- 1Department of Biotechnology, The University of Burdwan, Burdwan, India
- 2Department of Biophysics, Molecular Biology and Bioinformatics, University of Calcutta, Kolkata, India
- 3Center of Excellence in Systems Biology and Biomedical Engineering (TEQIP Phase-III), University of Calcutta, Kolkata, India
Plants cannot uptake the insoluble form of phosphate from soil. Phosphate-solubilizing microbes (PSMs) release gluconic acid (C6H12O7) that is synthesized by the interaction between co-factor pyrroloquinoline quinine (PQQ) and glucose dehydrogenase within themselves and hence convert the insoluble phosphate into a soluble form. Phylogenetic analyses based on individual sequences of PqqA–PqqE proteins involved in the PQQ biosynthetic pathway manifested clear clustering formation of the selected species according to their respective genera such as Pantoea, Rouxiella, Rahnella, Kosakonia, Mixta, Cronobacter, and Serratia. In multiple sequence alignment (MSA), numerous semi-conserved sites were identified that indicate acquired mutation during evolution. The selected pqq genes that appeared within an operon system sustain a specified order viz. pqqABCDE for both positive and negative strands. The nucleotide composition of the encoding genes displayed higher content of GCs at different positions of the codons and has also been properly reflected in relative synonymous codon usage (RSCU) values of the codons with few exceptions. The correspondence analysis (COA) based on RSCU proclaimed that the pqqB genes prefer A/U-ending codons over G/C, while for the pqqE gene, G/C-ending codons are comparatively more preferable (except CGU). Mutational pressure contributes to shaping the codon usage pattern for the selected pqq genes evinced from the COAs, while the ENc and neutrality plot gives attestation of natural selection. The higher values of CAI indicate the gene adaptability and codon usage bias. These comprehensive computational studies can be beneficial for further research in molecular phylogenetics, genomics, and proteomics and to better understand the evolutionary dynamics of PQQ.
Introduction
Microbes present in the rhizosphere, the nutrient-rich region of the soil surrounding the plant's roots, play important roles in the enhancement of plants' nutrient uptake, metabolic activities, crop productivity, and tolerance to multiple biotic and abiotic stresses. Plant growth-promoting rhizobacteria (PGPR) are a group of free-living soil bacteria found in the rhizosphere that directly or indirectly promote plant growth and development by employing various mechanisms. PGPR affects beneficial properties through direct mechanisms such as nitrogen fixation, phosphate solubilization, production of small, high-affinity iron-chelating compounds like siderophores to provide essential macronutrients, 1-aminocyclopropane-1-carboxylic acid (ACC) deaminase activity, and production of phytohormones such as auxin, cytokinin, gibberellin, ethylene, abscisic acid, jasmonic acid, and salicylic acid (Arora, 2015; Gupta et al., 2015; Alaylar et al., 2018; Backer et al., 2018; Mhlongo et al., 2018). PGPR also promote plant growth indirectly by increasing the production of various volatile organic compounds (VOCs) such as alkanes, alkenes, alcohols, ketones, terpenoids, and sulfur compounds, production of antibiotics, hydrolytic enzymes, hydrogen cyanide (HCN), lipopeptides (LPs), and induced systematic resistance (ISR) (Gupta et al., 2015; Backer et al., 2018; Mhlongo et al., 2018). PGPR produce ISR in plants, which activates pathogenesis-related genes, mediated by phytohormone signaling pathways and defense regulatory proteins to prime plants against future pathogen attack (Pieterse et al., 2014). Regardless of being the second most important macronutrient required for plant growth and development next to nitrogen, phosphorus (P) is unavailable to plants due to its least mobility and poor solubility. P plays an important role in carrying out metabolic processes such as photosynthesis, signal transduction, cell division, nutrient transport, and macromolecular biosynthesis, increasing the efficiency of nitrogen fixation in legumes and respiration in plants (Alaylar et al., 2019; Billah et al., 2019). It is a fundamental component of enzymes, coenzymes, proteins, phospholipids, nucleotides, and nucleic acids (Kafle et al., 2019; Kalayu, 2019; Alayler et al., 2020). Soil contains ~0.05% (w/w) P, and only 1% of this is available for plants' use (Alori et al., 2017). The requirement of P is traditionally fulfilled by the continuous addition of chemical fertilizers during each crop cycle. Plants can utilize only a smidgen amount of these fertilizers and the remaining portion precipitates out into the soil as perennial complexes, resulting in numerous adverse effects on Mother Nature like environmental degradation by the emission of CO2, eutrophication (Youssef and Eissa, 2014; Bhattacharyya et al., 2020), soil fertility depletion (Gyaneshwar et al., 2002), consumption of non-renewable energy, etc.
Phosphate-solubilizing microbes (PSMs) are eco-friendly and economically sustainable. Several PSM stains from bacterial genera (Serratia, Bacillus, Erwinia, Pseudomonas, Enterobacter, Rhizobium, Acinetobacter, Burkholderia, Micrococcus, Flavobacterium, Achromobacter, and Agrobacterium), fungal genera (Penicillium and Aspergillus), arbuscular mycorrhizal (AM) fungi, actinomycetes (Streptomyces), and cyanobacteria (Calothrix) are predominant (Alori et al., 2017). Some of the important PSMs in various plants are listed in Supplementary Table 1. Inorganic phosphate solubilization and organic phosphate mineralization are the predominant ways of dissemination of P in the soil by PSMs (Alori et al., 2017). Inorganic phosphorus like Ca3(PO4)2, FePO4, and AlPO4 are solubilized in the soil in the following ways: production of low-molecular-weight organic acid, inorganic acid (such as sulfuric, nitric, and carbonic acids), and H2S, proton release from (assimilation/respiration), direct oxidation pathway, production of siderophores, and exopolysaccharides (EPSs) (Rodríguez and Fraga, 1999; Sharma et al., 2013; Zhao et al., 2014; Alori et al., 2017). The main attention of this study is the phosphorus solubilization by organic acid production like gluconic, 2-keto gluconic, citric, oxalic, malic, fumaric, malonic, tartaric, glutaric, propionic, butyric, glyoxylic, and adipic acid exuded by PSMs (Sharma et al., 2013). The organic acid is produced by the oxidation pathway or fermentation and respiration of organic carbon compound in periplasmic space (Zhao et al., 2014). Excretion of organic acid chelates the phosphorus-bound cations such as Fe3+, Al3+, and Ca2+ (Goldstein, 1994) through their hydroxyl and carboxyl groups and lowers the rhizospheric pH through O2/CO2 exchange and proton-bicarbonate balance, thereby releasing the bound phosphorus (Kim et al., 1997). Gluconic acid and 2-ketogluconic acid are the most common acids excreted by PSMs. Previously, it has been reported that PSMs improve the growth and yield of various crops, including rice, maize, wheat, sugarcane, legumes, soybean, mustard, chickpea, peanut, sugar beet, tomato, potato, etc. (Rodríguez and Fraga, 1999; Alori et al., 2017; Kalayu, 2019). Thus, inoculating seeds/crops/soil with PSMs as biofertilizers is a promising approach to improve world food production without causing any environmental hazards (Alori et al., 2017). Glucose dehydrogenase (GDH) enzyme encoded by glucose dehydrogenase (gcd) gene accompanied by cofactor PQQ (pyrroloquinoline quinine) forms gluconic acid (An and Moe, 2016).
PQQ is a small, low-molecular-weight redox-active cofactor for several bacterial glucose/alcohol dehydrogenases and participates in methylotrophic metabolism (Duine, 1999; Anthony, 2001; Matsutani and Yakushi, 2018). The biosynthesis of PQQ is accomplished by the gene products of a specific PQQ operon that comprises six genes, pqqA–F (Meulenberg et al., 1992). In the PQQ operon, genes can be organized differently in various organisms. For example, in Acinetobacter calcoaceticus, pqqF is absent in the PQQ operon (Goosen et al., 1987, 1989); in Methylobacterium exotorquens, pqqD fuses with pqqC and pqqA–E gene present in the PQQ operon, but pqqF and pqqG are located outside the operon (Morris et al., 1994; Chistoserdova et al., 2003). It has been proposed that pqqF/pqqG is a labile element within the PQQ operon. Genetic knockout studies of pqqF suggest that it is not essential for cofactor production; other non-specific cellular proteases can make up for that during PQQ biogenesis (Velterop et al., 1995). Conservation of pqqA, pqqC, pqqD, and pqqE is an essential requirement for this pathway that is recognized by genetic knockout experiment (Sonnenburg and Sonnenburg, 2019). Klebsiella pneumoniae needs six genes designated as pqqA, pqqB, pqqC, pqqD, pqqE, and pqqF (Puehringer et al., 2008); seven genes are required for Methylobacterium extorquens (AM1) designated as pqqDGCBA and pqqEF (Morris et al., 1994), whereas A. calcoaceticus requires only four genes (gene I, II, III, and IV) (Goosen et al., 1989) for PQQ synthesis. In Pseudomonas fluorescens B16, the PQQ operon consists of 11 genes (pqqABCDEFHIJKM) (Choi et al., 2008). PqqA is a 22-amino-acid peptide containing conserved Glutamic acid (Glu) and Tyrosine (Tyr); side-direct mutagenesis identified these two residues on PqqA as a provider of carbon and nitrogen atoms required for PQQ synthesis (Goosen et al., 1987). Barr et al. (2016) reported carbon–carbon bond formation between Glu15 and Tyr19 side chain within the precursor peptide PqqA in the presence of PqqE and peptide chaperone PqqD. PQQ operon forms PQQ by the following chemical steps (Supplementary Figure 1): at first, PqqD interacts with PqqA to modify Glu and Tyr side chains of PqqA, and this modified complex gets attached to the active side of PqqE. PqqD is a small, free protein, but in the reaction, it fuses with PqqC at the C-terminal and with PqqE at the N-terminal (Shen et al., 2012). PqqE is a radical S-adenosyl-L-methionine (SAM) protein that contains a conserved CXXXCXXC motif (Menendez et al., 1995) and a [4Fe-4S] cluster near the N-terminal (Broderick et al., 2014), with a C-terminal SPASM domain containing two more iron–sulfur clusters [4Fe-4S] or [2Fe-2S] in Auxiliary Site 1 (Aux1) (Grell and Goldman, 2014; Saichana et al., 2017; Tao et al., 2019) and Auxiliary Site 2 (Aux2). The [4F-4s] cluster near the N-terminal in PqqE promotes the cleavage of SAM, in the presence of reducing reagent (Sodium dithionite) that leads to the formation of a 5′-deoxyadenosyl radical that eliminates a hydrogen atom from the conserved glutamate side chain of PqqA, to establish C–C cross-linking to conserve Tyr (Wecksler et al., 2009). This product is hydrolyzed by the PqqF/PqqG to cut off N- and C-terminal amino acids and cellular proteases generate a Glu-Tyr di-amino acid for PqqB. PqqB acts as an oxygenase that hydroxylates Tyr of Glu-Try di-amino acid into a 3,4 dihydroxy intermediate that oxidized again to a trihydroxy derivative of Tyr (Klinman and Bonnot, 2013; Koehn et al., 2019). Then, it undergoes spontaneous cyclization yielding 3a-(2-amino-2-carboxyethyl)-4,5-dioxo-4,5,6,7,8,9-hexahydroquinoline-7,9-dicarboxylic acid (AHQQ) as the substrate for the final oxidative steps catalyzed by PqqC (Zhu and Klinman, 2020). Escherichia coli and Salmonella typhimurium are impuissant to produce PQQ due to the lack of genes required for PQQ biosynthesis and, consequently, obtain the PQQ necessary for their survival in that environment (Matsushita et al., 1997; RoseFigura, 2010). It has been reported that strain EF260, a derivative of E. coli FB8, can synthesize PQQ after mutation and can oxidize glucose to gluconic acid via the GCD/PQQ pathway (Biville et al., 1991).
A previous study has reported that the expression of PqqA, a precursor of PQQ, is much higher than other Pqq proteins by using Pqq-lacZ protein fusion (Velterop et al., 1995). The authors have also depicted that the pqq genes can be expressed in anaerobic conditions but cannot produce PQQ, though synthesis of PQQ requires molecular oxygen. Toyama et al. (1997) reported that in Methylobacterium extoroquens AM1, PqqE is homologous to MoaA and nifB in the N-terminal region. It has been well-documented that MoaA protein involved in an early stage in the biosynthesis of the molybdopterin cofactor (Rajagopalan and Johnson, 1992; Rivers et al., 1993), and nifB is involved in an early step in the biosynthesis of the cofactor of nitrogenases (FeMo-, FeV-, and FeFe-cofactors). PQQC/D enzyme reaction requires molecular oxygen and reduced nicotinamide adenine dinucleotides (Toyama et al., 1997). Using Pulsed-field gel electrophoresis (PFGE), a DNA fingerprinting method for bacterial isolation has observed that the ribF gene that produces riboflavin cofactor for gluconic acid dehydrogenase is not closely linked with pqq genes. DNA sequencing of Tn5-induced glucose dehydrogenase (GDH)-deficient mutant of Gluconobacter oxydans IFO3263 has revealed that identity to pqqE gene in the insertion site appeared in an open reading frame (Felder et al., 2000). To evaluate whether a PQQ biosynthetic gene is suitable to study the phylogeny of phosphate-solubilizing pseudomonads, two new primers were designed (pqqCf1 and pqqCr1) that specifically amplify the pqqC gene of the Pseudomonas spp. isolated from the wheat root (Meyer et al., 2011). Meyer et al. (2011) reported that the pqqC gene is an excellent molecular marker to study the diversity and evolution of phosphate-solubilizing pseudomonads as pqqC polymorphism is high to ensure an exemplary phylogenetic resolving power complementary to the conventionally used housekeeping genes gyrB and rpoD. Phylogenetic analyses have also reported on pqqC genes in Pseudomonas sp. (Xu et al., 2014). PQQ-producing bacteria are invasive (≈50%) and pathogenic (≈25%), which indicate no association between PQQ biosynthetic capabilities and pathogenicity. A total of 144 distinct species have been found by performing sequence alignment of pqqC, pqqD, and pqqE genes. The majority of these are α, β, and γ classes of proteobacteria, prevalent in Gram-negative bacteria, wild-type pqq, and various active site mutants (RoseFigura, 2010). Regulation of glucose dehydrogenase and PQQ cofactor in the model Pseudomonas putida KT2440 of broccoli rhizosphere soil has been analyzed. In this study, glucose dehydrogenase and PQQ levels vary upon growth condition, with a high level of glucose as the sole carbon source and low soluble phosphate (An and Moe, 2016). Highly efficient phosphate-solubilizing bacteria (PSB) Burkholderia ultivorans WS-FJ9 from pine tree rhizosphere solubilizes both organic and inorganic phosphate. The amount of solubilized inorganic phosphates by the WS-FJ9 strain is ~140 mg/L. AP-2, gspE, and gspF genes are related to organic phosphate, hlyB is related only to inorganic phosphate, and phoR, phoA, AP-1, and AP-3 are related to both, as observed by using Next-Generation Sequencing (NGS) technology (Liu et al., 2020). Most of the studies on the phylogenetic analyses have targeted either individual or two genes, and none of them has employed every single gene involved in the entire PQQ biosynthetic pathway for understanding their evolution in Gammaproteobacteria. Phylogenetic analysis of pqqA, pqqB, pqqD, and pqqE genes has not been reported so far in Gammaproteobacteria. Hence, for the very first time in this article, a systematic study of the complete set of genes from selected Gammaproteobacteria, involved in the entire PQQ biosynthetic pathway, are studied to understand their phylogenetic relationships.
Codon usage bias is the non-random use of synonymous codons, observed in a wide range of organisms such as bacteria, yeast, plants, and mammals and mainly determined by mutation (e.g., GC content, mutation frequency, and their pattern) and natural or translational selection (e.g., gene expression level, tRNA abundance, protein length, gene translation initiation signals, and protein structure) (Ikemura, 1981, 1985; Gouy and Gautier, 1982; Sharp et al., 1986; Bulmer, 1991; Duret and Mouchiroud, 1999; Gu et al., 2004; Wan et al., 2004; Trotta, 2013). Studies have discovered that mutational pressure act upon genes encoding common and uncommon amino acids (Hershberg and Petrov, 2008), whereas selection favors specific codons that promote efficient and accurate translation of genes with a high level of expression (Duret, 2000; Hershberg and Petrov, 2008). Several studies on codon usage have revealed that other factors could influence codon usage patterns as well, including secondary protein structure, replication, hydrophobicity, and hydrophilicity of the protein and the external environment (Lobry and Gautier, 1994; D'Onofrio et al., 2002; Sharp et al., 2005). Codon usage bias helps to understand the evolution of different organisms, in addition to their environmental adaptation (Angellotti et al., 2007). In context, natural consortium of five acidophilic bacteria used for biomining preferentially has low codon usage bias. Bacterial behavior in community/consortia cannot be equated at all times with their isolated state (Hart et al., 2018).
The prime objectives of this contemporary study are to (i) investigate the phylogenetic relationships of pqq genes in selected Gammaproteobacteria for an advanced understanding of their functional evolution; (ii) reveal the amino acid composition of the selected proteins and nucleotide composition of the encoded genes along with their organization within the genome of the selected microorganisms; (iii) discern whether the pqq genes are affected by mutational and selection pressure; (iv) investigate domain analysis of pqq genes with glucose dehydrogenase; (v) investigate correspondence analysis (COA) and codon usage bias of the proteins and encoded genes involved in PQQ biosynthesis from Gammaproteobacteria to obtain an insight into the major forces that shape synonymous codon usage bias; (vi) recognize the optimal codons that provide useful information about genetic engineering, gene prediction, and molecular evolutionary studies; and (vii) measure the Codon Adaptation Index (CAI) as an indicator of the adaptability of those genes.
This study will provide an insight into the compositional analysis of codon usage in pqq genes from phosphate-solubilizing Gammaproteobacteria based on GC content along with GRAVY (Grand average of hydropathicity) and aromaticity score, amino acid composition, the relationship between GC3% and expected effective number of codons (ENc or Nc), neutrality plot, and exploration of a crucial statistical method for the analysis of the data. Analysis of various codon usage indices can provide a better understanding of the pattern of synonymous codon usage bias in pqq genes from Gammaproteobacteria. Moreover, the information on optimal codons and factors affecting codon usage will facilitate further research in molecular phylogenetics and genomics and will help to better understand the evolutionary dynamics of the PQQ biosynthetic pathway valuable for further biotechnological studies.
Our analyses have given a novel insight into the codon usage patterns of the genes involved in the PQQ biosynthetic pathway that would assist in better understanding of the synonymous codon usage pattern as well as the factors influencing it.
Materials and Methods
Collection of Data
Homologous pqq gene sequences of 78 Gammaproteobacteria-−33 species of Klebsiella, 2 of Enterobacter, 12 of Pantoea, 1 of Nissabacter, 1 of Erwinia, 4 of Kosakonia, 6 of Rahnella, 3 of Mixta, 5 of Cronobacter, 2 of Rouxiella, and 8 species of Serratia—were obtained by performing homology-based blastn (Altschul et al., 1997) searches using Serratia marcescens (DQ868536.1) sequence as queries (the parameter of target sequences 1,000; other parameters as default) and downloading those sequences from the National Center for Biotechnology Information (NCBI) database (http://www.ncbi.nlm.nih.gov/Genbank/index.html) (Supplementary Table 2). Sequence similarities of ≥70% with the sequence coverage of ≥98% were taken into consideration for sequence selection. Coding DNA sequences (CDS) were downloaded from the PATRIC2 database (www.brcdownloads.vbi.vt.edu/patric2/genomes/). In our study, we have used 11 bacterial genera, among them Klebsiella, Enterobacter, Kosakonia, Cronobacter, Nissabacter, and Rahnella belong to Enterobacteriaceae family; Pantoea, Erwinia, and Mixta belong to Erwiniaceae family; and Rouxiella and Serratia belong to Yersiniaceae family.
Phylogenetic Analysis
To study the evolutionary relationship of the pqqA, pqqB, pqqC, pqqD, and pqqE genes of 79 Gammaproteobacteria including S. marcescens (DQ868536.1) and different species, subspecies, and strains of Klebsiella, Enterobacter, Pantoea, Nissabacter, Erwinia, Kosakonia, Rahnella, Mixta, Cronobacter, Rouxiella, and Serratia, multiple sequence alignment (MSA) of the protein sequences was performed with Clustal W (Thompson et al., 1994) alignment program with default parameter values (gap opening penalty of 10 and a gap extension penalty of 0.20) available in MEGA6.60 (Molecular Evolutionary Genetics Analysis) (http://www.megasoftware.net/; Tamura et al., 2013). The phylogenetic trees based on the MSA of their protein sequences were constructed by using the unrooted Neighbor-joining method (NJ) (Saitou and Nei, 1987) using p-distance with a uniform rate and complete deletion of gaps/missing data, and the confidence level of each node was estimated by the Bootstrap method using 1,000 replications and displayed using MEGA6.06 program.
In bacterial phylogenetic studies, the 16S rRNA gene is one of the most frequently used genes. Phylogenetic analysis of 16S rRNAs allows the accurate statistical measurement of a broad range of evolutionary relationships due to highly conserved sequences. Therefore, we have used 16S rRNA gene sequences to understand the evolutionary relationship among various Gammaproteobacteria. After collecting the 16S rRNA sequences of 79 Gammaproteobacteria from NCBI (https://www.ncbi.nlm.nih.gov), a phylogram was constructed to analyze the relationship of those 16S rRNA genes.
Multiple Sequence Alignment
To study the conservation of the amino acids in the different sites within the domains, MSA was performed with ClustalOmega (http://www.ebi.ac.uk/Tools/msa/clustalo; Sievers et al., 2011) with the default parameters (gap penalty 10.0, gap extension penalty 0.05, weight matrix BLOSUM) and the JalView display option was used along with the sequence logo for the same by using WebLogo-3 (http://weblogo.threeplusone.com/; Crooks et al., 2004) (http://weblogo.berkeley.edu/logo.cgi).
Detection of Conserved Domains
For the identification of the different domains present in the pqqA–pqqE proteins from selected organisms, the identified protein sequences were analyzed using the Conserved Domain Search tool (http://www.ncbi.nlm.nih.gov/Structure/cdd/wrpsb.cgi) against Pfam database and HMMSCAN (http://www.ebi.ac.uk/Tools/hmmer/search/hmmscan) with default parameters.
Compositional Analysis and Codon Usage Indices
Codon W 1.4.4 (http://codonw.sourceforge.net/, Pedan, 2000) was used to calculate the indices of codon usage. Two single codons for methionine (AUG) and tryptophan (UGG) and three stop codons (UAA, UAG, and UGA) were excluded from the calculation. The following codon indices were determined: GC-content at the first, second, and third codon positions (GC1, GC2, and GC3); frequency of either G or C at the third codon position of synonymous codons (GC3s); and the average of GC1% and GC2%, i.e., GC12%, general average hydropathicity (GRAVY) score (Kyte and Doolittle, 1982), Aromaticity score (Lobry and Gautier, 1994), and Effective number of codons (ENc) (Wright, 1990). The abovementioned parameters were calculated using the cDNA sequences of the genes obtained from Gammaproteobacteria.
GRAVY score was calculated as the sum of the hydropathy values for all the amino acids in a protein divided by the total number of residues in it (Kyte and Doolittle, 1982). A more negative GRAVY value indicates that the protein is more hydrophilic and vice versa. Aromaticity scores denote the frequency of aromatic amino acids Phenylalanine (Phe), Tyrosine (Tyr), and Tryptophan (Trp) in the hypothetically translated gene product (Lobry and Gautier, 1994). The hydropathicity and aromaticity protein scores are indices of amino acid usage and the variation in amino acid compositions can influence the results of codon usage analysis. The 20 amino acids have been classified into five groups based on their polarity and charge, i.e., Acidic polar: Aspartic acid (D) and Glutamic acid (E); Basic polar: Histidine (H), Lysine (K), and Arginine (R); Aromatic: Phenylalanine (F), Tyrosine (Y), and Tryptophan (W); Neutral non-polar: Proline (P), Cysteine (C), Methionine(M), Glycine (G), Alanine (A), Valine (V), Isoleucine (I), and Leucine (L); and Neutral polar: Glutamine (Q), Asparagine (N), Serine (S), and Threonine (T). An in-house PERL program was used to calculate the amino acid frequency of the protein encoded by pqqA, pqqB, pqqC, pqqD, and pqqE genes, using a particular codon for each time.
Relative synonymous codon usage (RSCU) is another well-known measure of codon bias, used to examine the frequency of each synonymous codon that encodes the same amino acid. It was calculated as the ratio of the observed frequency of codons relative to the expected frequency of the codon under a uniform synonymous codon usage (Sharp and Li, 1986). RSCU values are not affected by sequence length and amino acid frequencies since these factors were eliminated during the computation. RSCU values of every codon for pqqA, pqqB, pqqC, pqqD, and pqqE genes were calculated using an in-house or home-based PERL program (Mondal et al., 2016). A codon with an RSCU value of more than 1.0 has a positive codon usage bias, while a value of <1.0 has a negative codon usage bias. RSCU value equal to 1.0 indicates that all the synonymous codons encoding the same amino acid were used equally and randomly, and that is nearly unbiased (Sharp and Li, 1986). Moreover, the synonymous codons with RSCU values >1.6 and <0.6 are considered as over-represented and under-represented codons, respectively (Sharp and Li, 1986).
The neutrality plot is an analytical method used to measure codon usage patterns. To estimate the extent of directional mutational pressure against selection in the codon usage bias, a neutrality plot was drawn that reveals the results of the equilibrium coefficient of mutation and selection. GC12%, i.e., the average of GC1% and GC2% along the y-axis, is plotted against GC3% along the x-axis (Sueoka, 1988). In neutrality plots, if the dots are distributed diagonally with a regression coefficient value close to 1, mutation bias is assumed to be the principal force, shaping codon usage, whereas scattered distribution of dots suggests a significant role of natural selection with the regression coefficient close to zero (Sueoka, 1988).
The ENc or Nc vs. GC3% plot is widely referred to ascertain whether the codon usage of a gene is affected by mutational or selection pressure (Wright, 1990). An ENc or Nc plot has been drawn using the ENc or Nc value as the ordinate and the GC3 value as the abscissa. When the corresponding points fall near the expected curve, the mutation is considered as the principal force shaping codon usage, and when the corresponding points fall considerably below the expected curve, the prime force shaping codon usage is the selection. The ENc or Nc is used to measure the codon bias in an individual gene that is essentially independent of gene length (Wright, 1990). In this study, the ENc values were calculated for each gene of the selected microorganisms from GC3s under H0 (Null hypothesis, i.e., no selection) referring to the given equation, where S denotes GC3 (Wright, 1990).
The ENc value ranges from 20 (for a gene with extreme bias using only one codon per amino acid) to 61 (for a gene with no bias, using synonymous codons equally), and in case the value of ENc is >40, the codon usage bias is regarded as low (Wright, 1990). ENc values <35 denote higher codon usage bias, and the value that is more than 50 denotes general random codon usage (Wright, 1990).
Correspondence Analysis
The COA is a widely employed multivariate statistical method used to study the significant trends in codon usage variation among genes. In COA, all genes are plotted in a 59-dimensional hyperspace according to the RSCU values of 59 sense codons (Greenacre, 1984). Sequences where a given codon is applied in a similar fashion lie close to each other on the graph. COA provides an important trend of factors related to codon usage in different gene sets. The major trends in codon usage variation can be determined with relative inertia, according to which the genes are located to investigate the major factors affecting the codon usage pattern.
To investigate the major trend in RSCU variation among genes and distribute the genes along continuous axes in accordance with these trends, the COA (Sharp et al., 1986) has been performed using the program Codon W 1.4.4.
Determination of Optimal Codons
Based on the principal axis of COA (axis 1), the top and the bottom 5% of genes were considered as the high and low datasets, respectively. When one codon's RSCU values in both the datasets were significantly correlated by a two-way Chi-squared contingency test (p < 0.01), then the codon was defined as optimal codon (Liu, 2006; Huang et al., 2017; Begum and Mondal, 2020).
Expressional Probability
The geometric mean of the weight associated with each codon over the length of the gene sequence (measured in codons) is considered as the CAI (Sharp and Li, 1987), which measures the degree with which genes use preferred codons and assess the level to which selection can effectively mold the pattern of codon usage. The CAI values may range from 0 to 1, and the larger ones reveal a higher gene expression level as well as codon bias. The Sharp and Li method has been followed to calculate the CAI by employing an in-house PERL script and MS Excel 2007.
Statistical Analysis
We have calculated the average and standard deviation of RSCU of the codons of pqqA, pqqB, pqqC, pqqD, and pqqE genes of selected Gammaproteobacteria and amino acid frequencies for the proteins encoded by the genes in different microorganisms and for the entire selected microorganisms as well. To understand the preference of either codon on the respective microorganisms, Student's t-test (Ewens and Grant, 2001) was performed based on G- and C-ending codons of amino acids having a minimum of four degenerative codons with variation only in the third position using Microsoft Office Excel 2007. Under the null assumption, t statistics were calculated, from which the p-value was derived and taken as significant when p < 0.05.
Results and Discussion
Phylogenetic Analysis
The phylogenetic tree based on PqqA amino acid sequences (Supplementary Figure 2) has disclosed a proximate relation between Klebsiella and Enterobacter together with Pantoea, Nissabacter, Erwinia, Kosakonia, and Rahnella; Mixta, Cronobacter, Rouxiella, and Serratia. Phylogenetic analyses of PqqA, PqqB, PqqC, PqqD, and PqqE proteins involved in the PQQ biosynthetic pathway have promulgated a close association of Klebsiella and Enterobacter. Similarly, a phylogenetic tree based on PqqB amino acid sequences (Supplementary Figure 3) has revealed a close association of Nissabacter, Pantoea, Rouxiella, Erwinia, and Rahnella; Kosakonia, Mixta, and Cronobacter and all the species of Serratia. The phylogenetic tree based on the amino acid sequences of PqqC (Figure 1) has displayed a close association of Kosakonia and Cronobacter; Rouxiella and Rahnella, including the entire set of species of Pantoea and Serratia as well. By the same token of the above case, the phylogenetic tree based on PqqD amino acid sequences (Supplementary Figure 4) has revealed a close interrelation of Kosakonia and Cronobacter; Erwinia, Nissabacter, and Rahnella; three species of Mixta; and every selected species of Serratia. The phylogenetic tree based on the amino acid sequences derived from the pqqE genes (Supplementary Figure 5) has exhibited a neighboring association of Kosakonia and Cronobacter; Erwinia, Rouxiella, and Rahnella; as well as every single species of Serratia.
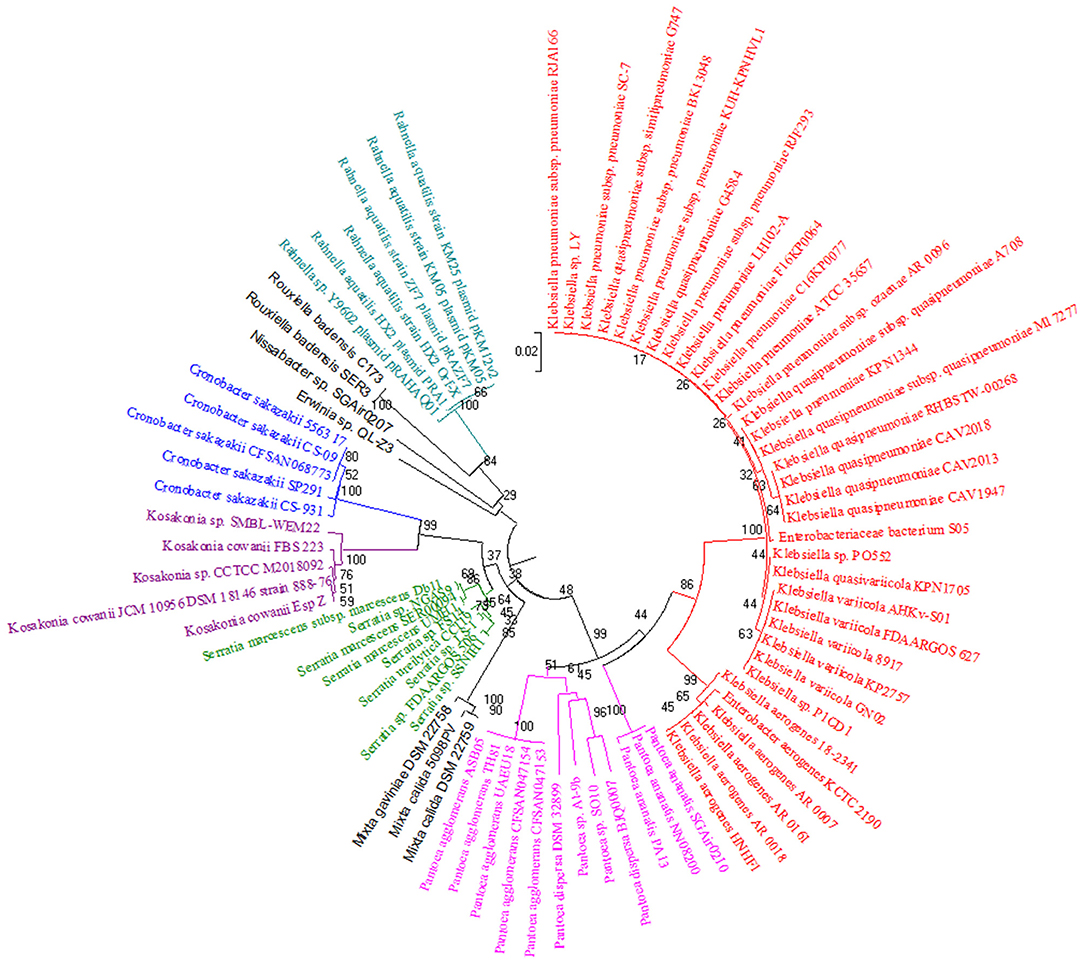
Figure 1. Phylogenetic tree showing the relationship between the diverse Gammaproteobacterial groups considered in this study based on homologs of PQQ biosynthesis pathway-associated pqqC gene sequences. Amino acid sequences of pqqC genes from S. marcescens (DQ868536.1) and their homologous sequences from various Gammaproteobacterial species, including Klebsiella, Enterobacter, Pantoea, Nissabacter, Erwinia, Kosakonia, Rahnella, Mixta, Cronobacter, Rouxiella, and Serratia, were aligned with Clustal W algorithm, and the unrooted phylogenetic tree was constructed by the Neighbor-joining method based on the p-distance using MEGA6.06 software (http://www.megasoftware.net/, Kumar et al., 2008). The bootstrap consensus tree inferred from 1,000 replicates is taken to represent the evolutionary relationship of the taxa analyzed (Felsenstein, 1985). The number in each node indicates the confidence value of that branch after bootstrapping the phylogenetic tree. A uniform rate was used to model evolutionary rate differences among sites. Complete deletion of gaps/missing data was used. Phylogenetic trees generated from pqqA, pqqB, pqqD, and pqqE are shown in Supplementary Figures 1–4.
Overall, the phylogenetic analysis indicated a relatively close phylogenetic association of Klebsiella and Enterobacter, and all selected species of Pantoea, Rouxiella, Rahnella, Kosakonia, Mixta, Cronobacter, and Serratia. There was a close association of Kosakonia and Cronobacter in pqqC, pqqD, and pqqE genes. The similarity between the proteins of each clade may reflect their evolutionary interrelation.
Phylogenetic analyses have reported on pqqC genes in Pseudomonas sp. (Meyer et al., 2011; Xu et al., 2014). Meyer et al. (2011) reported that the pqqC gene is suitable to study the phylogeny of phosphate-solubilizing Pseudomonas populations in natural habitats. Comparing pqqC phylogeny and phosphate solubilization activity, they identified one phylogenetic group with high solubilization activity. The phylogenetic tree of the protein coding sequences of the pqq operon showed two main clusters of phosphate-solubilizing endophytic P. fluorescens strains (Oteino et al., 2015). These two clusters were consistent with the presence or absence of a 139- to 204-bp intergenic region between the pqqB and pqqC genes (Oteino et al., 2015). Matsumura et al. (2014) found that basidiomycete Coprinopsis cinerea (mushroom) contains a new type of PQQ-dependent sugar oxidoreductase. Phylogenetic analyses from the amino acid sequences of Coprinus cinereus sugar dehydrogenase (CcSDH), proteins homologous to CcSDH, and known quinoproteins suggested that these quinoproteins may be members of a new family that is widely distributed not only in prokaryotes, but also in eukaryotes (Matsumura et al., 2014). Phylogenetic analysis of full-length sequences of pqqE gene of several bacterial strains revealed that it was related to other Serratia strains, closely related to S. marcescens strains (Ludueña et al., 2017). Earlier, it has been reported that the pqqE gene is highly conserved within genus taxonomic level (Wecksler et al., 2009). The phylogenetic and codon usage analysis of the soil bacteria consistently demonstrated the relatedness of Azotobacter chroococcum with different species of the genus Pseudomonas (Saha et al., 2019).
The phylogenetic tree obtained using 16S rRNA sequences (Figure 2) manifested a relatively close phylogenetic interconnection of Klebsiella and Enterobacter that authenticates our previous phylogenetic tree. It was observed that there was a close association of seven species of Serratia that appeared after 30 species of Klebsiella and Enterobacter, whereas 2 species of Serratia were observed between 8 species of Pantoea and 3 species of Klebsiella. Eventually, three species of Mixta appear in between three species of Pantoea at the forepart and six species of Pantoea at the tag end in contrast, which does not corroborate our previous phylogenetic tree. Mukhtar et al. (2020) identified halophilic bacteria using 16S rRNA sequence analysis and characterized their plant growth-promoting abilities. They had shown that bacterial strains belonging to Bacillus, Halobacillus, and Pseudomonas were dominant in the rhizosphere of halophytes. The phylogenetic tree based on gyrB, rpoB, and the 16S rRNA sequences of Alteromonas species revealed that the four Alteromonas stellipolaris strains showed a high level of relatedness among this group of species (Torres et al., 2019).
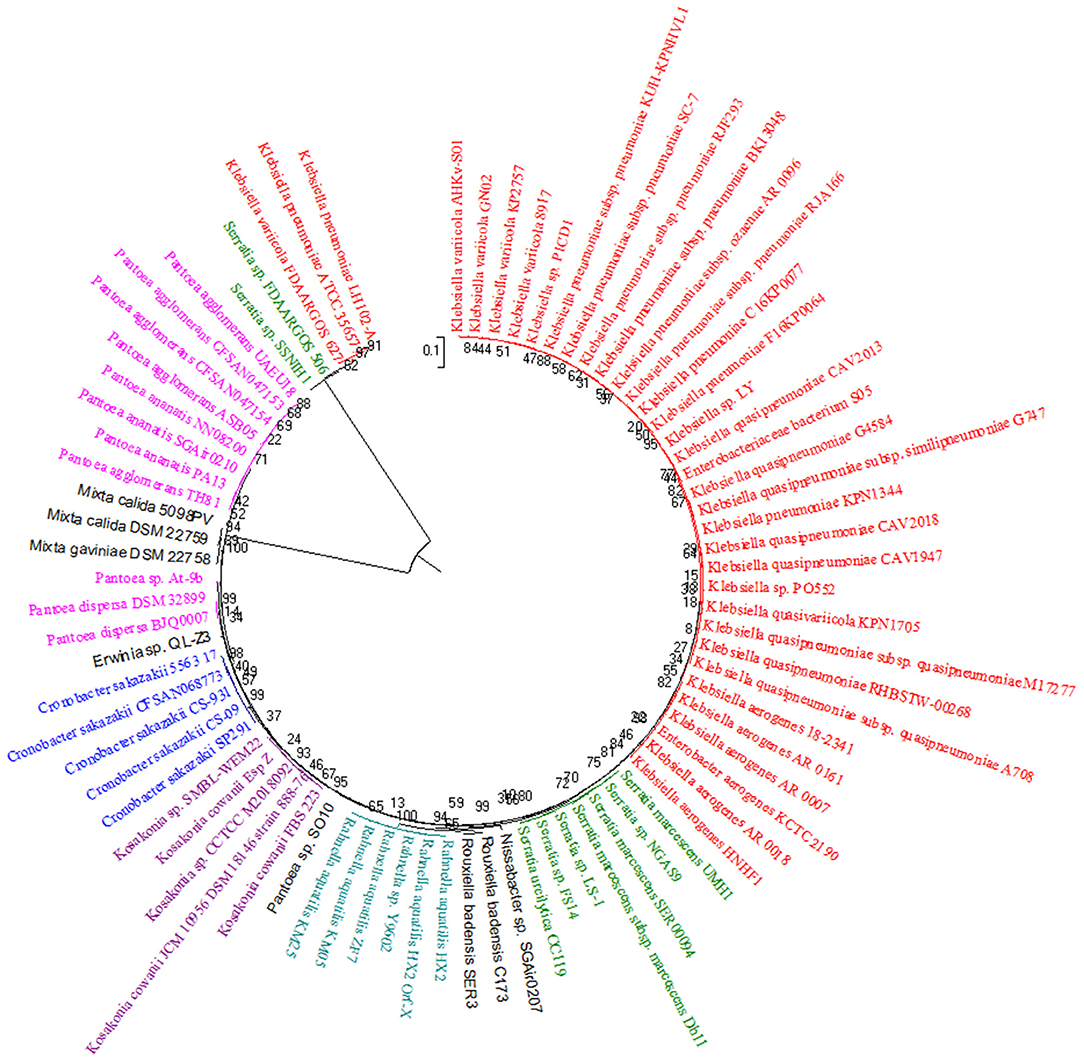
Figure 2. A phylogenetic tree showing the relationship based on 16S rRNA gene sequences among the diverse Gammaproteobacterial groups considered in this study. 16s rRNA gene sequences were aligned with the Clustal W algorithm and an unrooted by the Neighbor-joining Maximum Composite Likelihood (MCL) method (NJ-MCL) using MEGA6.06 software (http://www.megasoftware.net/, Kumar et al., 2008). The bootstrap consensus tree inferred from 1,000 replicates is taken to represent the evolutionary relationship of the taxa analyzed (Felsenstein, 1985). The number in each node indicates the confidence value of that branch after bootstrapping the phylogenetic tree. A uniform rate was used to model evolutionary rate differences among sites. Complete deletion of gaps/missing data was used.
Multiple Sequence Alignment
MSA methods refer to a series of algorithmic solutions for aligning three or more homologous biological sequences (DNA, RNA, or protein) considering evolutionary events (mutations, insertions, deletions, etc.) gradually. MSA was performed to study amino acid conservation at PqqA, PqqB, PqqC, PqqD, and PqqE protein sequences.
In the Jalview representation, the corresponding height of the different amino acid residues in the sequence logo and the color conservation at the different sites disclose a few variations in the PQQ encoding genes, suggesting the level of conservation of amino acids. In contrast to the fully conserved amino acid sites, the non- or semi-conserved sites are more beneficial for providing information for evolutionary study. The amino acid pattern present in the non/semi-conserved sites gathers quite useful facts on the possible places of changes or mutations (Supplementary Table 3) that occurred in these sequences during evolution, accentuating differences in the organization of the identified proteins in different clades, as evidenced in the phylogenetic analyses (Figure 3).
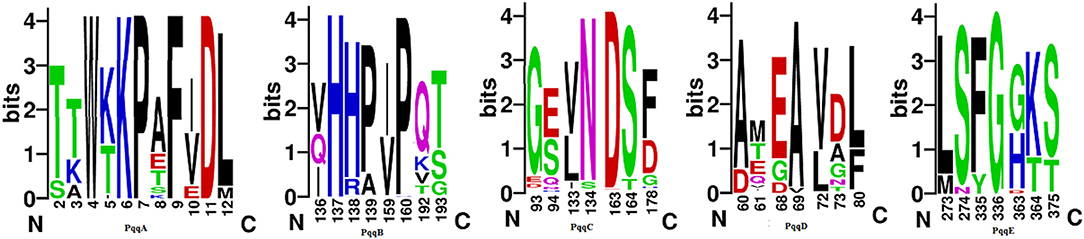
Figure 3. Semi-conservative sites of PqqA–PqqE proteins. Notes: The conserved sites generated from the MSA of all the identified pqqA–pqqE proteins from different species, subspecies, and strains of Klebsiella, Enterobacter, Pantoea, Nissabacter, Erwinia, Kosakonia, Rahnella, Mixta, Cronobacter, Rouxiella, and Serratia, respectively, were represented in sequence logos based on the alignment of full-length sequences of all the Pqq proteins and their homologous sequences. The overall height of each stack indicates the conservation of the sequence at the corresponding location, while the height of the letters within each stack indicates the relative frequency of the corresponding amino acid. All conserved site-wise analyses have been represented in Supplementary Table 2.
From the Jalview representation, we have observed many non-conserved amino acids present in the PqqA. Depending on that, we have detected several mutable sites as follows with a positive impression over the phylogenetic tree (details of the mutable sites in Supplementary Materials). Site 2 in the aligned condition was occupied by amino acids Serine and Threonine. Serratia, Cronobacter, and Rouxiella have possessed Threonine. Mixta possesses Serine at this site. Serine and Threonine both are neutral, polar, and hydrophilic in nature. Therefore, at this site, a silent mutation has occurred. Effect of mutation is observed on the phylogenetic tree. Site 3 holds amino acids Threonine, Lysine, and Alanine. Serratia and Rouxiella were occupied by the amino acid Threonine. Cronobacter possesses Lysine. Only Mixta possesses Alanine. Alanine is non-polar and hydrophobic, while Lysine and Threonine are polar and hydrophilic. Alanine and Threonine are neutral, while Lysine is basic. Considering this, a mutation occurred at that site, and phylogenetic impact was observed. Just as in PqqB, a kind of similarity was noticed for the mutable sites from the Jalview representation too (details of the mutable sites in Supplementary Materials). At site 193, Serratia possesses Glycine. Kosakonia, Cronobacter, Mixta, Nissabacter, and Rahnella possess Serine and Pantoea, and Klebsiella possesses Threonine at this site. Serine and Threonine both are neutral, polar, and hydrophilic but Glycine, which does not possess any hydrophobicity or hydrophilicity, is neutral, a non-polar special type of amino acid, although a mutation occurred at this site, and the phylogeny impact was notable. Site 159 was almost occupied by the amino acid Isoleucine. Only Klebsiella holds the amino acid Valine. Isoleucine and Valine are neutral, non-polar, and hydrophobic. Considering this, a silent mutation occurs at this site, and phylogenetic impact is observed. By the same token in the conserved domain in PqqC, some inequalities were noted in the event of mutation at different mutable sites (details of the mutable sites in Supplementary Materials). Site 178 holds amino acids Aspartic acid, Histidine, Phenylalanine, and Glycine. Nissabacter, Erwinia, and Rahnella possess the amino acid Glycine. Serratia, Kosakonia, Cronobacter, and Mixta possess Aspartic acid. Only Rouxiella was occupied by Histidine. Pantoea and Klebsiella possess Phenylalanine. Phenylalanine and Glycine both are neutral and non-polar. Phenylalanine is hydrophobic and Aspartic acid and Histidine are hydrophilic. Therefore, a mutation occurred at this site with a positive phylogeny impact. PqqD manifests some mutable sites in Jalview representation too (details of the mutable sites in Supplementary Materials). Site 80 in the aligned condition was occupied by amino acids Phenylalanine and Leucine. Serratia, Nissabacter, Mixta, Rahnella, and Erwinia have possessed Phenylalanine. Kosakonia, Pantoea, Cronobacter, and Klebsiella possess Leucine at this site. Phenylalanine and Leucine are neutral, non-polar, and hydrophobic. Therefore, at this site, a silent mutation has occurred with no phylogeny impact. In PqqE, different kinds of similarities were noticed for the mutable sites from the Jalview representation as well (details of the mutable sites in Supplementary Materials). Site 364 hold amino acids Lysine and Threonine. Serratia, Kosakonia, Cronobacter, and Pantoea dispersa possess the amino acid Threonine. The rest of the organisms possess Lysine. Threonine is neutral; on the other hand, Lysine is basic. Both amino acids are polar and hydrophilic. Although mutation occurred at this site, no phylogenetic impact was observed. In contrast, site 273 was almost occupied by the amino acid Leucine. Serratia filled up with Methionine. Leucine and Methionine both are neutral, non-polar, and hydrophobic. The silent mutation occurred at this site, and the phylogenetic impact was notable. These abovementioned outcomes substantiate the evolutionary relationship of the PQQ protein from selected organisms as acquired in the phylogenetic tree.
Detection of Conserved Domains
Conserved domains are present in PqqA to PqqE (Table 1). Protein domains are the structural, functional, and evolutionary units of proteins.
Compositional Analysis of Codon Usage Based on GC Content
GC content is a very important feature in the analysis of codon usage bias, and the GC contents at the third base of one codon (GC3) are considered to most likely reflect codon usage pattern without deviation (Sueoka, 1988; Palidwor et al., 2010; Mondal et al., 2016). Overall and different positional GC% can provide some information about the relative contribution of mutational and selection pressure on codon usage bias.
After analyzing the results, we found that the average GC3s, GC1%, and GC3% in pqqB, pqqC, pqqD, and pqqE genes were high, whereas in pqqA genes, the average of GC1%, GC2%, and GC3% were comparatively low in all six Gammaproteobacteria. The GC content value for the pqqA genes from Cronobacter, Klebsiella, Serratia, Kosakonia, and Rahnella was 54.62, 46.47, 46.80, 45.84, and 42.36%, respectively, revealing a slightly AT-rich and GC-poor genome (Table 2). The GC content values for the pqqB, pqqC, pqqD, and pqqE genes from Rahnella to Cronobacter were 62.45–68.78%, 65.08–71.43%, 59.81–71.83%, and 61.31–66.89%, respectively, demonstrating a GC-rich genome. It was observed that GC% at the third position was highest in all the pqq genes in Cronobacter but lowest in the pqqA gene of Rahnella compared to other Gammaproteobacteria. pqq genes were found to be GC-rich; the GC content at the one and third position was higher than at the second codon position, and a significant contrast of GC content was found between the first and second positions and second and third codon positions. Hence, the overall nucleotide composition suggested that the nucleotide C and G occurred more frequently in comparison to A and T in the coding sequences, and it was expected that G/C-ending codons might be preferred over A/T-ending codons in the pqqA, pqqB, pqqC, pqqD, and pqqE genes from all Gammaproteobacteria except for pqqA genes from Klebsiella, Serratia, Kosakonia, and Rahnella.
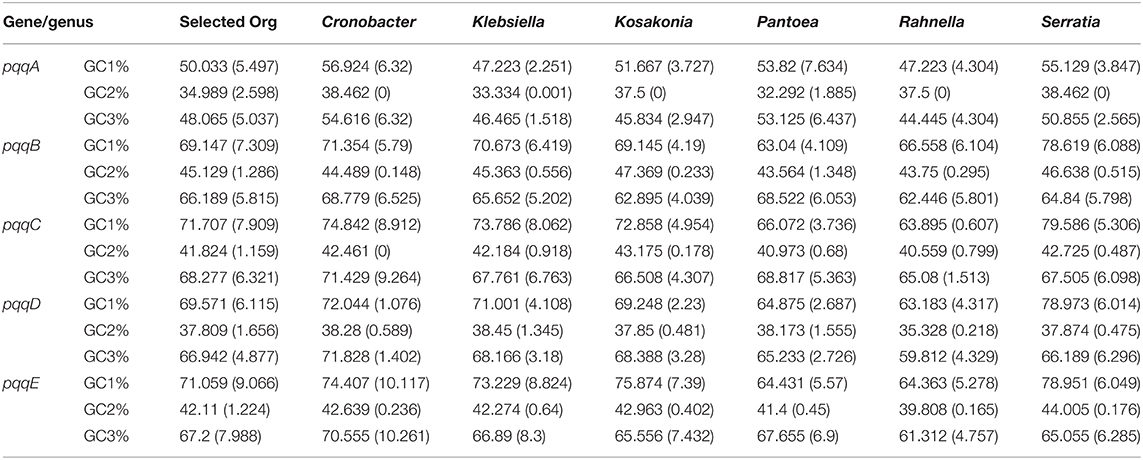
Table 2. Average and standard deviation of different positional GC%s (GC1%, GC2%, and GC3%) values of the pqqA–pqqE genes involved in the PQQ biosynthesis pathway from Cronobacter, Klebsiella, Kosakonia, Pantoea, Rahnella, and Serratia.
Genomic GC content varies both within and, considerably, between microbial genomes. Previous studies reported that the GC content of bacterial genomes ranges from 16 to 75%, and wide ranges of genomic GC content were observed within many bacterial phyla, including both Gram-negative and Gram-positive phyla (Lightfield et al., 2011). An increase in genomic GC content can result in increased bacterial fitness (Raghavan et al., 2012), and this is associated with stronger selection on base composition (Hildebrand et al., 2010; Raghavan et al., 2012; Bohlin et al., 2017). Ran et al. (2014) demonstrated the coupling between the selection on protein sequences and the optimization of codon usage in a broad range of bacteria and archaea. The strength of this coupling varies over a wide range and strongly and positively correlates with the genomic GC content. They proposed that optimization of codon usage could be one of the key factors that determine the evolution of GC-rich genomes (Ran et al., 2014). Abundance of nitrogen, in soil, alters codon bias and has been identified as a driver for increased genomic GC content in parasitic microorganisms and nitrogen-fixing aerobic bacteria (McEwan et al., 1998; Seward and Kelly, 2016). Higher GC3 in genes is involved in cellular metabolism, and lower GC3 is involved in information storage and processing. High GC3 content provides more targets for methylation, which can serve as an additional mechanism of transcriptional regulation and affect the variability of gene expression (D'Onofrio et al., 2007; Tatarinova et al., 2010, 2013). Botzman and Margalit (2011) have demonstrated that variations in prokaryotic global codon usage bias and GC3 are highly associated with species tolerances to environmental variability. On the other hand, the AT content leads to low thermodynamic stability, which plays a crucial role in the initiation of replication (Rajewska et al., 2012).
Neutrality Plot Analysis
To estimate the extent of directional mutation pressure against selection on the codon usage bias, a neutrality plot (GC12% vs. GC3%) was drawn that reveals the results of the equilibrium coefficient of mutation and selection. Neutrality plot for pqqA, pqqB, pqqC, pqqD, and pqqE genes (Figure 4) revealed no significant correlation between GC3% and GC12% (R2 = 0.007, 0.368, 0.349, 0.015, and 0.458, respectively), which indicates that some additional major factors, such as selection, can also influence the synonymous codon usage bias in all pqq genes of Cronobacter, Klebsiella, Kosakonia, Pantoea, Rahnella, and Serratia. In the neutrality plot, the average value of GC12% varied from 37.5 to 50% for pqqA, 50.81–64.47% for pqqB, 51.19–62.30% for pqqC, 42.71–60.75% for pqqD, and 48.61–62.13% for pqqE genes, whereas GC3% varied from 39.58 to 50%, 58.22 to 80.26%, 60.71 to 83.73%, 56.25 to 75.26%, and 57.77 to 85.53% for pqqA–pqqE genes, respectively. The linear regression line was not along the diagonal plane, which indicates that the genes were not under mutational pressure. Besides, the slope of the regression line near zero suggested that there was a low level of mutation biases or high-level conservation of GC contents throughout the genome. Neutrality plot analysis revealed that natural selection played a prominent role over mutation pressure in shaping the codon usage bias of chloroplast genes in two species of Pisum, viz. P. fulvum and P. sativum (Bhattacharyya et al., 2019), chloroplyll synthesis pathway-associated genes in monocot and dicot (Begum and Mondal, 2020), and Ginkgo biloba (He et al., 2016). The results of our neutrality plot indicated that natural selection played a pivotal role in determining the selective constraints on codon usage bias in the PQQ biosynthetic pathway-associated genes from selected Gammaproteobacteria.
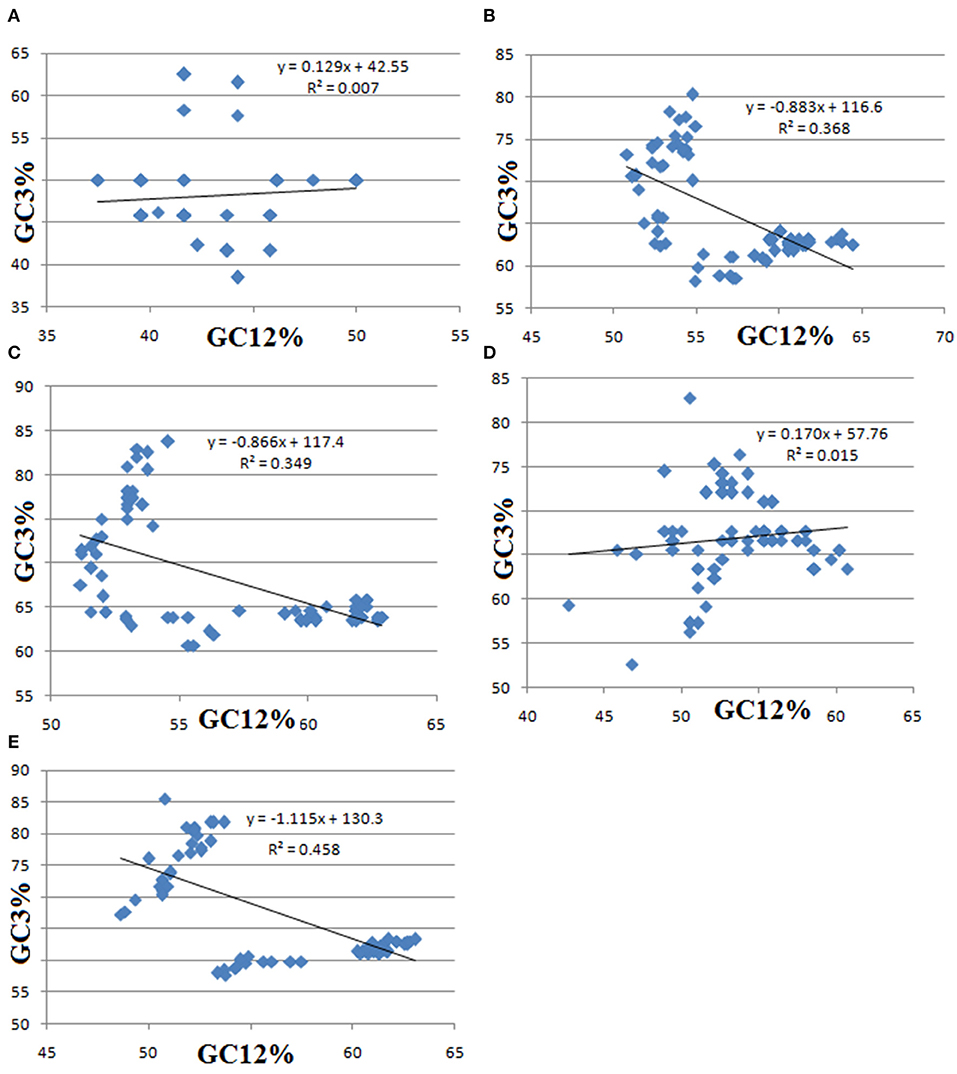
Figure 4. Neutrality plot of GC12% and GC3% contents of pqqA (A), pqqB (B), pqqC (C), pqqD (D), and pqqE (E) genes of Cronobacter, Klebsiella, Kosakonia, Pantoea, Rahnella, and Serratia. In this regression plot, GC12 (y-axis) represents the average value of GC contents at the first and second positions in each codon, and GC3 (x-axis) is the GC content at the third position.
Study of Codon Preference by t-Test
The average and standard deviation of RSCU values of the pqq genes from selected Gammaproteobacteria with preferred codons were furnished in Supplementary Table 4. In Cronobacter, Klebsiella, Kusunoki, Pantoea, Rahnella, and Serratia, significant differences at p < 0.05 among the pqq genes in their codon usage from the t-test have been observed (Table 3). The abovementioned Gammaproteobacteria showed a preference for the amino acids Alanine (A), Glycine (G), Lysine (L), Proline (P), Arginine (R), Serine (S), Threonine (T), and Valine (V). In Glycine, pqqB, pqqC, pqqD, and pqqE genes of Cronobacter have substantiated preferences toward both U3 and C3 except the pqqA gene that appeared to be more biased to U3 over A3. All pqq genes of Cronobacter evinced predilections toward both U3 and G3 in Lysine. pqqC, pqqD, and pqqE genes of Cronobacter were preferably more biased to both A3 and G3 in Proline; therewithal, pqqB gene was more partial to A3 over U3. Interestingly, all pqq genes of Cronobacter, Klebsiella, and Serratia revealed an increased proclivity toward U3 over A3 in Glycine. Likewise, in the case of Lysine, all pqq genes of Cronobacter and Klebsiella were more biased to U3 over A3. All the pqq genes of Klebsiella, Kosakonia, and Pantoea were biased to C3 over G3 in Arginine. Just in the case of Proline, every ppq gene of Klebsiella, Pantoea, and Serratia and pqqB, pqqC, pqqD, and pqqE genes of Cronobacter and Kosakonia exhibit preferences toward A3 over U3. All genes of Kosakonia (except pqqE) and Pantoea are more biased to G3 over C3 in Valine.
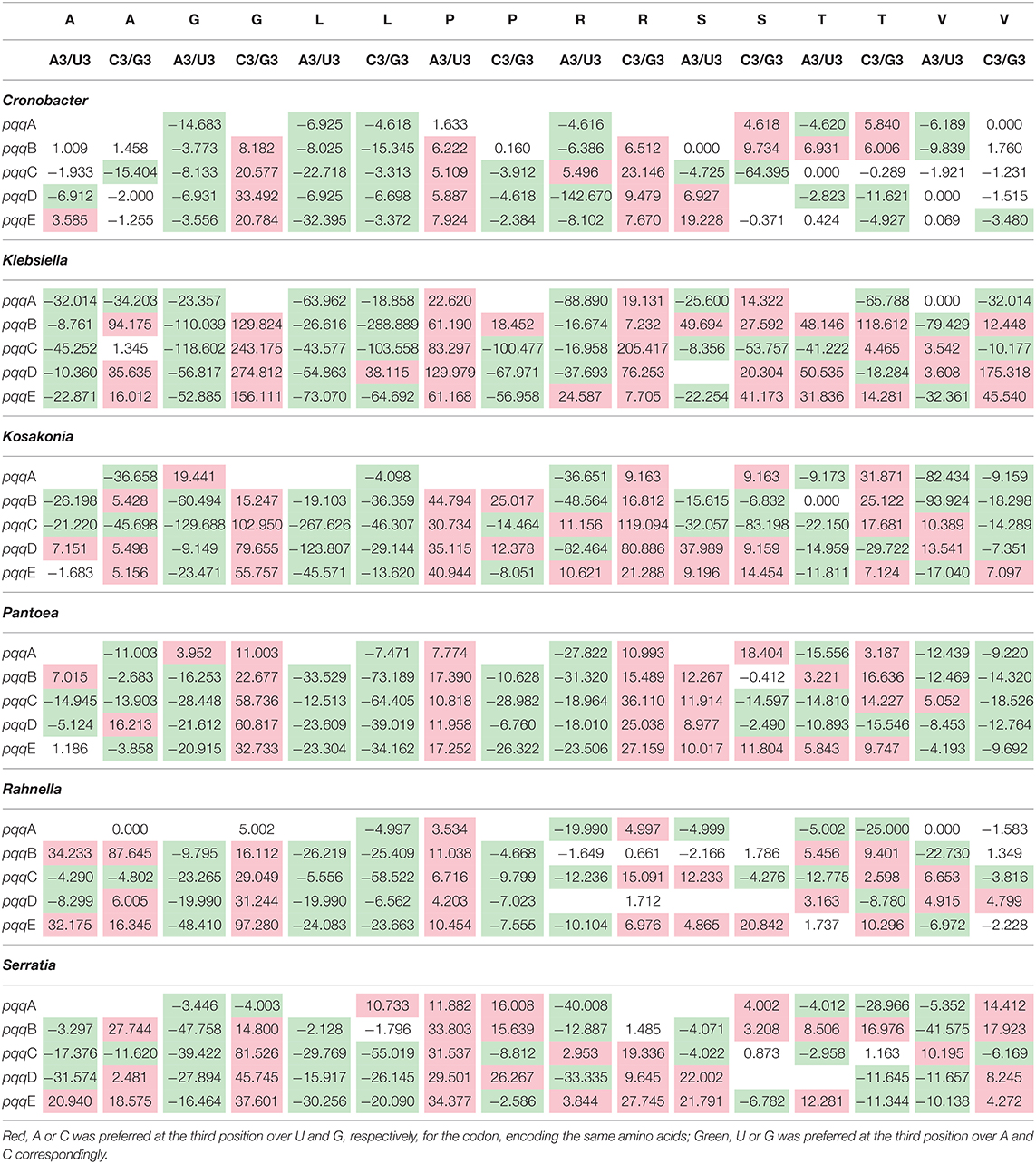
Table 3. T-tests of the RSCU values of synonymous codons for Gammaproteobacteria pqqA, pqqB, pqqC, pqqD, and pqqE genes, at 5% level of significance (p < 0.05).
Correspondence Analysis
The COA, a multivariate statistical method, is used to study the significant trends in codon usage variation (Greenacre, 1984). The COA can describe the distribution of genes and reflects the distribution of their corresponding codons, unveiling potential influences on codon usage bias (Romero et al., 2000; Mondal et al., 2016; Begum and Mondal, 2020).
As shown in Figure 5, the pqqA genes were not separated along the major axis 1 and 2; consequently, other orthogonal axes contributed to codon bias. The pqqA genes from different species of Gammaproteobacteria that were clustered among themselves were Pantoea agglomerans ASB05 and Pantoea ananatis NN08200; Kosakonia cowanii FBS 223, K. cowanii JCM 109, and Kosakonia sp. SMBL-WEM22; Pantoea sp. At-9b and Pantoea sp. SO10; Mixta calida DSM 22759 and Nissabacter sp. SGAir0207. The majority of the pqqB genes were not separated along the major axis 1 and 2 (Figure 5B). A number of genes were present along the major axis 1, such as pqqB genes from Serratia sp. FS14, Enterobacter aerogenes KC, P. dispersa DSM 3289, and Mixta gaviniae DSM 22758. Enthrallingly, some species of Klebsiella, like Klebsiella quasipneumoniae CAV2018, K. pneumoniae subsp. KUH-KPNHVL1, K. pneumoniae LH102-A, K. pneumoniae subsp. RJF293, K. pneumoniae AT CC35657, K. pneumoniae subsp. AR-0096, and Klebsiella variicola FDAARGOS627, have clustered themselves. Klebsiella aerogenes 18-2341, M. calida 5098PV, M. calida DSM 22759, and Rahnella aquatilis strain pRAZF7 remain close to each other in the same way. The closeness of pqqB genes from several strains of Serratia such as Serratia sp. NGAS9, S. marcescens subsp. Db11, S. marcescens SER00094, Serratia sp. SSNIH1, and Serratia sp. FDAARGOS 506 reflects the similarities of their codon usages.
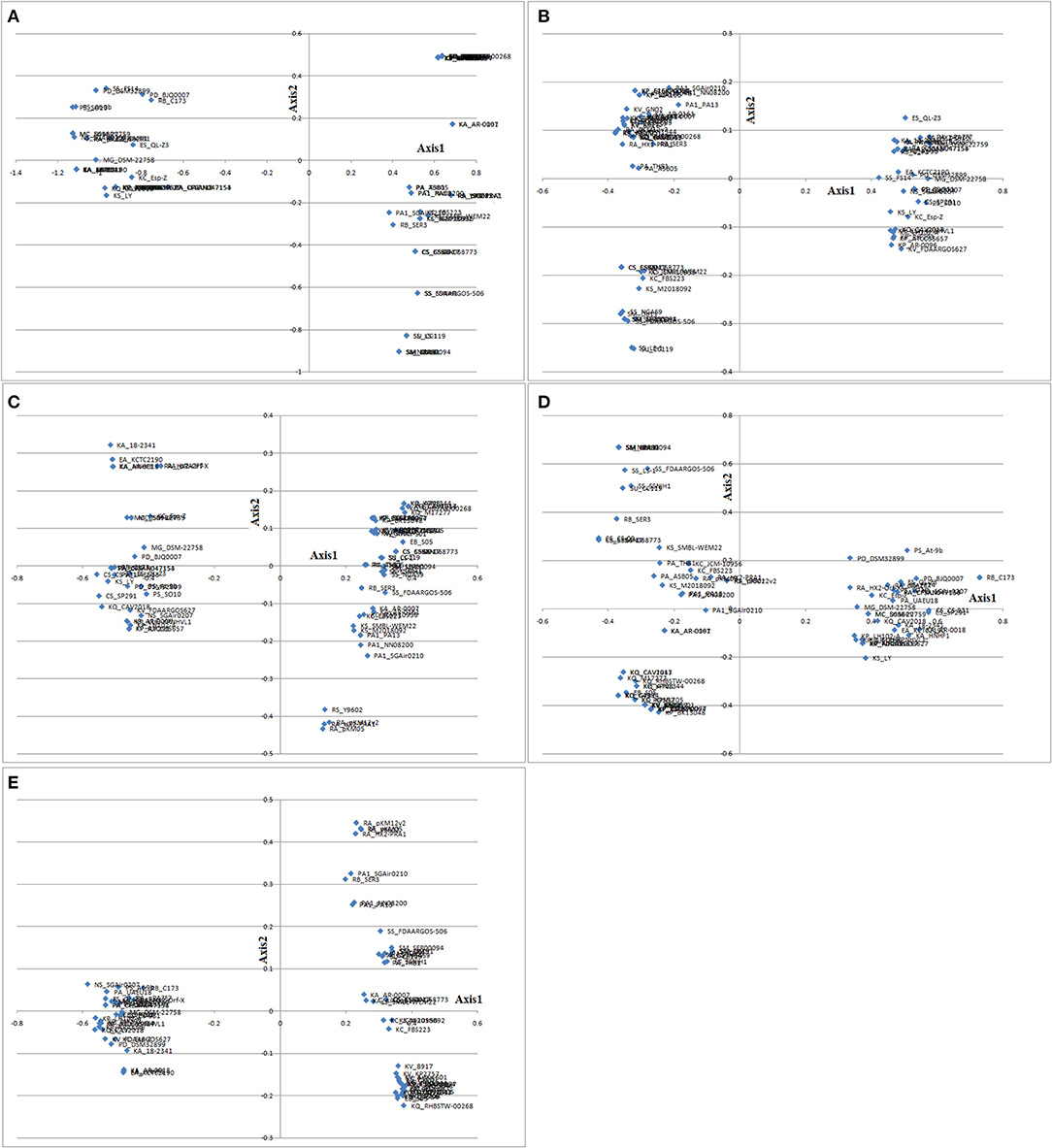
Figure 5. Position of genes along the first and second axes produced by correspondence analysis based on RSCU values of the 59 synonymous codons from pqqA (A), pqqB (B), pqqC (C), pqqD (D), and pqqE (E) genes from 79 Gammaproteobacterial species. x- and y-axis correspond to Axis 1 and Axis 2.
The pqqC genes from some Gammaproteobacterial species present along the positive side of the major axis 1, such as P. agglomerans ASB05, P. agglomerans TH81, S. marcescens SER0094, Serratia sp. LS-1, Serratia ureilytica CC119, Serratia sp. NGAS9, and Serratia sp. SSNIH1, as well as Rouxiella badensis C173, P. agglomerans CFSAN047154, and Serratia sp. FS14 along the negative side of axis 1, prefer to remain close. The closeness of these genes reflects the similarities of their codon usages. Genes from different Gammaproteobacterial species clustered around the major axis 1 may indicate their selection over mutation bias. The pqqC genes from some species of K. pneumoniae, K. quasipneumoniae, and K. variicola, and three strains of R. aquatilis, such as R. aquatilis pKM05, R. aquatilis pKM12v2, and R. aquatilis pKM05, prefer to remain clustered, which indicates a comparatively greater role of selection pressure in codon bias for these genes. The pqqD genes from some Gammaproteobacterial species separated along the major axis 1, such as M. gaviniae DSM 22758, M. calida 5098PV, Cronobacter sakazakii 931, C. sakazakii SP291, and P. ananatis SGAir02. In a similar manner to pqqC genes, some pqqD genes from K. pneumoniae and K. quasipneumoniae prefer to remain close as well. Two bacterial species of Cronobacter, i.e., C. sakazakii CS-09 and C. sakazakii CFSAN068773, and C. sakazakii 931 and C. sakazakii SP291 remain close to each other. The pqqE genes from some Gammaproteobacterial species were not separated along the major axis 1. Genes were not clustered in a particular axis; accordingly, other orthogonal axes contribute to codon bias. Like pqqC genes, some pqqE genes from two Klebsiella species, K. quasipneumoniae and K. variicola, prefer to remain close. Four strains of Rahnella, such as R. aquatilis pKM12v2, R. aquatilis pKM05, and Rahnella sp. Y9602, were close to each other, and two species of Pantoea, such as P. ananatis NN08200 and P. ananatis PA13, were close to each other as well. K. aerogenes AR-0018, K. aerogenes HNHF1, and E. aerogenes KCTC2190 prefer to remain clustered. The closeness of these species reflected the similarities of their codon usages.
COA was performed based on the RSCU values of each gene to analyze the variation of codon usage of the genes (pqqA, pqqB, pqqC, pqqD, and pqqE) from selected Gammaproteobacteria involved in the PQQ biosynthetic pathway (Figure 6). Axis 1 (horizontal axis) represents the main index for affecting codon usage bias.
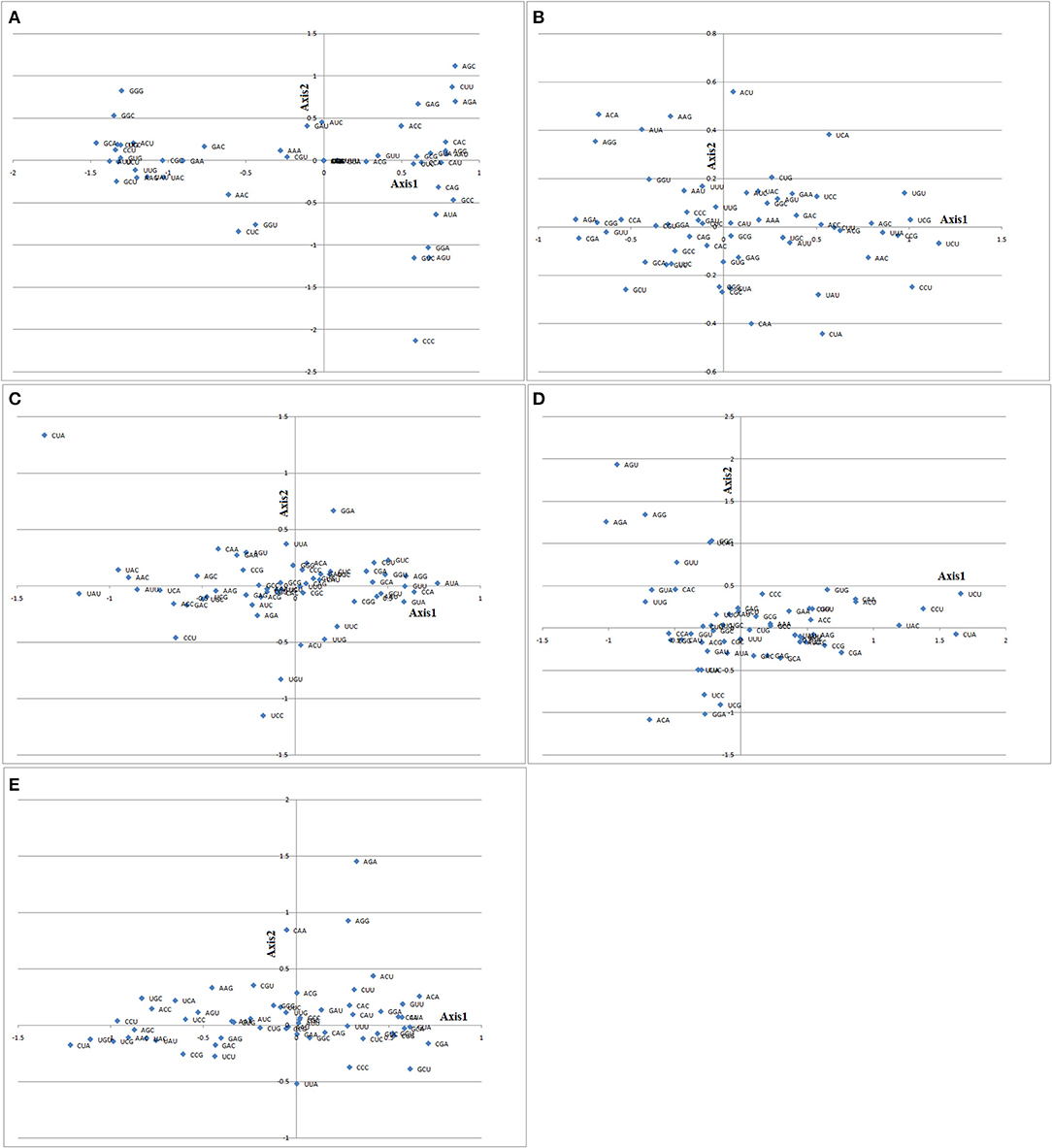
Figure 6. The codon position along the first and second axes produced by correspondence analysis based on RSCU values of the 59 synonymous codons from pqqA (A), pqqB (B), pqqC (C), pqqD (D), and pqqE (E) genes from Cronobacter, Klebsiella, Kosakonia, Pantoea, Rahnella, and Serratia. x- and y-axis correspond to Axis 1 and Axis 2.
In the pqqA gene, some codons were separated along the major axis 1 and others remain scattered. Codons such as UUU (Phe), UUA (Leu), ACG (Thr), GUU (Val), UUC (Ser), GCG (Ala), CCA (Pro), and CAU (Arg) were along axis 1. Both G/C-ending codons and A/U-ending codons were scattered above or below axis 1. It was also observed that some codons prefer to remain close to each other such as UUU (Phe) and UUA (Ala); GCG (Ala), GUA (Val), AAU (Asn), AGG (Arg), UUC (Phe), CCA (Pro), and CAU (His); CUC (Val), UGC (Cys), CCU (Pro), GUG (Val), AUU (Ile), and UCU (Ser); and AAG (Lys) and UAU (Tyr). The preference of codons to remain clustered indicates their selection over mutational bias. In the pqqB, pqqC, pqqD, and pqqE genes, majority of codons remain scattered, and some were separated along major axis 1 and axis 2. In the pqqB gene, codons such as CAU (His), ACC (Thr), CUU (Val), ACG (Thr), and AGC (Ser) were along axis 1 and GUG (Val) and CGC (Arg) were along axis 2. Both G/C-ending codons and A/U-ending codons were preferred. In Serratia, AGC (Ser) was the preferred optimal codon (Table 4). The average RSCU value of the pqqB gene from Serratia AGC (Ser) codon was 4.334 (2.646) (Supplementary Table 4). PQQ biosynthetic genes pqqB and pqqC from selected Gammaproteobacteria prefer to remain scattered, specifying that mutational pressure had played a comparatively prodigious role in codon bias in these genes. In the pqqC gene, codons such as UUU (Phe), GCA (Ala), GUU (Val), and AUA (Ile) were along axis 1 and GGG (Gly) and GCG (Ala) were along axis 2. The average RSCU value was <1 for the abovementioned codons in Klebsiella, Kosakonia, Pantoea, Rahnella, and Serratia except for GCG (Ala) whose average RSCU value was 2.114 (0.235) in Cronobacter. In the pqqC gene from Kosakonia, GCC (Ala), whose average RSCU value was 2.154 (0.666), was the preferred optimal codon (Table 4). In the pqqD gene, some codons CUG (Lys), AAA (Lys), GCC (Ala), and UAC (Tyr) were along the positive side of axis 1; GUC (Val), UGC (Cys), and GGC (Gly) were along the negative side of axis 1 and CAG (Gln), GCU (Ala), and CGC (Arg) were along axis 2. The average RSCU value of these codons was >1 except for GGC and GCU. The clustered codons in pqqD genes were AAA (Lys) and GCC (Ala); UCU (Ser) and UUC (Phe); AAG (Val), UAU (Tyr), AUA (Ile), UAC (Tyr), CCG (Pro), and AAC (Asn); CAA (Gln) and ACU (Asn); CAG (Gln) and GCU (Ala); and CGG (Arg) and UUU (Phe), which signifies their selection over mutational bias. The closeness of these codons in the pqqD gene reflected the similarities of their codon usages. Similarly, in the pqqE gene, a few codons such as AAU (Asn), UUU (Lys), GUA (Lys), UCC (Ser), and CCU (Pro) were along axis 1 and ACG (Thr), GCG (Ala), and UUA (Lys) were along axis 2. The average RSCU value of some codons UUU, CCU, and UUA was <1. The clustered codons in pqqE genes were CCC (Pro), CGC (Arg), AUU (Ile), and AAU (Asn); CCU (Pro) and AAA (Lys); and AAA (Lys) and GUG (Val), which evidenced their selection over mutational bias. In the pqqE gene from Pantoea, GUG (Val), whose average RSCU value was 1.821 (1.137), was the preferred optimal codon (Table 4).
ENc Plot Analysis
The ENc is an important index to measure the codon usage bias within a genome and plays a crucial role in their codon usage profile. ENc or Nc was plotted against the GC3s for pqqB, pqqC, pqqD, and pqqE genes from Klebsiella, Kosakonia, Pantoea, Rahnella, and Serratia (Figure 7). pqqB, pqqC, pqqD, and pqqE genes from selected groups of microorganisms were located below the expected curve as per Figure 7. These results indicate that translational selection was involved in determining the selective constraints on codon bias in pqqB, pqqC, pqqD, and pqqE genes. Only a small number of pqqD genes from Gammaproteobacteria were slightly near the expected curve, and only one has been observed absolutely on the expectation curve. Microorganisms that are positioned on or close to the curve line were considered to be under mutational pressure. In the present study, the Nc values of the pqqC gene varied from 31.2 (M. gaviniae DSM and Serratia sp. FS14) to 46.73 (K. pneumoniae C16 and K. pneumoniae F16). The Nc values of the pqqD gene varied from 29.65 (Serratia sp. FS14) to 53.16 (Serratia Db11, Serratia SER00094, and Serratia UMH1). The Nc value of the Cronobacter 931 pqqD gene was 31.67. The Nc values of the pqqE gene varied from 32.84 (C. sakazakii SP2) to 48.33 (P. ananatis NN08200). For C. sakazakii CS, Nissabacter sp. SGAir0207, K. pneumoniae LH, and K. pneumoniae sub-pqqE gene, the respective Nc values were 33.6, 33.81, 34.8, and 34.87. The lowest value indicated a high degree of codon bias resulting from a low number of codons preferentially used for amino acids. The calculated Nc values of the rest of the genes from Gammaproteobacteria were observed to be >35, suggesting a weak codon bias.
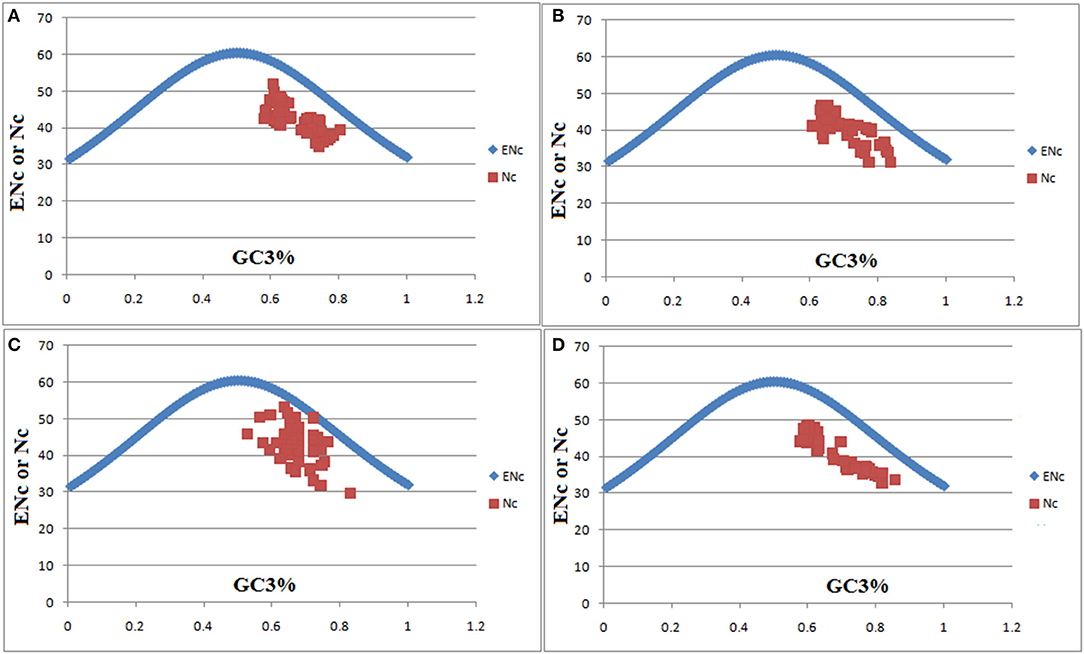
Figure 7. ENc plot analyses (ENc or Nc plotted against GC3) of pqq genes; pqqB (A), pqqC (B), pqqD (C), and pqqE (D) from Klebsiella, Kosakonia, Pantoea, Rahnella, and Serratia. ENc represents the effective number of codons, and GC3 is the GC content of synonymous codons at the third position. In the plot, the ENc from GC3 were shown as a bell-shaped bisymmetric curve. The solid line represents the expected curve when codon usage bias is only affected by mutational pressure. Nc was obtained from Codon W 1.4.4 (http://codonw.sourceforge.net/, Pedan, 2000). Due to the short length of the pqqA gene (23–25 amino acids), numerous codons were absent and, in consequence, Nc values were not calculated through CodonW.
Previous studies reported that few nif (nitrogen fixation) genes such as nifK, nifD, and nifH from Firmicutes, Euryarchaeota, Proteobacteria alpha and delta (Mondal et al., 2016) and rbcL (ribulose-1, 5-bisphosphate carboxylase oxygenase) gene in three prokaryotic families such as archaea, cyanobacteria, and proteobacteria (Mondal et al., 2013) were found under mutational pressure. ENc analysis suggested that mutational pressure and selection constraint were the main factors for shaping codon usage in Chlorophyll (Chl) synthesis and degradation pathway-associated genes in monocots and dicots (Begum and Mondal, 2020). The results of our ENc plot indicated that natural selection played a very important and dominant role in determining the selective constraints on codon bias in pqqB, pqqC, pqqD, and pqqE genes from Klebsiella, Kosakonia, Pantoea, Rahnella, and Serratia. Begum and Mondal (2020) also reported that the majority of the Chl synthesis and degradation pathway-associated genes in monocots and dicots preferred weak codon bias. Moreover, Rahman et al. (2018) also found relatively low codon usage bias and translation selection factor shaping codon usage pattern in Crimean-Congo hemorrhagic fever virus (CCHFV). Natural consortium of five acidophilic bacteria used for biomining preferentially has low codon usage bias (Hart et al., 2018). Likewise, in our study, Gammaproteobacteria pqqB, pqqC, and pqqE genes including a mass of pqqD genes from all selected groups of microorganisms preferred weak codon bias. A lack of strong codon usage bias is expected to promote efficient usage of more codons and thereby speed up the translation process (Jenkins and Holmes, 2003). It has been previously reported that stronger selection provides high translational accuracy to minimize the missense and nonsense errors (Stoletzki and Eyre-Walker, 2007; Hershberg and Petrov, 2008) and accelerates the translation elongation in protein expression (Ran et al., 2014), which is advantageous for genome stability in species evolution.
Identification of Optimal Codons
COA based on the RSCU values of the codons for every organism from pqq genes involved in the PQQ biosynthetic pathway exhibits optimal codons. Frequently used codons are known to be optimal codons, while less frequently used codons are non-optimal codons. The codon usage bias ensures that the optimal codons can pair with the anticodons of the most abundant tRNA genes and avoids the misincorporation of amino acids to reduce processing errors. We have identified a total of 55 optimal codons (three-letter code for encoding amino acids) that encode 18 amino acids in pqqA–pqqE genes (Table 4), among them, 6 are for pqqA, 17 for pqqB, 9 for both pqqC and pqqD, and 14 optimal codons for pqqE genes. Optimal codons ending with A/U and G/C in pqqA, pqqB, and pqqD signify that codon usage in selected organisms is biased to A/U- and G/C-ending synonymous codons. Contrastingly, in the pqqC gene, optimal codons end with A/U and C, suggesting that codon usage is biased to A/U and C-ending synonymous codons. Whereas, the pqqE gene possesses 13 optimal codons with G/C ending, and only 1 optimal codon ended with A/U (CGU). Optimal codons identified in our study might provide useful information for genetic engineering, gene prediction, and molecular evolution studies.
Gene Organization Study
The organization of the pqq genes from selected microorganisms in the PQQ operon was studied and deduced that the organization of pqqA–pqqE genes in PQQ operon from some Gammaproteobacteria was in the positive strand and some in the negative strand (Figure 8). Figure 8 revealed that the pqqD and pqqE genes from different strains of Cronobacter, Erwinia, Enterobacter, Klebsiella, Kosakonia, Mixta, Nissabacter, Pantoea, Rahnella, Rouxiella, and Serratia consistently overlapped. It has been observed that pqqB and pqqC genes from Enterobacteriaceae bacterium, K. aerogenes, K. pneumoniae, K. quasipneumoniae, Klebsiella quasivariicola, K. variicola, and P. dispersa were overlapped as well. Furthermore, there was a single character overlap in the pqqC gene from C. sakazakii, K. cowanii, M. calida, M. gaviniae, S. marcescens, Serratia sp., and S. ureilytica. PQQ biosynthetic pathway-associated genes have been extensively studied in several bacteria (Guo et al., 2009). They have reported that in R. aquatilis, pqqABCDEF might be regulated as an operon in which the pqqD and pqqE genes were fused. The relative order of pqqA to pqqF was identical for all four bacterial species such as Enterobacter intermedium 60-2G, K. pneumoniae NCTC418, A. calcoaceticus, and G. oxydans ATCC 9937, except that pqqF in P. fluorescens B16 existed in a different location (Guo et al., 2009). In P. fluorescens B16, a plant growth-promoting rhizobacterium, the genes pqqABCDEFHIJM are organized as an operon. However, genetic variation has been identified between different bacteria (Choi et al., 2008). Genetic organization of the pqqC gene in PQQ operon in Pseudomonas kilonensis JX22 was pqqF, pqqA, pqqB, pqqC, pqqD, pqqE, and pqqG, respectively (Xu et al., 2014). The seven genes have the same transcriptional orientation (Xu et al., 2014). The pqq operon gene order was pqqFABCDE in endophytic Pseudomonas (Oteino et al., 2015).
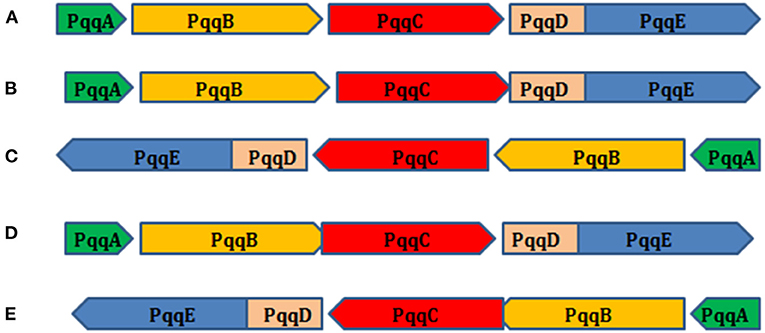
Figure 8. Gene organization of the pqqA (A), pqqB (B), pqqC (C), pqqD (D), and pqqE (E) genes in the PQQ operon of selected Gammaproteobacteria. The right arrow indicates a positive strand, and the left arrow indicates a negative strand.
Study of Codon Preference Among the pqq Genes in the Positive and Negative Strand by Z-Test
Among 79 selected Gammaproteobacteria, 31 organisms were in the positive strand and 48 organisms were in the negative strand of the pqq genes. In the PQQ biosynthetic pathway, significant differences at p < 0.05 among the positive and negative strand of the pqq genes in their codon usage were observed according to the Z test (Supplementary Table 5). The pqq genes in the positive strand showed significantly high (p < 0.05) biasness in CUG (Leu), AAC (Asn), CCU (Pro), and GUG (Val). pqqA, pqqB, pqqC, and pqqD genes in the positive strand showed significantly high (p < 0.05) preferences toward AUU (Ile). pqqB, pqqC, pqqD, and pqqE genes in the positive strand showed significantly high (p < 0.05) biasness in CCU (Pro), CGU (Arg), ACC (Thr), and GUG (Val). pqqA, pqqB, pqqC, and pqqE genes in the positive strand showed significantly high (p < 0.05) preferences toward GGC (Gly) and CGC (Arg). pqqB, pqqC, and pqqE genes in the positive strand showed significantly high (p < 0.05) biasness in GCG (Ala), but pqqC gene in the positive strand also showed significantly high (p < 0.05) biasness in GCC (Ala). pqqC and pqqE genes in the positive strand showed significantly high (p < 0.05) biasness in AUC (Ile) and UAU (Tyr). pqqD and pqqE genes in the positive strand showed significantly high (p < 0.05) biasness in AAG (Lys) and AGC (Ser).
The pqq genes in the negative strand of the pqq genes showed significantly high (p < 0.05) biasness in AAU (Asn) and CCA (Pro). pqqB, pqqC, pqqD, and pqqE genes in the negative strand showed significantly high biasness (p < 0.05) toward GAU (Asp), AUA (Ile), CGG (Arg), and GUU (Val). pqqA, pqqB, pqqC, and pqqD genes in the negative strand showed significantly high (p < 0.05) biasness in (Arg). pqqB, pqqC, and pqqE genes in the negative strand showed significantly high (p < 0.05) biasness in GCA (Ala), GCU (Ala), GGU (Gly), and CUC (Leu). pqqA, pqqC, pqqD, and pqqE genes in the negative strand showed significantly high biasness (p < 0.05) toward GUA (Val).
Compositional Analysis of Amino Acid Frequencies of pqq Genes From Selected Gammaproteobacteria
There are 20 amino acids, but for a better understanding of their effect on different genes and organisms, the 20 amino acids had been classified into five groups based on their polarity and charge, i.e., Acidic polar (D and E), Basic polar (H, K, and R), Aromatic (F, Y, and W), Neutral non-polar (P, C, M, G, A, V, I, and L), and Neutral polar (Q, N, S, and T).
Average and standard deviation data of amino acid frequencies (from pqqA, pqqB, pqqC, pqqD, and pqqE gene-encoded proteins) in Cronobacter, Klebsiella, Kosakonia, Pantoea, Rahnella, and Serratia are mentioned in Table 5. From Table 5 and Supplementary Table 6, it can be concluded that the frequency of acidic polar amino acid E (Glu) was comparatively high in pqqC, pqqD, and pqqE genes. The frequency of E was high in the pqqA gene of Serratia and Rahnella but quite low in the case of the pqqD gene of Cronobacter in respect to other Gammaproteobacteria. The aromatic amino acid W (Trp) was immensely less in frequency in each of the genes except pqqA and pqqC. Identically, the frequency of neutral non-polar amino acid M (Met) was also inadequate in all genes except pqqA. Furthermore, the frequency of neutral non-polar amino acid A (Ala) was high in pqqB, pqqC, pqqD, and pqqE genes and highest in the pqqD gene. Overall, the frequency of neutral non-polar amino acid C was very low (absent in pqqA), and the frequency of neutral polar S (Ser) was high in all pqq genes obtained from Gammaproteobacteria (except pqqD with lower S). Despite the frequency of neutral non-polar amino acid L (Leu) being miraculously high in every gene, the highest level of frequency has been found in the pqqA gene. In Klebsiella, the amount of the neutral polar amino acid T (Thr) was exceptionally low in comparison to other Gammaprotobacteria. Interestingly, in the pqqA gene, H (His), C (Cys), and Q (Gln) were absent. The elevated frequency of L (Leu) was biased for pqqA, pqqB, pqqC, pqqD, and pqqE genes. A (Ala) was also considerably biased for pqqB, pqqC, pqqD, and pqqE genes. Cysteine residues are capable of the formation of disulfide bonds, which plays an essential role in the stability and folding of the protein structure. The very low amounts of cysteine residues indicated that the protein gains its stability from other interactions as chances of disulfide bond formation are very low.
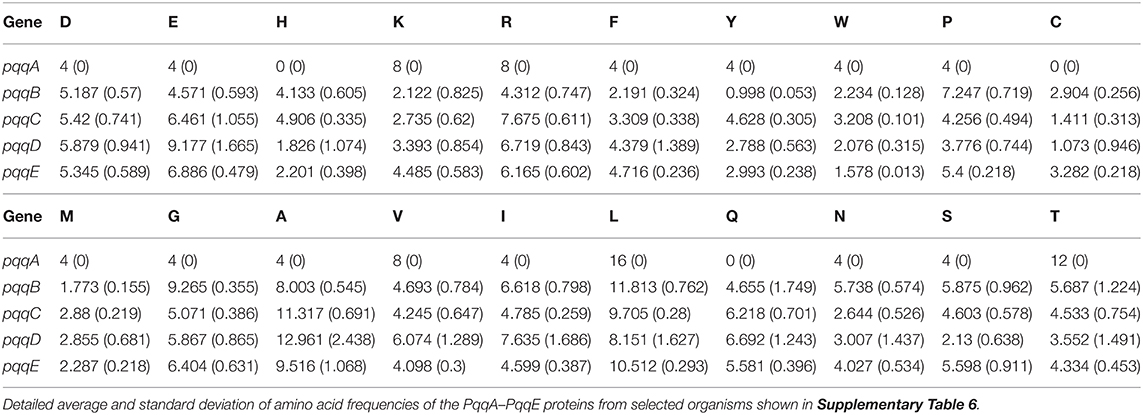
Table 5. Average and standard deviation of amino acid frequencies of the PQQ pathway-associated genes from selected Gammaproteobacteria.
Estimation of GRAVY and AROMO
A higher GRAVY score of proteins represents the physicochemical properties of the integral membrane-bound characteristics, while a negative GRAVY score suggests the soluble nature of the protein (Kyte and Doolittle, 1982). In our study, the GRAVY scores of the protein sequences obtained from all the pqq genes involved in the PQQ biosynthetic pathway from selected Gammaproteobacteria except pqqC and pqqD gene of Serratia rendered a negative value, evincing the soluble nature (hydrophilic) of these proteins (Table 6).
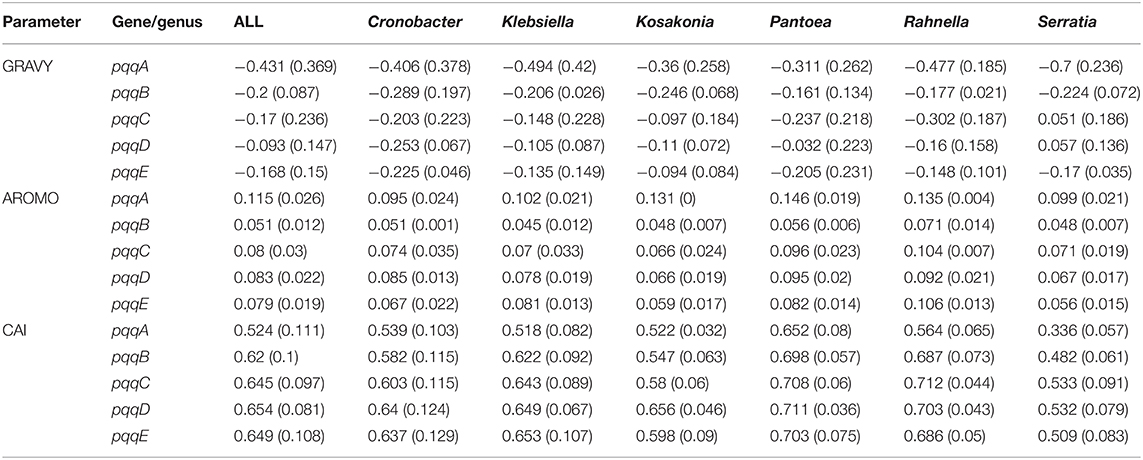
Table 6. Average and standard deviation of GRAVY, AROMO, and CAI of the pqqA, pqqB, pqqC, pqqD, and pqqE genes from Cronobacter, Klebsiella, Kosakonia, Pantoea, Rahnella, and Serratia.
From the aromaticity score, we have found that the average AROMO value of the pqqA gene from selected Gammaproteobacteria was about 0.1, for pqqB, pqqC, pqqD, and pqqE genes that varied from 0.045 to 0.071, 0.066 to 0.095, 0.066 to 0.0092, and 0.056 to 0.106 in succession. From the amino acid frequency table (Table 5), it was observed that the average frequency of Phenylalanine (Phe) of the pqqB gene from Cronobacter, Kosakonia, Rahnella, and Serratia were 1.98 and for Klebsiella and Pantoea were 2.65 and 2.56, respectively. Similarly, the average Tyrosine (Tyr) frequency from Cronobacter, Kosakonia, Rahnella, and Serratia were 0.99. The overall frequency of Tryptophan (Trp) was 2.3 for pqqB genes. On the whole, the frequency of Phe, Tyr, and Trp was far better in other pqq genes than pqqB. In pqqD (5.1) and pqqE (4.64), the frequency of Phe seems much superior, whereas the average frequency of Trp of pqqE genes from all selected Gammaproteobacteria was low (1.57), and Phe frequency of pqqD from Rahnella was comparatively much higher (7.51). It was observed that Tyr frequency was extremely low in the pqqB gene from Cronobacter, Kosakonia, Rahnella, and Serratia in respect to other pqq genes.
Gene Adoptability Analysis
CAI is a ratio of the synonymous codon bias with a value that ranges from 0 to 1 of a gene to a highly expressed reference gene. An adequately higher CAI value indicates a strong bias of synonymous codon usage and a moderately high gene expression with potential gene adaptability (Sharp and Li, 1987; Gupta et al., 2004). To predict the probability of the degree of expression of the five pqq genes (pqqA, pqqB, pqqC, pqqD, and pqqE) among the 79 Gammaproteobacteria CAI was analyzed. In the case of pqqA, pqqB, and pqqE genes, Serratia holds the lowest values 0.336, 0.482, and 0.509, respectively, while the highest values were obtained from Pantoea, 0.652, 0.698, and 0.703, respectively (Table 6). The CAI value of the pqqB gene from Rahnella was about 0.687, with an average value of 0.6 for others. Likewise, the lowest CAI values of the pqqC and pqqD genes were found in Serratia (0.533 and 0.532), whereas the highest values were occupied by Pantoea (0.708 and 0.711) and Rahnella (0.712 and 0.703). All other Gammaproteobacteria of pqqC and pqqD genes possess a CAI value of nearly 0.6 and 0.65, respectively. The CAI values in pqqC, pqqD, and pqqE genes from Pantoea and Rahnella were virtually alike. In contrast, the average CAI of the pqqB, pqqC, pqqD, and pqqE genes from different species of Gammaproteobacteria except for Serratia indicated a similar pattern with a comparatively high level of gene expression, reinforcing the verity of gene adaptability. The results demonstrate that gene expression level shaped codon usage in Klebsiella, Cronobacter, Kosakonia, Pantoea, and Rahnella, and the pqq genes with higher expression levels had a greater degree of codon usage bias and richer GC. Several studies on codon usage have revealed that natural selection on codon usage increases both translation accuracy and efficiency, which have long been known to affect gene sequences. Such selection is stronger on highly expressed genes, resulting in higher levels of codon bias within genes with higher expression levels (Gouy and Gautier, 1982; Duret, 2000; Hershberg and Petrov, 2008). Selection on translation accuracy affects more strongly codons encoding conserved amino acids, since these will more often affect protein folding and/or function (Yannai et al., 2018). Yannai et al. (2018) demonstrated that selection on translation accuracy affects both lowly and highly expressed genes in E. coli.
Conclusions
In this article, an in silico approach was used to investigate the molecular evolution of the genes involved in the PQQ biosynthetic pathway among different species of Gammaproteobacteria. All the selected genes were present in an operon system maintaining pqqA–pqqE order either in the positive or in the negative strand. Phylogenetic analyses based on individual sequences of PqqA–PqqE proteins involved in the PQQ biosynthetic pathway depicted all the selected species getting clustered prominently according to their genera, such as Pantoea, Rouxiella, Rahnella, Kosakonia, Mixta, Cronobacter, and Serratia, suggesting their phylogenetic closeness. Moreover, all the phylogenetic trees, including 16S rRNA-based phylogeny, revealed a relatively close association of Klebsiella and Enterobacter; both of them belong to Enterobacteriaceae family. In this study, some of the sites within the MSA of those proteins that were not occupied by the identical character have been recognized to correlate the evolutionary relationship for a better understanding of the mutation prone sites within the sequences.
GC1% and GC3% in pqqB, pqqC, pqqD, and pqqE genes were higher in the selected Gammaproteobacteria, revealing that these genes were GC-rich, whereas the average of GC1%, GC2%, and GC3% in the pqqA gene was comparatively low, revealing that the pqqA gene was AT-rich. The GC% at the third codon position was highest in all pqq genes of Cronobacter, which might be due to better adaptation to the environment. The content of GCs at different positions of the codons has also been properly reflected in RSCU values of the codons with few exceptions. Moreover, there is significant difference in their codon usage preference according to the position of the pqq genes; mostly G/C-ending codons have the preference for the genes in the positive strand and A/U-ending codons have the preference in the negative strand.
Phe for pqqD from Rahnella was higher than other Gammaproteobacteria. Tyr frequency was very low in the pqqB gene from Cronobacter, Kosakonia, Rahnella, and Serratia in respect to other pqq genes. The CAI of the pqqB, pqqC, pqqD, and pqqE genes from the selected species indicated high adaptability except for Serratia. The COA based on RSCU optimal codons in the pqqB gene ending with A/U are higher in number than those in the pqqE gene ending with G/C (except CGU). These optimal codons might provide significant information for gene expression prediction. The COAs suggested the mutational pressure on the pqq genes from selected organisms to shape the codon usage pattern; however, ENc and neutrality plot indicated natural selection. Besides, the pqq genes prefer weak codon bias. These systematic and comprehensive computational studies might be useful for the identification and characterization of the genes responsible for phosphate solubilization in other organisms.
To recognize the interface residues, the structural analysis of PQQ followed by molecular docking analysis with glucose dehydrogenase will be performed in the future. The variation of the interface residues and their efficiency for binding with glucose dehydrogenase as well as the production of gluconic acid will be quantified. PQQ, acquired from various genera of microorganisms with appropriate composition and better efficiency, might be competently recognized from the above methodical study.
Data Availability Statement
The datasets presented in this study can be found in online repositories. The names of the repository/repositories and accession number(s) can be found in the article/Supplementary Materials.
Author Contributions
EB and SM conceived the study and designed and performed the experiment. YB, EB, RD, and SM analyzed the results. YB, RD, and EB wrote the manuscript. All the authors read, edited, and approved the manuscript.
Conflict of Interest
The authors declare that the research was conducted in the absence of any commercial or financial relationships that could be construed as a potential conflict of interest.
Acknowledgments
EB, RD, and SM are thankful to the Department of Biotechnology, the University of Burdwan, for the computational facilities. YB gratefully acknowledges Department of Biophysics, Molecular Biology and Bioinformatics, University of Calcutta, and also Centre of Excellence in Systems Biology and Biomedical Engineering (TEQIP Phase-III), University of Calcutta, JD-2, Sector III, Salt Lake, Kolkata-700106, West Bengal, India, for infrastructural support.
Supplementary Material
The Supplementary Material for this article can be found online at: https://www.frontiersin.org/articles/10.3389/fagro.2021.667339/full#supplementary-material
Abbreviations
PSM, phosphate solubilizing microbes; PQQ, pyrroloquinoline quinine; RSCU, relative synonymous codon usage; COA, correspondence analysis; ENc, expected effective number of codons; MSA, multiple sequence alignment; CAI, Codon Adaptation Index.
References
Alaylar, B., Güllüce, M., Karaday,ý, G., and Karadayý, M. (2018). Isolation of PGPR strains with phosphate solubilizing activity from Erzurum and their molecular evaluation by using newly designed specific primer for pqqB gene. Int. J. Sci. Eng. Res. 9, 103–106.
Alaylar, B., Güllüce, M., Karadayi, M., and Isaoglu, M. (2019). Rapid detection of phosphate solubilizing bacteria from agricultural areas in Erzurum. Curr. Microbiol. 76, 804–809. doi: 10.1007/s00284-019-01688-7
Alayler, B., Egamberdieva, D., Gulluce, M., Karadayi, M., and Arora, N. K. (2020). Integration of moleculartools in microbial phosphate solubilization research in agriculture perspective. World J. Microbiol. Biotechnol. 36, 1–12. doi: 10.1007/s11274-020-02870-x
Alori, E. T., Glick, B. R., and Babalola, O. O. (2017). Microbial phosphorus solubilization and its potential for use in sustainable agriculture. Front. Microbiol. 8:971. doi: 10.3389/fmicb.2017.00971
Altschul, S. F., Madden, T. L., Schäffer, A. A., Zhang, J., Zhang, Z., Miller, W., et al. (1997). Gapped BLAST and PSI-BLAST: a new generation of protein database search programs. Nucleic Acids Res. 25, 3389–3402. doi: 10.1093/nar/25.17.3389
An, R., and Moe, L. A. (2016). Regulation of pyrroloquinoline quinone-dependent glucose dehydrogenase activity in the model rhizosphere-dwelling bacterium Pseudomonas putida KT2440. Appl. Environ. Microbiol. 82, 4955–4964. doi: 10.1128/AEM.00813-16
Angellotti, M. C., Bhuiyan, S., Chen, G., and Wan, X. F. (2007). CodonO: codon usage bias analysis within and across genomes. Nucleic Acid Res. 35, 132–136. doi: 10.1093/nar/gkm392
Anthony, C. (2001). Pyrroloquinoline quinone (PQQ) and quinoprotein enzymes. Antioxid Redox Signal. 3, 757–774. doi: 10.1089/15230860152664966
Arora, N. K., (ed.). (2015). Plant Microbes Symbiosis: Applied Facets. New Delhi: Springer, 381. doi: 10.1007/978-81-322-2068-8
Backer, R., Rokem, J. S., Ilangumaran, G., Lamont, J., Praslickova, D., Ricci, E., et al. (2018). Plant growth-promoting rhizobacteria: context, mechanisms of action, and roadmap to commercialization of biostimulants for sustainable agriculture. Front. Plant Sci. 9:1473. doi: 10.3389/fpls.2018.01473
Barr, I., Latham, J. A., Iavarone, A. T., Chantarojsiri, T., Hwang, J. D., and Klinman, J. P. (2016). Demonstration that the Radical S-Adenosylmethionine (SAM) enzyme PqqE catalyzes de novo carbon-carbon cross-linking within a peptide substrate PqqA in the presence of the peptide chaperone PqqD. J. Biol. Chem. 291, 8877–8884. doi: 10.1074/jbc.C115.699918
Begum, Y., and Mondal, S. K. (2020). Comprehensive study of the genes involved in chlorophyll synthesis and degradation pathways in some monocot and dicot plant species. J. Biomol. Struct. Dyn. 39, 1–28. doi: 10.1080/07391102.2020.1748717
Bhattacharyya, C., Roy, R., Tribedi, P., Ghosh, A., and Ghosh, A. (2020). “Biofertilizers as substitute to commercial agrochemicals,” in Agrochemicals Detection, Treatment and Remediation, ed M. N. V. Prasad (Hyderabad: Butterworth-Heinemann), 263–290. doi: 10.1016/C2018-0-02947-3
Bhattacharyya, D., Uddin, A., Das, S., and Chakraborty, S. (2019). Mutation pressure and natural selection on codon usage in chloroplast genes of two species in Pisum L. (Fabaceae: Faboideae). Mitochondrial DNA. Part A DNA Mapp Seq Anal. 30, 664–673. doi: 10.1080/24701394.2019.1616701
Billah, M., Khan, M., Bano, A., Hassan, T. U., Munir, A., and Gurmani, A. R. (2019). Phosphorus and phosphate solubilizing bacteria: keys for sustainable agriculture. Geomicrobiol. J. 36, 904–916. doi: 10.1080/01490451.2019.1654043
Biville, F., Turlin, E., and Gasser, F. (1991). Mutants of Escherichia coli producing pyrroloquinoline quinone. J. Gen. Microbiol. 137, 1775–1782. doi: 10.1099/00221287-137-8-1775
Bohlin, J., Eldholm, V., Pettersson, J. H., Brynildsrud, O., and Snipen, L. (2017). The nucleotide composition of microbial genomes indicates differential patterns of selection on core and accessory genomes. BMC Genomics 18:151. doi: 10.1186/s12864-017-3543-7
Botzman, M., and Margalit, H. (2011). Variation in global codon usage bias among prokaryotic organisms is associated with their lifestyles. Genome Biol. 12:R109. doi: 10.1186/gb-2011-12-10-r109
Broderick, J. B., Duffus, B. R., Duschene, K. S., and Shepard, E. M. (2014). Radical S-adenosylmethionine enzymes. Chem. Rev. 114, 4229–4317. doi: 10.1021/cr4004709
Bulmer, M. (1991). The selection-mutation-drift theory of synonymous codon usage. Genetics 129, 897–907. doi: 10.1093/genetics/129.3.897
Chistoserdova, L., Chen, S. W., Lapidus, A., and Lidstrom, M. E. (2003). Methylotropy in Methylobacterium extorquens AM1 from a genomic point of view. J. Bacteriol. 185, 2980–2987. doi: 10.1128/JB.185.10.2980-2987.2003
Choi, O., Kim, J., Kim, J. G., Jeong, Y., Moon, J. S., Park, C. S., et al. (2008). Pyrroloquinoline quinone is a plant growth promotion factor produced by Pseudomonas fluorescens B16. Plant Physiol. 146, 657–668. doi: 10.1104/pp.107.112748
Crooks, G. E., Hon, G., Chandonia, J. M., and Brenner, S. E. (2004). WebLogo: a sequence logo generator. Genome Res. 14, 1188–1190. doi: 10.1101/gr.849004
D'Onofrio, G., Ghosh, T. C., and Bernardi, G. (2002). The base composition of the genes is correlated with the secondary structures of the encoded proteins. Gene 300, 179–187. doi: 10.1016/S0378-1119(02)01045-4
D'Onofrio, G., Ghosh, T. C., and Saccone, S. (2007). Different functional classes of genes are characterized by different compositional properties. FEBS Lett. 581, 5819–5824. doi: 10.1016/j.febslet.2007.11.052
Duine, J. A. (1999). The PQQ story. J. Biosci. Bioeng. 88, 231–236. doi: 10.1016/S1389-1723(00)80002-X
Duret, L. (2000). tRNA gene number and codon usage in the C. elegans genome are co-adapted for optimal translation of highly expressed genes. Trends Genet. 16, 287–289. doi: 10.1016/S0168-9525(00)02041-2
Duret, L., and Mouchiroud, D. (1999). Expression pattern and, surprisingly, gene length shape codon usage in Caenorhabditis, Drosophila, and Arabidopsis. Proc. Natl. Acad. Sci. U.S.A. 96, 4482–4487. doi: 10.1073/pnas.96.8.4482
Ewens, W. J., and Grant, G. R. (2001). Statistical Methods in Bioinformatics. New York, NY: Springer.
Felder, M., Gupta, A., Verma, V., Kumar, A., Qazi, G. N., and Cullum, J. (2000). The pyrroloquinoline quinone synthesis genes of Gluconobacter oxydans. FEMS Microbiol. Lett. 193, 231–236. doi: 10.1111/j.1574-6968.2000.tb09429.x
Felsenstein, J. (1985). Confidence limits on phylogenies: an approach using the bootstrap. Evolution 39, 783–791. doi: 10.1111/j.1558-5646.1985.tb00420.x
Goldstein, A. H. (1994). “Involvement of the quinoprotein glucose dehydrogenase in the solubilization of exogenous phosphates by Gram-negative bacteria,” in Phosphate in Microorganisms: Cellular and Molecular Biology, eds A. Torriani-Gorini, E. Yagil, and S. Silver (Washington, DC: ASM Press), 197–203.
Goosen, N., Horsman, H. P., Huinen, R. G., and van de Putte, P. (1989). Acinetobacter calcoaceticus genes involved in biosynthesis of the coenzyme pyrrolo-quinoline-quinone: nucleotide sequence and expression in Escherichia coli K-12. J. Bacteriol. 171, 447–455. doi: 10.1128/JB.171.1.447-455.1989
Goosen, N., Vermaas, D. A., and Putte, P. V. D. (1987). Cloning of the genes involved in synthesis of coenzyme pyrrolo-quinoline-quinone from Acinetobacter calcoaceticus. J. Bacteriol. 169, 303–307. doi: 10.1128/JB.169.1.303-307.1987
Gouy, M., and Gautier, C. (1982). Codon usage in bacteria: correlation with gene expressivity. Nucleic Acid Res. 10, 7055–7074. doi: 10.1093/nar/10.22.7055
Greenacre, M. J. (1984). Theory and Applications of Correspondence Analysis. Academic Press: London.
Grell, T. A., Goldman, P. J., and Drennan, C. L. (2014). SPASM and twitch domains in S-adenosylmethionine (SAM) radical enzymes. J. Biol. Chem. 290, 3964–3971. doi: 10.1074/jbc.R114.581249
Gu, W., Zhou, T., Ma, J., Sun, X., and Lu, Z. (2004). The relationship between synonymous codon usage and protein structure in Escherichia coli and Homo sapiens. BioSystems 73, 89–97. doi: 10.1016/j.biosystems.2003.10.001
Guo, Y. B., Li, J., Li, L., Chen, F., Wu, W., Wang, J., et al. (2009). Mutations that disrupt either the pqq or the gdh gene of Rahnella aquatilis abolish the production of an antibacterial substance and result in reduced biological control of grapevine crown gall. Appl. Environ. Microbiol. 75, 6792–6803. doi: 10.1128/AEM.00902-09
Gupta, G., Parihar, S. S., Ahirwar, N. K., Snehi, S. K., and Singh, V. (2015). Plant growth promoting rhizobacteria (PGPR): current and future prospects for development of sustainable agriculture. J. Microb. Biochem. Technol. 7, 96–102. doi: 10.4172/1948-5948.1000188
Gupta, S., Bhattacharyya, T., and Ghosh, T. C. (2004). Synonymous codon usage in Lactococcus lactis: mutational bias versus translational selection. J. Biomol. Struct. Dyn. 21, 527–535. doi: 10.1080/07391102.2004.10506946
Gyaneshwar, P., Naresh, K. G., Parekh, L. G., and Poole, P. S. (2002). Role of soil microorganisms in improving P nutrition of plants. Plant Soil. 245, 83–89. doi: 10.1023/A:1020663916259
Hart, A., Cortés, M. P., Latorre, M., and Martinez, S. (2018). Codon usage bias reveals genomic adaptations to environmental conditions in an acidophilic consortium. PLoS ONE 13:e0195869. doi: 10.1371/journal.pone.0195869
He, B., Dong, H., Jiang, C., Cao, F., Tao, S., and Xu, L. A. (2016). Analysis of codon usage patterns in Ginkgo biloba reveals codon usage tendency from A/U-ending to G/C-ending. Sci. Rep. 6:35927. doi: 10.1038/srep35927
Hershberg, R., and Petrov, D. A. (2008). Selection on codon bias. Annu. Rev. Genet. 42, 287–299. doi: 10.1146/annurev.genet.42.110807.091442
Hildebrand, F., Meyer, A., and Eyre-Walker, A. (2010). Evidence of selection upon genomic GC-content in bacteria. PLoS Genet. 6:e1001107. doi: 10.1371/journal.pgen.1001107
Huang, X., Xu, J., Chen, L., Wang, Y., Gu, X., Peng, X., et al. (2017). Analysis of transcriptome data reveals multifactor constraint on codon usage in Taenia multiceps. BMC Genomics 18:308. doi: 10.1186/s12864-017-3704-8
Ikemura, T. (1981). Correlation between the abundance of Escherichia coli transfer RNAs and the occurrence of the respective codons in its protein genes: a proposal for a synonymous codon choice that is optimal for the E. coli translational system. J. Mol. Biol. 151, 389–409. doi: 10.1016/0022-2836(81)90003-6
Ikemura, T. (1985). Codon usage and tRNA content in unicellular and multicellular organisms. Mol. Biol. Evol. 2, 13–34.
Jenkins, G. M., and Holmes, E. C. (2003). The extent of codon usage bias in human RNA viruses and its evolutionary origin. Virus Res. 92, 1–7. doi: 10.1016/S0168-1702(02)00309-X
Kafle, A., Cope, K., Raths, R., Krishna, Y. J., Subramanian, S., Bucking, H., et al. (2019). Harnessing soil microbes to improve plant phosphate efficiency in cropping systems. Agronomy 9:127. doi: 10.3390/agronomy9030127
Kalayu, G. (2019). Phosphate solubilizing microorganisms: promising approach as biofertilizers. Int. J. Agron. 10, 1–7. doi: 10.1155/2019/4917256
Kim, M. S., McDonald, G. A., and Jordan, D. (1997). Solubilization of hydroxyapatite by Enterobacter agglomerans and cloned Escherichia coli in culture medium. Biol Fertil Soils 24, 337–342. doi: 10.1007/s003740050256
Klinman, J. P., and Bonnot, F. (2013). Intrigues and intricacies of the biosynthetic pathways for the enzymatic quinocofacters: PQQ, TTQ, CTQ, TPQ, and LTQ. Chem. Rev. 114, 4343–4365. doi: 10.1021/cr400475g
Koehn, E. M., Latham, J. A., Armand, T., Evans, R. L., Tu, X., Wilmot, C. M., et al. (2019). Discovery of hydroxylase activity for PqqB provides a missing link in the pyrroloquinoline quinone biosynthetic pathway. J. Am. Chem. Soc. 141, 4398–4405. doi: 10.1021/jacs.8b13453
Kumar, S., Nei, M., Dudley, J., and Tamura, K. (2008). MEGA: a biologistcentric software for evolutionary analysis of DNA and protein sequences. Brief Bioinform. 9, 299–306. doi: 10.1093/bib/bbn017
Kyte, J., and Doolittle, R. F. (1982). A simple method for displaying the hydropathic character of a protein. J. Mol. Biol. 157, 105–132. doi: 10.1016/0022-2836(82)90515-0
Lightfield, J., Fram, N. R., and Ely, B. (2011). Across bacterial phyla, distantly-related genomes with similar genomic GC content have similar patterns of amino acid usage. PLoS ONE 6:e17677. doi: 10.1371/journal.pone.0017677
Liu, Q. (2006). Analysis of codon usage pattern in the radioresistant bacterium Deinococcus radiodurans. Biosystem 85, 99–106. doi: 10.1016/j.biosystems.2005.12.003
Liu, Y. Q., Wang, Y. H., Kong, W. L., Liu, W. H., Xie, X. L., and Wu, X. Q. (2020). Identification, cloning and expression patterns of the genes related to phosphate solubilization in Burkholderia multivorans WS-FJ9 under different soluble phosphate levels. AMB Expr. 10:108. doi: 10.1186/s13568-020-01032-4
Lobry, J. R., and Gautier, C. (1994). Hydrophobicity, expressivity and aromaticity are the major trends of amino-acid usage in 999 Escherichia coli chromosome-encoded genes. Nucleic Acid Res. 22, 3174–3180. doi: 10.1093/nar/22.15.3174
Ludueña, L. M., Anzuay, M. S., Angelini, J. G., Barros, G., Luna, M. F., Monge, M. P., et al. (2017). Role of bacterial pyrroloquinoline quinone in phosphate solubilizing ability and in plant growth promotion on strain Serratia sp. S119. Symbiosis 72, 31–43. doi: 10.1007/s13199-016-0434-7
Matsumura, H., Umezawa, K., Takeda, K., Sugimoto, N., Ishida, T., Samejima, M., et al. (2014). Discovery of a eukaryotic pyrroloquinoline quinone-dependent oxidoreductase belonging to a new auxiliary activity family in the database of carbohydrate-active enzymes. PLoS ONE 9:e104851. doi: 10.1371/journal.pone.0104851
Matsushita, K., Arents, J. C., Bader, R., Yamada, M., Adachi, O., and Postma, P. W. (1997). Escherichia coli is unable to produce pyrroloquinoline quinone (PQQ). Microbiology 143 (Pt 10), 3149–3156. doi: 10.1099/00221287-143-10-3149
Matsutani, M., and Yakushi, T. (2018). Pyrroloquinoline quinone-dependent dehydrogenases of acetic acid bacteria. Appl. Microbiol. Biotechnol. 102, 9531–9540. doi: 10.1007/s00253-018-9360-3
McEwan, C. E., Gatherer, D., and McEwan, N. R. (1998). Nitrogen-fixing aerobic bacteria have higher genomic GC content than non-fixing species within the same genus. Hereditas 128, 173–178. doi: 10.1111/j.1601-5223.1998.00173.x
Menendez, C., Igloi, G., Henninger, H., and Brandsch, R. (1995). A pAO-1-encoded molybdopterin cofactor gene (moaA) of Arthrobacter nicotinovorans: characterization and site-directed mutagenesis of the encoded protein. Arch. Microbiol. 164, 142–151. doi: 10.1007/BF02525320
Meulenberg, J. J., Sellink, E., Riegman, N. H., and Postma, P. W. (1992). Nucleotide sequence and structure of the Klebsiella pneumonia pqq operon. Mol. Gen. Genet. 232, 284–294. doi: 10.1007/BF00280008
Meyer, J. B., Frapolli, M., Keel, C., and Maurhofer, M. (2011). Pyrroloquinoline quinone biosynthesis gene pqqC, a novel molecular marker for studying the phylogeny and diversity of phosphate-solubilizing Pseudomonads. Appl. Environ. Microbiol. 77, 7345–7354. doi: 10.1128/AEM.05434-11
Mhlongo, M. I., Piater, L. A., Madala, N. E., Labuschagne, N., and Dubery, I. A. (2018). The chemistry of plant-microbe interactions in the rhizosphere and the potential for metabolomics to reveal signaling related to defense priming and induced systemic resistance. Front. Plant Sci. 9:112. doi: 10.3389/fpls.2018.00112
Mondal, S. K., Kundu, S., Das, R., and Roy, S. (2016). Analysis of phylogeny and codon usage bias and relationship of GC content, amino acid composition with expression of the structural nif genes. J. Biomol. Struct. Dyn. 34, 1649–1666. doi: 10.1080/07391102.2015.1087334
Mondal, S. K., Shit, S., and Kundu, S. (2013). A comparative computational study of the ‘rbcL’ gene in plants and in the three prokaryotic families-Archaea, cyanobacteria and proteobacteria. Ind. J. Biotech. 12, 58–66.
Morris, C. J., Biville, F., Turlin, E., Lee, E., Ellermann, K., Fan, W. H., et al. (1994). Isolation, phenotypic characterization, and complementation analysis of mutants of Methylobacterium extorquens AM1 unable to synthesize pyrroloquinoline quinone and sequences of pqqD, pqqG, and pqqC. J. Bacteriol. 176, 1746–1755. doi: 10.1128/JB.176.6.1746-1755.1994
Mukhtar, S., Zareen, M., Khaliq, Z., Mehnaz, S., and Malik, K. A. (2020). Phylogenetic analysis of halophyte-associated rhizobacteria and effect of halotolerant and halophilic phosphatesolubilizing biofertilizers on maize growth under salinity stress conditions. J. Appl. Microbiol. 128, 556–573. doi: 10.1111/jam.14497
Oteino, N., Lally, R. D., Kiwanuka, S., Lloyd, A., Ryan, D., Germaine, K. J., et al. (2015). Plant growth promotion induced by phosphate solubilizing endophytic Pseudomonas isolates. Front. Microbial. 6:745. doi: 10.3389/fmicb.2015.00745
Palidwor, G. A., Perkins, T. J., and Xia, X. (2010). A general model of codon bias due to GC mutational bias. PLoS ONE 5:e13431. doi: 10.1371/journal.pone.0013431
Pedan, J. F. (2000). Analysis of codon usages (Ph.D. thesis). University of Nottingham. Nottingham, United Kingdom.
Pieterse, C. M., Zamioudis, C., Berendsen, R. L., Weller, D. M., Van Wees, S. C., and Bakker, P. A. (2014). Induced systemic resistance by beneficial microbes. Annu. Rev. Phytopathol. 52, 347–375. doi: 10.1146/annurev-phyto-082712-102340
Puehringer, S., Metlitzky, M., and Schwarzenbacher, R. (2008). The pyrroloquinoline quinone biosynthesis pathway revisited: a structural approach. BMC Biochem. 9:8. doi: 10.1186/1471-2091-9-8
Raghavan, R., Kelkar, Y. D., and Ochman, H. (2012). A selective force favoring increased GC content in bacterial genes. Proc. Natl. Acad. Sci. U.S.A. 109, 14504–14507. doi: 10.1073/pnas.1205683109
Rahman, S. U., Yao, X., Li, X., Chen, D., and Tao, S. (2018). Analysis of codon usage bias of Crimean-Congo hemorrhagic fever virus and its adaptation to hosts. Infect. Genet. Evol. 58, 1–16. doi: 10.1016/j.meegid.2017.11.027
Rajagopalan, K. V., and Johnson, J. L. (1992). The pterin molybdenum cofactors. J. Biol. Chem. 267, 10199–10202. doi: 10.1016/S0021-9258(19)50001-1
Rajewska, M., Wegrzyn, K., and Konieczny, I. (2012). ATrich region and repeated sequences - the essential elements of replication origins of bacterial replicons. FEMS Microbiol. Rev. 36, 408–434. doi: 10.1111/j.1574-6976.2011.00300.x
Ran, W., Kristensen, D. M., and Koonin, E. V. (2014). Coupling between protein level selection and codon usage optimization in the evolution of bacteria and archaea. mBio 5, e00956–e00914. doi: 10.1128/mBio.00956-14
Rivers, S. L., McNairn, E., Blasco, F., Giordano, G., and Boxer, D. H. (1993). Molecular genetic analysis of the moa operon of Escherichia coli K-12 required for molybdenum cofactor biosynthesis. Mol. Microbiol. 8, 1071–1081. doi: 10.1111/j.1365-2958.1993.tb01652.x
Rodríguez, H., and Fraga, R. (1999). Phosphate solubilizing bacteria and their role in plant growth promotion. Biotechnol. Adv. 17, 319–339. doi: 10.1016/S0734-9750(99)00014-2
Romero, H., Zavala, A., and Musto, H. (2000). Codon usage in Chlamydia trachomatisis the result of strand-specific mutational biases and a complex pattern of selective forces. Nucleic Acids Res. 28, 1084–2090. doi: 10.1093/nar/28.10.2084
RoseFigura, J. M. (2010). Investigation of the structure and mechanism of a PQQ biosynthetic pathway component, PqqC, and a bioinformatics analysis of potential PQQ producing organisms (Ph.D. thesis). University of California, Berkeley, CA, United States.
Saha, J., Saha, B. K., Pal Sarkar, M., Roy, V., Mandal, P., and Pal, A. (2019). Comparative genomic analysis of soil dwelling bacteria utilizing a combinational codon usage and molecular phylogenetic approach accentuating on key housekeeping genes. Front. Microbiol. 10:2896. doi: 10.3389/fmicb.2019.02896
Saichana, N., Tanizawa, K., Ueno, H., Pechoušek, J., Novák, P., and Frébortová, J. (2017). Characterization of auxiliary iron–sulfur clusters in a radical S-adenosylmethionine enzyme PqqE from Methylobacterium extorquens AM1. FEBS Open Bio. 7, 1864–1879. doi: 10.1002/2211-5463.12314
Saitou, N., and Nei, M. (1987). The neighbor-joining method: a new method for reconstructing phylogenetic trees. Mol. Biol. Evol. 4, 406–425.
Seward, E. A., and Kelly, S. (2016). Dietary nitrogen alters codon bias and genome composition in parasitic microorganisms. Genome Biol. 17:226. doi: 10.1186/s13059-016-1087-9
Sharma, S. B., Sayyed, R. Z., Trivedi, M. H., and Gobi, T. A. (2013). Phosphate solubilizing microbes: sustainable approach for managing phosphorus deficiency in agricultural soils. Springerplus 2, 587–600. doi: 10.1186/2193-1801-2-587
Sharp, P. M., Bailes, E., Grocock, R. J., Peden, J. F., and Sockett, R. E. (2005). Variation in the strength of selected codon usage bias among bacteria. Nucleic Acid Res. 33, 1141–1153. doi: 10.1093/nar/gki242
Sharp, P. M., and Li, W. H (1986). An evolutionary perspective on synonymous codon usage in unicellular organisms. J. Mol. Evol. 24, 28–38. doi: 10.1007/BF02099948
Sharp, P. M., and Li, W. H. (1987). The codon Adaptation Index–a measure of directional synonymous codon usage bias, and its potential applications. Nucleic Acids Res. 15, 1281–1295. doi: 10.1093/nar/15.3.1281
Sharp, P. M., Tuohy, T. M. F., and Mosurski, K. R. (1986). Codon usage in yeast: cluster analysis clearly differentiates highly and lowly expressed genes. Nucleic Acid Res. 14, 5125–5143. doi: 10.1093/nar/14.13.5125
Shen, Y. Q., Bonnot, F., Imsand, E. M., Rosefigura, J. M., Sjölander, K., and Klinman, J. P. (2012). Distribution and properties of the genes encoding the biosynthesis of the bacterial cofactor, pyrroloquinoline quinine. Biochemistry 51, 2265–2275. doi: 10.1021/bi201763d
Sievers, F., Wilm, A., Dineen, D., Gibson, T. J., Karplus, K., Li, W., et al. (2011). Fast, scalable generation of high-quality protein multiple sequence alignments using Clustal Omega. Mol. Syst. Biol. 7:539. doi: 10.1038/msb.2011.75
Sonnenburg, E. D., and Sonnenburg, J. L. (2019). The ancestral and industrialized gut microbiota and implications for human health. Nat. Rev. Microbiol. 17, 383–390. doi: 10.1038/s41579-019-0191-8
Stoletzki, N., and Eyre-Walker, A. (2007). Synonymous codon usage in Escherichia coli: selection for translational accuracy. Mol. Biol. Evol. 24, 374–381. doi: 10.1093/molbev/msl166
Sueoka, N. (1988). Directional mutation pressure and neutral molecular evolution. Proc. Natl. Acad. Sci. U.S.A. 85, 2653–2657. doi: 10.1073/pnas.85.8.2653
Tamura, K., Stecher, G., Peterson, D., Filipski, A., and Kumar, S. (2013). MEGA6: molecular evolutionary genetics analysis version 6.0. Mol. Biol. Evol. 30, 2725–2729. doi: 10.1093/molbev/mst197
Tao, L., Zhu, W., Klinman, J. P., and Britt, R. D. (2019). Electron paramagnetic resonance spectroscopic identification of the Fe-S clusters in the SPASM domain-containing radical SAM enzyme PqqE. Biochemistry 58, 5173–5187. doi: 10.1021/acs.biochem.9b00960
Tatarinova, T., Elhaik, E., and Pellegrini, M. (2013). Crossspecies analysis of genic GC3 content and DNA methylation patterns. Genome Biol. Evol. 5, 1443–1456. doi: 10.1093/gbe/evt103
Tatarinova, T. V., Alexandrov, N. N., Bouck, J. B., and Feldmann, K. A. (2010). GC3 biology in corn, rice, sorghum and other grasses. BMC Genomics, 11, 308. doi: 10.1186/1471-2164-11-308
Thompson, J. D., Higgins, D. G., and Gibson, T. J. (1994). Clustal W: improving the sensitivity of progressive multiple sequence alignment through sequence weighting, position-specific gap penalties and weight matrix choice. Nucleic Acids Res. 22, 4673–4680. doi: 10.1093/nar/22.22.4673
Torres, M., Hong, K. W., Chong, T. M., Reina, J. C., Chan, G. K., Dessaux, Y., et al. (2019). Genomic analyses of two Alteromonas stellipolaris strains reveal traits with potential biotechnological applications. Sci. Rep. 9:1215. doi: 10.1038/s41598-018-37720-2
Toyama, H., Chistoserdoval, L., and Lidstrom, M. E. (1997). Sequence analysis of pqq genes required for biosynthesis of pyrroloquinoline quinone in Methylobacterium extorquens AM1 and the purification of a biosynthetic intermediate. Microbiology 144, 183–191. doi: 10.1099/00221287-143-2-595
Trotta, E. (2013). Selection on codon bias in yeast: a transcriptional hypothesis. Nucleic Acids Res. 41, 9382–9395. doi: 10.1093/nar/gkt740
Velterop, J. S., Sellink, E., Meulenberg, J. J., David, S., Bulder, I., and Postma, P. W. (1995). Synthesis of the pyrroloquinoline quinone in vivo and in vitro and detection of an intermediate in the biosynthetic pathway. J. Bacteriol. 177, 5088–5098. doi: 10.1128/JB.177.17.5088-5098.1995
Wan, X. F., Xu, D., Kleinhofs, A., and Zhou, J. (2004). Quantitative relationship between synonymous codon usage bias and GC composition across unicellular genomes. BMC Evol. Biol. 4:19. doi: 10.1186/1471-2148-4-19
Wecksler, S. R., Stoll, S., Tran, H., Magnusson, O. T., Wu, S., King, D., et al. (2009). Pyrroloquinoline quinine biogenesis: demonstration that PqqE from Klebsiella pneumoniae is aradical S-adenosyl-L-methionine enzyme. Biochemistry 48, 10151–10161. doi: 10.1021/bi900918b
Wright, F. (1990). The ‘effective number of codons’ used in a gene. Gene 87, 23–29. doi: 10.1016/0378-1119(90)90491-9
Xu, J., Deng, P., Showmaker, K. C., Wang, H., Baird, S. M., and Lu, S. E. (2014). The pqqC gene is essential for antifungal activity of Pseudomonas kilonensis JX22 against Fusarium oxysporum f. sp. lycopersici. FEMS Microbiol. Lett. 353, 98–105. doi: 10.1111/1574-6968.12411
Yannai, A., Katz, S., and Hershberg, R. (2018). The Codon usage of lowly expressed genes is subject to natural selection. Genome Biol. Evol. 10, 1237–1246. doi: 10.1093/gbe/evy084
Youssef, M. M. A., and Eissa, M. F. M. (2014). Biofertilizers and their role in management of plant parasitic nematodes. J. Biotechnol. Pharmaceutical. Res. 5, 1–6.
Zhao, K., Penttinen, P., Zhang, X., Ao, X., Liu, M., Yu, X., et al. (2014). Maize rhizosphere in Sichuan, China, hosts plant growth promoting Burkholderia cepacia with phosphate solubilizing and antifungal abilities. Microbiol. Res. 169, 76–82. doi: 10.1016/j.micres.2013.07.003
Keywords: plant solubilizing microbes, pyrroloquinoline quinine, phylogeny, codon usage bias, correspondence analysis
Citation: Bhanja E, Das R, Begum Y and Mondal SK (2021) Study of Pyrroloquinoline Quinine From Phosphate-Solubilizing Microbes Responsible for Plant Growth: In silico Approach. Front. Agron. 3:667339. doi: 10.3389/fagro.2021.667339
Received: 12 February 2021; Accepted: 22 April 2021;
Published: 04 June 2021.
Edited by:
Avishek Banik, Presidency University, IndiaReviewed by:
Ayon Pal, Raiganj University, IndiaDr. Siddiq Ur Rahman, Khushal Khan Khattak University, Pakistan
Copyright © 2021 Bhanja, Das, Begum and Mondal. This is an open-access article distributed under the terms of the Creative Commons Attribution License (CC BY). The use, distribution or reproduction in other forums is permitted, provided the original author(s) and the copyright owner(s) are credited and that the original publication in this journal is cited, in accordance with accepted academic practice. No use, distribution or reproduction is permitted which does not comply with these terms.
*Correspondence: Sunil Kanti Mondal, c2ttb25kYWxAYmlvdGVjaC5idXJ1bml2LmFjLmlu
†These authors have contributed equally to this work