- 1Shandong Provincial Key Laboratory of Crop Genetic Improvement, Physiology and Ecology, Shandong Academy of Agriculture Sciences, Jinan, China
- 2Qilu University of Technology, Science and Technology Service Platform of Shandong Academy of Sciences, Jinan, Shandong, China
- 3Department of Biology, Sinovita Bioengineering Group, Jinan, Shandong, China
- 4Institute of Wetland Agriculture and Ecology, Shandong Academy of Agricultural Sciences, Jinan, China
- 5Department of Biology, Shandong Siwenhouji Agricultural Development Co. Ltd., Jinan, China
- 6Shandong Small and Medium-Sized Enterprises Productivity Promotion Center, Jinan, Shandong, China
Using silicate fertilizer and bacterial inoculum as biofertilizer is significant for increasing soil silicon (Si) availability and rice agronomic performance. To use microbial technology for sustainable agriculture, it is crucial to have a deeper knowledge of how microbial populations shift among the plant hosts and related compartments, as well as how they respond to various fertilization models. In this study, the effects of silicate fertilizer, a single bacterial strain Bacillus mucilagniosis as biofertilizer, and their integrated application on soil physiochemical properties and soil microbiota structure, composition, and diversity in two eco-geographically diverse races (Indica and Japonica rice) were evaluated. Plant compartment, cultivar type, and fertilizer treatments contributed to microbiome variation. Indica and Japonica harbor different root microbiota; notably, taxa enriched in the rhizosphere soil were more diverse than in the root. Bacterial genera Leptonema, Azospira, Aquabacterium, Fluviicola, Aquabacterium, Leptonema, and fungal genera Metarhizium, Malassezia, and Cladosporium all were found in the rice core microbiome. Both silicate and biofertilizer applications increase the relative abundance of Betaproteobacteria, Deltaproteobacteria, and Actinobacteria, while suppressing fungal pathogens Alternaria and Fusarium. Silicate and bacterial inoculum applications increased the soil pH, available silicon content (ASi), available phosphorous (AP), available potassium (AK), and organic carbon (OC), while reduced the total nitrogen (N). These changes were also associated with major bacterial phyla Spirochaetes, Bacteroidetes, Actinobacteria, and Proteobacteria, except for Acidobacteria, and fungal phyla Ascomycota, Mortierellomycota and unassigned fungi. Several treatment-specific biomarkers were revealed through Linear discriminant analysis effect size (LEfSe) analysis. In conclusion, the change in the structure of root-associated communities driven by plant compartment and genetics suggests dynamic interactions in the host plant microbiome. Short-term silicate and biofertilizer amendments improved soil physiochemical status and altered bacterial and saprotrophic fungal communities, which have important implications for sustainable rice production.
1 Introduction
A plant’s body can be considered as the dynamic interplay of ecological niches that harbor a mesmerizing number of microorganisms on their root surface (soil-root interface or rhizoplane), endosphere (inside the root), and rhizosphere (soil zone influenced by roots), collectively called microbiota (Bulgarelli et al., 2013; Edwards et al., 2015). Each spatially separable compartment recruits a distinct group of prokaryotes and eukaryotes (Wei et al., 2021). These host-associated soil-born bacterial and fungal communities play significant roles in host nutrition, development, and immunity (Hacquard et al., 2017; Jacoby et al., 2017; Lebreton et al., 2019). Niche-based clear differentiation among the rhizosphere and endosphere communities has been previously reported (Ding et al., 2019; Mukhtar et al., 2021). The rhizosphere is a hot spot for microorganisms since exudates secreted by plant roots are a preferred food reserve for microbes and a driving factor behind their population size and activity. For instance, bacterial populations dominated the rice rhizosphere zones found more diverse than in non-rhizosphere regions, regardless of rice growth stage or soil depth (Breidenbach et al., 2015; Lee et al., 2015). In another study, rhizosphere microbial communities were discovered to be more diverse than the microbiota in the root endosphere and phyllosphere, which were also highly sensitive to fertilization (Sun et al., 2021). Such selection of different microbial communities by plants associated with their roots and surrounding soil can contribute to the selective enrichment of diverse microbes and can promote overall plant health. However, the enrichment process of niche-based selection by the host plants for these microbial populations occupying specific plant compartments remain poorly characterized.
Rice is the most important staple food that feeds 50% population globally, and we are just beginning to study its below-ground microbiome. Rice root and rhizosphere microbiome structures vary depending on the plant genotype, which results from interactions between the host and rhizosphere microbiomes (Edwards et al., 2015; Zhang et al., 2019). Indica and japonica are the two most common types of Asian cultivated rice (Oka, 1958). The genetic and phenotypic differences between these two rice subspecies are well-documented (Huang et al., 2012; Wang et al., 2018). A recent study showed that Indica and japonica cultivars recruited different root microbiota, and taxa enriched in Indica were more diverse than those in the japonica (Zhang et al., 2019). Further, soil type, physicochemical properties, and host genetics all influence the structure of the rice microbial community in the rhizosphere (Edwards et al., 2015; Yu and Hochholdinger, 2018). Nevertheless, host genetics and environmental factors such as silicate fertilizer application and inoculation of silicate solubilizing bacteria as biofertilizers that may influence the total host microbiome are poorly defined, notwithstanding that they are crucial in understanding the development of these symbiotic relationships.
Silicon (Si), one of the most abundant elements in the Earth’s crust, is considered agronomically important for rice production. Intensive cultivation to suit the demand of an ever-increasing population reduces the Si content of paddy soils, degrading the soil and lowering rice yield (Makabe-Sasaki et al., 2014). Thus, Si fertilizer is strongly advised for Si-deficient soils to boost plant performance and crop yield. Additionally, the boom in iron and steel production in recent years and the generation of a significant volume of its byproduct (slag) necessitate its utilization efficiently. Steel slags are rich in silicon Si, Ca, Mg, P, Fe, and Mn as the main fertilizer components; these are crucial for their application in the agriculture (Deus et al., 2018). The proportion of such components in each slag differs depending on source materials, steel type, furnace type, and other variables (Shi, 2004; Deus et al., 2018). Several studies have demonstrated that applying slag-based silicate fertilizer in agriculture has tremendous potential for improving rice productivity, plant disease resistance, and metal detoxification (Ning et al., 2014; Das et al., 2019b; Wang et al., 2020). At the microbiome level, recent studies have shown the positive effects of silicate fertilizer on bacteria and fungi populations (Das et al., 2019c; Wang et al., 2020). It has been revealed that several bacterial phyla were enriched and depleted while exposed to silicate fertilizer. The increased abundance of several genes related to the carbon cycle, nitrogen fixation, and heavy metal reclamation functions was associated with the silicate fertilization (Das et al., 2019a). The same group reported the alteration in the saprotrophic fungal communities and accelerated organic decomposition under the silicate fertilizer application (Das et al., 2019c). In another study, silicon fertilizer increased crop yield while decreasing the heavy metal content in multiple metals contaminated soil and modifying the structure and heterogeneity of the bacterial community associated with the metal-resistance (Wang et al., 2020).
Besides, modern agricultural practices employ a range of management tools including, developing plant varieties with varied genetic backgrounds, use of organic and inorganic fertilizers, and highly efficient microbiological biofertilizers, to boost plant growth and yield by minimizing the consumption of chemical fertilizers (Mi et al., 2018; Eshed and Lippman, 2019; Pirttila et al., 2021). More recently, several studies have shown the importance of organic amendment techniques for different crops grown in contaminated soils (Nawab et al., 2016; Nawab et al., 2018; Ghani et al., 2022; Ullah et al., 2022). It was demonstrated that using organic geo-sorbents, such as bicolor, in mine-degraded soils could promote spinach growth, improve nutritional profile, minimize bioaccumulation of potential hazardous elements, and lower health risk indices (Nawab et al., 2019; Hussain et al., 2020). In another study, organic additives considerably lowered Cd levels in vegetables such as tomatoes and cucumber, improved food quality, while improving biomass supply (Khan et al., 2018). Certain agricultural approaches, however, may cause native plant-associated microbiome dysbiosis, which ultimately influences crop yield (Kent and Triplett, 2002). For instance, inoculation with genetically engineered microbes has been shown to have nontargeted and negative impacts such as excessive carbon turnover, displaced indigenous beneficial Pseudomonas communities, and prolonged repression of fungal populations (Nacamulli et al., 1997). On a positive note, silicate solubilizing bacteria as biofertilizers has been demonstrated to play an important role in boosting soil fertility by solubilizing insoluble silica and assisting in the solubilization of potassium and phosphates, resulting in higher plant production (Maleva et al., 2017). Bacteria that are capable to dissolve silicates are mainly found in the phyla Proteobacteria and Firmicutes. The majority of silicate solubilizing bacteria belong to Bacillus, Pseudomonas, and others. Bacillus mucilaginous, generally known as silicate bacteria, generate a variety of exopolysaccharides. Bacillus mucilaginous has been widely used in agriculture as a multifunctional microbial fertilizer capable of making K, P, and other useful elements available by dissolving insoluble minerals in the soil (Liu et al., 2017). It was reported that Bacillus mucilaginous could dissolve mica and soil minerals and simultaneously emit K(+) and SiO(2) from crystal lattices (Liu et al., 2006). The use of Bacillus mucilaginous as a biofertilizer, alone and in combination with other biofertilizers, was demonstrated to improve maize growth attributes such as plant height, dry weight of vegetative parts, and grain yield (Hussien et al., 2019). Other plant growth-promoting effects of transgenic Bacillus mucilaginous on model plant tobacco have been well documented (Li et al., 2007). Although these studies report the functional role of Bacillus mucilaginous in other crops; However, its effects as a biofertilizer together with the silicate fertilizer on bacterial and fungal community structure, diversity and composition in a single study have not yet been studied, particularly in rice.
One of the fundamental questions of ecological importance that can subsequently intervene in the success of sustainable agricultural practices to produce plants like rice is to what extent does biofertilizer inoculation with effective biofertilizer such as Bacillus mucilaginous, with silicate fertilizer, or the combined effect of both treatments disrupt the native microbial communities associated with its root system and surrounding soil. The objectives of this study were to evaluate how the slag amendment biofertilizer such as Bacillus mucilaginous affects soil nutrient status and the structure and composition of the total bacterial and fungal population in the root and rhizosphere soil in rice cropping systems. In this work, we specifically assess whether the assessed factors (host genetics, plant compartment, and fertilization) would lead to a change in the makeup of the soil’s microbial population, which would then affect the sustainability of crop production by the addition of slag to a rice cropping system.
2 Materials and methods
2.1 Pot experiments and plant growth
All data were generated from an open-air pot experiment at the Shandong Academy of Agricultural Sciences facility in the summer of 2021. In brief, four treatments were used for two different types of rice verities, i.e., Ming Hui-63 (Oryza sativa ssp. Indica) and Sheng Xiang-1826 (Oryza sativa ssp. Japonica). The four treatments include soil with silicate solubilizing bacterial strain Bacillus mucilaginosus as biofertilizer, hereafter as (BM), application of slag silicate fertilizer alone (Si), soil with both types of fertilizers silicate + biofertilizer (SiBM) and soil without either fertilizer application or control group (Control). The soil used for the pot experiments was collected from the plough layer (approximately 8 inches) using a shovel from a paddy field with a decade history of rice plantation in Jinan, Shandong province, China (latitude 36°40′N; longitude 117°00′E). The soil was placed into clean tubs to crush, airdry, sieve (0.5 cm sieve), homogenize, and 14 kg soil was packed in Wagner pots (0.24 m diameter and 0.3 m height) to a bulk density of 1.2 g cm (Das et al., 2019a). The silicate fertilizer made-up of industrial slag at the rate of 2 Mg ha−1 was applied three days before transplanting, and the soil was immediately flooded. The composition of slag fertilizer is presented in (Supplementary Table S1).
Rice seedlings were germinated and grown for 25 days in a greenhouse tray nursery, established with the above-described unsterilized soil. The specific treatment-based alterations in the rice-associated microbiomes were developed within the rice rhizosphere and roots grown in the potting soil under four different experimental conditions. 25 days old seedlings of rice variety Ming Hui-63 (Indica) and Sheng Xiang-1826 (Japonica) were transplanted. Pots were kept open-air to provide maximum natural field conditions. The first community, control, was obtained from uninoculated roots and rhizosphere, receiving no fertilizer treatments. The second treatment community, Si, was obtained from uninoculated roots and rhizosphere soil receiving silicate fertilizer treatment. The third treatment community, designated as BM, was obtained from roots and rhizosphere soil inoculated with 1 ml of a pure culture containing 1.34 × 108 CFU of Bacillus mucilaginosus strain. The fourth treatment community, SiBM, was obtained from roots and rhizosphere receiving the same dose of silicate fertilizer plus biofertilizer inoculation of the Bacillus mucilaginosus strain. Each treatment was replicated with five seedlings in each of three separate pots. A total of 24 pots, from 4 treatments (3 x 4 = 12 pots for Indica, and 3 x 4 = 12 pots for Japonica) were prepared in greenhouse experiments. The slag silicate fertilizer used in this study was in powder form. Besides, mineral fertilizers N, P2O5, and K2O using urea, fused superphosphate, and potassium chloride at the rate of 110, 45, and 58 kg ha−1 respectively were applied to the pots. Basal fertilizers broadcasted one day before transplanting were 55 kg N ha−1, 45 kg P2O5 ha−1, and 40 kg K2O ha−1. Moreover, 55 kg N ha−1 and 18 kg K2O ha−1 were broadcasted at the mid-vegetative stage (tillering), two weeks after transplanting. The equal water level (about 5 cm above the soil surface) for all pots was retained throughout the cropping season. Distinctive replicated characteristics of the potted soil comprised fertilizer amendments, irrigation with water when needed, and an open area to provide a possible natural environment.
2.2 Sample collection and preparation
For microbial community analysis, samples from roots along with the rhizosphere soil attached were collected after 90 days of silicate and biofertilizer treatments from the root system of each rice plant (three randomly selected plants from each treatment). The excess soil was manually shaken from the roots, leaving approximately 1mm of soil still attached to the roots. The rhizosphere soil (soil that tightly adhered to the root) was selected over non-rhizosphere soil (bulk soil) because compared to non-rhizosphere soil, the rhizosphere is the dynamic region where plant root exudates sustain high microbial activity. Samples were collected in the field by placing the roots with soil still attached in a 50 ml falcon tube containing 35 ml of sterile Phosphate Buffered Saline (PBS) solution. Tubes containing root and rhizosphere soil samples were immediately kept on the icebox, brought back to the laboratory, and stored at 4°C until the rhizosphere fraction separated the next morning. To clean all the soil from the root surface, tubes were shaken at high speed on the vortex for 20 seconds; roots were removed with the help of sterile forceps and placed into a new 50 ml falcon tube. Soil washed from the roots was named rhizosphere compartment and stored at -80°C. Washed roots were rinsed three more times with 25 ml PBS until all soil was washed out, and tubes containing clean roots were archived at -80°C until nucleic acid (DNA) extraction.
2.3 Preparation and application of bacterial inoculum as biofertilizer
The bacterial strain Bacillus mucilaginosus was purchased from the China General Microbiological Culture Collection Center (CGMCC), Beijing, China. The inoculant was prepared followed by previous published protocol (Zhao et al., 2012); In brief, pure cultures of the Bacillus mucilaginosus strain were grown in a nitrogen-free medium consisting of 1 L distilled water with mannitol or sucrose 10.0g, Calcium sulfate dihydrate (CaSO42H2O) 0.1g, Dipotassium phosphate (K2HPO4) 0.2g, Calcium Carbonate (CaCO3) 5g, Magnesium sulfate (MgSO47H2O) 0.2g, Sodium chloride (NaCl) 0.2g, Agar 15g (for solid medium), shaking at 150 rpm and 25°C for 26–36 h. Five days after the transplantation of rice seedlings, the soil was inoculated with 1 ml of a pure culture containing 1.34 x 108 CFU of Bacillus mucilaginosus strain to the bottom of each rice seedling.
2.4 Nucleic acid extraction and amplicon sequencing
Microbial DNA from each rhizosphere soil and root sample was extracted using the PowerSoil® DNA Isolation Kit (Mo Bio, USA), according to the manufacturer’s instructions. The purity and quantity of genomic DNA were determined using the NanoDrop spectrophotometer and walk-through gel electrophoresis. Each DNA sample was subjected to amplify the V4 domain in 16S bacterial ribosomal DNA using standard primer pair 515F/806R; the same was used in the Earth Microbiome Project (Caporaso et al., 2011). The primers used for fungal ribosomal internal transcribed spacers (ITS) amplification were ITS1F/ITS2R (White et al., 1990). Polymerase chain reaction (PCR) amplification details have been described in (Tong et al., 2021). PCR amplification for each sample was done in triplicate with each set of primer pairs. Amplicons were extracted from 2% agarose gels and purified using the AxyPrep DNA Gel Extraction Kit (Axygen Biosciences, Union City, CA, USA) according to the manufacturer’s instructions before being quantified using the ABI StepOnePlus Real-Time PCR System (Life Technologies, Foster City, USA). Afterwards, the purified amplicons were pooled into equimolar quantities. Pooled libraries were then sequenced with a paired-end strategy (2 x 250) on the Hiseq 2500 platform (Illumina, San Diego, CA).
2.5 Bioinformatics and statistical analysis
The raw sequence reads were processed and analyzed using the open-source pipeline, Quantitative Insights Into Microbial Ecology (QIIME release-2020.8) (Bolyen et al., 2019). For quality control, reads with quality scores <20, containing primers and any extra spacer nucleotide sequences (N bases), were removed from forward and reverse raw reads using the CutAdapt tool within the QIIME pipeline. The DADA2 algorithm (Callahan et al., 2016) was used to filter, demultiplex, and denoise the truncated reads to find the amplicon sequence variants (ASVs). The resulting ASVs data were then compiled into feature/OTU tables. The taxonomic classification of the representative sequences for each prokaryotic ASVs was performed using the Naive-Bayes classifier against the SILVA database release-132 (Yilmaz et al., 2014). For ITS sequences, a pretrained Naive-Bayes classifier trained on the UNITE dynamic database release was used, available on the QIIME website (https://unite.ut.ee). α diversity (Shannon) and β diversity (phylogenetic) were calculated using core metrics analysis within the QIIME pipeline, using feature tables rarefied at even sequencing depth (Supplementary Figure S2). Significant differences in the data, unless otherwise stated, were analyzed by the Kruskal test to compare the mean for each variable for α diversity. For β diversity, the Analysis of Similarity (ANOSIM) test with 999 permutations was employed to assess the statistically significant differences between variables using Bray Curtis dissimilarity for 16S and Jaccard indices for ITS. Analysis of microbiome composition (ANCOM) test was used to determine significant taxonomic abundance differences between grouped samples. To identify substantially different bacterial species among groups, a linear discriminant analysis (LDA) effect size (LEfSe) was conducted. Taxa with LDA scores >2 with a p-value <0.05 were considered significant.
3 Results
3.1 Overview of amplicon sequencing and predominant microbial taxa
After initial processing of sample de-multiplexing and primer matching, 3,027,839 sequence reads for 16S and 2,986,437 for the ITS dataset were retrieved. Quality filtering procedure (i.e., denoising, filtering, merging, chimera filtering) decreased this amount to 2,500,210 reads (Min 47,335, Max 58,807) with an average of 52,087 reads per sample for 16S and 2,914,828 total reads (Min 27,187, Max 62,891), with an average of 60,725 reads per sample for ITS; reflecting a 17.43% and 2.39% reduction in size respectively (Supplementary Table S2). The high-quality sequences for community analysis had an average length of 252 bp (16S) and 249 bp (ITS). Further processing was performed on the classification results by removing chloroplast and other non-desired reads that could not be confidently assigned to bacteria or fungi domains. The clean reads were clustered into 7,961 bacterial and 4,771 fungal ASVs (amplicon sequencing variants) (Supplementary Tables S3, S4). The resulting ASVs or feature tables were rarefied at minimum read numbers to avoid sequencing bias. Results of alpha rarefaction showed sufficient sequencing depth for the meaningful analysis of two ecological diverse rice cultivar microbial communities from two different niches under four treatments (Supplementary Figure S2). The 16S amplicon data set comprises 18 bacterial phyla, 48 classes, 81 orders, 165 families, and 387 genera identified among all the samples. Proteobacteria was the most prevalent phylum in all groups, accounting for 48% of the overall community composition; following that, Acidobacteria (15.7-18.7%), Actinobacteria (14.9-17.1%), Firmicutes (11.9-14.8%), while Bacteroidetes were accessed in a relatively small abundance (0.9-1.8%) (Figure 1A, Supplementary Figure S3, and Table S5). Likewise, 7 phyla, 30 subphyla, 77 orders, 179 families, and 324 genera were inferred from fungal community sequence features. Ascomycota was the most abundant among the phyla detected, accounting for 86% of the total fungal community diversity in all samples, followed by Basidiomycota (4.8-9.3%) and Mortierellomycota (1.1-1.6%). Other phyla were found in low abundance (Figure 1B; Supplementary Figure S3, and Table S6).
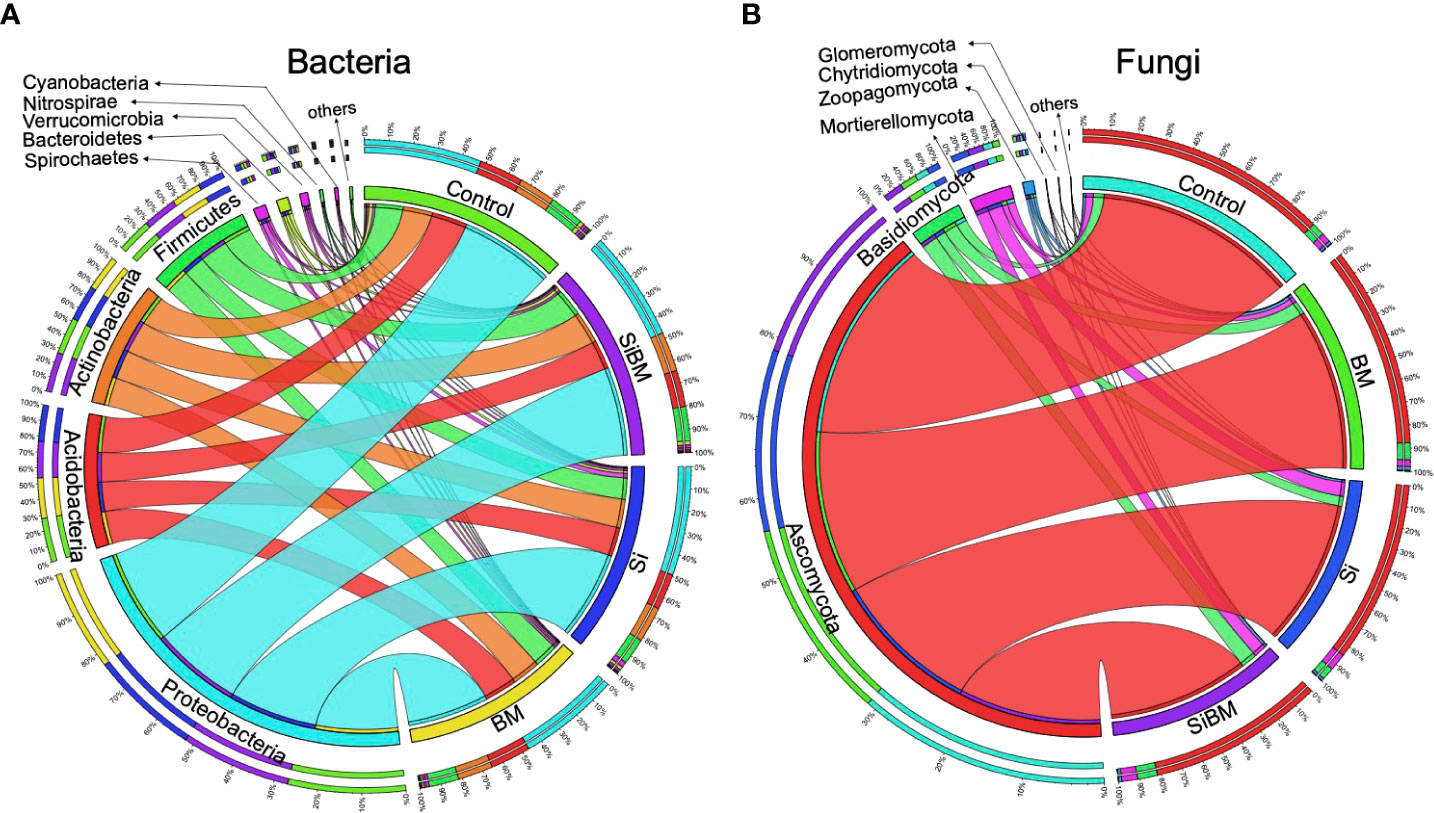
Figure 1 Representation of the microbial communities at the phylum level, receiving industrial silicate fertilizer and bacteria as biofertilizer. The bacterial microbiome population was determined by 16S amplicon sequencing (A). ITS amplicon sequencing generated fungal microbiome communities (B). Each color in the left circle represents a phylum, and the samples are on the right panel. Sample names are denoted as, treatment with silicate (Si); treatment with bacteria inoculant (BM); bacteria plus silicate treatment (SiBM); and the control group. The outer rings, organized by sample type or phylum, show the composition of each phylum or sample, respectively. Additional detailed percentage information at the phylum level is provided in (Figure S3 and Supplementary Tables S5, S6).
3.2 Factors affecting rice microbial diversity
The alpha (α) diversity analysis revealed that compartment, cultivar type, and to a lesser extent, treatment were the factors that drive the rice whole microbial communities. For the bacterial community, at the compartment level, higher α diversity was found in the rhizosphere soil compared to the root samples (Figures 2A, B and Table 1). Further analysis of cultivar type revealed that Indica had higher bacterial diversity than their counterpart Japonica type (Figures 2C, D and Table 1), indicating that Indica recruited diverse taxa on their roots compared to the Japonica. At the treatment level for all bacterial samples, Si and control samples showed increased α diversity compared to the BM and their integrated treatment SiBM (Supplementary Figure S4). Simultaneously, fungal communities were highly influenced by both compartment and cultivar types (Figures 2E–H and Table 1). In the root compartment, fungal communities between Indica and Japonica samples were less affected (Figure 2G and Table 1). We did not find any significant differences between the treatment groups.
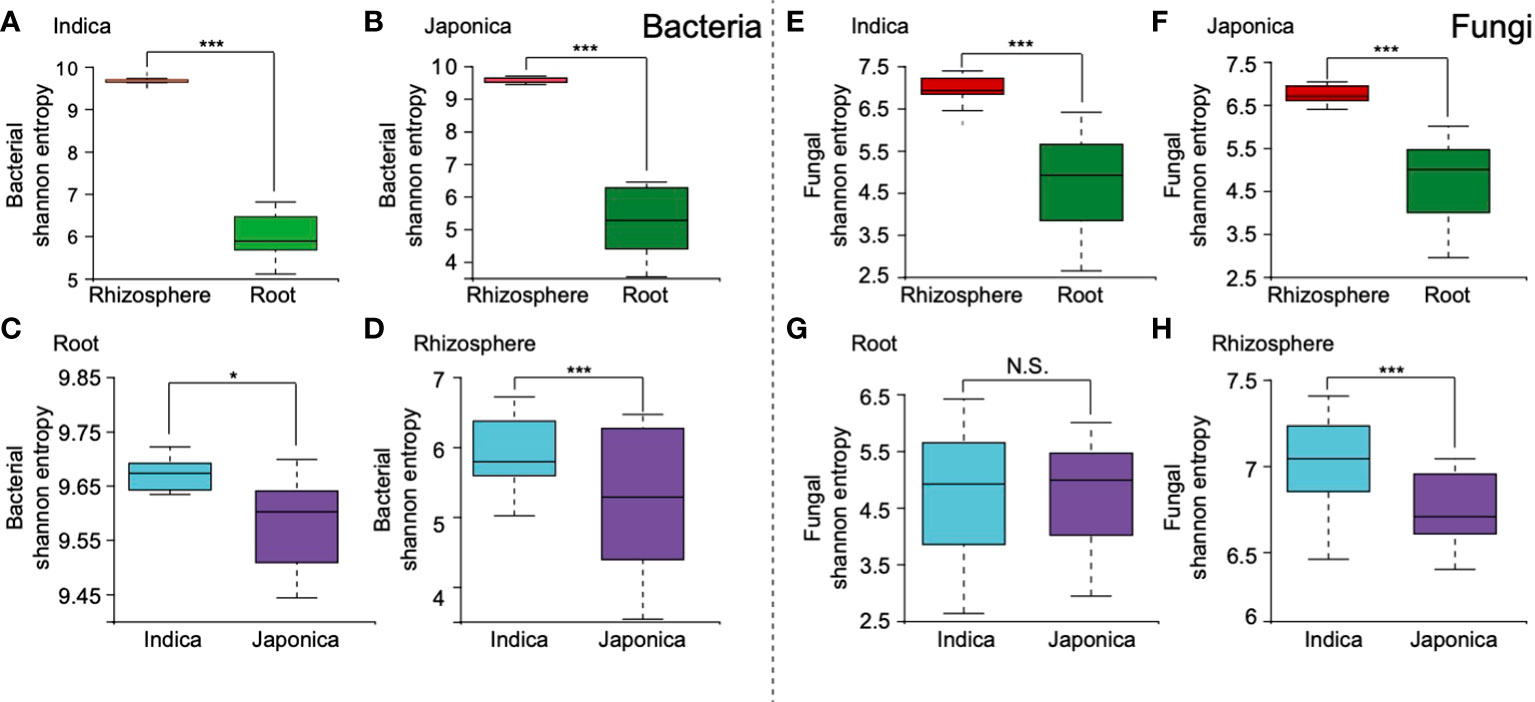
Figure 2 Microbiome α diversity of compartment and cultivar type. For bacterial samples, Shannon-based α diversity of Indica and Japonica, (A, B), root and rhizosphere (C, D). Shannon-based α diversity of fungal microbiome communities, Indica and Japonica (E, F), and root and rhizosphere sample (G, H). “***, **, *” Indicate the significant test using ANOSIM test under p-value < 0.001; <0.01; <0.05, N.S, Non-significant.
Beta (β) diversity measures, on the other hand, showed compartment-specific and cultivar-dependent separation. Analysis of similarity (ANOSIM) test with 999 permutations was applied to see if the differences in bacterial diversity across the groups (compartment, cultivar type, and treatment) were significant. There were statistically significant differences in the bacterial and fungal diversity across species in the root and rhizosphere compartments (p-value 0.001), as well as under four treatments (p-value <0.005). Principle Coordination Analysis (PCoA) of the bacterial community using bray distance indices for cultivar type showed that Indica samples clustered far from the Japonica samples and showed clear separation patterns in each compartment (Figures 3A, B). A similar trend was observed for compartments, where rhizosphere samples clustered together at one end and root samples at the other (Figures 3C, D). β diversity analysis of the fungal communities using Jaccard distance revealed clear patterns of separation for both compartments (Figures 3E, F) and cultivar type (Figures 3G, H), where each group was clustered separately (Figures 3E, H). Since β diversity differences between the compartments and cultivar type were obvious, a separate analysis under silicate and biofertilizer treatments for Indica and Japonica revealed that β diversity for both microbial kingdoms changed dramatically (Figure 4). This was true for bacteria in general and fungus in particular. The β diversity of bacterial and fungal groups at the individual compartment level was impressively evidenced by the PCoA plots. For instance, in the rhizosphere compartment of Indica, the bacterial communities of Si alone and SiBM treated samples clustered separately from the control, while BM samples showed a similar pattern to control (Figures 4A, C). Unlike Indica, the control group samples of bacterial and fungal communities of Japonica in the rhizosphere were clustered separately from the rest of the three treatment groups (Figures 4B, D). The same was true for the root compartment in Japonica (Figures 4B, D). Notably, both Indica and Japonica bacterial communities in the root compartments were less affected than the rhizosphere compartment under silicate and biofertilizer treatments (Figures 4B, D).
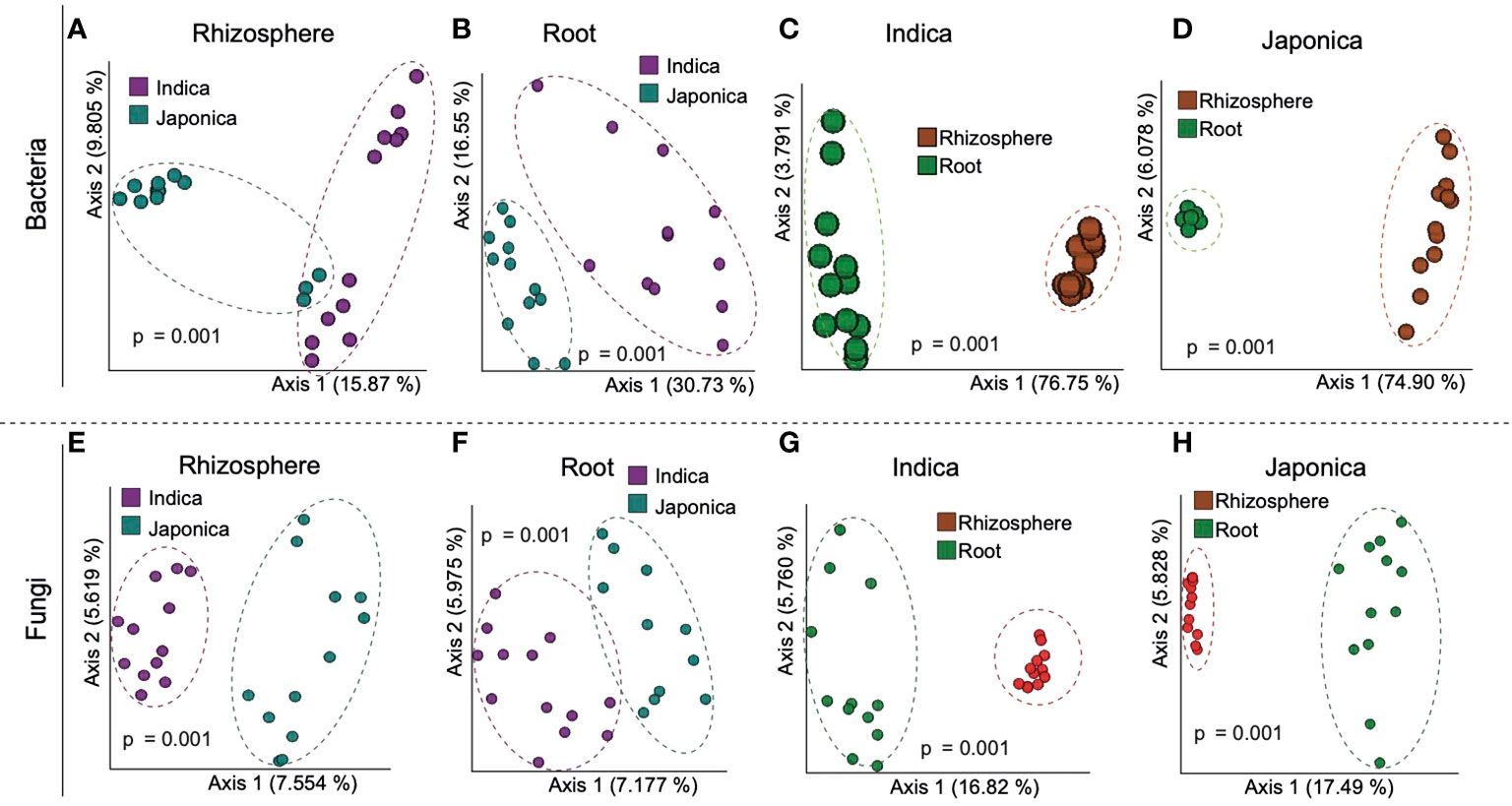
Figure 3 β diversity of bacterial (A, D) and (E, H) fungal microbial communities of indica and Japonica rice. Each group of samples is indicated by one color and circled; pink color represents Indica, green color represents Japonica; red represents rhizosphere, and green represents root samples. ANOSIM test was used for statistical analysis, and a p-value < 0.05 was considered significant.
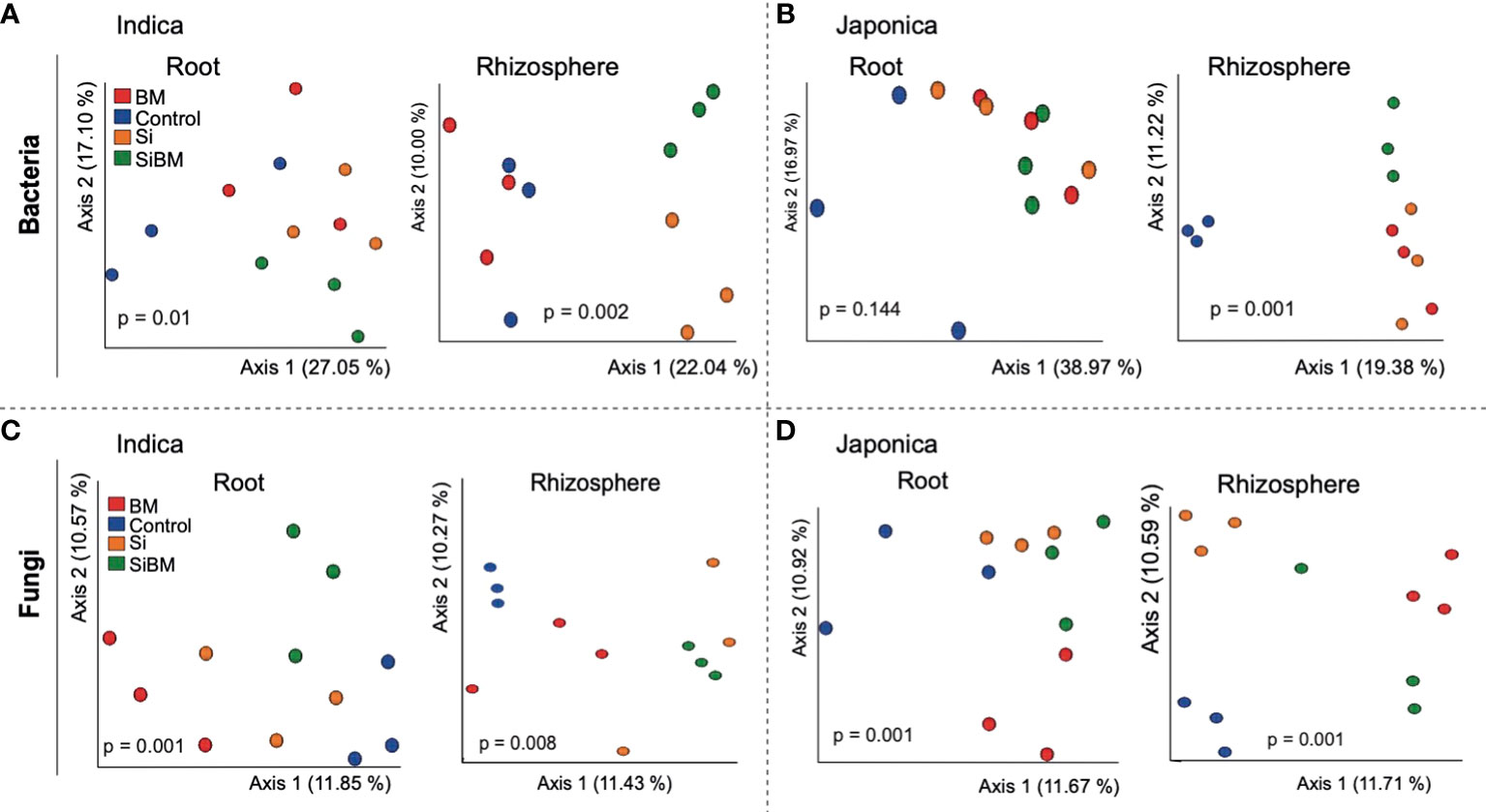
Figure 4 β diversity of bacterial and fungal microbial communities of rice under silicate and biofertilizer treatments. PCoA plots showing the Bray Curtis-based beta diversity of bacterial samples (A, B) and Jaccard distance-based separation of ITS samples (C, D). Each group of samples is Indicated by one color and circled; the red color represents biofertilizer treatment, the blue color represents control, the orange color represents silicate treated samples, and the green represents silicate plus biofertilizer treatment. ANOSIM test was applied for statistically significant differences among the groups, and a p-value < 0.05 was considered significant.
3.3 Taxa responsive to silicate and biofertilizer treatments
The topmost abundant bacterial and fungal classes detected among the groups are shown in (Figure 5). The bacterial community mainly consisted of class Betaproteobacteria (22.8-28.0%), Actinobacteria (14.9-17.1%), Acidobacteria_Gp6 (10.9-12.9%), Deltaproteobacteria (7.8-12.1%), Alphaproteobacteria (7.2-11.8%), Clostridia (4.6-6.3%), Negativicutes (3.9-5.1%), and Bacilli (2.9-4.4%). The proportions of these taxa changed among the compartments; for instance, Betaproteobacteria, Deltaproteobacteria, Clostridia, and Negativicutes, were highly abundant in roots. In contrast, Acidobacteria_Gp6, Actinobacteria, Alphaproteobacteria, and Bacilli were enriched in the rhizosphere compartment (Figure 5A and Supplementary Table S7). Among the cultivars, members of Proteobacteria, including Betaproteobacteria and Deltaproteobacteria, were overrepresented in Japonica, while Actinobacteria, Acidobacteria_Gp6, and Clostridia were overrepresented in Indica. Likewise, a comparative analysis of these taxonomic groups between the control and treatment groups showed an increased abundance of Betaproteobacteria, Deltaproteobacteria, and Actinobacteria in all three treatment groups. In contrast, the relative abundance of Alphaproteobacteria was decreased under three fertilization treatments compared to the control (Figure 5A and Supplementary Table S7). In the case of fungi, some important subphyla were increased or decreased among the compartment, cultivar type, and under the silicate and biofertilizer amendments (Figure 5B). The most abundant fungal classes were, Sordariomycetes (28.0-34.8%), Dothideomycetes (11.7-33.4%), Eurotiomycetes (4.8-21.9%), Leotiomycetes (6.6-10.3%), unclassified Ascomycota (5.6-8.2%), Unidentified_Fungi (1.5-5.8%), Tremellomycetes (2.1-5.7%) and Agaricomycetes (0.9-5.5%). Among these classes, Sordariomycetes, Leotiomycetes, and Ascomycota were enriched in the root compartment, and Dothideomycetes, Eurotiomycetes, Unidentified_Fungi, and Agaricomycetes, were abundant in the rhizosphere. Both Eurotiomycetes, and Ascomycota, were enriched in Indica, and Sordariomycetes, Dothideomycetes, Leotiomycetes, and Tremellomycetes were enriched in Japonica. There was a drastic change in the fungal taxa at the treatment level between fertilizer treatments and the control group. The most abundant class of Sordariomycetes increased in all the treated samples regardless of treatment type compared to the control samples (Figure 5B and Supplementary Table S8). In contrast, an opposite trend was observed for Dothideomycetes, the relative abundance of this class was higher in the control samples but decreased under both Si and BM treatments. Other notable differences were in the relative abundance of Eurotiomycetes, which was higher in control and BM samples compared to the Si and SiBM samples (Figure 5B and Supplementary Table S8), Unidentified_Fungi, on the other hand, increased in Si as well as in SiBM samples. Other fungal taxa, such as Agaricomycetes and Leotiomycetes, were more abundant in SiBM compared to the control and BM treatment (Figure 5B and Supplementary Table S8).
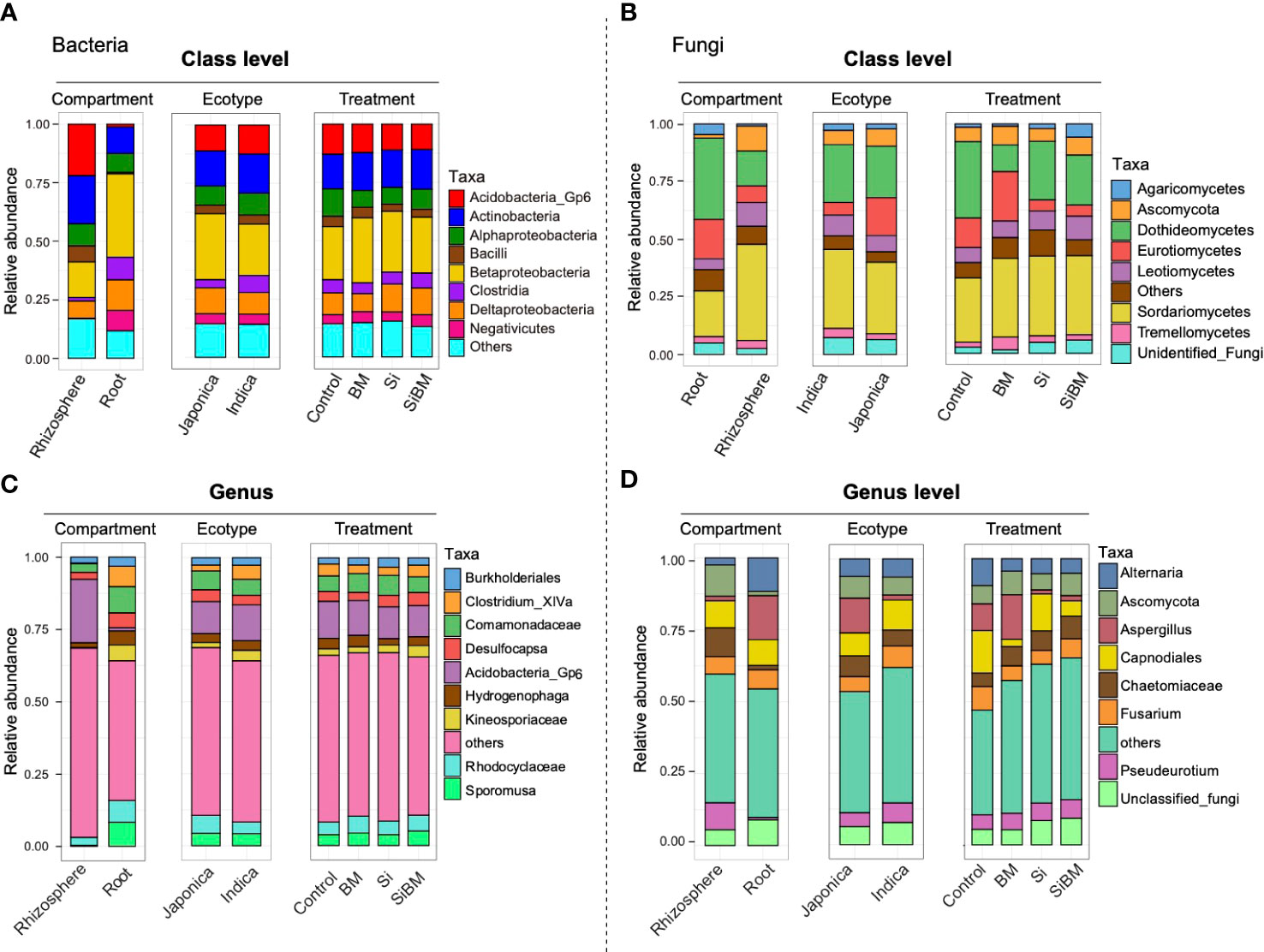
Figure 5 Taxonomic profiles of rice microbial communities at class (A, B) and genus (C, D) levels. Bar graphs depicting the relative abundance of the most prominent bacterial (A) and fungal (B) classes and bacterial (C) and Fungal (D) genera found for the compartment, cultivar type, and treatment.
In addition, the top most abundant bacterial genera were, Acidobacteria Gp6 (10.9-12.9%), members of Comamonadaceae (5.4-7.0%), and Rhodocyclaceae (4.5-5.9%), Sporomusa (3.7-5.0%), Desulfocapsa (2.8-4.5%), Clostridium_XlVa (2.8-4.1%), Hydrogenophaga (2.2-4.0%), members of Kineosporiaceae (2.1-4.0%), and Burkholderiales (2.1-3.2%). Comparative analysis of the bacterial genera between compartments showed that Clostridium_XlVa, members of Comamonadaceae, Rhodocyclaceae, Desulfocapsa, and Sporomusa were the most abundant genera in root samples, and Acidobacteria_Gp6 were most abundant in rhizosphere samples (Figure 5C and Supplementary Table S9). Members of Comamonadaceae and Rhodocyclaceae were overrepresented in Japonica, and Acidobacteria_Gp6, Clostridium_XlVa, and unclassified Kineosporiaceae were most abundant in Indica. As for the treatments concerned, most bacterial genera were equally presented in all the treatments; except for the Gp6 (Acidobacteria) and Hydrogenophaga, both were depleted under Si and BM treatments, while the relative abundance of Desulfocapsa increased (Figure 5C and Supplementary Table S9). Likewise, Rhodocyclaceae and Sporomusa were abundant in BM treatment and SiBM compared to the control. Bacterial phylotypes that belong to Kineosporiaceae were increased in SiBM treatment (Figure 5C and Supplementary Table S9). Unlike bacteria, the taxonomic profiles of the fungal community at the genus level were more dynamic and highly affected by the fertilizer treatments (Figure 5D). The top most abundant fungal genera were Capnodiales (2.6-14.8%), Aspergillus (1.4-15.6%), Alternaria (4.4-9.4%), Fusarium (4.9-8.2%), Ascomycota (5.6-8.2%), unclassified Chaetomiaceae (4.7-7.9%) and Pseudeurotium (5.2-6.5%). Comparing the relative abundance at the compartment level, genera Alternaria, Aspergillus, and unclassified fungi were overrepresented in the root, and members of Ascomycota, Chaetomiaceae, and Pseudeurotium were overrepresented in the rhizosphere compartment (Figure 5D and Supplementary Table S10). Among these lineages, Aspergillus and Pseudeurotium were highly abundant in Japonica, while, Capnodiales, Fusarium, and Chaetomiaceae were highly abundant in Indica. Interestingly, both Si and BM suppress the relative abundance of pathogen fungal genera Alternaria and Fusarium. On the other hand, the relative abundance of Aspergillus, Capnodiales, and unclassified fungi increased (Figure 5D and Supplementary Table S10).
3.4 Core communities and differential abundant taxa
ASVs shared by more than 95% of samples in the 16S dataset, consisting of 538 bacterial ASVs belonging to 203 genera, and more than 75% of samples in the ITS dataset representing 291 fungal ASVs associated with 122 genera were defined as the core microbiome. A heat map analysis of the most prevalent (top fifty) core microbiomes at the genera level is given to display the dominance of these communities among the control, BM, Si, and SiBM (Supplementary Figures S5, S6). Both bacterial and fungal core communities were clustered into two groups, first group consisting of all the Indica samples and the second group of Japonica samples; within each group, these core communities were differentially abundant between the control and fertilizer treatments. At the genus level, Gp6 (Acidobacteria), Comamonadaceae, Rhodocyclaceae, Sporomusa, Desulfocapsa, Clostridium, Hydrogenophaga, Kineosporiaceae, Burkholderiales, Desulfovibrio were the topmost abundant core taxa among the bacteria (Supplementary Figures S5). The most fungal core microbiome includes Capnodiales, Ascomycota, Chaetomiaceae, Fusarium, Aspergillus, Pseudeurotium, Alternaria, and Unclassified fungi (Supplementary Figures S6). The analysis of the composition of microbiomes (ANCOM) test was applied to these core communities to see whether genera if any, were statistically significant between the cultivar-type (Indica versus Japonica), compartment (root versus rhizosphere), and (control versus treatments) samples. The ANCOM results confirmed that a few genera are benchmarks for the differences across the groups (Supplementary Tables S11, S12 and Figures S7, S8). Most bacterial taxa at the compartment level were highly significant; for instance, 83 genera were significantly changed between the rhizosphere and root compartments (Supplementary Tables S11 and Figure S7). Other bacterial genera, such as Leptonema, Azospira, and Aquabacterium, significantly differed between the Indica and Japonica types. Fluviicola, Aquabacterium, and Leptonema were statistically significant between the four treatments (Supplementary Tables S11 and Figure S7). Similarly, in the case of fungi, members of Sordariales, Helotiales, Pleosporales, Pleosporaceae, Metarhizium, and Malassezia differed significantly among the root and rhizosphere compartments. Genera Cladosporium and members of Capnodiales were significantly varied under the control, Si, BM, and SiBM treatment (Supplementary Tables S12 and Figures S8).
3.5 Bacterial and fungal biomarkers under silicate and biofertilizer treatments
Linear Discriminate Size effect (LEfSe) approach was performed to check the effects on taxa (phylum to genera) by the host (Indica and Japonica), niche (rhizosphere and root), and the fertilizer treatments. The significant abundance of the bacterial and fungal biomarkers resulting from the fertilizer treatments in the Indica and Japonica rice is presented in (Figure 6). Those taxa with LDA (Linear discriminant analysis) scores >2 with a p-value <0.05 were considered significant. LDA is a statistical test that permits comparisons between treatments and subgroup comparisons within groups to determine taxa with significant variation in abundance across groups. In the Indica, with LDA scores >2, a total of 99 taxa belonging to 27 phyla were identified, including 34 taxa in BM, 33 taxa in Si, 29 in SiBM, and 4 taxa in control, (Supplementary Figure S9). Comparatively, more numbers of significant taxa were identified in samples inoculated with Bacillus Mucilagniosis or BM treatment than in the control, with significant phyla Actinobacteria order Gaiellales, Firmicutes, Bacillales (genera Bacillus, Fictibacillus, Paenibacillus, Tumebacillus), and Gp16 & Gp17 (Acidobacteria) had the highest abundance (Figure 6A). Likewise, taxa associated with phyla Verrucomicrobia, family Opitutaceae, Spirochaetes, family saprospiraceae, Proteobacteria, family Methylobacteriaceae, and Acetobacteraceae were significantly enriched in silicate (Si) treatment. In the combined treatment of silicate and biofertilizer (SiBM), phyla Bacteroidetes, order Cytophagales, Flavobacteriales, and Rhodocyclales were enriched. Only 4 genera were enriched in control compared to the other three treatments, including Nannocystis, Povalibacter, Ornatilinea, and Aquisphaera (Figure 6A). Simultaneously, 54 significant taxa were identified in the Japonica (Supplementary Figure S10). The two treatment groups, Si, and BM had the most numbers of significant taxa compared to the control (Figure 6B). Thaumarchaeota, Nitrososphaerales, Nitrososphaeraceae genera Nitrososphaera were highly abundant in BM treatment. Phyla Spirochaetes, Spirochaetales, Leptospiraceae genera Leptonema, and Deltaproteobacteria were enriched in Si treatment. Similarly, Opitutaceae (genera Opitutus), Desulfovibrionaceae (genera Desulfovibrio), Iamiaceae (genera Aquihabitans), and Cryomorphaceae (genera Fluviicola) were more abundant in SiBM. Interestingly, compared to treatment groups, only 6 taxa consisting of Acidobacteria_Gp21 and Hyphomonadaceae were abundant in the control (Figure 6B and Supplementary Figure S10). Unlike bacteria, the number of significant biomarkers taxa for the fungal community was fewer in the treatment groups compared to the control (Figure 6). In the Indica, 15 taxa were identified as significantly different, including 7 in control, 4 in BM, 2 in Si, and 1 in the SiBM (Supplementary Figure S11A). The most abundant phyla Pezizomycetes order Pezizales, Myxotrichaceae (genera Oidiodendron), and Cephalothecaceae in control; while Agaricomycetes, order Polyporales were highly abundant in Si treatment. Only one taxon belonging to Mycosphaerellaceae was significantly abundant in SiBM (Figure 6C). In the Japonica, 41 taxa were significantly different between the four groups, including 18 taxa in control, 1 in BM, 9 in Si, and 13 taxa in SiBM (Supplementary Figure S11B). Dothideomycetes, order Capnodiales, and family Cladosporiaceae (genera Cladosporium) were abundant in control. Saccharomycetes, family Saccharomycetaceae (genera Kazachstania) were most enriched in Si treatment. Glomeraceae (genera Funneliformis) were significantly more abundant in BM than in other treatments. Sordariomycetes, family Nectriaceae, and Mortierellaceae were highly abundant in SiBM (Figure 6D).
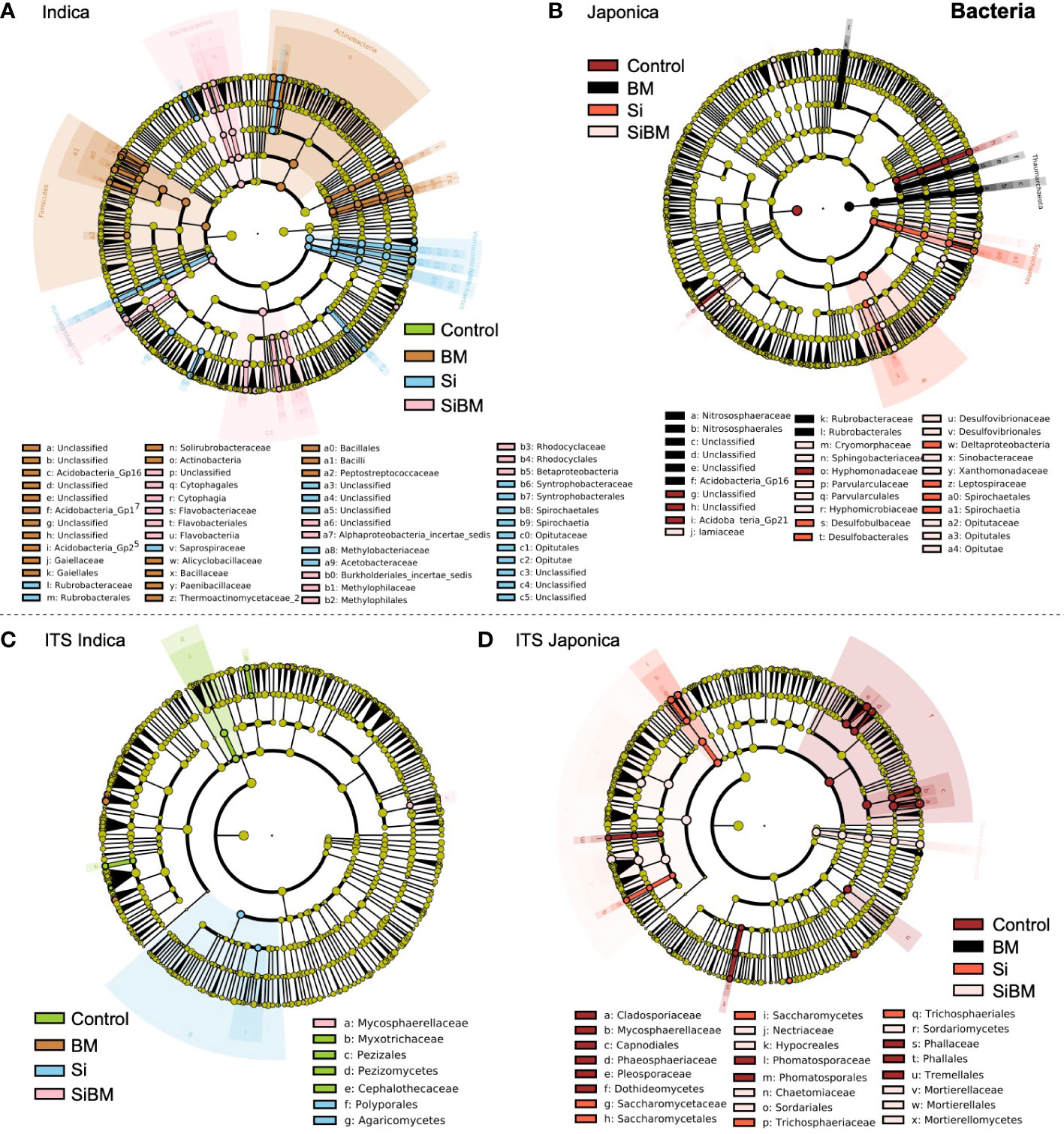
Figure 6 A linear discriminant analysis effect size (LEfSe) approach identifies significantly abundant taxa of 16S (A, B) and ITS (C, D) in Indica and Japonica under the fertilization treatments. The colored circles represent the significantly abundant taxa, with the color matching the corresponding treatments (green for CK, orange for biofertilizer (BM), blue for silicate (Si), and pink for silicate plus biofertilizer (SiBM) for Indica; and (red for CK, black for biofertilizer (BM), red for silicate (Si), and pink for silicate plus biofertilizer (SiBM) for Japonica. The six rings of the cladogram represent domain (innermost), phylum, class, order, family, and genus, and taxa with LDA values >2.0 are presented.
Moreover, 589 bacterial taxa were significantly different between the rhizosphere and root compartments. In the rhizosphere, a relatively large number of taxa (395) were significantly enriched with the major enriched taxa including, Acidobacteria, Actinobacteria (genera Gaiella, Solirubrobacter), Thaumarchaeota (genera Nitrososphaera), Planctomycetes, members of Planctomycetaceae, Nitrososphaeraceae, and Gaiellaceae (Supplementary Figure S12). Simultaneously, 194 taxa were significantly enriched in roots, and phylum Proteobacteria, its associated class Deltaproteobacteria (genera Desulfocapsa, Hydrogenophaga, and Desulfovibrio), Firmicutes subclass Clostridia (genera Clostridium_XlVa, Desulfosporomusa), Verrucomicrobia (genera Alterococcus) and Bacteroidetes (genera Ohtaekwangia) had the highest relative abundance (Supplementary Figure S12). In the case of fungi, 274 taxa belonging to 6 phyla were identified between the rhizosphere and root compartments. The rhizosphere had the most prominent differences with 229 significant fungal taxa; among them, Ascomycota subclass Sordariomycetes, Hypocreales (genera Humicola), Pseudeurotiaceae, Leotiomycetes (genera Pseudeurotium), Tremellomycetes, Mortierellomycetes were the most abundant. On the other hand, the root compartment had 45 significant taxa, with the most abundant taxa including, Dothideomycetes, order Pleosporales (genera Alternaria), Sordariomycetes (genera Fusarium and Zopfiella), and Basidiomycota order Agaricales (Supplementary Figure S12).
3.6 Soil physiochemical properties
Soil physiochemical analysis of pH, electric conductivity (EC), available silicon content (ASi), soil organic carbon (OC), total nitrogen (TN), available phosphorous content (AP), and available phosphorus (AP) was performed to check the alterations of these parameters under the addition of silicate, biofertilizer, or their combined applications. Results showed that OC and AP increased significantly (p < 0.05) under biofertilizer treatment (BM) compared to control, while EC decreased significantly (and Table 2). Although, under silicate application (Si), the pH, ASi, and AP increased significantly (p < 0.05), however, a significant reduction in TN was observed compared to the control. Interestingly, we found elevated significant levels of pH, OC, TN, ASi, and AP, under the combined application of silicate plus biofertilizer treatment (SiBM), except for the EC and AK content that was reduced significantly (and Table 2).
3.7 Rice microbial communities linked to soil physiochemical components
Redundant Discriminate Analysis (RDA) was applied to determine the association between the soil physiochemical variables, including pH, EC, OC, TN, ASi, AP, and AK, and the abundance of different microbes in the rice rhizosphere (Figure 7). Results revealed that major soil physiochemical components in the RDA of bacteria and fungi explained a substantial portion of the variation in the first two RDA axes (>90%), demonstrating that the content of soil-related components is influenced by both bacterial and fungal microbiomes. For instance, Bacteria of the phylum Spirochaetes, Bacteroidetes, Actinobacteria, and Proteobacteria showed a strong association in their abundance with pH, ASi, and AP, and Acidobacteria showed a strong negative association with pH and ASi; while Verrucomicrobia had a close association with AK (Figure 7A). Similarly, fungal phyla Mortierellomycota, and unassigned fungi showed a strong association with TN, AP, and OC, while Ascomycota had a negative association with ASi, and pH (Figure 7B). We also applied the Pearson correlation of coefficient analysis to identify the significant correlation of taxa with the soil physiochemical properties. We found that several bacterial and fungal taxa had a significant positive and negative correlation with the soil physiochemical constituents (Figures 7C, D). For instance, bacterial phylum Spirochaetes showed a highly significant positive correlation with pH and ASi. In contrast, Acidobacteria were significantly negatively correlated with pH and ASi but significantly positively correlated with OC. Bacteroidetes and Planctomycetes were found significantly positively correlated with AP and OC respectively. Both Cyanobacteria, and Verrucomicrobia were significantly negatively correlated with TN (Figure 7C). Likewise, fungal phyla Mortierellomycota showed a highly significant negative association with AK. Other taxa such as Ascomycota had negative correlation with ASi, and Chytridiomycota had highly negative correlation with TN, while unclassified fungi were found to be positively correlated with soil OC (Figure 7D). The results of Pearson test showed similar patterns of correlation as were observed for the RDA analysis.
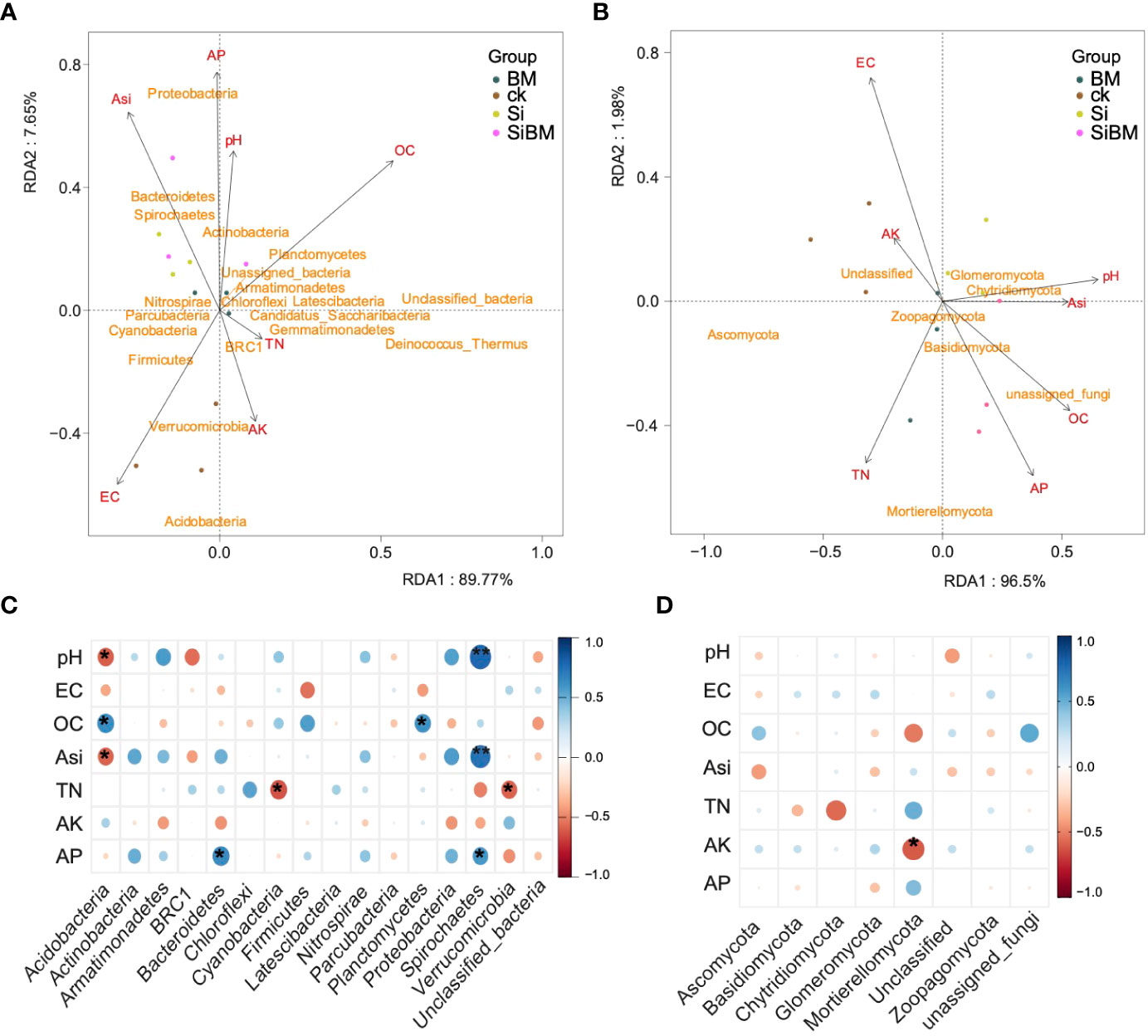
Figure 7 Redundancy discriminate analysis (RDA) approach identifies significantly abundant taxa of 16S (A) and ITS (B) and Pearson correlation of coefficient analysis of 16S (C) and ITS (D).
4 Discussion
Soil microbial biodiversity and composition are sensitive indicators of soil health and fundamental for the long-term sustainability of soil ecosystems, however, they are frequently influenced by agricultural perturbations (Dangi et al., 2020; Srour et al., 2020; Xue et al., 2020).Thus, a deeper understanding of the regulatory factors (drivers) governing the belowground microbiome is inevitable (Zhang et al., 2017). Although, some reports suggest that an appropriate fertilization regimen, combined with plant growth-promoting bacteria, can improve crop productivity by preserving soil fertility and modifying microbial community composition and abundance (Di Salvo et al., 2018; Jha et al., 2020). In contrast, chemical fertilizer application can suppress microorganisms’ growth and have a substantial negative impact on the bacterial diversity (Wang et al., 2021a). On the other hand, short-term application of slag-based silicate fertilizer, can improve the crop yield and alter the abundance of some bacteria, their associated functional genes, and saprophytic fungal communities in paddy soils (Das et al., 2019a; Das et al., 2019c). Yet, the degree to which the indigenous microbial community composition and diversity are affected either by the exogenous inoculation of bacteria as a biofertilizer, silicate fertilizer alone, or their combined application is largely unknown.
In this study, a fertilization experiment was established with silicate fertilizer, inoculation with single strain bacteria (Bacillus Mucilagniosis) as biofertilizer, and their combined application to investigate the shift in bacterial and fungal communities in two diverse eco-geographical rice types, i.e., Indica and Japonica. The results of our investigation have revealed a rich microbial population in the rhizosphere and roots of both Indica and Japonica rice. Fertilization with silicate and biofertilizer, host genetics, and plant compartments are all described as the major source of variance in the microbial communities tested. Rhizosphere compartment communities and cultivar Indica exhibited the highest α diversity, compared to the root tissue communities and the Japonica cultivar that showed the lowest alpha diversity (Figure 2). The same was true for the silicate fertilizer treatment which had the highest alpha diversity. Notably, the community diversity in the BM and mixed SiBM treatment soil was the lowest, as evidenced by the lowest Shannon indices (Supplementary Figure S4). This phenomenon may be caused by the fact that the combined treatment may cause a decrease in the biodiversity of the soil bacterial community overall while increasing the abundance of particular bacteria with specific functions. As a result, combined strain treatment may not be the optimum choice. The highest α diversity in the Indica samples suggests that the Indica root recruits more diverse taxa than the Japonica. This variation might be explained by differences in root exudate profiles formed by various rice cultivars, as root exudate spectra have been demonstrated to differ between varieties and cultivars of the same species (Eisenhauer et al., 2017). Our findings confirm the previously reported results where host genetics could influence the microbial diversity (Zhang et al., 2019). It is worth highlighting here that the community structure and composition we witnessed in each compartment are most likely not driven solely by plant genetics. The variations between the compartments might potentially be explained by microbial interactions including both cooperation and competition. Since soil microbes are sensitive to multiple fertilization treatments (Niu et al., 2019), types of microbial agents (Song et al., 2019), and various agronomic practices, causing varying degrees of changes in the microbial population structure. Therefore, the effects observed in this study such as increased α diversity in Si-treated samples could be the result of the silicon fertilizer and biofertilizer applied and soil chemical components, in addition to the plant host and plant compartments. The pattern of separation in the microbial communities (β diversity) between compartments was consistent across Indica and Japonica with a spatial gradient from the rhizosphere to the root for both bacterial and fungal populations (Figure 3). Similar trends of compartment separation from rhizosphere to root communities are reported in studies on the rice and tea microbiome (Edwards et al., 2015; Arafat et al., 2017). Both bacterial and fungal communities were affected by fertilization, as evidenced in PCoA plots where all the samples that were treated with biofertilizer or silicate fertilizer were clustered apart from the ones that were not treated regardless of cultivar type. These results suggest that biofertilizer and silicate fertilizer both are essential in reconstructing the structure of microbial communities.
We observed that bacterial phyla, including Proteobacteria, Acidobacteria, Actinobacteria, and two fungal phyla Ascomycota and Basidiomycota are the most prevalent groups that were consistently enriched in that perspective order, irrespective of the cultivar type and treatment (Figure 1). A recent study on the bacterial communities has shown the dominant trends of these phyla, that were affected under long-term fertilization and both silicate and microbial agent applications (Li et al., 2021; Yu et al., 2022). Both Ascomycota and Basidiomycota, are the two most dominant fungal phyla that participate in degradation processes (decomposers) (Morrison et al., 2016; Egidi et al., 2019). We observed that the relative abundance of proteobacteria increased and Acidobacteria decreased in the root compartment compared to rhizosphere soil, which mirrors the findings of the previous studies on the model plant Arabidopsis and rice (Bulgarelli et al., 2012; Edwards et al., 2015); suggesting the possibility that all land plants may have similar distributions of diverse bacterial phyla inside their roots. Within the Proteobacteria, the relative abundance of class Betaproteobacteria, Deltaproteobacteria, and class Actinobacteria were increased under the silicate and biofertilizer treatment (Figure 5). Betaproteobacteria are considered to be among the main inhabitants of the paddy rhizosphere and are believed to respond quickly to carbon availability (Das et al., 2016), their relative abundance was increased under silicate applications (Das et al., 2020). Contrary to our results, Deltaproteobacteria were not affected by the silicate application in the earlier study. Again, the inconsistencies in the outcomes of the two studies could be the result of differences in the sequencing depth and structural resolution, or soil type. Since most members of the silicate solubilizing bacteria belong to proteobacteria. The increase in the relative abundance of Proteobacteria members in our study is most likely due to the copiotrophic nature of these microorganisms to flourish in the belowground microenvironments relying on the root-derived carbon availability (Fierer et al., 2007; Peiffer et al., 2013). Similarly, the increased abundance of Actinobacteria under BM and Si treatments is in accordance with the previous results where the application of long-term silicate fertilizer raised the relative abundances of Actinobacteria as well as the abundance of the corresponding class, family, order, and genus (Samaddar et al., 2019). In this study, fungal taxa Sordariomycetes, and Leotiomycetes, were enriched in the root while Dothideomycetes, Unclassified Fungi, and Agaricomycetes, were found abundant in the rhizosphere. The presence of such taxa on different plant niches support the notion that plant compartments play a significant role in defining the composition of the plant-associated microbiota (Trivedi et al., 2020). Comparably, in our study, saprotrophic fungi like sordariomycetes increased in all samples regardless of treatment type, and class Dothideomycetes which comprises many plant pathogens depleted under both silicate and biofertilizer applications (Figure 5). Previously, it was established that exposure to silicate fertilizer could enhance the relative abundance of saprotrophic fungi (Das et al., 2019c). Although most bacterial genera were found to be equally present in all the treatments, some core genera, however, showed differential abundance patterns to both silicate and biofertilizer or in the combined application of both fertilizers. For instance, Gp6 (Acidobacteria) and Hydrogenophaga, were depleted in only treatments in which silicate was applied but not in biofertilizer treatment, while Desulfocapsa was enriched in the same silicate-treated samples; Indicating that these bacterial taxa are more sensitive to silicate fertilizer, either applied alone or in combination with biofertilizer. Previously it was shown that inoculation of a single bacterial strain or consortia can increase the abundance of certain bacterial taxa (Wang et al., 2021b; Wang et al., 2021d). In our study, Rhodocyclaceae and Sporomusa were highly abundant in biofertilizer and biofertilizer plus silicate, suggesting that application of bacterial strain Bacillus Mucilagniosis as biofertilizer has positive symbioses with these root-associated taxa. Likewise, fungal communities were more sensitive and highly affected by the plant host, compartments, and fertilizer treatments. The majority of Fusarium and Alternaria species in the Ascomycota division are the most significant plant pathogens, these endophytes are capable of producing mycotoxins in cereal crops like rice and potentially affecting human health through the food chain (Fones et al., 2020; Demers, 2022). Interestingly, we found that the application of silicate and biofertilizer suppresses the relative abundance of such pathogens, Alternaria and Fusarium. In contrast, Aspergillus, Capnodiales, and unclassified fungi increased. Previously such fungal genera were also reported as the core rice seed microbiome (Eyre et al., 2019). Previously it was shown that the dominant specie Aspergillus which is abundantly found in different soil habitats were increased under the silicate application (Das et al., 2019c).
The core microbiomes are thought to be a consistent and stable component of a particular ecosystem. Deciphering the core microbiome is therefore supposed to be critical in exposing the ecology of a microbial community since these core species are expected to perform important roles in the ecosystem. In our study, the ANCOM test confirmed that bacterial genera Leptonema, Azospira, Aquabacterium, Fluviicola, Aquabacterium, and Leptonema and fungal genera Metarhizium, and Malassezia, Cladosporium, and members of Capnodiales all as core microbiome and benchmarks for the significant differences across the treatment, compartment and cultivar type. ANCOM test is exceptionally sensitive for detecting taxa that appear in certain groups; it is also the only approach tested that has adequate false discovery rate control; however, it is less sensitive when the comparison is made between groups with less than 20 samples per group (Weiss et al., 2017). If the sample size is bigger, we expect to find more variations in the core microbiome that are specific to treatments. Additionally, we identified many treatment-specific biomarkers with substantial differential abundance patterns using the LfSe technique. The relative abundance of some important biomarkers, including Actinobacteria, Firmicutes, Bacillales, Bacteroides, Betaproteobacteria, and Desulfovibrionaceae, were significantly increased in soil treated with biofertilizer either alone or in combination with silicate fertilizer (Figure 6). Similarly, Verrucomicrobia, Spirochaetes, and Deltaproteobacteria were screened out to be potential biomarkers in Si treatment. Similar to this investigation, Xu et al., found that R. palustris inoculated by foliar spray boosted the abundance of the phylum Actinobacteria (Xu et al., 2016). According to Wang et al., Bacillus fusiformis L13, a compound microbial fertilizer application, enhanced the proportion of Firmicutes as well as the number of beneficial bacteria, such as Bacillales (Wang et al., 2021c). Bacillales are regarded as helpful bacteria due to their capacity to promote plant development while preventing the formation of phytopathogens. Verrucomicrobia composition and richness are particularly sensitive to changes in chemical variables related to soil fertility. When B. cepacia ISOP5 was inoculated, the Phyla Verrucomicrobia and Bacteroidetes were greatly affected, but under mixed consortia conditions, only Verrucomicrobia was significantly increased (Wang et al., 2021d). Members of Verrucomicrobia are capable to decompose recalcitrant organic material (Li et al., 2017) and might be vital for effectively decomposing organic matter and changing the amount of OM content in soils treated with bacterial inoculation. In contrast to our results, Deltaproteobacteria were not significantly changed when exposed to a silicate fertilizer (Das et al., 2020), this may be due to sequencing depth, structural resolution, or different soil used in the previous study. Unlike bacteria, fungal community significant biomarkers were fewer in the treatment groups compared to the control, suggesting that fungal communities are more sensitive and influenced by the silicate and biofertilizer treatments. Dothideomycetes, Capnodiales, Cladosporiaceae (genera Cladosporium) in control samples, Agaricomycetes, Polyporales, Saccharomycetaceae, and its associated genera Kazachstania, Mycosphaerellaceae, Sordariomycetes, Nectriaceae, and Mortierellaceae were found significantly abundant in the Si treatment alone or in combined treatment SiBM. In prior research, the silicate modification resulted in a rise in the relative abundance of fungal taxa like Sordariales and members of Mortierellaceae, the majority of which are saprotrophic fungi (Das et al., 2019c). It is well observed that in the presence of a readily available C supply, saprotrophic fungi expand, and the allocation of photosynthetic C to roots can stimulate the saprotrophic fungus growth (Anthony et al., 2017).
In this study, soil chemical components changed under exposure to silicate fertilizer, and these changes were also found to be associated with major bacterial and fungal phyla (Figure 7). For instance, pH, ASi, and AP were all elevated after adding silicate except TN, which was much lower than in control. Similar findings were observed in previous studies where the application of silicate fertilizer increased the soil pH and silicon content (Das et al., 2019a). A significant rose was observed in OC and AP, while EC dramatically dropped under bacterial inoculation. B.Mucilagniosis inoculation improved soil nutrient levels in this study, consistent with prior findings (Han and Lee, 2006). The significant increase in the soil components observed here most likely results from the P and K solubilizing bacteria B.Mucilagniosis. Previously, it was reported that the availability of P and K was greatly boosted by co-inoculation with P and K solubilizing bacteria inoculation alone or with rock P material (Ma et al., 2018). This study also provides unique information regarding the link between environmental variables, such as pH, ASi, and AP, with bacterial and fungal community diversity and the abundance of various groups in paddy soil. Spirochaetes had a strong positive relationship with pH and ASi. Acidobacteria, on the other hand, had a strong positive correlation with OC but a substantial negative correlation with pH and ASi. Bacteroidetes and Planctomycetes were significantly positively linked with both AP and OC. While Cyanobacteria and Verrucomicrobia were negatively associated with TN (Figure 7C). The fungus phylum Mortierellomycota, Ascomycota, and Chytridiomycota also showed an extremely substantial negative correlation with AK, ASi, and TN. Ascomycota and unclassified fungi were discovered to have a positive link with soil OC. Since, short-term bacterial inoculant and silicate fertilization affect the structure and composition of the soil microbial populations (Xu et al., 2016; Das et al., 2020). The current investigation using silicate fertilizer as well as bacterial strain (Bacillus Mucilagniosis) as biofertilizer revealed dramatic changes in the composition and structure of the total fungal and bacterial indigenous communities in the rhizosphere soil of rice. This might be due to the fact that the fertilization duration was short to have a major influence on the entire soil microbial community. Thus, compared to short-term fertilization, long-term fertilization may produce more consistent microbial community alterations.
5 Conclusion
This study established that slag silicate fertilizer and inoculation of bacterial strain Bacillus Mucilaginous as biofertilizers markedly altered the soil’s total bacterial and fungal community structure and composition; Such changes were mostly driven by plant compartments, soil nutrient availability, and pH. Despite being extremely different, the communities developed in the root tissues and rhizosphere were affected by the host’s genetic background and fertilization. There was a change in the content of several important soil physiochemical components. Specifically, we found that silicate application could increase root and rhizosphere compartments which was also positively and negatively linked with some of the root and rhizosphere microbes. Long-term use of the slag fertilizer might lead to the accumulation of heavy metals in the soil and boost its alkalinity, which may alter the topsoil physicochemical and biological attributes and crop yield. Multiple bacterial and fungal genera as key biomarkers identified in this study between silicate and biofertilizer treatments that can be utilized to improve plant health and agricultural production. This investigation was carried out in greenhouse settings to reduce the impact of different weather events on soil bacterial and fungal communities. More in-depth and long-term field research is required to validate the current observations in diverse soil types under varying climate conditions to reveal the microbial behavior and their implications on plant health and yield in response to silicate fertilizer application.
Data availability statement
The datasets presented in this study can be found in online repositories. The names of the repository/repositories and accession number(s) can be found in the article/Supplementary Material.
Author contributions
AM designed the study. AM, HW, XZ analyzed the data. AM and XZ performed experimental procedures and data analysis. AM, GJ, HW, and XZ were involved in data curation, experimental procedures, formal analysis, and validation. CH, BS, and AM acquired funding, wrote the original draft, and critically revised and reviewed the manuscript. All authors read and approved the final manuscript.
Funding
This study was supported by Science and Technology Service Platform of Shandong Academy of Sciences, Qilu University of Technology, Jinan, China, Shandong SME Productivity Promotion Center, Jinan, China, and Foreign Youth Talent Project, No. QN2022023003.
Acknowledgments
We thank the Jinan Daxin Biotechnology Co, Ltd., China for their technical facilities during this study and the Institute of Wetland Agriculture and Ecology, Shandong Academy of Agricultural Sciences, Jinan, China for providing the rice seeds.
Conflict of interest
Author GJ was employed by Shandong Siwenhouji Agricultural Development Co. Ltd.
The remaining authors declare that the research was conducted in the absence of any commercial or financial relationships that could be construed as a potential conflict of interest.
Publisher’s note
All claims expressed in this article are solely those of the authors and do not necessarily represent those of their affiliated organizations, or those of the publisher, the editors and the reviewers. Any product that may be evaluated in this article, or claim that may be made by its manufacturer, is not guaranteed or endorsed by the publisher.
Supplementary material
The Supplementary Material for this article can be found online at: https://www.frontiersin.org/articles/10.3389/fagro.2022.1071890/full#supplementary-material
References
Anthony M., Frey S., Stinson K. (2017). Fungal community homogenization, shift in dominant trophic guild, and appearance of novel taxa with biotic invasion. Ecosphere 8, e01951. doi: 10.1002/ecs2.1951
Arafat Y., Wei X., Jiang Y., Chen T., Saqib H. S. A., Lin S., et al. (2017). Spatial distribution patterns of root-associated bacterial communities mediated by root exudates in different aged ratooning tea monoculture systems. Int. J. Mol. Sci. 18 (8), 1727. doi: 10.3390/ijms18081727
Bolyen E., Rideout J. R., Dillon M. R., Bokulich N. A., Abnet C. C., Al-Ghalith G. A., et al. (2019). Reproducible, interactive, scalable and extensible microbiome data science using QIIME 2. Nat. Biotechnol. 37, 852–857. doi: 10.1038/s41587-019-0209-9
Breidenbach B., Pump J., Dumont M. G. (2015). Microbial community structure in the rhizosphere of rice plants. Front. Microbiol. 6, 1537. doi: 10.3389/fmicb.2015.015
Bulgarelli D., Rott M., Schlaeppi K., Ver Loren Van Themaat E., Ahmadinejad N., Assenza F., et al. (2012). Revealing structure and assembly cues for arabidopsis root-inhabiting bacterial microbiota. Nature 488, 91–95. doi: 10.1038/nature11336
Bulgarelli D., Schlaeppi K., Spaepen S., Ver Loren Van Themaat E., Schulze-Lefert P. (2013). Structure and functions of the bacterial microbiota of plants. Annu. Rev. Plant Biol. 64, 807–838. doi: 10.1146/annurev-arplant-050312-120106
Callahan B. J., Mcmurdie P. J., Rosen M. J., Han A. W., Johnson A. J. A., Holmes S. P. (2016). DADA2: High-resolution sample inference from illumina amplicon data. Nat. Methods 13, 581–583. doi: 10.1038/nmeth.3869
Caporaso J. G., Lauber C. L., Walters W. A., Berg-Lyons D., Lozupone C. A., Turnbaugh P. J., et al. (2011). Global patterns of 16S rRNA diversity at a depth of millions of sequences per sample. Proc. Natl. Acad. Sci. 108, 4516–4522. doi: 10.1073/pnas.1000080107
Dangi S., Gao S., Duan Y., Wang D. (2020). Soil microbial community structure affected by biochar and fertilizer sources. Appl. Soil Ecol. 150, 103452. doi: 10.1016/j.apsoil.2019.103452
Das S., Chou M. L., Jean J. S., Liu C. C., Yang H. J. (2016). Water management impacts on arsenic behavior and rhizosphere bacterial communities and activities in a rice agro-ecosystem. Sci. Total Environ. 542, 642–652. doi: 10.1016/j.scitotenv.2015.10.122
Das S., Gwon H. S., Khan M. I., Jeong S. T., Kim P. J. (2020). Steel slag amendment impacts on soil microbial communities and activities of rice (Oryza sativa l.). Sci. Rep. 10, 1–11. doi: 10.1038/s41598-020-63783-1
Das S., Gwon H. S., Khan M. I., Van Nostrand J. D., Alam M. A., Kim P. J. (2019a). Taxonomic and functional responses of soil microbial communities to slag-based fertilizer amendment in rice cropping systems. Environ. Int. 127, 531–539. doi: 10.1016/j.envint.2019.04.012
Das S., Kim G. W., Hwang H. Y., Verma P. P., Kim P. J. (2019b). Cropping with slag to address soil, environment, and food security. Front. Microbiol. 10, 1320. doi: 10.3389/fmicb.2019.013
Das S., Lee J. G., Cho S. R., Song H. J., Kim P. J. (2019c). Silicate fertilizer amendment alters fungal communities and accelerates soil organic matter decomposition. Front. Microbiol. 10, 2950. doi: 10.3389/fmicb.2019.02
Demers M. (2022). Alternaria alternata as endophyte and pathogen. Microbiology 168, 001153. doi: 10.1099/mic.0.001153
Deus A. C. F., De Almeida Bertani R. M., Meirelles G. C., Soares A.D.A.V.L., Moreira L. L. Q., Büll L. T., et al. (2018). “The comprehensive utilization of steel slag in agricultural soils,” in Recovery and utilization of metallurgical solid waste (London: IntechOpen). doi: 10.5772/intechopen.81440
Ding L. J., Cui H. L., Nie S. A., Long X. E., Duan G. L., Zhu Y. G. (2019). Microbiomes inhabiting rice roots and rhizosphere. FEMS Microbiol. Ecol. 95 (9), fiz040. doi: 10.1093/femsec/fiz040
Di Salvo L. P., Cellucci G. C., Carlino M. E., De Salamone I. E. G. (2018). Plant growth-promoting rhizobacteria inoculation and nitrogen fertilization increase maize (Zea mays l.) grain yield and modified rhizosphere microbial communities. Appl. Soil Ecol. 126, 113–120. doi: 10.1016/j.apsoil.2018.02.010
Edwards J., Johnson C., Santos-Medellin C., Lurie E., Podishetty N. K., Bhatnagar S., et al. (2015). Structure, variation, and assembly of the root-associated microbiomes of rice. Proc. Natl. Acad. Sci. U.S.A. 112, E911–E920. doi: 10.1073/pnas.1414592112
Egidi E., Delgado-Baquerizo M., Plett J. M., Wang J., Eldridge D. J., Bardgett R. D., et al. (2019). A few ascomycota taxa dominate soil fungal communities worldwide. Nat. Commun. 10, 2369. doi: 10.1038/s41467-019-10373-z
Eisenhauer N., Lanoue A., Strecker T., Scheu S., Steinauer K., Thakur M. P., et al. (2017). Root biomass and exudates link plant diversity with soil bacterial and fungal biomass. Sci. Rep. 7, 1–8. doi: 10.1038/srep44641
Eshed Y., Lippman Z. B. (2019). Revolutions in agriculture chart a course for targeted breeding of old and new crops. Science 366 (6466), eaax0025. doi: 10.1126/science.aax0025
Eyre A. W., Wang M., Oh Y., Dean R. A. (2019). Identification and characterization of the core rice seed microbiome. Phytobiomes J. 3, 148–157. doi: 10.1094/PBIOMES-01-19-0009-R
Fierer N., Bradford M. A., Jackson R. B. (2007). Toward an ecological classification of soil bacteria. Ecology 88, 1354–1364. doi: 10.1890/05-1839
Fones H. N., Bebber D. P., Chaloner T. M., Kay W. T., Steinberg G., Gurr S. J. (2020). Threats to global food security from emerging fungal and oomycete crop pathogens. Nat. Food 1, 332–342. doi: 10.1038/s43016-020-0075-0
Ghani J., Nawab J., Khan S., Khan M. A., Ahmad I., Ali H. M., et al. (2022). Organic amendments minimize the migration of potentially toxic elements in soil–plant system in degraded agricultural lands. Biomass Conversion Biorefinery, 1–19. doi: 10.1007/s13399-022-02816-3
Hacquard S., Spaepen S., Garrido-Oter R., Schulze-Lefert P. (2017). Interplay between innate immunity and the plant microbiota. Annu. Rev. Phytopathol. 55, 565–589. doi: 10.1146/annurev-phyto-080516-035623
Han H.-S., Lee K. (2006). Effect of co-inoculation with phosphate and potassium solubilizing bacteria on mineral uptake and growth of pepper and cucumber. Plant Soil Environ. 52, 130. doi: 10.17221/3356-PSE
Huang X., Kurata N., Wang Z.-X., Wang A., Zhao Q., Zhao Y., et al. (2012). A map of rice genome variation reveals the origin of cultivated rice. Nature 490, 497–501. doi: 10.1038/nature11532
Hussain Z., Alam M., Khan M. A., Asif M., Shah M. A., Khan S., et al. (2020). Bioaccumulation of potentially toxic elements in spinach grown on contaminated soils amended with organic fertilizers and their subsequent human health risk. Arabian J. Geosciences 13, 1–9. doi: 10.1007/s12517-020-05938-y
Hussien A., Abdul-Ratha H., Hadwan H. (2019). Evaluation use of bacillus mucilaginosus as biofertilizer interfere with glomus mosseae on growth and yield of corn. Iraqi J. Agric. Sci. 50, 64–75. doi: 10.1007/s12517-020-05938-y
Jacoby R., Peukert M., Succurro A., Koprivova A., Kopriva S. (2017). The role of soil microorganisms in plant mineral nutrition-current knowledge and future directions. Front. Plant Sci. 8, 1617. doi: 10.3389/fpls.2017.01617
Jha P. N., Gomaa A.-B., Yanni Y. G., El-Saadany A.-E. Y., Stedtfeld T. M., Stedtfeld R. D., et al. (2020). Alterations in the endophyte-enriched root-associated microbiome of rice receiving growth-promoting treatments of urea fertilizer and rhizobium biofertilizer. Microbial Ecol. 79, 367–382. doi: 10.1007/s00248-019-01406-7
Kent A. D., Triplett E. W. (2002). Microbial communities and their interactions in soil and rhizosphere ecosystems. Annu. Rev. Microbiol. 56, 211–236. doi: 10.1146/annurev.micro.56.012302.161120
Khan M. A., Ding X., Khan S., Brusseau M. L., Khan A., Nawab J. (2018). The influence of various organic amendments on the bioavailability and plant uptake of cadmium present in mine-degraded soil. Sci. total Environ. 636, 810–817. doi: 10.1016/j.scitotenv.2018.04.299
Lebreton L., Guillerm-Erckelboudt A. Y., Gazengel K., Linglin J., Ourry M., Glory P., et al. (2019). Temporal dynamics of bacterial and fungal communities during the infection of brassica rapa roots by the protist plasmodiophora brassicae. PLoS One 14, e0204195. doi: 10.1371/journal.pone.0204195
Lee H. J., Jeong S. E., Kim P. J., Madsen E. L., Jeon C. O. (2015). High resolution depth distribution of bacteria, archaea, methanotrophs, and methanogens in the bulk and rhizosphere soils of a flooded rice paddy. Front. Microbiol. 6, 639. doi: 10.3389/fmicb.2015.00639
Li Y., Liu X., Hao T., Chen S. (2017). Colonization and maize growth promotion induced by phosphate solubilizing bacterial isolates. Int. J. Mol. Sci. 18 (7), 1253. doi: 10.3390/ijms18071253
Liu S., Tang W., Yang F., Meng J., Chen W., Li X. (2017). Influence of biochar application on potassium-solubilizing bacillus mucilaginosus as potential biofertilizer. Prep Biochem. Biotechnol. 47, 32–37. doi: 10.1080/10826068.2016.1155062
Liu W., Xu X., Wu X., Yang Q., Luo Y., Christie P. (2006). Decomposition of silicate minerals by bacillus mucilaginosus in liquid culture. Environ. Geochem Health 28, 133–140. doi: 10.1007/s10653-005-9022-0
Li J., Wen Y., Yang X. (2021). Understanding the responses of soil bacterial communities to long-term fertilization regimes using DNA and RNA sequencing. Agronomy 11, 2425. doi: 10.3390/agronomy11122425
Li X., Wu Z., Li W., Yan R., Li L., Li J., et al. (2007). Growth promoting effect of a transgenic bacillus mucilaginosus on tobacco planting. Appl. Microbiol. Biotechnol. 74, 1120–1125. doi: 10.1007/s00253-006-0750-6
Ma M., Jiang X., Wang Q., Guan D., Li L., Ongena M., et al. (2018). Isolation and identification of PGPR strain and its effect on soybean growth and soil bacterial community composition. Int. J. Agric. Biol. 20, 1289–97. doi: 10.17957/IJAB/15.0627
Makabe-Sasaki S., Kakuda K.-I., Sasaki Y., Ando H. (2014). Effects of slag silicate fertilizer on silicon content of rice plants grown in paddy fields on the shounai plain, yamagata, Japan. Soil Sci. Plant Nutr. 60, 708–721. doi: 10.1080/00380768.2014.936305
Maleva M., Borisova G., Koshcheeva O., Sinenko O. (2017). Biofertilizer based on silicate solubilizing bacteria improves photosynthetic function of brassica juncea. AGROFOR 2 (3), 13–19. doi: 10.7251/AGRENG1703013M
Mi W., Sun Y., Xia S., Zhao H., Mi W., Brookes P. C., et al. (2018). Effect of inorganic fertilizers with organic amendments on soil chemical properties and rice yield in a low-productivity paddy soil. Geoderma 320, 23–29. doi: 10.1016/j.geoderma.2018.01.016
Morrison E. W., Frey S. D., Sadowsky J. J., Van Diepen L. T., Thomas W. K., Pringle A. (2016). Chronic nitrogen additions fundamentally restructure the soil fungal community in a temperate forest. Fungal Ecol. 23, 48–57. doi: 10.1016/j.funeco.2016.05.011
Mukhtar S., Mehnaz S., Malik K. A. (2021). Comparative study of the rhizosphere and root endosphere microbiomes of cholistan desert plants. Front. Microbiol. 12, 618742. doi: 10.3389/fmicb.2021.618742
Nacamulli C., Bevivino A., Dalmastri C., Tabacchioni S., Chiarini L. (1997). Perturbation of maize rhizosphere microflora following seed bacterization with burkholderia cepacia MCI 7. FEMS Microbiol. Ecol. 23, 183–193. doi: 10.1111/j.1574-6941.1997.tb00401.x
Nawab J., Ghani J., Khan S., Xiaoping W. (2018). Minimizing the risk to human health due to the ingestion of arsenic and toxic metals in vegetables by the application of biochar, farmyard manure and peat moss. J. Environ. Manage. 214, 172–183. doi: 10.1016/j.jenvman.2018.02.093
Nawab J., Khan S., Aamir M., Shamshad I., Qamar Z., Din I., et al. (2016). Organic amendments impact the availability of heavy metal (loid) s in mine-impacted soil and their phytoremediation by penisitum americanum and sorghum bicolor. Environ. Sci. pollut. Res. 23, 2381–2390. doi: 10.1007/s11356-015-5458-7
Nawab J., Khan N., Ahmed R., Khan S., Ghani J., Rahman Z., et al. (2019). Influence of different organic geo-sorbents on spinacia oleracea grown in chromite mine-degraded soil: a greenhouse study. J. Soils Sediments 19, 2417–2432. doi: 10.1007/s11368-019-02260-3
Ning D., Song A., Fan F., Li Z., Liang Y. (2014). Effects of slag-based silicon fertilizer on rice growth and brown-spot resistance. PLoS One 9, e102681. doi: 10.1371/journal.pone.0102681
Niu C., Geng G., Yu L., Xie Q., Liao J., Qi H. (2019). Reducing fertilizer input combined with application of trichoderma to increase yield, quality of melon and soil microbial functional diversity. J. Plant Nutr. Fertil 25, 620–629.
Oka H. (1958). Intervarietal variation and classification of cultivated rice. Ind. J. Genet. Plant Breed. 18, 79–89. doi: 10.4236/ajps.2011.24068
Peiffer J. A., Spor A., Koren O., Jin Z., Tringe S. G., Dangl J. L., et al. (2013). Diversity and heritability of the maize rhizosphere microbiome under field conditions. Proc. Natl. Acad. Sci. 110, 6548–6553. doi: 10.1073/pnas.1302837110
Pirttila A. M., Mohammad Parast Tabas H., Baruah N., Koskimaki J. J. (2021). Biofertilizers and biocontrol agents for agriculture: How to identify and develop new potent microbial strains and traits. Microorganisms 9 (4), 817. doi: 10.3390/microorganisms9040817
Samaddar S., Truu J., Chatterjee P., Truu M., Kim K., Kim S., et al. (2019). Long-term silicate fertilization increases the abundance of actinobacterial population in paddy soils. Biol. Fertility Soils 55, 109–120. doi: 10.1007/s00374-018-01335-6
Shi C. (2004). Steel slag–its production, processing, characteristics, and cementitious properties. J. materials civil Eng. 16, 230–236. doi: 10.1061/(ASCE)0899-1561(2004)16:3(230)
Song Y., Yu J., Chen S., Xiao C., Li Y., Su X., et al. (2019). Effects of complex microbial agent on cotton physiological characteristics, microorganism and physicochemical properties in rhizosphere soil. Soils 51, 477–487. doi: 10.3389/fmicb.2022.993147
Srour A. Y., Ammar H. A., Subedi A., Pimentel M., Cook R. L., Bond J., et al. (2020). Microbial communities associated with long-term tillage and fertility treatments in a corn-soybean cropping system. Front. Microbiol. 11, 1363. doi: 10.3389/fmicb.2020.01363
Sun A., Jiao X.-Y., Chen Q., Wu A.-L., Zheng Y., Lin Y.-X., et al. (2021). Microbial communities in crop phyllosphere and root endosphere are more resistant than soil microbiota to fertilization. Soil Biol. Biochem. 153, 108113. doi: 10.1016/j.soilbio.2020.108113
Tong W., Yu J., Wu Q., Hu L., Tabys D., Wang Y., et al. (2021). Black tea quality is highly affected during processing by its leaf surface microbiome. J. Agric. Food Chem. 69, 7115–7126. doi: 10.1021/acs.jafc.1c01607
Trivedi P., Leach J. E., Tringe S. G., Sa T., Singh B. K. (2020). Plant–microbiome interactions: from community assembly to plant health. Nat. Rev. Microbiol. 18, 607–621. doi: 10.1038/s41579-020-0412-1
Ullah I., Khan S., Nawab J., Khan M. A., Jehan S. (2022). Hardwood modified and unmodified biochar amendments used for saline alkali soil remediation: phosphorus availability and its plant uptake. Arabian J. Geosciences 15, 1–12. doi: 10.1007/s12517-022-10157-8
Wang F., Chen S., Qin S., Sun R., Zhang Y., Wang S., et al. (2021a). Long-term nitrogen fertilization alters microbial community structure and denitrifier abundance in the deep vadose zone. J. Soils Sediments 21, 2394–2403. doi: 10.1007/s11368-021-02931-0
Wang B., Chu C., Wei H., Zhang L., Ahmad Z., Wu S., et al. (2020). Ameliorative effects of silicon fertilizer on soil bacterial community and pakchoi (Brassica chinensis L.) grown on soil contaminated with multiple heavy metals. Environ. pollut. 267, 115411. doi: 10.1016/j.envpol.2020.115411
Wang X., Ji C., Song X., Liu Z., Liu Y., Li H., et al. (2021c). Biocontrol of two bacterial inoculant strains and their effects on the rhizosphere microbial community of field-grown wheat. BioMed. Res. Int. 12, 693535. doi: 10.1155/2021/8835275
Wang J., Liu L., Gao X., Hao J., Wang M. (2021b). Elucidating the effect of biofertilizers on bacterial diversity in maize rhizosphere soil. PLoS One 16, e0249834. doi: 10.1371/journal.pone.0249834
Wang W., Mauleon R., Hu Z., Chebotarov D., Tai S., Wu Z., et al. (2018). Genomic variation in 3,010 diverse accessions of Asian cultivated rice. Nature 557, 43–49. doi: 10.1038/s41586-018-0063-9
Wang Y., Peng S., Hua Q., Qiu C., Wu P., Liu X., et al. (2021d). The long-term effects of using phosphate-solubilizing bacteria and photosynthetic bacteria as biofertilizers on peanut yield and soil bacteria community. Front. Microbiol. 12. doi: 10.3389/fmicb.2021.693535
Wei G., Ning K., Zhang G., Yu H., Yang S., Dai F., et al. (2021). Compartment niche shapes the assembly and network of cannabis sativa-associated microbiome. Front. Microbiol. 12, 714993. doi: 10.3389/fmicb.2021.714993
Weiss S., Xu Z. Z., Peddada S., Amir A., Bittinger K., Gonzalez A., et al. (2017). Normalization and microbial differential abundance strategies depend upon data characteristics. Microbiome 5, 1–18. doi: 10.1186/s40168-017-0237-y
White T. J., Bruns T., Lee S., Taylor J. (1990). Amplification and direct sequencing of fungal ribosomal RNA genes for phylogenetics. PCR protocols: guide to Methods Appl. 18, 315–322. doi: 10.1016/B978-0-12-372180-8.50042-1
Xue L., Ren H., Brodribb T. J., Wang J., Yao X., Li S. (2020). Long term effects of management practice intensification on soil microbial community structure and co-occurrence network in a non-timber plantation. For. Ecol. Manage. 459, 117805. doi: 10.1016/j.foreco.2019.117805
Xu J., Feng Y., Wang Y., Luo X., Tang J., Lin X. (2016). The foliar spray of rhodopseudomonas palustris grown under stevia residue extract promotes plant growth via changing soil microbial community. J. soils sediments 16, 916–923. doi: 10.1007/s11368-015-1269-1
Yilmaz P., Parfrey L. W., Yarza P., Gerken J., Pruesse E., Quast C., et al. (2014). The SILVA and “all-species living tree project (LTP)” taxonomic frameworks. Nucleic Acids Res. 42, D643–D648. doi: 10.1093/nar/gkt1209
Yu P., Hochholdinger F. (2018). The role of host genetic signatures on root-microbe interactions in the rhizosphere and endosphere. Front. Plant Sci. 9, 1896. doi: 10.3389/fpls.2018.01896
Yu Y., Zhang L., Li Y., Hou L., Yang H., Shi G. (2022). Silicon fertilizer and microbial agents changed the bacterial community in the consecutive replant soil of lilies. Agronomy 12, 1530. doi: 10.3390/agronomy12071530
Zhang J., Liu Y.-X., Zhang N., Hu B., Jin T., Xu H., et al. (2019). NRT1. 1B is associated with root microbiota composition and nitrogen use in field-grown rice. Nat. Biotechnol. 37, 676–684. doi: 10.1038/s41587-019-0104-4
Zhang X., Zhang R., Gao J., Wang X., Fan F., Ma X., et al. (2017). Thirty-one years of rice-rice-green manure rotations shape the rhizosphere microbial community and enrich beneficial bacteria. Soil Biol. Biochem. 104, 208–217. doi: 10.1016/j.soilbio.2016.10.023
Keywords: Biofertilizer, Bacillus Mucilagniosis, Microbial responses, Rice, Rhizosphere, Silicate fertilizer
Citation: Mallano AI, Zhao X, Wang H, Jiang G, Sun B and Huang C (2022) Divergent taxonomic responses of below-ground microbial communities to silicate fertilizer and biofertilizer amendments in two rice ecotypes. Front. Agron. 4:1071890. doi: 10.3389/fagro.2022.1071890
Received: 17 October 2022; Accepted: 30 November 2022;
Published: 21 December 2022.
Edited by:
Waheed Ullah, Kohat University of Science and Technology, PakistanReviewed by:
Zahid Ullah, China University of Geosciences Wuhan, ChinaAziz Ullah, Kohat University of Science and Technology, Pakistan
Copyright © 2022 Mallano, Zhao, Wang, Jiang, Sun and Huang. This is an open-access article distributed under the terms of the Creative Commons Attribution License (CC BY). The use, distribution or reproduction in other forums is permitted, provided the original author(s) and the copyright owner(s) are credited and that the original publication in this journal is cited, in accordance with accepted academic practice. No use, distribution or reproduction is permitted which does not comply with these terms.
*Correspondence: Chao Huang, Y2F1aGNoQGhvdG1haWwuY29t; Botong Sun, c3Vubnlib3RvbmdAMTYzLmNvbQ==