- 1Indaba Agricultural Policy Research Institute, Lusaka, Zambia
- 2Norwegian University of Life Sciences, School of Economics and Business, Ås, Norway
- 3Center for International Forestry Research, Bogor, Indonesia
- 4Department of Agricultural, Food and Resource Economics, Michigan State University, East Lansing, MI, United States
Conservation Agriculture (CA) aims to concurrently promote agricultural productivity, local livelihoods, climate resilience and other environmental objectives. We review the emerging evidence base in Eastern and Southern Africa to address whether CA is climate smart and why adoption rates by smallholders remain generally very low. We first develop an adoption framework that can be used to assess when and where the different components of CA are expected to be adopted under different conditioning factors and consider options to make CA climate smart. Our results suggest that CA can contribute positively to productivity and adaptation/resilience objectives, although the degree of success varies considerably by farm, household and regional characteristics. Overall, we find that capital-intensive (mechanized) CA is more likely to be adopted in areas of economic dynamism where capital is cheap relative to labor. Labor-intensive CA practices are more likely to be adopted in regions of economic stagnation where capital is expensive, and labor is abundant and cheap. A subnational focus is needed to identify economic conditions of different regions and agro-ecological zones and to test hypotheses derived from the framework in this paper and to propose the most appropriate CA packages for promotion. Our findings suggest that labor using variants of CA such as planting basins are more likely to be adopted than are capital using mechanized options in densely populated parts of Malawi, Ethiopia, Kenya, Tanzania, Zambia and Zimbabwe where labor is abundant, and presumably cheap, but capital is expensive. However, rising land scarcity (prices) and wages in the region present an opportunity for capital intensive, mechanized CA operations to be adopted if the cost of capital can be kept low and if there is a supportive environment for mechanization. We conclude that CA is climate smart and if adopted widely, it has the potential to help build resilience in smallholder farming systems. CA can be more climate smart, and its uptake can be enhanced by reframing, better targeting, adapting CA to location-specific economic and biophysical, and through greater and more effective public spending on agricultural research and development.
Introduction
The tight nexus between climate change and rural livelihoods is becoming increasingly clear. The fifth assessment report (AR5) of the Intergovernmental Panel on Climate Change (IPCC) concludes that climate change will worsen multidimensional poverty and create new poor in most developing countries including rural sub-Saharan Africa (SSA) (Olsson et al., 2014). For these regions, agriculture is the primary impact channel for climate change because the majority of rural households depend on rainfed farming for their livelihoods (Thurlow et al., 2012; Hallegatte et al., 2016). Climate change directly affects agricultural incomes, food security, and poor people's ability to escape poverty. Indirectly, climate change alters factor price ratios, and thus the course of technical innovation and entire food systems (Olsson et al., 2014; Porter et al., 2014).1
SSA is projected to receive less rainfall, which will negatively affect long-term crop yield in the region (Lobell et al., 2008; Niang et al., 2014). In Zambia, rainfall is projected to decline by 3 and 0.6% by 2050 and 2100, while temperature is projected to increase by 1.9°C and 2.3°C by 2050 and 2100 (Hamududu and Ngoma, 2019). These changes in temperature and rainfall will likely reduce water availability by 13% in 2100 in the country (Hamududu and Ngoma, 2019), with significant differences between regions (the southern part likely to become the most affected).
The challenge for the region is twofold: (i) to raise agricultural productivity to feed a growing population, projected to reach 2 billion by 2050 (Canning et al., 2015) and to meet changing dietary preferences, and (ii) to address the negative consequences of current and projected climate change and strengthen the resilience of the region's agrifood systems. The suite of conservation agriculture (CA) practices is widely considered part of the solution that can reduce downside crop yield loss from extreme weather events and may therefore contribute to the climate smart agriculture (CSA) objectives of (i) raising productivity and household income, (ii) enhancing climate adaptation and resilience, and (iii) reducing greenhouse gas (GHG) emissions from agriculture (Thierfelder et al., 2017). Resilience is the capacity of rainfed farming systems to cope with current and projected climate change and variability so that they maintain their main functions (IPCC, 2014). Resilience can also be defined as the ability of a system to respond to transitory effects such as shocks or the more persistent adverse trends such as stressors (Hoddinott, 2014). In the spirit of Barrett and Constas (2014), resilience can be defined in terms of well-being movements; a resilient household is one that was never poor over the time considered or one that escaped from poverty.
CA is built on three principles: (i) minimum tillage (MT), (ii) in-situ crop residue retention to provide mulch, and (iii) diversified crop rotation and intercropping. CA is considered an attractive option to intensify agricultural production and to enhance resilience in rainfed farming systems in SSA (Thierfelder and Wall, 2010; Arslan et al., 2014; IPCC, 2014; Thierfelder et al., 2015b, 2017; Droppelmann et al., 2017). However, there are questions raised on the actual extent of CA adoption (Andersson and D'Souza, 2014), its compatibility with smallholder farming systems in the region (Giller et al., 2009; Andersson and Giller, 2012), yield benefits in the short-term (Corbeels et al., 2020), and its impacts on carbon sequestration, mitigation, deforestation and environmental efficiency (Powlson et al., 2014, 2016; Abdulai and Abdulai, 2016; Ngoma et al., 2018a). There are mixed results on its yield and welfare effects (Ngwira et al., 2012; Arslan et al., 2015; Ngoma et al., 2015; Thierfelder et al., 2015b, 2016; Abdulai, 2016; Ng'ombe et al., 2017; Ngoma, 2018; Corbeels et al., 2020).2 Ndah et al. (2018) call for more flexibility in the definition of CA so that farmers can adapt the practices to their local contexts.
We review the available evidence and assess the extent to which CA, as currently practiced in Eastern and Southern Africa (ESA), contributes to two out of the three core CSA objectives of raising productivity and incomes, and building resilience to climate change. The paper complements and adds to existing literature in two main ways. First, we provide a comprehensive review of the evidence on CA uptake and its impacts, incorporating a considerable body of new studies in ESA, and addressing the extent to which CA achieves CSA and sustainable agricultural intensification (SAI) targets. Second, drawing upon Boserupian and induced innovation theory (Boserup, 1965; Hayami and Ruttan, 1971), we develop a framework that can be used to assess when the different components of CA are expected to be adopted under different conditioning factors and propose ways to improve CA's contribution to SAI. We hypothesize that population density and changes in land, labor and capital prices can explain CA adoption in different ESA contexts.
Such an analysis is important given the potentially important role that CA may plan in promoting sustainable intensification in the region (Baudron et al., 2009; Govaerts et al., 2009; Thierfelder and Wall, 2010; UNEP, 2013; IPCC, 2014; Powlson et al., 2016; Droppelmann et al., 2017; Mockshell and Kamanda, 2018).3 CA garners strong political support in the region. For example, CA is part of the regional agricultural policies by the New Partnership for Africa's Development (NEPAD), the Common Market for Eastern and Southern Africa's (COMESA) and the Southern African Development Community (SADC) (Giller et al., 2015). CA is part of national policies in several SSA countries, including Tanzania, Kenya, Malawi, Mozambique, Zimbabwe, Zambia, and Lesotho (Giller et al., 2015).
What Constitutes Conservation Agriculture (CA) Adoption?
Much of the debate on the extent of CA uptake boils down to lack of a common understanding on what CA adoption means (Andersson and D'Souza, 2014; Glover et al., 2016). Following Ngoma (2016), a fundamental question is how to determine whether a farmer qualifies as an adopter. Does a farmer have to use one, two, or all three core principles of CA each year? Should CA be applied to all, most, or even a fraction of her cultivated land in order to qualify as an adopter? The fact that some CA elements such as rotations and intercropping are old-age practices prevalent under conventional farming raises the question whether a farm that practices crop rotations or intercropping alone should be counted as a CA adopter. While it may not be necessary for analysts and practitioners to agree on the answers to such questions, it would be a step forward analytically if studies explained clearly how CA adoption is defined; it would make it easier to assess why adoption rates apparently differ across studies in the same area.
In this study, we define MT as a tillage system with reduced soil disturbance where tillage is done only in planting stations and the rest of the soil is left undisturbed. MT has three main variants: ripping, planting basins and zero-tillage. Rip lines are made with ox- or tractor-drawn rippers, planting basins are made with hand-hoes. Zero-tillage is based on handheld or mechanized direct planters. MT is the most prevalent and non-negotiable CA component in Zambia. Other CA components include mulching and crop rotation or intercropping. Residue retention requires leaving at least 30% of crop residues to serve as mulch or cover crop, while crop rotation involves planting cereals and nitrogen-fixing legumes in succession on the same plot in order to improve soil fertility (Haggblade and Tembo, 2003). Full adoption involves use of all three CA principles, i.e., minimum tillage, residue retention and crop rotation (or intercropping); partial adoption involves the use of MT alone or with either rotation/ intercropping or with residue retention.
A Framework of Expected CA Adoption Under Various Economic Contexts
We adopted the framework proposed in Jayne et al. (2019) to characterize when the adoption of CA is expected to be high or low, depending on different economic contexts. This framework is underpinned by the induced innovation and the Boserup hypotheses and provides a means to identify pathways that can explain CA adoption (Figure 1). The induced innovation hypothesis postulates that factors prices influence adoption of innovations in agricultural development, while the Boserup hypothesis suggests that, if land is abundant, farmers will tend to practice extensive agriculture before intensifying (Boserup, 1965; Hayami and Ruttan, 1971). As land scarcity is a key determinant of relative land prices, the frameworks are overlapping. In this paper, we extend the basic framework in Jayne et al. (2019) and propose alternative options to strengthen CA's contributions to CSA under each adoption scenario.
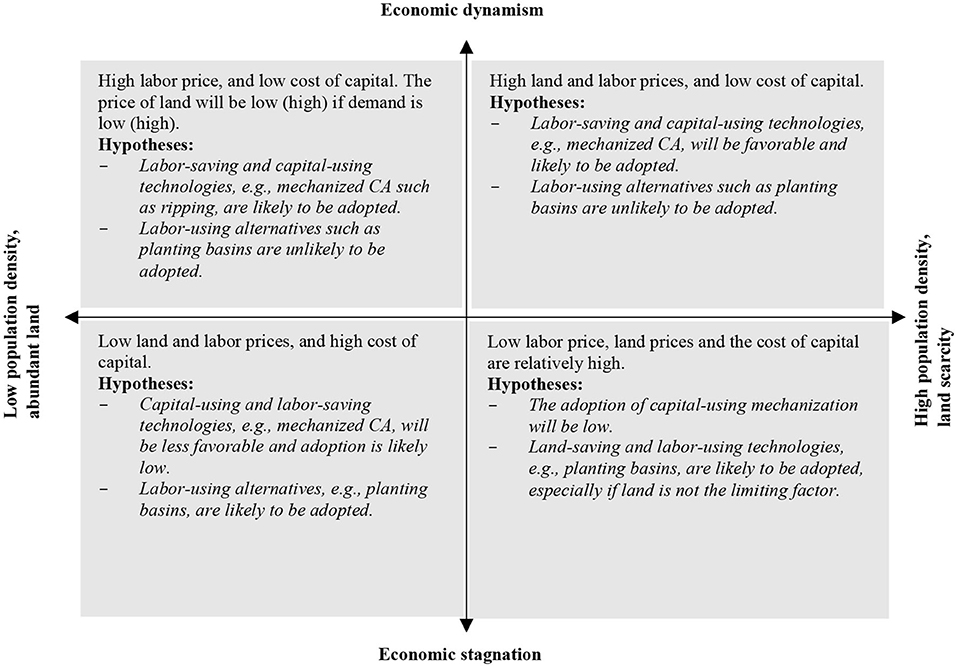
Figure 1. Expected adoption of different elements of conservation agriculture as determined by population density and economic dynamism.
To keep things tractable, we only consider two conditioning (exogenous) factors: economic dynamism and population density. We assess how these exogenous factors are likely to affect factor prices for labor, land, and capital, and therefore CA adoption. We posit that land, labor and capital are necessary for CA adoption, see section on Drivers of CA Adoption. Economic dynamism is characterized by relatively well-functioning markets for agricultural inputs, commodities and credit, and growth in real wages; economic stagnation is the polar opposite. The continuum of economic dynamism is represented by the vertical axis in Figure 1. The horizontal axis represents the population density continuum (the Boserupian dimension), ranging from land-abundant, low population density settings on the left to densely populated settings on the right where land is scarce and real land values are rising rapidly.
High land and labor costs, and low cost of capital characterize the NE quadrant in Figure 1, where there is economic dynamism and high population density. The low cost of capital is likely to facilitate the adoption of capital-using, labor-saving and land-saving mechanized CA technologies such as minimum tillage ripping and zero tillage. The high cost of labor and land will, however, limit the adoption of labor-intensive CA such as planting basins, and land-using extensive agriculture.
Low population density, abundant land and economic stagnation is associated with low land and labor costs, and high cost of capital in the SW quadrant. Under this context, capital-using and labor-saving technologies such as mechanized CA will be less favorable, while labor-using alternatives such as planting basins as well as land-using extensive or conventional agriculture are likely to be adopted. Labor-intensive CA will only be adopted in this case if its returns are superior to conventional agriculture. Labor-intensive farming systems, whether it is CA or conventional agriculture will only be adopted if the opportunity cost of labor is low.
Under low population density and economic dynamism in the NW quadrant, the cost of capital is expected to be low, while the cost of labor will be high. Given that land is abundant, the land price will be low (high) if demand is low (high). In this case, labor-saving and capital-using technologies such mechanized CA will be more likely to be adopted than are labor-using alternatives such as planting basins.
With high population density and economic stagnation in the SE quadrant, the land price is expected to be high, while the cost of labor will be low. The cost of capital will depend on its effective demand as created by markets, but it is likely to be high given economic stagnation. We would expect the adoption of capital-using mechanization to be low (high) if the cost of capital is high (low), whereas land-saving and labor-using technologies such as planting basins are likely to be adopted. Land- and labor-using extensive agriculture will be adopted if land is not the limiting factor and if the returns from labor-using CA are not higher than returns from conventional agriculture.
In sum, Figure 1 suggests that economic and demographic conditions shape the specific technical pathways for CA adoption. Capital-intensive or mechanized CA is more likely to be adopted under economic dynamism where capital is cheaper but labor is expensive, while labor-intensive CA practices are more likely to be adopted under economic stagnation where capital is expensive, and labor is cheap and abundant. This typology, if it conforms to the empirical realities on the ground, may be utilized to provide location-specific targeting of CA promotion programs in SSA. Equally important is a better understanding of how best CA practices for these varied locations can become more climate smart and hence better contribute to the SAI objectives of raising productivity, farm incomes and adaptation, and mitigation.
Data and Approaches
We use data from studies published in peer-reviewed journals on SSA between 2007 and 2018 to identify drivers of CA adoption. To do this, we searched for “Adoption of Conservation Agriculture” in “Sub-Saharan Africa” and “Impacts” of “Conservation Agriculture on yield, livelihoods or carbon sequestration/deforestation” in “Sub-Saharan Africa” in Google Scholar and Scopus. This search returned about 55 articles published between 2007 and January 2018. For the forest plots, we narrowed down our analysis to include 15 distinct studies4 that focus on discernible and well-defined agricultural technologies (minimum tillage, planting basins, ripping, full CA, agroforestry, pigeon pea, improved seed etc.) and report both the effect size (marginal or average partial effects) and the standard error of the estimates. By these metrics, our list of key studies is biased toward those in economics and mostly from ESA but additionally, we reviewed a large body of on-station experimental studies, as can be seen from the discussions.
Data on adoption should be viewed with critical eyes, as there might be several biases. Adverse selection and incentive (“moral hazard”) problems can influence “official” CA adoption rates. Adverse selection may manifest where the “wrong” farmers (e.g., project-dependent) are targeted by CA projects as beneficiaries. Such farmers may pretend to adopt some CA components for as long as they receive project benefits (e.g., input vouchers) but they still maintain most of their cultivated land under conventional tillage or are quick to revert to conventional methods as they await the next project (Ngoma et al., 2016). This leads to problems of inclusion and exclusion where deserving farmers are excluded and those who are not supposed to be in the program are included. In other instances, adoption estimates are intentionally over-reported (e.g., by the choice of definitions) to impress funding agencies or serve other interests.
There are two limitations of this study worthy to be pointed out. First, the methods used – although aligned to – do not follow the standard systematic review or discourse analysis approaches but instead review only the main studies on CA in parts of SSA published between 2007 and early 2018. Second, although the study draws evidence from SSA, the studies reviewed do not represent the entire region but are biased toward ESA.
Drivers of CA Adoption
The age-old question about drivers of agrarian change remains relevant given the strategic position of CA as a means to address climate change, increase food production and household incomes in SSA and contribute to SAI. We used forest plots to collate results from various studies on the effects of access to credit, farm size, and labor availability on CA adoption. These factors are major impediments to the spread of CA in the region (Thierfelder et al., 2015b) and are used here as proxies for the two dimensions of economic dynamism and population density in Figure 1. For each factor, we present the results in a forest plot (on the left) showing the study name, year, country, effect size, explanatory variable/factor and the specific technology. Each forest plot is accompanied by a funnel plot (on the right) to measure publication bias or small study effects.
A forest plot is a graphical display of estimated results from a number of scientific studies addressing the same question, along with the overall results. The horizontal lines in the forest plots are the confidence intervals (CIs) and the gray areas are study weights generated in the meta function. The dotted vertical line in the left panel shows the overall/combined effects. CIs that cross the zero line indicate statistical insignificance with those on the left and right showing negative and positive statistically significant effects, respectively. Adoption in these studies is mainly defined as any use of a given practice by farmers.
Although the factors affecting CA adoption do not directly align with the two dimensions of Figure 1, they do so indirectly. Easy access to cheap credit and high labor availability should be associated with economic dynamism, and large farm size associated with land abundance (low population density).
Effects of Access to Credit on CA Adoption
Although access to credit is considered a major enabler of technology adoption in agriculture, the evidence for CSA practices more broadly and CA in particular is mixed in ESA (Figure 2). For the studies reviewed, the effect of access to credit on CA adoption is negative and statistically insignificant on average, suggesting that farmers in the region face other immediate non-financial constraints to adoption. In the lens of our adoption framework in Figure 1, these findings suggest that the studies reviewed here were done in countries or parts of countries that fit into the SE or SW quadrants where either land and labor prices are low, and capital is expensive (SE), or land and capital prices are relatively high but labor is abundant and cheap (SW). In either context, labor using variants of CA such as planting basins are more likely to be adopted than are capital using mechanized options. In this case, it is expected that access to credit might not be the most liming factor for CA adoption. This would be expected in parts of Ethiopia, Tanzania and Zambia, the host countries for studies collated in Figure 2.
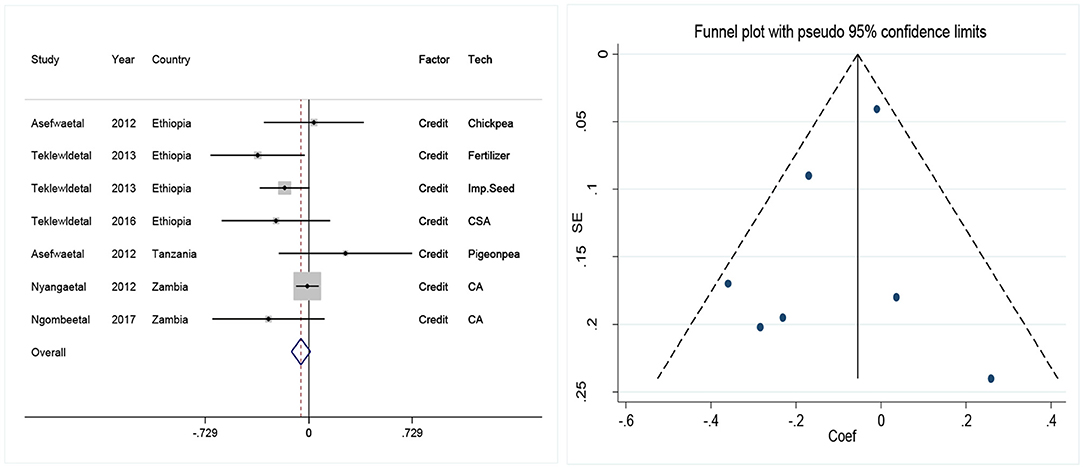
Figure 2. The effects of access to credit on the adoption of various CSA practices in parts of eastern and southern Africa. Source: Computed from Asfaw et al. (2012), Nyanga (2012), Teklewold et al. (2013), and Ng'ombe et al. (2017). The horizontal lines in the forest plots are the confidence intervals (CIs) and the gray areas are study weights generated in the meta function. The dotted vertical line in the left panel shows the overall/combined effects. CIs that cross the zero line indicate statistical insignificance, while those on the left (right) show statistically significant negative (positive) effects. CSA denotes combined technologies.
Effects of Farm Size on CA Adoption
The effects of farm size on adoption are dispersed and a good number of the reviewed study results are not statistically significant and fall outside the 95% confidence band (Figure 3, right panel). However, and on average, farm size is positively correlated with the adoption of various CA practices in parts of ESA (Figure 3, left panel). This result could reflect the importance of wealth and household resources in facilitating adoption of new practices (if farm size is correlated with household wealth). It could also reflect the fact that larger farm holdings give farmers leverage to experiment with CA on some parts of their land, while maintaining the low-risk, low-return conventional methods on the rest. Similar results are reported by Ward et al. (2018) for Malawi. These findings highlight two main points in the context of the adoption framework in Figure 1. First, in countries with relatively low population densities like Zambia and Tanzania (and Zimbabwe), farm size (and presumably low land prices) plays an important in CA adoption. Whether farm size increases or reduces the probability of adoption will differ by characteristics such as wealth status, market access, age and education of the farmer, etc. In such places, either land using CA practices are more favorable but capital-intensive CA practices may be adopted depending on the cost of capital. This is aligned to hypotheses in the SE quadrant of the adoption framework in Figure 1. Second, farm size may be an impediment to adoption in countries with rising land scarcity such as Malawi, Kenya and Ethiopia. In these countries, both land and labor prices are high, which implies that capital intensive mechanized CA are an option depending on the cost of capital and average operable land sizes (NW quadrant, Figure 1). In cases where average land sizes are too small, e.g., in Malawi, an option would be for adjacent fields/plots to be aggregated in order to make the required minimum operable areas by mechanized operations.
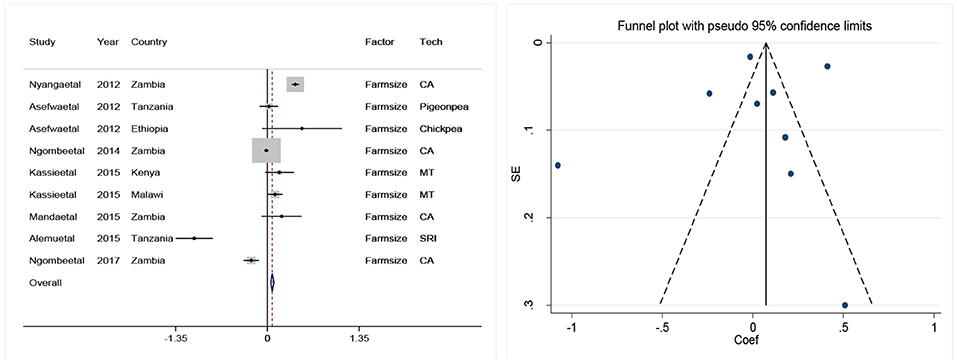
Figure 3. The effects of farm size on the adoption of various CSA practices in parts of eastern and southern Africa. Source: Computed from Asfaw et al. (2012), Nyanga et al. (2012), Ngombe et al. (2014), Alem et al. (2015), Kassie et al. (2015), and Ng'ombe et al. (2017). The horizontal lines in the forest plots are the confidence intervals (CI) and the gray areas are study weights generated in the meta function. The dotted vertical line in the left panel shows the overall/combined effects. CIs that cross the zero line indicate statistical insignificance, while those on the left (right) show statistically significant negative (positive) effects. SRI denotes sustainable rice intensification and CA denotes the full set of minimum tillage, mulching and rotation.
Effects of Labor Availability on CA Adoption
Labor availability is another potential enabler of CA adoption. For the studies reviewed, labor availability measured by number of persons working per ha, number of adults, adult equivalents, man-days per acre, household size (Nyanga et al., 2012; Ngombe et al., 2014; Pedzisa et al., 2015; Jaleta et al., 2016; Ngoma et al., 2016) has an insignificant to a positive and significant effect on adoption (Figure 4, left panel). The effect size in most studies is small with wide confidence intervals (Figure 4, right panel). Labor was positively correlated with the adoption of CA practices in Zambia (Ng'ombe et al., 2017) but insignificant to the adoption of MT in Ethiopia (Jaleta et al., 2016) and Zambia (Nyanga, 2012; Ngoma et al., 2016), and CA in Zimbabwe (Pedzisa et al., 2015). In other contexts, labor saving benefits are among major drivers of CA adoption, e.g., Lalani et al. (2016) for Mozambique. These findings can fit in either the SE or SW quadrants of Figure 1. In land abundant and low population density countries like Zambia, limited labor availability (a proxy for high labor price) appears to limit the adoption of MT. However, labor availability is associated with increased chance of adopting any CA practice. Thus, how CA is defined and measured matters. Results from countries with high population densities like Ethiopia and Tanzania give a similar picture. On average, these findings also seem aligned to the predictions in the SE and SW quadrants in Figure 1.
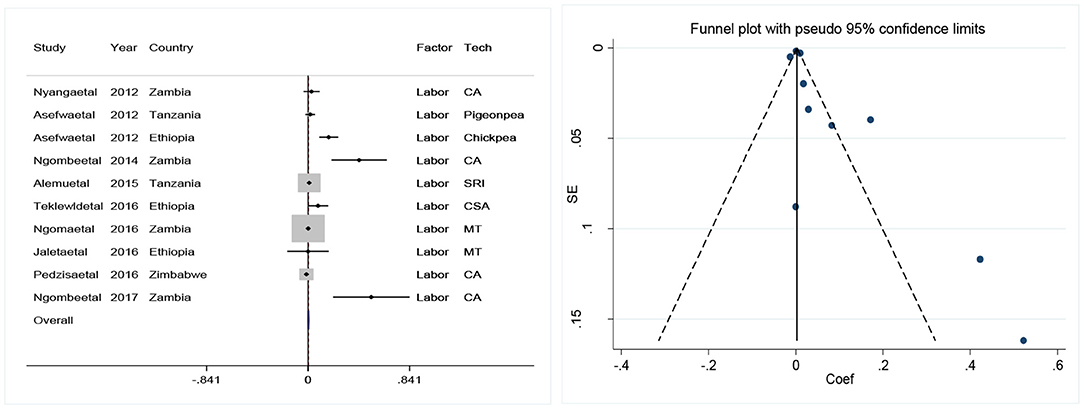
Figure 4. The effects of labor availability on the adoption of various CSA practices in parts of Eastern and Southern Africa. Source: Computed from Asfaw et al. (2012), Nyanga (2012), Teklewold et al. (2013), Ngombe et al. (2014), Alem et al. (2015), Pedzisa et al. (2015), Ngoma et al. (2016), and Ng'ombe et al. (2017). The horizontal lines in the forest plots are the confidence intervals (CI) and the gray areas are study weights generated in the meta function. CIs that cross the zero line indicate statistical insignificance, while those on the left (right) show statistically significant negative (positive) effects.
Other Factors Influencing CA Adoption
Several other factors might influence CA adoption. Farmers may be reluctant to adopt CA due to lack of technical know-how or due to culture and traditions, or both, limited linkages to output and input markets, drudgery of some technologies and high discounting (Ngoma et al., 2016; Zulu-Mbata et al., 2016). Ndah et al. (2018) suggests a need to address weed infestation under CA, improve market access conditions and to find ways to get youthful farmers engaged in CA.
Insights from behavioral and experimental economics could be relevant to advance our understanding of the apparent CA conundrum where even after several years of promotion and given that CA presumably promotes the achievement of many smallholder farmer objectives – namely low productivity, soil health, and mitigation of adverse weather events – its uptake does not spread like wildfire.
Farmers' risk and time preferences, and risk perceptions also matter for CA adoption. Ngoma et al. (2018b) found that risk aversion, impatience, and farmers' subjective perceptions of the riskiness of CA significantly reduced the probability of adoption. Although CA is considered risk reducing, risk averse farmers may not adopt it because they may be unwilling to take on unfamiliar farming practices or because they do not understand the risk reducing capabilities of CA. Impatient farmers or farmers with high discount rates may not adopt CA if they believe that significant benefits only accrue in the medium to long-term, and yet their primary interests to feed families, are short term. Using economic field experiments, Ward et al. (2018) and Bell et al. (2018) found that providing subsidies and payments for ecosystem services improved the adoption of CA and other sustainable land management practices in Malawi. There is scope to apply behavioral and experimental economics to study pecuniary and non-pecuniary incentives for CA adoption among smallholder farmers. In sum, drivers of CA vary by context as suggested in Knowler and Bradshaw (2007).
Impacts of CA ON Productivity and Livelihoods
In general, the evidence on the impacts of CA on land productivity (yield) and livelihoods (income, food security) based on large observational data and beyond field/station experimental plots is still thin in SSA. This is partly because doing a proper and credible impact assessment is challenging; it requires accounting for what adopters would have earned had they not adopted and what non-adopters would have earned had they adopted, while controlling for confounding observables and unobservables. Creating the counterfactual is an empirical challenge.
There is, however, some studies trying to fill this gap, with some finding positive impacts of CA adoption on maize/crop yield in Zambia (Kuntashula et al., 2014; Ngoma et al., 2015; Abdulai, 2016; Ng'ombe et al., 2017; Ngoma, 2018), Tanzania (Arslan et al., 2017) and Ethiopia (Jaleta et al., 2016). There are also several studies based on experimental station or field data that show positive impacts of adopting CA on crop yield in Malawi (Ngwira et al., 2012), Zambia (Thierfelder et al., 2013), Zimbabwe (Nyamangara et al., 2014) and across SSA (Rusinamhodzi et al., 2011; Thierfelder et al., 2015a,b; Thierfelder et al., 2016). In a meta-analysis involving 933 observations from 16 sub-Saharan African countries, Corbeels et al. (2020) find that yield gains from CA relative to conventional practices are small and conclude that “although CA may bring soil conservation benefits, it is not a technology for African smallholder farmers to overcome low crop productivity and food insecurity in the short term, page 451.”
A closer look at the results, such as the impacts of CA on crop yield reveals several nuances. While some studies find that CA confers immediate yield gains (Ngwira et al., 2013), others find lags of 2–5 cropping seasons or longer before any significant yield gains are observed (Giller et al., 2009; Thierfelder et al., 2017). And yet, other studies find that CA has no statistically significant yield effects (Arslan et al., 2015). There appears to be a tacit agreement that CA practices are viable climate change adaptation strategies for farmers in SSA (IPCC, 2014), with clear links between rainfall variability and adoption (Arslan et al., 2014, 2017; Ngoma et al., 2016).
There is less agreement on the impacts of CA on household incomes. Some argue that the positive yield benefits from CA may be insufficient to offset the costs of implementation, at least in the short term (Jaleta et al., 2016; Ngoma, 2018). Among the more favorable assessments, Tambo and Mockshell (2018) report significant income gains from full adoption of CA across nine SSA countries. Lalani et al. (2017) compares net present values for CA vs. conventional agriculture from 197 farmers and find CA to be beneficial to both poor and rich farmers in Mozambique. Combining CA practices with improved inputs seems to offer greater benefits, as was found in Manda et al. (2016). There is also evidence from experimental studies suggesting that CA adopters have had higher incomes and profits than non-adopters in some instances (Ngwira et al., 2013). The foregoing discussions seem to suggest that while CA adoption remains lower than expected, CA has modest positive yield effects in the medium to long term with climate adaptation co-benefits.
Discussion: How To Increase and Sustain CA Adoption
Our review suggests that the uptake of CA is limited by various factors including drudgery of some technologies like planting basins, financial, labor and information constraints, limited market access, cultural norms and risk perceptions (section Drivers of CA Adoption). The studies reviewed do not tell one consistent story, and they contain a high number of insignificant results. Furthermore, while the framework (section A Framework of Expected CA Adoption Under Various Economic Contexts) offered specific hypotheses on the type of CA technologies likely to be adopted, most studies reviewed considered CA in general.
Yet, we find some evidence in line with the induced innovation theory. Labor availability is key for CA adoption, while credit access appears to play a minor role. These findings suggest that the studies reviewed here were done in contexts that fit into the SE or SW quadrants of the adoption framework in section A Framework of Expected CA Adoption Under Various Economic Contexts, where either land and labor prices are low, and capital is expensive (SE), or land and capital prices are relatively high but labor is abundant and cheap (SW). In either context, labor using variants of CA such as planting basins are more likely to be adopted than are capital using mechanized options and it is expected that access to credit might not be the most limiting factor. This would be expected in parts of Ethiopia, Tanzania and Zambia.
The results for farm size, which might be a proxy for land scarcity, is as expected, as large farm size (higher land abundance and low land prices) is associated with more CA adoption. This is expected in countries with relatively low population densities like Zambia and Tanzania (and Zimbabwe) (section Drivers of CA Adoption). One explanation may be in the nature of household or farm data, where the results might reflect intra-village inequality in farm size, rather than inter-village differences (and land scarcity is a village or higher scale measure). Further, large farm size might be correlated to other household attributes that make adoption more likely and might it make feasible for households to experiment with new technologies like CA without putting at risk the goal of meeting subsistence needs. Farm size may impede CA adoption in countries with rising land scarcity and wages such as Malawi, Kenya and Ethiopia. In these countries, capital intensive mechanized CA are an option depending on the cost of capital and average operable land sizes. In cases where average land sizes are too small, e.g., in Malawi, an option would be for adjacent fields/plots to be aggregated in order to make the required minimum operable areas by mechanized operations. The use of small two-wheel tractors is another option (Baudron et al., 2015). Finally, to the extent that CA adoption is considered risky, farmers with little land might be reluctant to take that risk.
Given that CA is part of national policy in several ESA countries and given its potential benefits on average and in the medium to long term, we contend that CA will remain a key policy instrument for raising agricultural productivity and addressing climate change in smallholder farming systems in the region. Therefore, and while we recognize that there is an adoption problem, we propose that the most relevant questions in development discourse going forward will not be on the extent of CA adoption, but rather what can be done to improve CA adoption and effectiveness.
This review highlights at least four potential pathways to make CA more climate smart and sustainably improve its uptake. First, CA can contribute to the productivity objective at scale by facilitating intensification. This is more likely if CA is complemented with more use of improved inputs such as inorganic fertilizers and hybrid seed, mechanization, irrigation and organic manure.5 Inorganic fertilizer is important to help restore soil health and irrigation facilitates all-year-round production. Minimum tillage concentrated only to planting stations under CA would help minimize leaching and make the inorganic fertilizer available for plant growth. Inorganic fertilizer under CA systems would help boast yields, biomass and organic matter in form of crop residues (Vanlauwe et al., 2014). In fact, Vanlauwe et al. (2014) advocates for the appropriate use of inorganic fertilizers to be the fourth principle of CA.6 Organic matter improves crop response to inorganic fertilizer (Jayne et al., 2019), while mechanization would allow implementing CA on a larger scale and, this in turn, improves returns to labor. Over time, the use of inorganic fertilizer is expected to help build organic matter in the planting stations under CA farming systems. For the current drive to mechanize CA operations to work, there is need to address the high cost of capital. Baudron et al. (2015) suggest that the use of inexpensive and efficient small two-wheel tractors might be appropriate for CA.
Second, CA can also better contribute to the productivity and adaptation objectives if combined with market and value chain developments to improve market access. For example, necessary support policies are needed to make receipt of agricultural subsidies conditional on verified CA adoption, especially in countries with national subsidy programs. For example, Ngoma et al. (2018b) using framed economic field experiments found that providing a green subsidy framed as an add-on incentive to the current farmer input subsidies for verified CA adopters raised the probability of adopting CA by 12% points among smallholder farmers in the study areas in Zambia. Ward et al. (2018) and Bell et al. (2018) found that providing subsidies and payments for ecosystem services improved the adoption of CA and other sustainable land management practices in Malawi. The use of premium prices for produce with certified low carbon footprint is another market option.
Third, the foregoing options can be complemented with improved public spending on research and development to build evidence on the adaptation and mitigation potential of specific CA practices and extension to encourage CA adoption and adaptation of CA to local contexts. Conditional input subsidies to limit cropland expansion and linking carbon credits from CA adoption to payments for environmental services (PES) are alternatives that can be explored and used to incentivize mitigation under CA. A World Bank funded Community Markets for Conservation (COMACO) Landscape Management Project that promotes sustainable agriculture and forest conservation in Zambia is a good example of such initiatives and Bell et al. (2018) reports on the positive effects of PES on land management in Malawi.7
And, lastly, CA can also better contribute to the adaptation objectives if the full CA suite including crop diversification, rotation and residue retention is applied. Adoption of the full CA suite improves infiltration and soil moisture conservation (Thierfelder et al., 2017).
Conclusion
A rapidly growing population in sub-Saharan Africa (SSA), expected to reach 2 billion by 2050, and the projected negative consequences of climate change on agriculture and livelihoods require rapid and radical transformation of rainfed farming systems in the region. To respond effectively to the dual challenge, the prevalent smallholder farming systems require a paradigm shift to become more climate smart. Conservation agriculture (CA) is largely seen as part of this transition toward climate smart agriculture. We reviewed the evidence on the extent to which CA is climate smart and contributes to the sustainable agricultural intensification (SAI) objectives of raising productivity and income and climate adaptation/resilience in Southern and Eastern Africa. We end the paper by offering pragmatic options to enhance CA's contribution to SAI objectives.
The strong policy support CA has enjoyed suggests that it will remain an important option for transforming smallholder farming systems in the region. The sizeable medium and long-term productivity and resilience benefits suggest that CA can contribute to sustainable agricultural intensification. But there is an adoption problem requiring urgent attention. From our review, we postulate that capital-intensive or mechanized CA is more likely to be adopted under economic dynamism where capital is cheap relative to labor, while labor-intensive CA practices are more likely to be adopted under economic stagnation where capital is expensive, and labor is cheap and abundant. A subnational focus in research is needed to identify economic conditions of different regions and agro-ecological zones and to test hypotheses derived from the framework in this paper and to propose the most appropriate packages for CA promotion. According to the adoption framework developed in this paper, our findings suggest that the studies reviewed were done in countries or parts of countries where either land and labor prices are low, and capital is expensive, or land and capital prices are relatively high but labor is abundant and cheap. In either context, labor using variants of CA such as planting basins are more likely to be adopted than are capital using mechanized options. There are appreciable differences across countries in ESA. In general, we expect capital to be expensive in parts of Malawi, Ethiopia, Kenya, Tanzania, Zambia and Zimbabwe where economic dynamism is low. Although labor using CA practices are more likely to adopted in the region, rising land scarcity (prices) and wages may present an opportunity for capital intensive, mechanized CA operations if the cost of capital can be kept low and if there is a supportive environment for mechanization. The current drive to use small two-wheel tractors to mechanize CA is a promising option (Baudron et al., 2015).
We therefore conclude that CA is climate smart and if adopted widely, it has the potential to help build resilience in smallholder farming systems. The findings in this paper have some implications for targeting CA interventions in the region and on how CA policies and implementation can make smallholder farming systems climate smart. First, CA needs to be reframed, better targeted and adapted to local contexts (Brown et al., 2018; section Discussion: How to Increase and Sustain CA Adoption) and linked to support programs such as conditional input subsidies, mechanization and other market and climate smart subsidies in order to address resource constraints and enhance its short-term uptake and welfare benefits. Our framework, which predicts that labor using CA practices are more likely to be adopted in ESA (and yet these practices are associated with drudgery) call for concerted efforts to find options to mechanize CA operations.
Second, CA can be more climate smart by integrating CA with other climate smart agriculture (CSA) practices such as agroforestry (Duguma et al., 2017), and improved inputs and organic manure; market and value chain development to improve market access; linking CA carbon credits to payments for environmental services-type schemes; improved enabling policy environment and public spending on research and development.
There is an urgent need to confront the adoption problem through sustainable market-based incentives in order to make the options identified in this paper feasible for the individual farmers. If the adoption predictions in our framework hold, there is a need to transition CA from the labor-using, drudgery-laden variants that are more likely to be adopted in ESA to mechanized options that improve land and labor productivity. The favorable institutional support, rising demand for mechanization and availability of low cost two-wheel tractors in the region (Baudron et al., 2015) would facilitate this transition. How to scale-up and scale-out CA effectively and efficiently – and have this backed by sufficient and coherently applied resources from governments, NGOs and donors – remains an open question.
Author Contributions
HN, AA, and TJ conceptualized the paper. HN conducted the analysis and wrote the first draft. TJ, AA, and AC provided inputs into the analysis and contributed to the editing of the final paper. All authors contributed to the article and approved the submitted version.
Funding
This work was funded by the Swedish International Development Agency (SIDA) and the United States Agency for International Development (USAID) through the Indaba Agriculture Policy Research Institute (IAPRI) in Lusaka. We acknowledge the technical and capacity building support provided by Michigan State University through the financial support from the USAID funded Zambia buy-in to the Feed the Future Innovation Lab for Food Security Policy (FSP) [grant number AID-OAA-L-13-00001]. This work also benefited from financial support from the CGIAR Research Program on Policies, Institutions, and Markets (PIM), led by the International Food Policy Research Institute, Project No. 203002.002.217.
Conflict of Interest
The authors declare that the research was conducted in the absence of any commercial or financial relationships that could be construed as a potential conflict of interest.
Acknowledgments
Mooya Nzila (MHSRIP) provided excellent research assistance at an early stage of this paper. Parts of section Introduction and What Constitutes Conservation Agriculture (CA) Adoption? drew heavily on the HN's PhD thesis, Conservation agriculture, livelihoods and deforestation in Zambia PhD Thesis, School of Economics and Business, Norwegian University of Life Sciences.
Footnotes
1. ^Food systems refer to the whole range of processes and infrastructure involved in satisfying people's food security requirements (Porter et al., 2014).
2. ^An extended and influential online discussion following Giller et al. (2009) is available here https://conservationag.wordpress.com/2009/12/01/ken-gillers-paper-on-conservation-agriculture/.
3. ^Powlson et al. (2016). posit that “even if increases in soil organic carbon stock are small, and of limited value for climate change mitigation [under CA], there will almost always be an improvement in soil quality which would be expected to contribute to increased resilience to climate change”.
4. ^Some studies were done in multiple countries at the same time, we count separately for each country and by this metric, we used studies from Zambia, Zimbabwe, Tanzania, Ethiopia, Kenya and Malawi.
5. ^The use of inorganic fertilizer may be at odds with mitigation objectives, but not so much at low levels of use as is currently common in SSA.
6. ^Of course, fertilizer should be used in moderation otherwise, there is a risk of raising the fertilizer carbon footprint, see for example Chojnacka et al. (2019) for the European Union case.
7. ^Documenting lessons from such initiatives will be important going forward. Project details available at: http://www.biocarbonfund.org/node/8.
References
Abdulai, A.-N., and Abdulai, A. (2016). Examining the impact of conservation agriculture on environmental efficiency among maize farmers in Zambia. Environ. Dev. Econ. 22, 177–201. doi: 10.1017/S1355770X16000309
Abdulai, A. N. (2016). Impact of conservation agriculture technology on household welfare in Zambia. Agric. Econ. 47, 729–741. doi: 10.1111/agec.12269
Alem, Y., Eggert, H., and Ruhinduka, R. (2015). Improving welfare through climate-friendly agriculture: the case of the system of rice intensification. Environ. Resour. Econ. 62, 243–263. doi: 10.1007/s10640-015-9962-5
Andersson, J. A., and D'Souza, S. (2014). From adoption claims to understanding farmers and contexts: a literature review of conservation agriculture (CA) adoption among smallholder farmers in southern Africa. Agric. Ecosyst. Environ. 187, 116–132. doi: 10.1016/j.agee.2013.08.008
Andersson, J. A., and Giller, K. A. (2012). “On heretics and God's blanket salemen: Contested claims for conservation agriculture and the politics of its promotion in African smallholder farming,” in Contested Agronomy: Agricultural Research in a Changing World, eds J. Sumberg and J. Thomson (London: Earthscan), 22–46.
Arslan, A., Belotti, F., and Lipper, L. (2017). Smallholder productivity and weather shocks: adoption and impact of widely promoted agricultural practices in Tanzania. Food Policy 69, 68–81. doi: 10.1016/j.foodpol.2017.03.005
Arslan, A., McCarthy, N., Lipper, L., Asfaw, S., and Cattaneo, A. (2014). Adoption and intensity of adoption of conservation farming practices in Zambia. Agric. Ecosyst. Environ. 187, 72–86. doi: 10.1016/j.agee.2013.08.017
Arslan, A., McCarthy, N., Lipper, L., Asfaw, S., Cattaneo, A., and Kokwe, M. (2015). Climate smart agriculture? Assessing the adaptation implications in Zambia. J. Agric. Econ. 66, 753–780. doi: 10.1111/1477-9552.12107
Asfaw, S., Shiferaw, B., Simtowe, F., and Lipper, L. (2012). Impact of modern agricultural technologies on smallholder welfare: evidence from Tanzania and Ethiopia. Food Policy 37, 283–295. doi: 10.1016/j.foodpol.2012.02.013
Barrett, C. B., and Constas, M. A. (2014). Toward a theory of resilience for international development applications. Proc. Natl. Acad. Sci. U.S.A. 111, 14625–14630. doi: 10.1073/pnas.1320880111
Baudron, F., Corbeels, M., Monicat, F., and Giller, K. (2009). Cotton expansion and biodiversity loss in African savannahs, opportunities and challenges for conservation agriculture: a review paper based on two case studies. Biodivers. Conserv. 18, 2625–2644. doi: 10.1007/978-94-007-0174-8_6
Baudron, F., Sims, B., Justice, S., Kahan, G. D., Rose, R., Mkomwa, S., et al. (2015). Re-examining appropriate mechanization in Eastern and Southern Africa: two-wheel tractors, conservation agriculture, and private sector involvement. Food Secur. 7, 889–904. doi: 10.1007/s12571-015-0476-3
Bell, A. R., Benton, T. G., Droppelmann, K., Mapemba, L., Pierson, O., and Ward, P. S. (2018). Transformative change through payments for ecosystem services (PES): a conceptual framework and application to conservation agriculture in Malawi. Glob. Sustain. 1:e4. doi: 10.1017/sus.2018.4
Boserup, E. (1965). The Condition of Agricultural Growth. The Economics of Agrarian Change Under Population Pressure. London: George Allen and Unwin.
Brown, B., Llewellyn, R., and Nuberg, I. (2018). Global learnings to inform the local adaptation of conservation agriculture in Eastern and Southern Africa. Glob. Food Secur.17, 213–220. doi: 10.1016/j.gfs.2017.10.002
Canning, D., Sangeeta, R., and Abdo, Y. S. (2015). Africa's Demographic Transition: Dividend or Disaster? Washington, DC: World Bank; and Agence Française de Développement. © World Bank.
Chojnacka, K., Kowalski, Z., Kulczycka, J., Dmytryk, A., Górecki, H., Ligas, B., et al. (2019). Carbon footprint of fertilizer technologies. J. Environ. Manag. 231, 962–967. doi: 10.1016/j.jenvman.2018.09.108
Corbeels, M., Naudin, K., Whitbread, A. M., Kühne, R., and Letourmy, P. (2020). Limits of conservation agriculture to overcome low crop yields in sub-Saharan Africa. Nat. Food 1, 447–454. doi: 10.1038/s43016-020-0114-x
Droppelmann, K. J., Snapp, S. S., and Waddington, S. R. (2017). Sustainable intensification options for smallholder maize-based farming systems in Sub-Saharan Africa. Food Secur. 9, 133–150. doi: 10.1007/s12571-016-0636-0
Duguma, L. A., Nzyoka, J., Minang, P. A., and Bernard, F. (2017). How Agroforestry Propels Achievement of Nationally Determined Contributions. ICRAF Policy Brief no. 34. Nairobi: World Agroforestry Center. Available online at: http://www.worldagroforestry.org/downloads/Publications/PDFS/PB17360.pdf (accessed April 28, 2021).
Giller, K. E., Andersson, J. A., Corbeels, M., Kirkegaard, J., Mortensen, D., Erenstein, O., et al. (2015). Beyond conservation agriculture. Front. Plant Sci. 6:870. doi: 10.3389/fpls.2015.00870
Giller, K. E., Witter, E., Corbeels, M., and Tittonell, P. (2009). Conservation agriculture and smallholder farming in Africa: the heretics' view. Field Crops Res. 114, 23–34. doi: 10.1016/j.fcr.2009.06.017
Glover, D., Sumberg, J., and Andersson, J. A. (2016). The adoption problem; or why we still understand so little about technological change in African agriculture. Outlook Agric. 45, 3–6. doi: 10.5367/oa.2016.0235
Govaerts, B., Verhulst, N., Castellanos-Navarrete, A., Sayre, K. D., Dixon, J., and Dendooven, L. (2009). Conservation agriculture and soil carbon sequestration: between myth and farmer reality. Crit. Rev. Plant Sci. 28, 97–122. doi: 10.1080/07352680902776358
Haggblade, S., and Tembo, G. (2003). “Development, diffusion and impact of conservation farming in Zambia,” in Food Security Research Project Working Paper # 8 (Lusaka: Food Security Research Project).
Hallegatte, S., Bangalore, M., Bonzanigo, L., Fay, M., Kane, T., Narloch, U., et al. (2016). Shock Waves: Managing the Impacts of Climate Change on Poverty. Available online at: https://openknowledge.worldbank.org/handle/10986/22787 (accessed April 28, 2021).
Hamududu, B. H., and Ngoma, H. (2019). Impacts of climate change on water resources availability in Zambia: implications for irrigation development. Environ. Dev. Sustain. 22, 2817–2838. doi: 10.1007/s10668-019-00320-9
Hayami, Y., and Ruttan, V. W. (1971). Induced Innovation in Agricultural Development. Minneapolis: Center for Economics Research, Department of Economics, University of Minnesota.
Hoddinott, J. F. (2014). Resilience: A Primer. Available online at http://ebrary.ifpri.org/cdm/ref/collection/p15738coll2/id/128159 (accessed April 28, 2021).
IPCC (2014). “Impacts adaptation and vulnerability. Part B: regional aspects contribution of working group II to the fifth assessment report of the intergovernmental panel on climate, change,” in Climate Change 2014, eds V. R. Barros, C. B. Field, D. J. Dokken, M. D. Mastrandrea, K. J. Mach, and T. E. Bilir (New York, NY: IPCC), 1–32.
Jaleta, M., Kassie, M., Tesfaye, K., Teklewold, T., Jena, P. R., Marenya, P., et al. (2016). Resource saving and productivity enhancing impacts of crop management innovation packages in Ethiopia. Agric. Econ. 47, 513–522. doi: 10.1111/agec.12251
Jayne, T. S., Snapp, S., Place, F., and Sitko, N. (2019). Sustainable agricultural intensification in an era of rural transformation in Africa. Glob. Food Secur. 20, 105–113. doi: 10.1016/j.gfs.2019.01.008
Kassie, M., Teklewold, H., Jaleta, M., Marenya, P., and Erenstein, O. (2015). Understanding the adoption of a portfolio of sustainable intensification practices in eastern and Southern Africa. Land Use Policy 42, 400–411. doi: 10.1016/j.landusepol.2014.08.016
Knowler, D., and Bradshaw, B. (2007). Farmers' adoption of conservation agriculture: a review and synthesis of recent research. Food Policy 32, 25–48. doi: 10.1016/j.foodpol.2006.01.003
Kuntashula, E., Chabala, L. M., and Mulenga, B. P. (2014). Impact of minimum tillage and crop rotation as climate change adaptation strategies on farmer welfare in smallholder farming systems of Zambia. J. Sustain. Dev. 7, 95–110. doi: 10.5539/jsd.v7n4p95
Lalani, B., Dorward, P., and Holloway, G. (2017). Farm-level economic analysis - is conservation agriculture helping the poor? Ecol. Econ. 141, 144–153. doi: 10.1016/j.ecolecon.2017.05.033
Lalani, B., Dorward, P., Holloway, G., and Wauters, E. (2016). Smallholder farmers' motivations for using conservation agriculture and the roles of yield, labour and soil fertility in decision making. Agric. Syst. 146, 80–90. doi: 10.1016/j.agsy.2016.04.002
Lobell, D. B., Burke, M. B., Tebaldi, C., Mastrandrea, M. D., Falcon, W. P., and Naylor, R. L. (2008). Prioritizing climate change adaptation needs for food security in 2030. Science 319, 607–610. doi: 10.1126/science.1152339
Manda, J., Alene, A. D., Gardebroek, C., Kassie, M., and Tembo, G. (2016). Adoption and impacts of sustainable agricultural practices on maize yields and incomes: evidence from rural Zambia. J. Agric. Econ. 67, 130–153. doi: 10.1111/1477-9552.12127
Mockshell, J., and Kamanda, J. (2018). Beyond the agroecological and sustainable agricultural intensification debate: is blended sustainability the way forward?. Int. J. Agric. Sustain. 16, 127–149. doi: 10.1080/14735903.2018.1448047
Ndah, H. T., Schuler, J., Diehl, D., Bateki, C., Sieber, S., and Knierim, A. (2018). From dogmatic views on conservation agriculture adoption in Zambia towards adapting to context. Int. J. Agric. Sustain. 16, 228–242. doi: 10.1080/14735903.2018.1447227
Ngoma, H. (2016). Conservation Agriculture, Livelihoods and Deforestation in Zambia. Aas: School of Economics and Business, Norwegian University of Life Sciences.
Ngoma, H. (2018). Does minimum tillage improve the livelihood outcomes of smallholder farmers in Zambia?. Food Secur. 10, 381–396. doi: 10.1007/s12571-018-0777-4
Ngoma, H., Angelsen, A., Carter, S., and Roman-Cuesta, R. M. (2018a). “Climate-smart agriculture: will higher yields lead to lower deforestation?,” in Transforming REDD+: Lessons and New Directions, eds A. Angelsen, C. Martius, V. De Sy, A. E. Duchelle, A. M. Larson, and T. T. Pham (Bogor: Center for International Forestry Research [CIFOR]), 174–192.
Ngoma, H., Mason, N. M., Samboko, P., and Hangoma, P. (2018b). “Switching up climate-smart agriculture adoption: do “green” subsidies, insurance, risk aversion and impatience matter?,” in IAPRI Working Paper 146 (Lusaka: Indaba Agricultural Policy Research Institute [IAPRI]). Available online at: http://www.iapri.org.zm/images/WorkingPapers/wp146_for_pdf_final_v2_clean.pdf (accessed January 10, 2021).
Ngoma, H., Mason, N. M., and Sitko, N. J. (2015). Does minimum tillage with planting basins or ripping raise maize yields? Meso-panel data evidence from Zambia. Agric. Ecosyst. Environ. 212, 21–29. doi: 10.1016/j.agee.2015.06.021
Ngoma, H., Mulenga, B. P., and Jayne, T. S. (2016). Minimum tillage uptake and uptake intensity by smallholder farmers in Zambia. Afr. J. Agric. Resour. Econ. 11, 249–262. doi: 10.22004/ag.econ.252456
Ngombe, J., Kalinda, T., Tembo, G., and Kuntashula, E. (2014). Econometric analysis of the factors that affect adoption of conservation farming practices by smallholder farmers in Zambia. J. Sustain. Dev. 7, 124–138. doi: 10.5539/jsd.v7n4p124
Ng'ombe, J. N., Kalinda, T. H., and Tembo, G. (2017). Does adoption of conservation farming practices result in increased crop revenue? Evid. Zambia Agrekon 56, 205–221. doi: 10.1080/03031853.2017.1312467
Ngwira, A. R., Aune, J. B., and Mkwinda, S. (2012). On-farm evaluation of yield and economic benefit of short term maize legume intercropping systems under conservation agriculture in Malawi. Field Crops Res. 132, 149–157. doi: 10.1016/j.fcr.2011.12.014
Ngwira, A. R., Thierfelder, C., and Lambert, D. M. (2013). Conservation agriculture systems for Malawian smallholder farmers: long-term effects on crop productivity, profitability and soil quality. Renewable Agric. Food Syst. 28, 350–363. doi: 10.1017/S1742170512000257
Niang, I., Ruppel, O. C., Abdrabo, M. A., Essel, A., Lennard, C., Padgham, J., et al. (2014). “Impacts, adaptation, and vulnerability. Part B: regional aspects, Africa. Contribution of working group II to the fifth assessment report of the intergovernmental panel on climate change,” in Climate Change 2014, eds V. R. Barros, C. B. Field, D. J. Dokken, M. D. Mastrandrea, K. J. Mach, T. E. Bilir, et al. (Cambridge; New York, NY: Cambridge University Press), 1199–1265.
Nyamangara, J., Nyengerai, K., Masvaya, E. N., Tirivavi, R., Mashingaidze, N., Mupangwa, W., et al. (2014). Effect of conservation agriculture on maize yield in the semi-arid areas of Zimbabwe. Exp. Agric. 50, 159–177. doi: 10.1017/S0014479713000562
Nyanga, P. H. (2012). Factors influencing adoption and area under conservation agriculture: a mixed methods approach. Sustain. Agric. Res. 1:27. doi: 10.5539/sar.v1n2p27
Nyanga, P. H., Johnsen, F. H., and Kalinda, T. H. (2012). Gendered Impacts of Conservation Agriculture and the Paradox of herbicide use in Zambia. Int. J. Technol. Dev. Stud. 3, 1–24.
Olsson, L., Opondo, M., Tschakert, P., Agrawal, A., Eriksen, S. H., Ma, S., et al. (2014). “Livelihoods and poverty. Part A: contribution of working group II to the fifth assessment report of the intergovernmental panel on climate change,” in Climate Change 2014, eds B. C. B. Field, V. R. Barros, D. J. Dokken, M. D. Mastrandrea, K. J. Mach, T. E. Bilir, et al. (Cambridge; New York, NY: Cambridge University Press), 793–832.
Pedzisa, T., Rugube, L., Winter-Nelson, A., Baylis, K., and Mazvimavi, K. (2015). The Intensity of adoption of Conservation agriculture by smallholder farmers in Zimbabwe. Agrekon 54, 1–22. doi: 10.1080/03031853.2015.1084939
Porter, J., Xie, L., Challinor, A. J., Cochrane, K., Howden, M., Iqbal, M. M., et al. (2014). “Food security and food production systems. Part A: contribution of working group II to the fifth assessment report of the intergovernmental panel on climate change,” in Climate Change 2014, eds B. C. B. Field, V. R. Barros, D. J. Dokken, M. D. Mastrandrea, K. J. Mach, T. E. Bilir, et al. (Cambridge; New York, NY: Cambridge University Press), 485–533.
Powlson, D. S., Stirling, C. M., Jat, M. L., Gerard, B. G., Palm, C. A., Sanchez, P. A., et al. (2014). Limited potential of no-till agriculture for climate change mitigation. Nat. Clim. Change 4, 678–683. doi: 10.1038/nclimate2292
Powlson, D. S., Stirling, C. M., Thierfelder, C., White, R. P., and Jat, M. L. (2016). Does conservation agriculture deliver climate change mitigation through soil carbon sequestration in tropical agro-ecosystems?. Agric. Ecosyst. Environ. 220, 164–174. doi: 10.1016/j.agee.2016.01.005
Rusinamhodzi, L., Corbeels, M., van Wijk, M. T., Rufino, M. C., Nyamangara, J., and Giller, K. E. (2011). A meta-analysis of long-term effects of conservation agriculture on maize grain yield under rain-fed conditions. Agron. Sustain. Dev. 31, 657–673. doi: 10.1007/s13593-011-0040-2
Tambo, J. A., and Mockshell, J. (2018). Differential Impacts of conservation agriculture technology options on household income in Sub-Saharan Africa. Ecol. Econ. 151, 95–105. doi: 10.1016/j.ecolecon.2018.05.005
Teklewold, H., Kassie, M., and Shiferaw, B. (2013). Adoption of multiple sustainable agricultural practices in rural Ethiopia. J. Agric. Econ. 64, 597–623. doi: 10.1111/1477-9552.12011
Thierfelder, C., Chivenge, P., Mupangwa, W., Rosenstock, T. S., Lamanna, C., and Eyre, J. X. (2017). How climate-smart is conservation agriculture (CA)? – its potential to deliver on adaptation, mitigation and productivity on smallholder farms in Southern Africa. Food Secur. 9, 537–560. doi: 10.1007/s12571-017-0665-3
Thierfelder, C., Matemba-Mutasa, R., Bunderson, W. T., Mutenje, M., Nyagumbo, I., and Mupangwa, W. (2016). Evaluating manual conservation agriculture systems in southern Africa, Agriculture. Ecosyst. Environ. 222, 112–124. doi: 10.1016/j.agee.2016.02.009
Thierfelder, C., Matemba-Mutasa, R., and Rusinamhodzi, L. (2015a). Yield response of maize (Zea mays L.) to conservation agriculture cropping system in Southern Africa. Soil Tillage Res. 146, 230–242. doi: 10.1016/j.still.2014.10.015
Thierfelder, C., Mwila, M., and Rusinamhodzi, L. (2013). Conservation agriculture in eastern and southern provinces of Zambia: Long-term effects on soil quality and maize productivity. Soil Tillage Res. 126, 246–258. doi: 10.1016/j.still.2012.09.002
Thierfelder, C., Rusinamhodzi, L., Ngwira, A. R., Mupangwa, W., Nyagumbo, I., Kassie, G. T., et al. (2015b). Conservation agriculture in Southern Africa: advances in knowledge. Renewable Agric. Food Syst. 30, 328–348. doi: 10.1017/S1742170513000550
Thierfelder, C., and Wall, P. C. (2010). Investigating conservation agriculture (CA) systems in Zambia and Zimbabwe to mitigate future effects of climate change. J. Crop Improv. 24, 113–121. doi: 10.1080/15427520903558484
Thurlow, J., Zhu, T., and Diao, X. (2012). Current climate variability and future climate change: estimated growth and poverty impacts for Zambia. Rev. Dev. Econ. 16, 394–411. doi: 10.1111/j.1467-9361.2012.00670.x
UNEP (2013). The Emissions Gap Report 2013. Nairobi: United Nations Enviornmental Programme (UNEP). Available online at: https://www.unep.org/resources/emissions-gap-report-2013 (accessed April 28, 2021).
Vanlauwe, B., Wendt, J., Giller, K. E., Corbeels, M., Gerard, B., and Nolte, C. (2014). A fourth principle is required to define conservation agriculture in Sub-Saharan Africa: the appropriate use of fertilizer to enhance crop productivity. Field Crops Res. 155, 10–13. doi: 10.1016/j.fcr.2013.10.002
Ward, P. S., Bell, A. R., Droppelmann, K., and Benton, T. G. (2018). Early adoption of conservation agriculture practices: understanding partial compliance in programs with multiple adoption decisions. Land Use Policy 70, 27–37. doi: 10.1016/j.landusepol.2017.10.001
Zulu-Mbata, O., Chapoto, A., and Hichaambwa, M. (2016). “Determinants of conservation agriculture adoption among zambian smallholder farmers,” in IAPRI Working Paper 114 (Lusaka: Indaba Agricultural Policy Research Institute [IAPRI]). Available online at: http://www.iapri.org.zm/images/WorkingPapers/Determinants_WP114.pdf (accessed April 28, 2021).
Keywords: conservation agriculture (CA), adoption, productivity, Eastern and Southern Africa, impacts
Citation: Ngoma H, Angelsen A, Jayne TS and Chapoto A (2021) Understanding Adoption and Impacts of Conservation Agriculture in Eastern and Southern Africa: A Review. Front. Agron. 3:671690. doi: 10.3389/fagro.2021.671690
Received: 24 February 2021; Accepted: 17 May 2021;
Published: 22 June 2021.
Edited by:
Cameron Pittelkow, University of California, Davis, United StatesReviewed by:
Andrea Basche, University of Nebraska System, United StatesJohannes Schuler, Leibniz Center for Agricultural Landscape Research (ZALF), Germany
Copyright © 2021 Ngoma, Angelsen, Jayne and Chapoto. This is an open-access article distributed under the terms of the Creative Commons Attribution License (CC BY). The use, distribution or reproduction in other forums is permitted, provided the original author(s) and the copyright owner(s) are credited and that the original publication in this journal is cited, in accordance with accepted academic practice. No use, distribution or reproduction is permitted which does not comply with these terms.
*Correspondence: Hambulo Ngoma, aC5uZ29tYUBjZ2lhci5vcmc=
†Present address: Hambulo Ngoma, International Maize and Wheat Improvement Center (CIMMYT), Harare, Zimbabwe