 = lowest value in data set, 1 = highest value in data set)]. Cell colors distinguish variables in relation to rotation (green), tillage/mechanical weeding (yellow), herbicides (red), sowing (purple), crop-residue shredding, mowing and topping (blue), and other (white). All values are averages over rotation.
Strategy S1 presented the lowest yield loss (27%, Table 7). Its spring crops were sown late; if maize was tilled, this was done close to sowing. Irrigation, particularly in maize, was limited in terms of frequency and rate. The same applied to manure spreading, particularly prior to wheat. Winter crops were sown before 3 November and mechanical weeding was rare. There were some herbicides and to limit treatment frequency, herbicides were highly efficient (notably preferring systemic and multi-entry herbicides), particularly on monocot weeds.
Strategy S2 presented some similarities but resulted in more than twice the yield loss (59%). The main differences concerned irrigation, with high frequencies and large amounts. Moreover, crop cover lasted less than 11.3 months (which excludes multiannual grass/lucerne crops or relay cropping), and years with no-till frequently alternated with years with tilled crops.
The two other strategies were very different, with earlier sown spring crops. The emphasis of strategy S3 was on late-sown and late-harvested winter crops with well-chosen herbicides. Despite allowing some tillage and herbicides, its grain yield loss was much higher than that of strategy S4 (59 vs. 41%), which used neither tillage nor herbicides. The latter consisted of short rotations with many early sown winter crops (but no wheat after cereals), but no cover crops or double.
We combined cropping-system data collected from farming practice with a simulation model playing the role of a virtual experimental field and a simulation plan aiming to decorrelate key weed-management levers. This approach was previously used to identify yield-limiting factors (Affholder et al., 2003, 2013; van Ittersum et al., 2003; Silva et al., 2017) and more recently to assess the effects of herbicide use intensity on weeds (Colbach and Cordeau, 2018). To our knowledge, the present study is the first attempt to apply this methodology to tillage intensity and the consequences for weeds, aiming to identify sustainable levers to reach the holy grail: herbicide-free, no-till and low yield loss due to weeds.
Combining field data with simulations allowed us to overcome major drawbacks of field experiments and farm-field surveys. Evaluating the cropping systems with a simulation model made possible a comprehensive multi-annual and multi-weather assessment, and this for a several hundred systems and a dozen contrasting pedoclimates and weed floras. The systems provided by farmers and advisors were essential to investigate the role of tillage in consistent systems whose components were chosen to be complementary whereas the “tillage-free” and “herbicide-free” simulation series allowed disentangling the role of the two major weed-management options from each other and from other management practices. The latter is possible in field experiment but takes time, and impossible in farm-field surveys as farmers compensate reduced tillage and/or reduced herbicide use by other practices, which can lead to wrong conclusions when simply correlating management intensities with weed-flora or yield data (Colbach et al., 2020b).
Using a mechanistic (process-based) simulation model was essential to understand the reasons for the observed cropping-system performances and tillage effects and to access detailed information on crops, weeds and soil which are impossible to measure in actual fields (Colbach, 2010, 2022). Working with what is essentially a virtual field made it possible to apply agronomic and ecological methods initially developed for cropping-system diagnosis and field surveys (Doledec et al., 1996; Doré et al., 2008). As a result, we produced emerging knowledge, e.g., which weed traits are selected by tillage or no-till as well as rules for designing sustainable no-till systems (see Section Implications for Conservation Agriculture).
The main drawback of simulation-based studies is that the results are conditional on the prediction quality of the model. In the case of FLORSYS, this was shown to be adequate in a previous study (Section Domain of Validity). Here, we focused on tillage-related observations.
It is impossible to find field observations that compare cropping systems that only differ in terms of presence and absence of tillage (as in our “recorded” and “tillage-free” simulation series). But, some field studies observed similar tendencies to our simulation study, e.g., an increase in spring/summer emerging species (as in our groups A and C of Figure 5 favored by tillage suppression) or shade-affiliated species (the opposite of the disfavoured group B) with the time since the adoption of conservation agriculture (Derrouch et al., 2021). Process-close traits were more influential in our analysis than broader features such as clade, even though monocot species are frequently reported to increase in conservation agriculture (Derrouch et al., 2021). Here, monocot species were both favored and disfavoured by tillage suppression, depending on their other traits, particularly emergence season.
Other field reports could not be confirmed, simply because the relevant species were not included in the FLORSYS model. This is the case of perennial weeds, which often increase of adopting conservation agriculture (Zanin et al., 1997; Derrouch et al., 2021). Another limit of our study is related to missing processes. For instance, pseudo-root herbicides worked better here without tillage whereas in practice, farmers implementing conservation agriculture use few such herbicides whose efficacy is expected to be reduced by the higher organic-matter content on the untilled soil surface (Locke et al., 2002; Derrouch et al., 2020), a process not included in FLORSYS. This means that the here identified increase in weed biomass and weed-caused yield loss might be either over- or underestimated and that potential levers for making no-till systems “weed-proof” need to focus more on perennials and biological weed regulation.
Other differences with the literature might result from the data set. Indeed, our very large data set allowed us to show that the effect of tillage suppression on weeds depended on the initial cropping systems. This would explain some contradictory reports from literature, concluding both an increase (Derrouch et al., 2021) and a decrease of species with large/heavy seeds (Thomas et al., 2004; Trichard et al., 2013; Armengot et al., 2016).
Moreover, we compared recorded systems to systems where tillage was deleted without other changes in management practices. In practice, tillage suppression comes with a complete system overhaul, including many other changes in management practices which might cause weed-flora shifts attributed to no-till. For instance, no-till often comes with increased crop-residue biomass on soil surface which favors species with smaller seeds (Menalled et al., submitted1). Despite our simulation plan and the diversity of the analyzed systems, such correlations could also impact some of our results. For instance, reducing manure spreading was identified here (Table 7) as a potential lever to reach our triple target (reduced herbicide and tillage intensities and yield loss) because it increased weed seed-soil contact and the resulting weed-seedbank depletion during fallow. But this might simply be because in the recorded cropping systems, tillage was used to incorporate manure. In untilled systems, weed-free manure spreading could actually reduce weed-caused yield loss as shown in Table 5. This beneficial effect could though be canceled out if the manure comprised viable weed seeds (Miyazawa et al., 2004; Cordeau et al., 2021) and it might be overestimated as no such reduction was ever reported in fields. Field studies report little or no impact of manure spreading (Menalled et al., 2005; De Cauwer et al., 2011) or significant increase in weed infestation (Miyazawa et al., 2004) due to weed-seed import (which was neglected here). But these field reports did not investigate weed seed germination, and manure spreading came with other modifications in the agroecosystem, notably nitrogen dynamics (which were not issue here as nitrogen was non-limiting in our simulations).
The present study showed that recorded cropping systems frequently present a trade-off between no-till and no herbicides, as in other studies (Lechenet et al., 2017; Yvoz et al., 2020). To date, farmers, advisors and experimenters do not imagine systems that are both tillage-free and herbicide-free. On the contrary, in our recorded systems, farmers tended to rely more on tillage and herbicides, as they are risk-adverse (Wilson et al., 2008; Jabbour et al., 2014), particularly in terms of weed management where they tend to “over-insure” to avoid any long-term problems (Gibson et al., 2006).
There were no recorded systems without herbicides and without tillage. All the best no-till systems identified here relied heavily on herbicides. The untilled crops with the lowest herbicide use intensity were unusual for arable-crop rotations, i.e., relay grass crops or multi-annual crops. This reminds us that conservation agriculture became possible in mechanized agriculture only once non-selective foliar herbicides which also targeted perennials became available (Evans, 1972).
Yield loss was not correlated to tillage frequency and/or intensity. This is not surprising as farmers adapt their practices when reducing tillage intensity, similarly to what we showed for herbicide intensity in a previous paper (Colbach and Cordeau, 2018). But tillage effects are not as straightforward as herbicide effects (Cordeau et al., 2017a). Tillage is essential to destroy weed plants prior to cash crop sowing to reduce weed emergence by burying seeds (Mulugeta and Stoltenbert, 1997), and to empty the weed seed bank through stale seed bed operations (Lamour and Lotz, 2007; Riemens et al., 2007; Travlos et al., 2020). But the germinating-favoring effect of tillage can be disastrous when it occurs close to cash-crop sowing and has not been preceded by stale seed bed operations, leading to an increase in weed emergence in the cash crop (Cordeau et al., 2017b).
Achieving low weed harmfulness in either herbicide-free or no-till systems was possible, which has already been reported in previous simulation studies (Colbach and Cordeau, 2018) and farm-field surveys (Mohler et al., 2018; Adeux et al., 2019). This does not mean that tillage does not control weeds. On the contrary, deleting tillage without compensatory measures resulted in an even huger increase in weed infestation and yield loss than when herbicides are deleted without compensation. This is consistent with field reports showing that tillage suppression tends to homogenize the ecological niche of weeds, providing them with a monotonous and relatively undisturbed habitat (Derrouch et al., 2021).
Completely eliminating the two major weed-management levers at the same time was impossible in the situations investigated here. Unsurprisingly, the higher the initial tillage frequency is in a cropping system, the more the cropping system needs to be redesigned to compensate for tillage suppression. This highlights what pioneer farmers found when transitioning their system from plow-based to conservation agriculture: tillage had to be reduced step by step (Derrouch et al., 2020; Shrestha et al., 2020), first removing plowing, then reducing tillage intensity as much as possible, and finally switching to no-till. This allows a more gradual shift of the weed community (Derrouch et al., 2021) and decreases the role of the buried weed seed bank in the regeneration of weed populations in subsequent years (Chauhan et al., 2012; Cordeau et al., 2015). Participatory workshops with farmers and other experts are a way to design such transitions, setting off either with organic systems and gradually reducing tillage to achieve no-till, or vice-versa with conventional conservation agriculture and gradually reducing herbicides. The most disruptive approach would consist in designing no-till herbicide-free systems from scratch, also including landscape infrastructure aiming to promote biological regulation (Petit et al., 2021). The resulting systems can then be tested either (or both) in cropping-systems trials or in simulations. But even systems designed by multiple experts do not necessarily succeed as shown by INRAE cropping-system trials. There, the herbicide-free tillage-free systems have been infested by weeds, notably grass weeds, in only 3 years.
We identified avenues to limit weed-caused yield in systems with reduced tillage and reduced herbicide. These avenues are potentially interesting for both conservation agriculture and organic farming, the latter now also trying to limit tillage to protect soils from erosion (Rhodes, 2017; Schreefel et al., 2020). Our results are consistent with what pioneer farmers learn from their transition phase toward conservation agriculture, such as the need for diversified crop rotations with different sowing periods in addition to a continuous crop cover (Derrouch et al., 2020). Many of these levers are also common to ecological weed management (Bastiaans et al., 2008), stressing that sustainable no-till no-herbicide systems require a good understanding of weed ecology and weed response to biological levers (Petit et al., 2018). However, the solutions identified here are few, they are feasible in only some production situations and may have other disadvantages, e.g., maize monoculture is limited to certain regions (e.g., South-Western France, Alsace), grassland-based systems focus on biomass production to the detriment of grain and protein production (Huyghe and Tabel, 2010). Some options are impossible in no-till systems, such as mechanical weeding with a harrow and similar tools (because of the presence of crop residues) or reduced row spacing (because most no-till drillers require about 17 cm between each rows). Our results highlight that crop rotation and cover cropping are the key to design no-till herbicide-free systems but that we must think beyond current rotation patterns and ways of introducing cover crops into cropping systems.
The simulation plan used here must be amended to disentangle the many correlations among management practices and to design no-till (and herbicide-free) systems for specific production conditions and farmers' constraints. For the first part, we need to survey contrasting no-till cropping systems practiced by farmers in different pedoclimates and add them to our simulated data base. This will allow identifying more generic and robust rules for designing sustainable no-till herbicide-free systems. These rules can then be used in participatory workshops with farmers aiming to design systems adapted to their particular context. We used this approach already in the past for sustainable low-herbicide systems (Cavan et al., 2020; Colbach et al., 2021; Cavan et al., in review2).
Our study showed that deleting the two major weed-control levers without compensation is disastrous. If we do so, systems need to be completely redesigned since there is no chance that changing the cropping systems through a substitution approach (Hill and MacRae, 1996) would succeed. Our results suggest that to achieve the holy grail of herbicide-free no-till systems providing enough foods with limited yield loss, systems must be redesigned in depth, probably going beyond what is actually known, practiced or feasible. This will also need a major effort in terms of crop breeding, particularly the need to breed new cover crop species mixtures that are able to outcompete weeds (Bybee-Finley et al., 2022), easier to terminate without glyphosate (Alonso-Ayuso et al., 2020) while limiting costs or damage to the environment (Frasconi et al., 2019).
The raw data supporting the conclusions of this article will be made available by the authors, without undue reservation.
NC and SC designed the research. NC ran the simulations, analyzed the data, and wrote the draft manuscript. SC contributed to the article and approved the submitted version. Both authors contributed to the article and approved the submitted version.
This project was supported by INRAE, the French Projects CoSAC (ANR-15-CE18-0007) and COPRAA which was supported by OFB as part of the Ecophyto call on Global approaches to limit the use of phytopharmaceuticals: Combining preventive and curative products in sectors, from farmers to consumers launched by the French Ministries in charge of Ecology, Agriculture, Health and Research, as well as the European Union's Horizon 2020 Research and Innovation Program under Grant Agreements No. 727321 (IWMPRAISE project), the Casdar ENGAGED (Weed Management in conservation agriculture without glyphosate) financed by the French Ministry in charge of Agriculture and Food (Ministère de l'Agriculture et de l'Alimentation), with financial contributions from the special purpose account (Développement agricole et rural), and ANR PPR SPECIFICS Project (ANR-20-PCPA-0008).
The authors declare that the research was conducted in the absence of any commercial or financial relationships that could be construed as a potential conflict of interest.
All claims expressed in this article are solely those of the authors and do not necessarily represent those of their affiliated organizations, or those of the publisher, the editors and the reviewers. Any product that may be evaluated in this article, or claim that may be made by its manufacturer, is not guaranteed or endorsed by the publisher.
The Supplementary Material for this article can be found online at: https://www.frontiersin.org/articles/10.3389/fagro.2022.823069/full#supplementary-material
1. ^Menalled, U. D., Adeux, G., Cordeau, S., Smith, R. G., Mirsky, S. B., and Ryan, M. R. S Cereal rye mulch biomass and crop density affect weed suppression and community assembly in no-till planted soybean: A species and trait-based analysis. Ecol. Appl. submitted.
2. ^Cavan, N., Omon, B., Dubois, S., Toqué, C., Van Inghelandt, B., Wilfried, Q., et al. (in review) In silico Evaluation of Three Approaches for Cropping-System Design in Terms of Farmer Implication, Weed Management and Overall Sustainability. Agricultural Systems, submitted.
Adeux, G., Munier-Jolain, N., Meunier, D., Farcy, P., Carlesi, S., Barberi, P., et al. (2019). Diversified grain-based cropping systems provide long-term weed control while limiting herbicide use and yield losses. Agron. Sust. Dev. 39, 42. doi: 10.1007/s13593-019-0587-x
Affholder, F., Poeydebat, C., Corbeels, M., Scopel, E., and Tittonell, P. (2013). The yield gap of major food crops in family agriculture in the tropics: assessment and analysis through field surveys and modelling. Field Crops Res. 143, 106–118. doi: 10.1016/j.fcr.2012.10.021
Affholder, F., Scopel, E., Madeira Neto, J., and Capillon, A. (2003). Diagnosis of the productivity gap using a crop model. Methodology and case study of small-scale maize production in central Brazil. Agronomie 23, 305–325. doi: 10.1051/agro:2003004
Alletru, C., and Labreuche, J. (2019). “Impact du travail du sol et des couverts vegetaux en interculture sur la gestion des adventices en grandes cultures,” in Végéphyl – 24e Conférence du columa journées internationales sur la lutte contre les mauvaises herbes. Orléans, France.
Alonso-Ayuso, M., Gabriel, J. L., Hontoria, C., Ibanez, M. A., and Quemada, M. (2020). The cover crop termination choice to designing sustainable cropping systems. Eur. J. Agron. 114, 126000. doi: 10.1016/j.eja.2020.126000
Armengot, L., Blanco-Moreno, J. M., Bàrberi, P., Bocci, G., Carlesi, S., Aendekerk, R., et al. (2016). Tillage as a driver of change in weed communities: a functional perspective. Agric. Ecosyst. Environ. 222, 276–285. doi: 10.1016/j.agee.2016.02.021
Barbercheck, M. E., and Wallace, J. (2021). Weed–insect interactions in annual cropping systems. Ann. Entomol. Soc. Am. 114, 276–291. doi: 10.1093/aesa/saab002
Bastiaans, L., Paolini, R., and Baumann, D. T. (2008). Focus on ecological weed management: what is hindering adoption? Weed Res. 48, 481–91. doi: 10.1111/j.1365-3180.2008.00662.x
Blaix, C., Moonen, A. C., Dostatny, D. F., Izquierdo, J., Le Corff, J., Morrison, J., et al. (2018). Quantification of regulating ecosystem services provided by weeds in annual cropping systems using a systematic map approach. Weed Res. 58, 151–164. doi: 10.1111/wre.12303
Breiman, L., Friedman, J. H., Olshen, R. A., and Stone, C. J. (1984). Classification and Regression Trees. New York, NY: Chapman and Hall (Wadswoth, Inc.).
Bürger, J., Granger, S., Guyot, S. H. M., Messéan, A., and Colbach, N. (2015). Simulation study of the impact of changed cropping practices in conventional and GM maize on weeds and associated biodiversity. Agric. Syst. 137, 51–63. doi: 10.1016/j.agsy.2015.03.009
Bybee-Finley, K. A., Cordeau, S., Yvoz, S., Mirsky, S. B., and Ryan, M. R. (2022). Finding the right mix: a framework for selecting seeding rates for cover crop mixtures. Ecol. Appl. 32:e02484. doi: 10.1002/eap.2484
Cavan, N., Omon, B., Colbach, N., and Angevin, F. (2019). Utilisation du modèle FLORSYS comme outil d'aide à la conception de systémes de culture innovants performants pour la gestion durable des adventices : exemple d'un groupe DEPHY Ferme de l'Eure. Agronomie, Environnement & Société 9, 131–144. Available online at: https://agronomie.asso.fr/aes-9-2-15
Cavan, N., Omon, B., Tailleur, A., Dubois, S., Queyrel, W., Van Inghelandt, B., et al. (2020). Comparaison de méthodes de conception de systèmes de culture innovants pour la gestion durable des adventices. Innov. Agronom. 81, 189–200.
Chantre, G. R., and González-Andújar, J. L. eds. (2020). Decision Support Systems for Weed Management. Cham: Springer International Publishing.
Chauhan, B. S., Singh, R. G., and Mahajan, G. (2012). Ecology and management of weeds under conservation agriculture: a review. Crop Prot. 38, 57–65. doi: 10.1016/j.cropro.2012.03.010
Chessel, D., Dufour, A. B., and Thioulouse, J. (2004). The ade4 package. I. One-table method. R News 4, 5–10. Available online at: https://hal.archives-ouvertes.fr/hal-00427558
Cohen, R. (2006). Introducing the GLMSELECT PROCEDURE for Model Selection. Available online at: http://www2.sas.com/proceedings/sugi31/207-31.pdf (accessed February 25, 2022).
Colbach, N. (2010). Modelling cropping system effects on crop pest dynamics: how to compromise between process analysis and decision aid. Plant Sci. 179, 1–13. doi: 10.1016/j.plantsci.2010.04.009
Colbach, N. (2022). “Modelling the effects of cropping systems on weed dynamics: the trade-off between process analysis and decision support,” in Advances in Integrated Weed Management, ed P. Kudsk (Cambridge: Burleigh Dodds Science Publishing). doi: 10.19103/AS.2021.0098.07
Colbach, N., Bertrand, M., Busset, H., Colas, F., Dugué, F., Farcy, P., et al. (2016). Uncertainty analysis and evaluation of a complex, multi-specific weed dynamics model with diverse and incomplete data sets. Environ. Modell. Software 86, 184–203. doi: 10.1016/j.envsoft.2016.09.020
Colbach, N., Bockstaller, C., Colas, F., Gibot-Leclerc, S., Moreau, D., Pointurier, O., et al. (2017a). Assessing broomrape risk due to weeds in cropping systems with an indicator based on a simulation model. Ecol. Indic. 82, 280–292. doi: 10.1016/j.ecolind.2017.05.070
Colbach, N., Busset, H., Roger-Estrade, J., and Caneill, J. (2014a). Predictive modelling of weed seed movement in response to superficial tillage tools. Soil Tillage Res. 138, 1–8. doi: 10.1016/j.still.2013.12.002
Colbach, N., Chauvel, B., Messéan, A., Villerd, J., and Bockstaller, C. (2020a). Feeding pollinators from weeds could promote pollen allergy. A simulation study. Ecol. Indic. 117, 106635. doi: 10.1016/j.ecolind.2020.106635
Colbach, N., Colas, F., Cordeau, S., Maillot, T., Queyrel, W., Villerd, J., et al. (2021). The FLORSYS crop-weed canopy model, a tool to investigate and promote agroecological weed management. Field Crops Res. 261, 108006. doi: 10.1016/j.fcr.2020.108006
Colbach, N., Colas, F., Pointurier, O., Queyrel, W., and Villerd, J. (2017b). A methodology for multi-objective cropping system design based on simulations. Application to weed management. Eur. J. Agron. 87, 59–73. doi: 10.1016/j.eja.2017.04.005
Colbach, N., Collard, A., Guyot, S. H. M., Mézière, D., and Munier-Jolain, N. M. (2014b). Assessing innovative sowing patterns for integrated weed management with a 3D crop:weed competition model. Eur. J. Agron. 53, 74–89. doi: 10.1016/j.eja.2013.09.019
Colbach, N., and Cordeau, S. (2018). Reduced herbicide use does not increase crop yield loss if it is compensated by alternative preventive and curative measures. Eur. J. Agron. 94, 67–78. doi: 10.1016/j.eja.2017.12.008
Colbach, N., Fernier, A., Le Corre, V., Messéan, A., and Darmency, H. (2017c). Simulating changes in cropping practices in conventional and glyphosate-resistant maize. I. Effects on weeds. Environ. Sci. Pollut. Res. 24, 11582–11600. doi: 10.1007/s11356-017-8591-7
Colbach, N., Granger, S., Guyot, S. H. M., and Mézière, D. (2014c). A trait-based approach to explain weed species response to agricultural practices in a simulation study with a cropping system model. Agricul. Ecosyst. Environ. 183, 197–204. doi: 10.1016/j.agee.2013.11.013
Colbach, N., Petit, S., Chauvel, B., Deytieux, V., Lechenet, M., Munier-Jolain, N., et al. (2020b). The pitfalls of relating weeds, herbicide use, and crop yield: don't fall into the trap! A critical review. Front. Agron. 2, e615470. doi: 10.3389/fagro.2020.615470
Colbach, N., Schneider, A., Ballot, R., and Vivier, C. (2010). Diversifying cereal-based rotations to improve weed control. Evaluation with the ALOMYSYS model quantifying the effect of cropping systems on a grass weed. OCL 17, 292–300. doi: 10.1051/ocl.2010.0331
Cordeau, S., Baudron, A., and Adeux, G. (2020). Is tillage a suitable option for weed management in conservation agriculture? Agronomy 10, 1746. doi: 10.3390/agronomy10111746
Cordeau, S., Guillemin, J. P., Reibel, C., and Chauvel, B. (2015). Weed species differ in their ability to emerge in no-till systems that include cover crops. Ann. Appl. Biol. 166, 444–455. doi: 10.1111/aab.12195
Cordeau, S., Smith, R. G., Gallandt, E. R., Brown, B., Salon, P., DiTommaso, A., et al. (2017a). Disentangling the effects of tillage timing and weather on weed community assembly. Agriculture-Basel 7, 66. doi: 10.3390/agriculture7080066
Cordeau, S., Smith, R. G., Gallandt, E. R., Brown, B., Salon, P., DiTommaso, A., et al. (2017b). Timing of tillage as a driver of weed communities. Weed Sci. 65, 504–514. doi: 10.1017/wsc.2017.26
Cordeau, S., Wayman, S., Ketterings, Q. M., Pelzer, C. J., Sadeghpour, A., and Ryan, M. R. (2021). Long-term soil nutrient management affects taxonomic and functional weed community composition and structure. Front. Agron. 3, e636179. doi: 10.3389/fagro.2021.636179
De Cauwer, B., D'Hose, T., Cougnon, M., Leroy, B., Bulcke, R., and Reheul, D. (2011). Impact of the quality of organic amendments on size and composition of the weed seed bank. Weed Res. 51, 250–260. doi: 10.1111/j.1365-3180.2010.00840.x
De'ath, G., Therneau, T. M., Atkinson, B., Ripley, B., and Oksanen, J. (2014). Mvpart: Multivariate Partitioning. R package. Available online at: https://CRAN.R-project.org/package=mvpart
Délye, C., Colbach, N., and LeCorre, V. (2020). Résistances aux herbicides : mécanismes, situation en France et bonnes pratiques. Innovations Agronomiques 81, 33–49. doi: 10.15454/8j8h-6610
Derrouch, D., Chauvel, B., Felten, E., and Dessaint, F. (2020). Weed management in the transition to conservation agriculture: farmers' response. Agronomy-Basel 10, 13. doi: 10.3390/agronomy10060843
Derrouch, D., Chauvel, B. S. C., and Dessaint, F. (2021). Functional shifts in weed community composition following adoption of conservation agriculture. Weed Res. in press. doi: 10.1111/wre.12517
Doledec, S., Chessel, D., terBraak, C. J. F., and Champely, S. (1996). Matching species traits to environmental variables: a new three-table ordination method. Environ. Ecol. Stat. 3, 143–166. doi: 10.1007/BF02427859
Doré, T., Clermont-Dauphin, C., Crozat, Y., David, C., Jeuffroy, M. H., Loyce, C., et al. (2008). Methodological progress in on-farm regional agronomic diagnosis. A review. Agron. Sustain. Dev. 28, 151–161. doi: 10.1051/agro:2007031
Evans, D. M. (1972). “Field performance of glyphosate derivatives in the control of Agropyron repens and other perennial weeds,” in British Weed Control Conference (Brighton), 64–70
Frasconi, C., Martelloni, L., Antichi, D., Raffaelli, M., Fontanelli, M., Peruzzi, A., et al. (2019). Combining roller crimpers and flaming for the termination of cover crops in herbicide-free no-till cropping systems. PLoS ONE 14, e0211573. doi: 10.1371/journal.pone.0211573
Fried, G., Norton, L. R., and Reboud, X. (2008). Environmental and management factors determining weed species composition and diversity in France. Agricu. Ecosyst. Environ. 128, 68–76. doi: 10.1016/j.agee.2008.05.003
Friedrich, T. (2005). Does no-till farming require more herbicides? Outlooks Pest Manag. 16, 188–191. doi: 10.1564/16aug12
Gardarin, A., Dürr, C., and Colbach, N. (2012). Modeling the dynamics and emergence of a multispecies weed seed bank with species traits. Ecol. Model. 240, 123–138. doi: 10.1016/j.ecolmodel.2012.05.004
Gibson, K., Johnson, W., and Hillger, D. (2006). Farmer perceptions of weed problems in corn and soybean rotation systems. Weed Technol. 20, 751–755. doi: 10.1614/WT-05-089R1.1
Hill, S. B., and MacRae, R. J. (1996). Conceptual framework for the transition from conventional to sustainable agriculture. J. Sustain. Agric. 7, 81–87. doi: 10.1300/J064v07n01_07
Hiltbrunner, J., Scherrer, C., Streit, B., Jeanneret, P., Zihlmann, U., and Tschachtli, R. (2008). Long-term weed community dynamics in Swiss organic and integrated farming systems. Weed Res. 48, 360–369. doi: 10.1111/j.1365-3180.2008.00639.x
Hobbs, P. R., Sayre, K., and Gupta, R. (2008). The role of conservation agriculture in sustainable agriculture. Phil. Trans. R. Soc. B Biol. Sci. 363, 543–555. doi: 10.1098/rstb.2007.2169
Huyghe, C., and Tabel, C. (2010). “Grasslands and forage crops in france: Context and stakes consequences for breeding,” in Proceedings of Conference of the Eucarpia Fodder and Amenity Species Section (La Rochelle), 3–13.
Jabbour, R., Gallandt, E. R., Zwickle, S., Wilson, R. S., and Doohan, D. (2014). Organic farmer knowledge and perceptions are associated with on-farm weed seedbank densities in Northern New England. Weed Sci. 62, 338–349. doi: 10.1614/WS-D-13-00098.1
Kudsk, P., and Mathiassen, S. K. (2020). Pesticide regulation in the European Union and the glyphosate controversy. Weed Sci. 68, 214–222. doi: 10.1017/wsc.2019.59
Lamour, A., and Lotz, L. A. P. (2007). The importance of tillage depth in relation to seedling emergence in stale seedbeds. Ecol. Model. 201, 536–546. doi: 10.1016/j.ecolmodel.2006.10.015
Lechenet, M., Dessaint, F., Py, G., Makowski, D., and Munier-Jolain, N. (2017). Reducing pesticide use while preserving crop productivity and profitability on arable farms. Nat. Plants 3, 17008. doi: 10.1038/nplants.2017.8
Locke, M. A., Reddy, K. N., and Zablotowicz, R. M. (2002). Weed management in conservation crop production systems. Weed Biol. Manag. 2, 123–132. doi: 10.1046/j.1445-6664.2002.00061.x
Menalled, F. D., Kohler, K. A., Buhler, D. D., and Liebman, M. (2005). Effects of composted swine manure on weed seedbank. Agric. Ecosyst. Environ. 111, 63–69. doi: 10.1016/j.agee.2005.04.027
Mézière, D., Petit, S., Granger, S., Biju-Duval, L., and Colbach, N. (2015). Developing a set of simulation-based indicators to assess harmfulness and contribution to biodiversity of weed communities in cropping systems. Ecol. Indicat. 48, 157–170. doi: 10.1016/j.ecolind.2014.07.028
Miyazawa, K., Tsuji, H., Yamagata, M., Nakano, H., and Nakamoto, T. (2004). Response of weed flora to combinations of reduced tillage, biocide application and fertilization practices in a 3-year crop rotation. Weed Biol. Manag. 4, 24–34. doi: 10.1111/j.1445-6664.2003.00114.x
Mohler, C. L., Caldwell, B. A., Marschner, C. A., Cordeau, S., Maqsood, Q., Ryan, M. R., et al. (2018). Weed seedbank and weed biomass dynamics in a long-term organic vegetable cropping systems experiment. Weed Sci. 66, 611–626. doi: 10.1017/wsc.2018.52
Mulugeta, D., and Stoltenbert, D. E. (1997). Seed bank characterization and emergence of a weed community in a moldboard plow system. Weed Sci. 45, 54–60. doi: 10.1017/S004317450009247X
Munier-Jolain, N. M., Collard, A., Busset, H., Guyot, S. H. M., and Colbach, N. (2014). Investigating and modelling the morphological plasticity of weeds in multi-specific canopies. Field Crops Res. 155, 90–98. doi: 10.1016/j.fcr.2013.09.018
Munier-Jolain, N. M., Guyot, S. H. M., and Colbach, N. (2013). A 3D model for light interception in heterogeneous crop:weed canopies. Model structure and evaluation. Ecol. Model. 250, 101–110. doi: 10.1016/j.ecolmodel.2012.10.023
Oerke, E.-C. (2006). Crop losses to pests. J. Agric. Sci. 144, 31–43. doi: 10.1017/S0021859605005708
Petit, S., Cordeau, S., Chauvel, B., Bohan, D., Guillemin, J. -P., and Steinberg, C. (2018). Biodiversity-based options for arable weed management: A review. Agron Sustain Dev. 38, 48. doi: 10.1007/s13593-018-0525-3
Petit, S., Deytieux, V., and Cordeau, S. (2021). Landscape-scale approaches for enhancing biological pest control in agricultural systems. Environ. Monit. Assess. 193, 75. doi: 10.1007/s10661-020-08812-2
Pittelkow, C. M., Liang, X. Q., Linquist, B. A., van Groenigen, K. J., Lee, J., Lundy, M. E., et al. (2015). Productivity limits and potentials of the principles of conservation agriculture. Nature 517, 365–U482. doi: 10.1038/nature.13809
R Core Team (2016). R: A Language and Environment for Statistical Computing. R Foundation for Statistical Computing, Vienna, Austria. Available online at: http://www.R-project.org/
R Core Team (2017). R: A Language and Environment for Statistical Computing. Vienna: RFfS Computing.
Ramsdale, B. K., Kegode, G. O., Messersmith, C. G., Nalewaja, J. D., and Nord, C. A. (2006). Long-term effects of spring wheat-soybean cropping systems on weed populations. Field Crops Res. 97, 197–208. doi: 10.1016/j.fcr.2005.09.010
Reicosky, D. C. (2015). Conservation tillage is not conservation agriculture. J. Soil Water Conserv. 70, 103–108. doi: 10.2489/jswc.70.5.103A
Rhodes, C. J. (2017). The imperative for regenerative agriculture. Sci. Prog. 100, 80–129. doi: 10.3184/003685017X14876775256165
Riemens, M. M., Van der Weide, R. Y., Bleeker, P. O., and Lotz, L. A. P. (2007). Effect of stale seedbed preparations and subsequent weed control in lettuce (cv. Iceboll) on weed densities. Weed Res. 47, 149–156. doi: 10.1111/j.1365-3180.2007.00554.x
Schreefel, L., Schulte, R. P. O., de Boer, I. J. M., Schrijver, A. P., and van Zanten, H. H. E. (2020). Regenerative agriculture – the soil is the base. Global Food Security 26, 100404. doi: 10.1016/j.gfs.2020.100404
Shrestha, J., Subedi, S., Timsina, K., Chaudhary, A., Kandel, M., and Tripathi, S. (2020). Conservation agriculture as an approach towards sustainable crop production: a review. Farm. Manag. 5, 7–15. doi: 10.31830/2456-8724.2020.002
Silva, J. V., Reidsma, P., Laborte, A. G., and van Ittersum, M. K. (2017). Explaining rice yields and yield gaps in Central Luzon, Philippines: an application of stochastic frontier analysis and crop modelling. Eur. J. Agron. 82, 223–241. doi: 10.1016/j.eja.2016.06.017
Storkey, J., Mead, A., Addy, J., and MacDonald, A. J. (2021). Agricultural intensification and climate change have increased the threat from weeds. Global Change Biol. 27, 2416–2425. doi: 10.1111/gcb.15585
Sun, Y. N., Zeng, Y. J., Shi, Q. H., Pan, X. H., and Huang, S. (2015). No-tillage controls on runoff: a meta-analysis. Soil Tillage Res. 153, 1–6. doi: 10.1016/j.still.2015.04.007
Thomas, A. G., Derksen, D. A., Blackshaw, R. E., Van Acker, R. C., Légère, A., Watson, P. R., et al. (2004). A multistudy approach to understanding weed population shifts in medium- to long-term tillage systems. Weed Sci. 52, 874–880. doi: 10.1614/WS-04-010R1
Travlos, I., Gazoulis, I., Kanatas, P., Tsekoura, A., Zannopoulos, S., and Papastylianou, P. (2020). Key factors affecting weed seeds' germination, weed emergence, and their possible role for the efficacy of false seedbed technique as weed management practice. Front. Agron. 2. doi: 10.3389/fagro.2020.00001
Trichard, A., Alignier, A., Chauvel, B., and Petit, S. (2013). Identification of weed community traits response to conservation agriculture. Agric. Ecosyst. Environ. 179, 179–186. doi: 10.1016/j.agee.2013.08.012
Van der Borght, K., Van Craenenbroeck, E., Lecocq, P., Van Houtte, M., Van Kerckhove, B., Bacheler, L., et al. (2011). Cross-validated stepwise regression for identification of novel non-nucleoside reverse transcriptase inhibitor resistance associated mutations. BMC Bioinform. 12, 386. doi: 10.1186/1471-2105-12-386
Van Inghelandt, B., Queyrel, W., Cavan, N., Colas, F., Guyot, B., and Colbach, N. (2019). “Combiner expertise et modèles en ateliers de co-conception de systèmes de culture pour une gestion durable des adventices: apports méthodologiques et perspectives,” in Gestion des adventices dans un contexte de changement - Séminaire CoSAC, eds N. Colbach, F. Angevin, C. Bockstaller, B. Chauvel, C. Denieul, D. Moreau, B. Omon, D. Pellet, A. Rodriguez, L. Trannoy, S. Volan and F. Vuillemin (Paris). Available online at: https://www.projet-cosac.fr/content/download/3348/34868/version/1/file/CoSAC%202019%20-%20actes%20colloque%20-%20diffusion%20post%20colloque.pdf
van Ittersum, M. K., Leffelaar, P. A., van Keulen, H., Kropff, M. J., Bastiaans, L., and Goudriaan, J. (2003). On approaches and applications of the Wageningen crop models. Eur. J. Agron. 18, 201–234. doi: 10.1016/S1161-0301(02)00106-5
Wilson, R. S., Tucker, M. A., Hooker, N. H., LeJeune, J. T., and Doohan, D. (2008). Perceptions and beliefs about weed management: perspectives of Ohio grain and produce farmers. Weed Technol. 22, 339–350. doi: 10.1614/WT-07-143.1
Yvoz, S., Petit, S., Biju-Duval, L., and Cordeau, S. (2020). A framework to type crop management strategies within a production situation to improve the comprehension of weed communities. Eur. J. Agron. 115, 126009. doi: 10.1016/j.eja.2020.126009
Zanin, G., Otto, S., Riello, L., and Borin, M. (1997). Ecological interpretation of weed flora dynamics under different tillage systems. Agric. Ecosyst. Environ. 66, 177–188. doi: 10.1016/S0167-8809(97)00081-9
Table A1. The 30 annual weed species included in FLORSYS in the present simulation study with values for a few major traits identified with the RLQ and 4th-corner analyses of Figure 5.
Table A2. Explanations of key cropping techniques that drive increase in weed densities when tillage is deleted without compensation, based on the RLQ and 4th-corner analyses of Figure 5.
Table A3. Explanations of key weed species traits that drive increase in weed densities when tillage is deleted without compensation, based on the RLQ and 4th -corner analyses of Figure 5.
Keywords: conservation agriculture, weed, yield loss, biodiversity, simulation, model
Citation: Colbach N and Cordeau S (2022) Are No-Till Herbicide-Free Systems Possible? A Simulation Study. Front. Agron. 4:823069. doi: 10.3389/fagro.2022.823069
Received: 26 November 2021; Accepted: 11 February 2022;
Published: 10 May 2022.
Edited by:
Cathy Hawes, The James Hutton Institute, United KingdomReviewed by:
Virginia Nichols, Arizona State University, United StatesCopyright © 2022 Colbach and Cordeau. This is an open-access article distributed under the terms of the Creative Commons Attribution License (CC BY). The use, distribution or reproduction in other forums is permitted, provided the original author(s) and the copyright owner(s) are credited and that the original publication in this journal is cited, in accordance with accepted academic practice. No use, distribution or reproduction is permitted which does not comply with these terms.
*Correspondence: Nathalie Colbach, bmF0aGFsaWUuY29sYmFjaEBpbnJhZS5mcg==
Disclaimer: All claims expressed in this article are solely those of the authors and do not necessarily represent those of their affiliated organizations, or those of the publisher, the editors and the reviewers. Any product that may be evaluated in this article or claim that may be made by its manufacturer is not guaranteed or endorsed by the publisher.
Research integrity at Frontiers
Learn more about the work of our research integrity team to safeguard the quality of each article we publish.