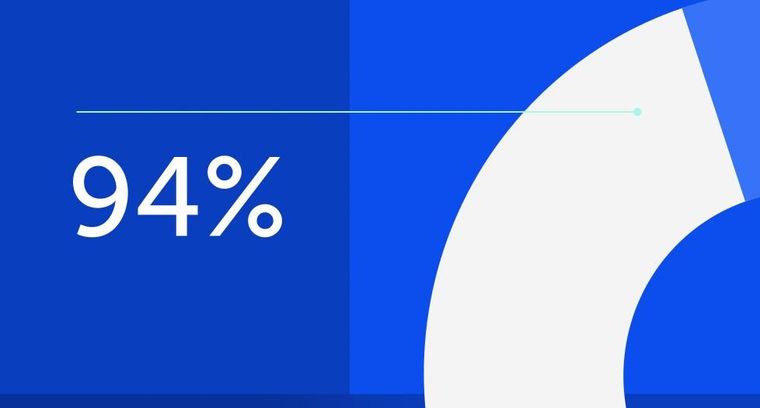
94% of researchers rate our articles as excellent or good
Learn more about the work of our research integrity team to safeguard the quality of each article we publish.
Find out more
ORIGINAL RESEARCH article
Front. Agron., 09 April 2021
Sec. Weed Management
Volume 3 - 2021 | https://doi.org/10.3389/fagro.2021.655383
This article is part of the Research TopicWeed Biology and Ecology in AgroecosystemsView all 13 articles
Throughout Europe, Cirsium arvense is the most problematic perennial weed in arable crops, whether managed under organic or conventional agriculture. Non-chemical control methods are limited with partial efficacy. Knowledge is missing on their effect across a wide gradient of cropping systems and pedoclimates. To achieve effective Cirsium arvense management ensuring crop productivity while limiting the reliance of cropping systems on herbicide, expert-based models are needed to gather knowledge on the effect of individual levers and their interactions in order to (i) design and assess finely tuned combinations of farming practices in different pedoclimates and (ii) support decisions for Cirsium arvense control. Based on expert-knowledge and literature, we developed IPSIM-Cirsium, a hierarchical qualitative model which evaluates the infestation of Cirsium arvense as a function of farming practices, climate conditions, soil descriptors and their interactions. IPSIM-Cirsium is a multi-attribute model considering all possibilities of interactions between factors, it estimates the infestation rate of the field graded according to a four-level scale. The model outputs were confronted to independent field observations collected across 6 fields, over a 16-year period in 3 sites. IPSIM-Cirsium showed a satisfactory predictive quality (accuracy of 78.2%). IPSIM-Cirsium can be used as a tool for crop advisors and researchers to assist the design of systems less reliant on herbicides, for farmers and advisers to assess ex-ante prototypes of cropping systems, and for teachers as an educational tool to share agroecological weed management knowledge.
Weed management is essential to limit their harmfulness against crops such as yield loss, decline of crop harvest quality and harvest difficulties (Colbach et al., 2021). Nowadays, weed management relies on herbicides, and its intensive use raises concern on public health, soil-water-air contamination, biodiversity maintenance (Stoate et al., 2009), and development of herbicide resistance (Powles and Yu, 2010). Reducing the reliance of cropping systems on pesticides is promoted throughout Europe (e.g., EU legislation and the French ECOPHYTO National Action Plan). Authorities strengthened the criteria to deliver marketing authorizations for pesticides, leading to a dynamic of withdrawal of herbicide (e.g., Carbamate herbicides such as Butylat or Chlorobufam) over the past decades (Chauvel et al., 2012). In addition, public policies aim at decreasing the use of widely used authorized herbicides, such as Glyphosate. Decreasing herbicide use while ensuring crop productivity and economic profitability of farming systems requires a deep redesign of cropping systems implementing ‘many little hammers' to curtail weed population increase (Liebman et al., 1997). However, the management of perennial weeds remain of high concern in integrated cropping systems (Favrelière et al., 2020). While annual weeds rely on their seed to maintain their population over the years, perennial weeds base their survival on their vegetative reproduction. Cirsium arvense (L) Scop. is the most problematic perennial weed in Europe. A density of 15 and 30 shoots/m2 can reduce cereal yield by 35% and more than 50%, respectively (Hodgson, 1968; Favrelière, 2019). Seed production by C. arvense is sometimes reported to be sizable (Gruber and Claupein, 2009), but Donald (1990) observed that it can be restricted, limiting harvest pollution with weed seeds. Restraining C. arvense infestation in a particular location and avoiding seed production is crucial to its establishment in new locations within a given landscape since, as many Asteraceae species, C. arvense seeds are transported by the wind (Tiley, 2010), implying a management at the landscape scale. Weedy green biomass at harvest timings located above the cutting bar of the combine harvest may increase harvest difficulties (Mézière et al., 2015), but this was not precisely quantified. The prickly mature foliage deters livestock from grazing (Schreiber, 1967). Non-chemical control methods are limited and with partial effects (Melander et al., 2013; Davis et al., 2018). Most herbicide-free weed management levers rely on intensive tillage, high diversity of crop in the crop sequence and increased competitiveness with subsidiary crops (Lukashyk et al., 2008; Brandsæter et al., 2012; Melander et al., 2012; Miller, 2016). Despite existing knowledge on particular levers and their effect with long-term perspectives, information on long-term combination of multiple levers in various production contexts remains scarce because the effect of interactions between cropping practices and pedoclimate remain only partially known.
Expert knowledge is needed to elucidate the significance of different integrated weed management tactics in various pedoclimates and production situations, evaluate the emphasis of each practice, their interactions and synthesize this knowledge as a model to assess designed strategies and forecast future weed dynamics. This expert approach, associated with literature, is the aim of the IPSIM framework (Injury Profile SIMulator) developed by Aubertot and Robin (2013), using wheat-eyespot as a case study to present a proof of concept. Models were developed to understand the impact of C. arvense on the yield in cereal fields (Donald and Khan, 1996; Rasmussen and Nielsen, 2020), without considering neither cropping practices, nor pedoclimate and field environment. In the literature, some models simulate the long-term effect of cropping systems on multiple weed species and quantify the impact of weeds through multiple criteria including yield loss (Colbach et al., 2021), but most of these models do not include perennial species. Models and/or decision support tools dedicated to perennial weeds are scarce. They focus only on non-chemical cropping practices (Favrelière et al., 2016), and on chemical efficiency (Liu et al., 2019), but do not consider the interaction of cropping practices with pedoclimate and field environment. In addition, they are not designed to assess cropping systems or to be used as an educational tool to design innovative cropping systems. Our objectives are: (i) to identify the most significant cropping practices and pedoclimate variables, and their combinations impacting the growth of C. arvense, (ii) to better understand their efficacy to replace chemical-only control methods, (iii) to determine interactions between cropping practices and pedoclimate to tackle the complexity of a limited part of agroecosystems, and finally (iv) to develop an evaluation tool for farmers and advisers through a consensual model, simple to use.
The conception of the C. arvense model relies on the IPSIM platform. IPSIM, i.e., Injury Profile SIMulator, was first designed by (Aubertot and Robin, 2013). IPSIM is a generic modeling method which aims at apprehending cropping practices, pedoclimate and environmental factors to explain injuries caused by a single or several pests, on a specific or a set of crops. Cropping practices refer to all the cultivation techniques used in the process of crop production (e.g., tillage, harvest, sowing, etc.), pedoclimate refer to the soil and weather components impacting the development of the considered pest (e.g., soil texture, rainfall, temperature, etc.), and field environment to the abiotic or biotic factors encountered in the field surroundings (e.g., field margins, host plants, etc.). All these components are selected according to their significance in the explanation of the injury profile of the considered pests.
This platform requires the organization of its hierarchy according to a specific plan, implemented with the DEXi software (Bohanec, 2020). The DEX method, implemented by the software DEXi, supports qualitative hierarchical attribute aggregation. Originally, this method was designed as a decision modeling method based on the subdivision of a complex problem into smaller and less complex subproblems. These subproblems are represented by hierarchically structured attributes, i.e., variables that characterize the complex problem. Terminal attributes of the hierarchy represent inputs (or input indicators), while the root represents the main output of the model. Any number of aggregated attributes (internal nodes in the hierarchy) can be placed between inputs and outputs; they correspond to subproblems and represent intermediate or partial outputs of evaluation. A DEX model is used so that the input attributes are filled in by the user of the model, providing a description of the problem at hand. Then, the values of aggregated attributes are determined with the aggregation of the corresponding input attributes or underlying attributes. The aggregation takes place in accordance with aggregating tables, previously formulated by domain experts. Aggregating tables consist of elementary “if-then” rules that describe output values for all combinations of input values. Each aggregated attribute in the model has an associated aggregating table. During and after their construction, all tables are verified by DEXi for completeness and consistency. Attributes used in the model are qualitative variables, either ordinal or nominal. The use of quantitative variables is not possible directly through the DEXi software, however upstream converters can be designed to discretize quantitative variables before they are used in the model, or to convert nominal variables into ordinal ones (e.g., the name of a cultivar can be converted into a qualitative level of resistance to a disease).
The building process of an IPSIM model requires three steps: (i) identifying and structuring the attributes, (ii) defining attribute scales, and (iii) defining the aggregating tables (Aubertot and Robin, 2013).
The IPSIM method aims at apprehending a wide variety of factors or indicators to model an injury profile. A generic pattern of IPSIM main attributes is to consider any factor that might harm or benefit to the single or multiple modeled organisms, directly (e.g., control method) or indirectly (e.g., type of soil). These factors are considered either punctual or on a larger scale to be considered for several years. IPSIM-Cirsium is a static deterministic model. IPSIM-Cirsium aims at representing only the infestation of C. arvense in an identified field. Therefore, the output of the model is defined to express a weed infestation rate, represented as a qualitative variable. This qualitative output variable can be translated into quantitative variables as density (number of shoots/m2), biomass above ground (g/m2), or percentage of covering of the field.
Factors were chosen first according to the literature with keywords involving general and generic growing factors (e.g., Temperature, Rainfall, Soil, Relative Humidity, Photoperiod) and control methods (e.g., Competitive crops, Cover crops, Tillage, Cropping practices, etc.) related to C. arvense. This literature analysis was made using commercial databases (EconLit, Food Science Source, Web of Science, MEDLINE®, Saga Web, Scopus, TAIR) and free databases (Google Scholar, Agricola, ProdINRA, PubMed). The list of beneficial and detrimental factors was then confronted to experts during workshops to co-design the model. The experts were chosen nationally from research institutes (INRAE), technical institutes (Arvalis, Terres Inovia, Acta), and Chambers of Agriculture according to their participation on Cirsium arvense control programs or expertise. These co-design workshops aimed at validating the input attribute choices, structure, and interactions and to identify new attributes that could have been omitted.
The aggregated attributes of IPSIM-Cirsium as main factors were defined according to (i) the weed environment and (ii) the weed management methods. The weed environment is spatially limited to the considered field and temporally to the current year of evaluation of weed infestation rate. Control methods were chosen to also focus on the spatial field environment and the transfer of individuals of Cirsium arvense between fields due to skipped cleaning of tool was omitted here in the sake of simplicity. However, control methods were considered in the 4 years preceding the evaluation of the weed infestation. This wide time window for control methods is explained by the perennial character of Cirsium arvense. Input attributes are then chosen as indicators of the risk of infestation linked to the environment of the field and control methods efficacy that impact the growth of C. arvense. The structure of attributes of IPSIM-Cirsium is presented in Figure 1.
Figure 1. Hierarchical structure of IPSIM-Cirsium. Bold and not in bold terms represent aggregated and basic attributes, respectively. (20 basic attributes and 13 aggregated attributes) (screenshot of the DEXi software).
The following step is to set scale values to each attribute. Aggregated and input attributes of IPSIM-Cirsium have either two or three levels of scale (e.g., Unfavorable, Moderately favorable, and Favorable). They are represented by words and can be either ordinal or nominal. Unfavorable means that this attribute is detrimental to the user and therefore detrimental to the control of Cirsium arvense (Figure 2). This scale order is designed directly under DEXi software and will be prevalent for the establishment of aggregating tables.
Figure 2. Attribute scales of IPSIM-Cirsium. All of the scales are ordered from values favorable to Cirsium arvense (i.e., detrimental to the user) on the left-hand side (Red color) to values unfavorable to Cirsium arvense (i.e., beneficial to the user) on the right-hand side (Green color). (screenshot of the DEXi software).
Scale values are sometimes a result of a conversion of quantitative or qualitative variables. For example, the amount of rain per month is categorized into three levels: Favorable to Cirsium, Moderately Favorable to Cirsium, and Unfavorable to Cirsium. The levels of this attribute were defined by a converter using two thresholds. Some attributes are purely descriptive and need to be converted prior being used in the model. A converter is then used to qualify this information into a qualitative value that can be used by the model (e.g., Tools used for the cover crop destruction must be categorized as Favorable or Unfavorable to the development of Cirsium arvense). The corresponding converters are defined by considering international literature and expertise, and need to be adapted for each considered region, especially for the sowing rate of the crop.
In a few instances, attributes are described by a two-level scale (e.g., for Return of the ley attribute, the user only must specify if he had ley in the three preceding years or not). Attributes generally have a three-level scale (e.g., Sown crop can generate a closed, moderately closed, or open canopy). The output attribute of the model IPSIM-Cirsium has a four-level scale (i.e., Very low infestation, Low infestation, Intermediate infestation, and High infestation). We chose to define four levels of infestation in order to describe the evolution of infestation throughout several years.
The last step to build an IPSIM model is the definition of aggregative tables for each aggregated attribute and the output of the model. During the aggregation of underlying attributes in the attribute tree, decision rules must be edited to characterize any aggregation possibilities. Collectively, these rules were initially called “Utility functions.” These aggregative rules are simple “if-then” functions that enable the model to provide a specific answer to any situation it is confronted to. Aggregative tables are represented in a tabular form in the DEXi software and aim at considering scale orders of the underlying attributes (Figure 3B).
Figure 3. Decision rules for final level of infestation (“Weed infestation of Cirsium arvense”). Rules are designed according to the Initial level of infestation, represented in (A) by square labeled here as four-level scale : High infestation, Intermediate infestation, Low infestation, and Very low infestation (Green cells are beneficial for the field, Gray cell is neutral for the field and Red cell is detrimental for the field); and the Risk of infestation, represented by arrows, enabling to lower the infestation of C. arvense (Green downward arrows), increase the infestation (Red upward arrows), or maintain the same level of infestation (Gray circular arrows). (B) Represents the “Weed infestation of Cirsium arvense” decision rules translated in the DEXi software (screenshot of the DEXi software) for the 20 possible combinations (four level of Initial infestation, five level of Infestation risk).
To consider each aggregation possibility, we consider all the combinations of scale levels of the underlying attributes. For example, Competitiveness of crop is composed of the aggregation of Sown crop and Sowing density. Sown crop and Sowing density are both three-level-scaled attributes. Therefore, nine aggregation possibilities need to be explored for the aggregated attribute Competitiveness of crop. Each possibility needs to be filled row by row. This process enables a high level of flexibility for each situation encountered. Aggregating tables are defined using literature and expert knowledge, as summarized in Table 1. However, some situations lack scientific consensus in the literature, especially in the combination of several cropping practices. This problem was fixed with expert knowledge. Yet, some possible decisions are sometimes marred with subjectivity of the experts during the process of filling in the aggregative rules.
Table 1. Literature on the effects of climate, soil and cropping practices on the growth of Cirsium arvense.
Weights are widely used in model analysis to describe the importance of each attribute. Weights are defined by the aggregative tables defined at each aggregation of attributes. Originally mainly used on quantitative models, the DEX method managed to adapt weight calculation to qualitative models, too. Weights are obtained by constructing a hyperplane that approximates the points (decision rules) of an aggregative table, to minimize the least squares criterion. Relative weights are then calculated from the slope of this hyperplane: the higher the slope in the direction of an attribute, the higher the weight of this attribute (Bohanec, 2020).
There are four types of weights: local and global weights, normalized or not. Normalized weights consider the number of values per scale (analysis of the weight of IPSIM-Cirsium will rely on normalized weights only); they are calculated by normalizing all scales to the unit interval, thus ruling out the effect of scales having different numbers of values. Local weights are described for each aggregate attribute and the corresponding aggregative table, regardless of attributes and functions elsewhere in the model. Consequently, the sum of the local weights of attributes underlying each aggregated attribute equals to 100%. In contrast, global weights represent the importance of attributes in the context of the whole model. For each attribute, they are calculated by multiplying the local weight of that attribute with the global weight of its parent attribute. The global weight of the root attribute is assumed to be 100%. In this way, the sum of all the input attributes' global weights in the model is 100%, too. For example: if we consider the global normalized weight of Competitiveness of crop (2%) and the local normalized weight of Sown crop (50%), the global normalized weight of Sown crop is 1% (2% × 50%), as shown in Table 3.
These weights enable an approximate overview of the importance of each attribute, input, or aggregated ones. It is an equivalent of sensitivity analysis for quantitative models (Aubertot and Robin, 2013). Weights can also be used to define the aggregative tables, in a reverted strategy of modeling with DEXi software. This strategy was left out in favor of the description of each situation row by row, taking into account the literature and expert knowledge available.
Several datasets (D) were used in the evaluation of the predictive quality of IPSIM-Cirsium, summarized in Table 2. D1 was collected at the INRAE experimental farm in Bretenière (47°14' 11.2” N, 5°05'56.1” E), 15 km southeast of Dijon, France. The complete description of the long-term cropping system experiment (crop sequence and associated management, including intensity of tillage herbicide, use herbicide types, mechanical weeding, etc.) implemented from 2000 to 2017 was synthesized by Adeux et al. (2019). The reference cropping system (CS) called S1 was characterized by a 3-year oilseed rape—winter wheat—winter barley rotation, systematic moldboard plowing in summer-autumn and herbicides as sole curative weed management tool. All alternative cropping systems (S2, S3, S4, and S5) were designed to mimic farmers aiming at reducing herbicide reliance through contrasted agronomical pathways and resulted in more complex 6-year rotations. S2 was a transition from reduced tillage (i.e., no inversion tillage, 2001–2010) to no-till conservation agriculture (2010–2017). S3, S4, and S5 implemented moldboard plowing every 2 years on average over the 2001–2017 period. However, weed management relies uniquely on herbicide in S3, on mechanical tools and herbicide in S4 and only on mechanical tools in S5 (Adeux et al., 2019). Cover crop was sown since 2007 in each of the summer fallow period of a preceding spring or summer crop. Alfalfa was implemented for 1–3 years in S5. These four alternative CS also implemented a wide array of preventive and cropping weed management tools such as false seedbed techniques, delayed sowing of winter cereals, and higher seeding rates. The set of decision rules characterizing each of the five cropping systems was replicated on two blocks (in a 1.7 ha field). All individual farming operations were recorded from 1999 to 2017 in the 10 fields. The abundance of Cirsium arvense was assessed every year from 2002 to 2017, at crop flowering, after all weeding operations, by counting the density of shoots in 8 fixed zones per field with four and one 0.36 m2 quadrats in 2001–2013 and 2014–2017, respectively. Since the zones were fixed over the 2001–2017 period, the maximal density recorded in the four quadrats per zone over the 2001–2013 period was selected to be representative of the zone level, to assess the evolution of Cirsium arvense with 1280 surveys (i.e., eight zones by five cropping systems by two blocks × 16 years). Maximal density was chosen here to represent the Potential of Infestation described in Adeux et al. (2017).
D2 was conducted in Sours (48°24′38.16″N, 1°35′53.16″ E), France. Three systems were surveyed from 2011 to 2020: Autonomous system, Dr. Durupt system and Productor system. These three systems were all conducted in organic conditions with different intensity of tillage, ley implement and rotation as Cirsium arvense control methods. Autonomous and Dr. Durupt systems were conducted in CAPABLE project (CASDAR AAP IP 2017) in a system experiment. Alfalfa was implemented for 3 years, with three management per year (e.g., chopping, mowing). No cover crop was implemented in these experiments. The compaction of soil was characterized as moderate. In Dr. Durupt, Autonomous and Productor systems, intensity of stubble tillage was low, high, and average, respectively. Dr. Durupt system was plowed every 2 years, Autonomous system every year, and Productor system only once every 4 years. Selective cutting and interrow hoeing intensity were low in the three systems. The abundance of Cirsium arvense was assessed every year in June with two different methods. Autonomous and Productor systems were surveyed according to the Chicouène method (Chicouene and Arbiotech, 2000) and Dr. Durupt and Autonomous systems were surveyed with the use of 16 0.5 m2-fixed-quadrats from 2018 to 2020. Only the Chicouène method was performed from 2011 to 2020, resulting in a single abundance value per system per year.
D3 was conducted in Salvagnac (43°54′23.0″N, 1°41′19.0″ E), South of France. Five cropping systems were evaluated from 2015 to 2017: Progressive Tillage Control, Progressive Sustainable Tillage Control, Cover crop, Shallow Tillage Control, and Shallow Sustainable Tillage Control. These different factors were all tested on the same field, on bare soil except for the cover crop (Sorghum) in the Cover crop treatment. Progressive Tillage Control consisted of an increase of 5 cm depth for each stubble cultivation performed each month. Progressive Sustainable Tillage Control consisted of an increase of 5 cm depth for each stubble cultivation performed whenever Cirsium arvense reached five-leaf stage. Cover crop consisted of the use of sorghum (Sorghum sudanense), chopped during summer to control Cirsium arvense, sown every year in May. Shallow Tillage Control consisted of repetitions of stubble cultivation at 8–10 cm depth every month, while Shallow Sustainable Tillage Control was performed at 8–10 cm whenever Cirsium arvense reached five-leaf stage. All the cropping systems are conducted in organic conditions, without the use of any herbicide (organic or not). No selective cutting nor interrow hoeing were performed. Plowing was performed once every four years. Each cropping system was repeated in three blocks. The number of shoots of Cirsium arvense was assessed every year in four fixed plots in each cropping system, composed of four quadrats of 0.25 m2. The four quadrats of each plot were then summed. D3 assessed 60 values of C. arvense density per year, resulting in 120 values for 2016 and 2017.
These survey values were then translated into four levels of infestation according to the scale of the output attribute of IPSIM-Cirsium: Very low, Low, Intermediate, and High corresponding to 0 thistle/m2, 0.01–2.99 thistle/m2, 3.00–6.99 thistle/m2, and ≥7thistle/m2, respectively. This scale was developed according to co-design workshops.
The evaluation of the predictive quality of IPSIM-Cirsium was performed by comparing calculated values (outputs of the model) and observed values (in the field experiment), described earlier. Values were calculated for June of each year, therefore calculated values were compared to values observed in June. The comparison of values led to the construction of a confusion matrix. The confusion matrix is a table that shows the performance of an ordinal or nominal model where rows represent observed values and columns represent calculated values. To summarize confusion matrix, several metrics were computed to evaluate the predictive quality of IPSIM-Cirsium: accuracy, quadratic weighted Cohen's kappa, precision, recall, and F1-Score. The accuracy is the number of correctly calculated values (i.e., calculated value is equal to observed value) among all the calculated values (Nguwi and Cho, 2010), defined as:
where A is the number of correctly assigned calculated values and N the number of calculated values. On the other hand, Cohen's kappa is expressing a score of agreement level between two annotators: observed and calculated value (Cohen, 1960), described as:
where κ is the agreement among observed and calculated annotators (po, the relative observed agreement; pe, the expected agreement when both annotators are randomly chosen). κ rates in between −1 and +1 and can be interpreted as the proportion of variability explained by the model (Fleiss and Cohen, 1973). Values of κ describe the agreement between observed and calculated annotators: <0; 0.01–0.20; 0.21–0.40; 0.41–0.60; 0.61–0.80; and 0.81–1 values qualify the agreement as Poor; Slight; Fair; Moderate; Substantial; and Almost perfect, respectively (McHugh, 2012). F1 score is calculated from the precision p (number of correctly calculated values divided by the number of observed values for each class) and the recall r (number of correctly calculated results divided by the total of calculated values for each class), expressing the harmonic mean between precision and recall, defined as:
with N the number of class, pi the precision of class i and ri the recall for class i. These calculations were performed using RStudio© Version 1.1.456 (Studio, Inc., 2009–2018).
IPSIM-Cirsium was designed focusing on the Risk of infestation of Cirsium arvense and the Initial infestation level observed the year preceding the evaluation year. The possible evolutions from one level of infestation to another are described according to decision rules illustrated in Figure 3. The risk of infestation is calculated for June, before the harvest during summer. The risk is based on the two main sub-trees Environment spring describing the pedoclimate of the field during March, April and May of the evaluation year, and Cropping practices describing the crop management of the field to control Cirsium arvense during the four preceding years of the evaluation year.
The first sub-tree of IPSIM-Cirsium (Figure 1), Environment spring focuses on two main indicators:
(i) Weather during March, April, and May of the evaluation year. To describe the weather, two factors were chosen: the average Temperature and the accumulated Rain. These two factors are described per month and an aggregation of the 3 months was then calculated. Thresholds of the converter used to describe the average temperature and accumulated rain per month were defined according to literature and expert knowledge.
(ii) Compaction of soil of the evaluated field. This indicator describes the compaction of soil during March, April, and May. The compaction of soil is assumed to be constant during this period. Compaction of soil is here seen as an indirect factor favorable to Cirsium arvense, benefiting from the lack of competition provoked by compaction of soil. Type of soil is not directly used as an attribute in the model but is indirectly impacting the compaction of soil.
The second sub-tree describes the Cropping practices on a four-year period preceding the evaluation year, with the help of three main factors:
(i) Competition includes practices implemented before the evaluated year, such as ley or cover crop, and during the evaluated year, such as the crop competitiveness. Ley or cover crop are described with four indicators such as the species used in the ley or cover crop (Crop type ley/cover crop), the Importance of ley and cover crop calculated with the length of the ley or cover crop (Length ley/cover crop) and the length of time since the destruction of the last ley or cover crop (Return ley/cover crop), the number of Management of the ley or cover crop (e.g. chopping, mowing, and pasture), and finally the Termination method of the ley or cover crop (e.g., Frost, Plowing, etc.). The Competitiveness of crop is described with two indicators: the Sown crop which has a score of competitive level for each species described according to literature and expert knowledge; and the Sowing density relative to the regional recommendation of sowing for the concerned species. Competitiveness of crop might be impacted by the use of nutrients, however neither consensus between the experts during workshops, nor in the literature was found on the impact of nutrients on the benefit ratio between crop and weed. Indeed, while crop slightly benefit from the nutrient increase, C. arvense also benefit from the increase of nutrient (Hume, 1982; Edwards et al., 2000; Líška et al., 2007).
(ii) Herbicide use frequency is used in this model as a curative method. However, to be efficient, herbicide must target Cirsium arvense and be repeated several years. The description of the use of herbicide is only related to the number of years that an herbicide control is implemented. Thus, this attribute assumes that herbicides were applied in the best conditions and are efficient on controlling Cirsium arvense, i.e., regardless of the conditions of application (moisture, temperature, etc.), and whatever the dose applied.
(iii) Mechanical operations characterized the physical and mechanical management methods applied during the evaluation year (i.e., Current crop mechanical work) and the ones applied in the four preceding years of the evaluation year (i.e., Stubble tillage effectiveness and Plowing effectiveness). Current crop mechanical work is an aggregated attribute composed of two indicators: the Selective cutting which aims at the cutting of the aerial part of Cirsium arvense, and the Interrow hoeing which aims at the weeding of the superficial roots and aerial parts of Cirsium arvense. These two indicators are quantified according to the number of passes per year. The more the passes the more effective the practices. Stubble tillage considers several indicators such as the tools used, and the number of passes allocated per year for the stubble cultivation. These indicators however can vary along the four preceding years that are considered in the model. Therefore, it is not possible to assume a generic average stubble cultivation. The choice here was to consider each year only the stubble tillage that involves at least three repetitions between the harvest of the previous crop and the sowing of the new one. The number of stubble tillage per year that reach these conditions are counted and will enable to qualify the stubble tillage effectiveness. That way, all the information needed for the model is complete and the input requirement is simplified by omitting all the situations where “wrong” tools are used or the number of passes is too low. Plowing effectiveness considers the number of years that at least one inversion tillage is performed along the four preceding years. The IPSIM-Cirsium model has 33 attributes, of which are 13 aggregated and 20 basic attributes.
Using weight calculation of attributes, each cropping practice and pedoclimate indicator can be described alone according to their importance to evaluate weed infestation. IPSIM-Cirsium, expert and literature-based model correctly reflects the knowledge available to build the model. Cropping practices were chosen to be more relevant in the explanation of Cirsium arvense than the environment of the field. This choice was supported both by literature and expert knowledge. Therefore, whenever cropping practices were rated as Ineffective to control Cirsium arvense, a mild or Favorable climate for the user did not influence the risk of weed infestation that was rated High already. Environment was thus accredited to a low weight by DEXi software, explained by the low number of rules directly influenced by the grade of its scale. The local normalized weights of Environment and Cropping practices are 33 and 67% respectively (Table 3).
Both herbicide control and competition control, by means of the use of ley, for example, enable a “cleaning” of the field by their curative aspect. These two methods are often chosen as the most effective practices to control C. arvense on a short-term basis. On the other hand, mechanical control of C. arvense is described as a method that will keep a constant pressure on this weed and more particularly on its sprouting capacity by exhausting root reserve. Therefore, Competition, Herbicide, and Mechanical control have a local normalized weight of 30, 35 and 35%, respectively. These weights match the perception of the expert's knowledge.
The use of converters was needed for each input attribute except for the compaction of soil, which is qualitatively evaluated according to the observer. All the converters were simple: a book of rules (Table 4 shows an example for Cover crop or ley termination converter rules) is written to describe each possible entry for the user. For each variable, quantitative (e.g., Temperature) or qualitative (e.g., Cover crop or ley termination), a qualitative value is associated to be directly used by the model. For some input attributes, regional context was important. Therefore, a regional threshold had to be specified for each location where the model is to be used. For example, the Sowing density is evaluated according to regional recommendations. This converter use quantitative references established by [Arvalis (2020); example on wheat sowing density in Centre region, France]. Converters are designed to have a certain genericity and apply to any pedoclimates and cropping practices. Some converters tackle several effects of the considered attribute. For example, Sown crop evaluate the competitiveness of the crop with a three-level scale: Closed canopy, Moderately closed canopy, Open canopy. To establish this scale, several components of the crop were studied: weed biomass (Gruber and Claupein, 2009; Thomsen et al., 2015), architecture of the plant (Edwards et al., 2000; Lukashyk et al., 2008), and growing speed (Weill, 2015, 2018).
By means of the large dataset, gathering many sites and years (220 situations) with a wide diversity of cropping practices and pedoclimates, it was possible to perform a reliable evaluation of the predictive quality of the model. Calculated values of infestation were very similar to the observed values in field, resulting in a satisfactory evaluation (78.2% of the values were correctly calculated). Figure 4 illustrates the confusion matrix between observed and calculated values of weed infestation. However, square weighted Cohen's kappa reached 0.543, meaning that slightly more than half of the variability of the observed values were explained by IPSIM-Cirsium. Here, kappa interprets the strength of agreement between calculated and observed values as moderate (Landis and Koch, 1977; Altman, 1999). Statistical results are presented in Table 5. The evaluation of the predictive quality of the model at the class-scale was less satisfactory. Very low infestation was the best evaluated class with 90% of correctly calculated values in this class (Table 5), followed by High infestation with 42% of correctly calculated values. However, Low infestation and Intermediate infestation obtained a F1-score of only 11 and 11%, respectively. It can be due to the low number of observations of Low infestation and Intermediate infestation, representing 5 and 3% of the observations, respectively; or it can also be due to a low predictive quality of the model. IPSIM-Cirsium seems to struggle with the evaluation of weed infestation from 1 to 7 shoots/m2 (Low infestation and Intermediate infestation).
Figure 4. Confusion Matrix of IPSIM-Cirsium. Color of cells are determined with the level of difference between calculated and observed values of weed infestation: Green cells are perfectly matched (0 level of difference), Yellow cells have one level of difference, Orange cells have two levels of difference, and Red cells have three level of differences expressing the furthest distance between calculated and observed values of weed infestation.
Agroecological management of pests relies on high complexity level systems. Agroecosystems require two integrations: a horizontal integration of the numerous populations of pests and a vertical integration of several combined management methods of pests (Aubertot et al., 2005; Malard et al., 2020). IPSIM-Cirsium only tackles the vertical integration of practices to control specifically C. arvense. The combination of partial effects practices and the interaction of C. arvense with the environment of the agroecosystem are the main bases of the agroecological management of pests. However, the impact of the combination of practices on pests is difficult to quantify because of the diversity and complexity of interactions of cropping practices, pedoclimate and field environment. It appears difficult to take all the possible interactions into account for the evaluation of C. arvense infestation.
Qualitative modeling approach enables the inclusion of numerous cropping practices, pedoclimates and field environments while considering their interactions. The DEX method used in the modeling approach permits to solve a complex decision problem by the evaluation of many simpler sub-problems. Furthermore, qualitative modeling is well suited to grasp large complex systems by reducing the complexity level of each attribute into a three or two levels scale. Integrated weed management gathers many cropping practices from soil cultivation to choice of sown crops (Rasmussen, 2011). It is important to focus on the aspect of each method that will determine its effectiveness (e.g., number of tillage instead of the type of tool used for cultivation) and to simplify it to a qualitative variable with a three- or two-level scale, i.e., Effective, Moderately effective, Ineffective. The interactions of cropping practices, pedoclimates and field environments are then easier to characterize with a defined number of rules according to aggregating tables. The IPSIM method rather focus on the accuracy of the model than on its precision (Aubertot and Robin, 2013).
The accuracy of IPSIM-Cirsium is 0.78, making IPSIM-Cirsium a highly accurate model of infestation of C. arvense. The precision of each control method alone is relatively low with a description of each control method made according to a single attribute (except for the description of ley and cover crop use, and competitiveness of crop), but the interactions between cropping practices, pedoclimate and field environment is well described. Attributes were first described with all the information available and then were simplified to a maximum to better discretize the multiplicity of complex interactions between attributes. Stubble tillage was for example hard to define because many factors impact its effectiveness (e.g., Weather after cultivation, Number of passes, Choice of tools, Depth of tools, etc.). Further, factors such as Choice of tools are described as non-significative (Moulin, 2011) or with marginal effect. Stubble tillage can then be simplified to the number of passes only. Simplifications of attributes might be seen as responsible for a reduction in accuracy of the model, by neglecting variability of the effectiveness of cropping practices, pedoclimates or field environments. Nevertheless, to widely integrate the vertical dimension of C. arvense control, it is necessary to tackle a large panel of control methods merely described.
Cirsium arvense, as other perennial weeds is hardly manageable on a single year and requires a long-term approach to tackle a massive infestation (Weill, 2018). The IPSIM approach permits to take into account several years-factors. Some adaptations can be done by considering cropping practices on a wider temporal window and characterizing these cropping practices as Favorable, Moderately favorable or Unfavorable to weed control. This approach was applied for many cropping practices to ensure that the effectiveness of the practice was correctly evaluated in regard to the previous year's practices. Indeed, considering long term methods such as Stubble tillage on a 2-year period would have been marred with errors. Stubble tillage on perennial weeds is effective only after 2–3 years (Régis Hélias, personal communication, April 28, 2020), and needs to be repeated several years to reduce the population of perennial weeds. Therefore, stubble tillage was not here considered as a curative method in a year, but as a proper control method to maintain low level of infestation, planned for several years in the crop sequence. Control methods such as the introduction of Ley in the crop sequence were also implemented in IPSIM-Cirsium and ensure the possibility to plan a control strategy of Cirsium arvense at the cropping system scale. Crop sequence on its own is not considered by the model. Only the current crop and the ley period in the crop sequence are considered.
IPSIM-Cirsium is a static deterministic model and is designed to be used on a single year to appreciate the infestation of Cirsium arvense in June, corresponding to the highest infestation of Cirsium arvense of the year. However, adaptations are possible here because the model is considering practices during the 4 years preceding the infestation evaluation. A visualization of the infestation as a function of the crop sequence to focus on “critical years”, where level of infestation can increase according to “improper” cropping practices or decrease with effective cropping practices. It is interesting to consider a larger lapse of time than just one year to evaluate a system and find its weakness regarding weed management. Indeed, some crops require cropping practices that are not suitable for perennial weed management (e.g., Canada thistle is more easily controlled with a long bare soil period in summer, where many stubble cultivation passes can be performed). Using an effective herbicide on C. arvense can also be jeopardized with the sowing of crops, where authorized use herbicide is limited or absent (MacLaren et al., 2021). With an evaluation on a longer scale, we can focus on the years presenting a weakness due to improper cropping practices resulting from crops or pedoclimates and better anticipate and build the crop sequence to maintain a low level of weed pressure in the field.
IPSIM models are designed according to a large, detailed literature on one or several pests, to provide significant factors as indicators of the pest infestation level. Scientific consensus according to literature is often hard to obtain and leads to generalizing a specific information. The most dangerous generalization is the regional bias. In the building of IPSIM-Cirsium, Cirsium arvense genotypes were considered as identical, no matter the region it was observed. This hypothesis can lead to many mistakes; indeed, weeds are known to have different genotypes according to different episodes for invasive species or recombination (Gaskin et al., 2013). Considering two different genotypes can lead to uncertainties, such as thresholds for temperature or rainfall. The evolution of weed populations would be conditioned by its environment and would lead to different thermal time need for germination, for example. The response to cropping practices can also change between region and the evolution history of the considered genotype. The genericity of the model therefore suffers from few limitations to be applied in other regions of the world. Adjustments have to be made according to regional conditions.
This kind of mistake can be observed in the research of literature and parameterization of factors for the model, but also during co-design workshops with experts. Indeed, expert-based models rely on the experience of the experts involved. This experience can be affected by subjectivity of the expert and of the designers of the model. Expert knowledge will be conditioned by their experience, in a particular region with its pedoclimate or in a particular cropping system. Therefore, experts are also encountering non-consensus. It is important to have a wide diversity of experts to avoid this regional and system bias. Subjectivity of experts can also be observed when many factors are compared. It is hard for experts to consider a wide range of cropping practices or pedoclimates, and to consider their interactions to explain the output of the model. Hierarchical construction here helps us lower the level of complexity for each interaction by only considering interaction between attributes aggregated together. However, three attributes aggregated together, each having three levels per scale, leads to 33 = 27 aggregating table rules to define. This kind of large consideration of attributes must be avoided at maximum to minimize uncertainties.
Cirsium arvense has a distribution of patches in the field and present therefore a high heterogeneity of weed infestation level, except for low infestations where the level is homogeneously low among the field. It is hard to define a general level of threat or infestation of the weed, according to the observations of densities of Cirsium arvense at some punctual surveys in the field. Our first approach was to assess an average density of Cirsium arvense, considering the patches and the untouched areas. However, many uncertainties might come from this approach, and the average value might underestimate the infestation and the high density in patches, reducing drastically the yield in these areas. One of the methods for the evaluation of the weed infestation was to consider the distribution of Cirsium arvense among the field. This approach is addressed to tackle the heterogeneity of the distribution of Cirsium arvense. However, this approach is more complicated to apply for the user without a high number of observations in the field.
Crop losses due to weeds can be quantified according to the harmfulness of the weed in the field. However, to be able to express weed harmfulness in the field, it is necessary to describe its spatial distribution in the field according to patches for C. arvense. A relationship between the mapping of Cirsium arvense shoots and their impact on yield loss has been established for a few specific crops (Gee and Denimal, 2020; Rasmussen and Nielsen, 2020). Representing the patches of Cirsium arvense is not possible in IPSIM-Cirsium and the choice of representation of the infestation was done according to weed pressure. Weed pressure was evaluated by the mean value of all the density of Cirsium arvense observed in the field. To ensure the correct use of IPSIM-Cirsium, Initial level of infestation observed the year preceding the evaluation, it is necessary to assess an average density of Cirsium arvense according to the method widely used for weed pressure calculation in data collection protocol [Chicouène method; (Chicouene and Arbiotech, 2000); random quadrat collection, etc.]. This requirement can be a limit for the accuracy of the prediction of weed infestation by IPSIM-Cirsium. Still, it is possible for the user of the model to provide a qualitative value of the level of initial infestation without using a quantitative value converted into a qualitative value. This qualitative estimation might bring a bias of subjectivity related to the user appreciation of the infestation severity. The use of Initial level of infestation is a strength for the accuracy of the model, but it requires data that are sometimes difficult to obtain, which is a drawback of the model.
The output of the model aims at evaluating weed infestation in June. This is particularly relevant to characterize weed infestation in a French commercial field because it was mainly designed with the help of French experts and farmers. However, IPSIM-Cirsium lacks genericity in the yearly period considered. IPSIM-Cirsium considers first March, April and May temperature and rainfall, which are linked to the emergence of C. arvense in France, thereby evaluate a risk of infestation in June. This bias needs to be corrected for each country to consider three months of temperature and rainfall after the beginning of emergence of C. arvense. The evaluation of weed infestation is calculated for the fourth month following emergence of C. arvense. One way to calculate the time of emergence is to focus on thermal time (Donald, 2000). Here, the choice to use a specific month instead of the emergence month of C. arvense was done to simplify the model and to evaluate its predictive quality in French conditions. Furthermore, climate change might alter the phenology of C. arvense which could lead to an overestimation of the favorable mean temperature for its development. In case of new adaptations of the weed to temperature raise, or increase of drought frequency, the model structure, or its parameters, would have to be adapted.
Moreover, the specification of the output should be adapted to each type of user in order to provide an adequate level of complexity. A lot of information is available for the user of the model, from the infestation of C. arvense to the level of risk of increase of the weed population, detailed by cropping practices. The choice of information to communicate should be adaptable to the requirements of the user. Currently, the model provides an answer of Cirsium arvense infestation in June, detailed in four levels, and a grade for practices and field environment, which are ranked Unfavorable, Moderately favorable, or Favorable for the user. The model output enables the user to access his farming practices effectiveness and his environment's impact on the growth of Cirsium arvense.
IPSIM-Cirsium can be used to test and evaluate ex-ante several cropping systems on their C. arvense management on a specific crop or combination of crops. IPSIM-Cirsium, giving an infestation level, can be used as an indicator of the functioning of agroecosystems, for farmers, advisers or in experimental systems less reliant on herbicide and intensive plowing. The information of weed pressure that can be expected in June is a major information for farmers to better anticipate and tackle the issue of weed population increase. Used ex-ante, this tool enables farmers to adapt their cropping practices to the field environment and pedoclimate to try to reduce crop losses. According to their initial level of infestation, farmers can choose cropping practices that might reduce the risk of weed infestation or keep it under an acceptable level, in their specific conditions.
Ex-post evaluation can also be used by means of IPSIM-Cirsium to better understand and analyze the functioning of current agroecosystems, in experimental or commercial fields. This ex-post evaluation enables an understanding of the strengths and weaknesses of the current cropping practices by spotting the effective combination of factors that reduce the level of infestation of Canada thistle and the combinations that might enhance Canada thistle population, in a specific production situation (Aubertot and Robin, 2013). IPSIM-Cirsium can therefore be an important tool in the decisions of the selected control methods of C. arvense for farmers and advisers.
Qualitative modeling enables users to understand the level of complexity of the considered agroecosystem. According to the multi-attribute approach of IPSIM-method models, many factors of cropping practices and pedoclimates are considered. The DEX method allows the description of all interactions between cropping practices. To control Cirsium arvense without herbicide, it is necessary to combine several control methods such as mechanical control, introduction of ley, or increase of competitiveness of the crop, planned for several years. These non-chemical methods are often providing low effectiveness to control Cirsium arvense and need to be seen as “many little hammers” methods. One use of this model is to provide a general picture of the effects of interactions of these only partly effective methods and the environment.
Multi-attribute hierarchical modeling in DEXi software perfectly fits the understanding of the complexity of agroecosystems, by reducing factors to only two to three scale levels. This approach greatly simplifies the conception of innovative agroecosystems by focusing on cropping practices that are directly described as efficient or not, depending on the chosen intensity of the implemented method. The interactions of simplified cropping practices are then described in aggregating tables, giving a new value to the aggregated attribute such as Mechanical control of Cirsium arvense. This value provides to the user a direct indicator of performance of the considered aggregated cropping practice. It is easier for the user to consider all the cropping practices instead of focusing on the improvement of one single practice that may not be sufficient to control weeds, even at high intensity. For example, it is not advised to perform every year only stubble tillage without inversion tillage to control C. arvense (Melander et al., 2013). IPSIM-Cirsium compiles expert-knowledge on the effect of individual tools and their interactions to manage C. arvense in interaction with pedoclimate conditions, so as to assess coherently design strategies to provide long-term control. Thus, this model gives practical answers to the question of whether or not the efficacy of C. arvense control can be increased through the combined use several non-chemical control methods at the same time, providing an indicator of risk of infestation, and an infestation level according to the initial infestation of the field.
Nonetheless, IPSIM-Cirsium is focused to help the design of agroecosystems less reliant on herbicide. It is not designed to address agronomic objectives such as conservation of soil, maintenance of biodiversity, yield, or economic return. Agroecosystem must be designed taking into account multiple objectives, which are not taken into account in this model. IPSIM-Cirsium can just provide an indicator of Cirsium arvense risk of infestation according to cropping practices and the considered production situation.
Model designing through co-design workshops emphasizes the need of knowledge transfer between agricultural actors. IPSIM-Cirsium was designed for farmers, technicians and advisers to evaluate ex-post or ex-ante weed infestation of a field, to develop innovative agroecosystems less reliant on herbicide. However, it can also be seen as an education tool for teachers and students in agriculture. In addition, this model can be seen as a communication educational tool for large groups of farmers, advisers, practitioners or students. IPSIM-Cirsium presents information in a user-friendly way through a range of colors, easily understood (i.e., from green being Favorable to the user, to red being Unfavorable to the user). The strength of this tool is its ability to transfer information and knowledge between actors of various fields, offering a support for interaction and communication between them.
IPSIM-Cirsium was built to represent specifically the infestation of Cirsium arvense according to cropping practices, pedoclimate and field environment. However, Cirsium arvense is not the only perennial weed that farmers are faced with. Two other perennial models have been built following the IPSIM method for Sonchus arvensis and Elytrigia repens evaluating their infestation levels according to cropping practices, pedoclimate and field environment. A first step to try to understand the perennial weed infestation of the field would be to combine these three qualitative models into a stand-alone model to represent an injury profile. This approach was first foreseen in the evaluation of severity of pests on wheat by Aubertot and Robin (2013). However, this approach implies to understand interactions between perennial weeds. Indeed, the three weeds here can benefit, ignore or suffer from the presence of other weeds. In order to grasp the interactions between weeds, additional aggregating tables would be required. In this multiple perennial weed approach, we would better take into account the horizontal dimension of agroecology.
Cirsium arvense, Sonchus arvensis and Elytrigia repens are not the only perennial weeds that can be found in an agroecosystem. Regrowth capacity according to the root reserve is not a specificity of Cirsium arvense. Therefore, it is important to aim at the generic traits that might distinguish two weeds from each other and describe weeds most efficiently. With accurate and specific traits, it would be possible to suggest a model that takes into account the response to pedoclimate, field environment and cropping practices. This trait modeling approach would not try to approach the assumption of plant diversity and ecosystem services of a field in response to pedoclimate and cropping practices, as many models are (Sande et al., 2017; Teixeira et al., 2021). This approach differs here with the use of traits as an input of the model to describe the pedoclimate and cropping practices that will reduce or enhance the weed infestation of one specific weed. This approach does not tend to represent weed ecology, but only the management effectiveness of one weed at a time. One of the main issues of developing a generic traits approach of weed management is the different thresholds of weed infestation levels. While keeping a qualitative modeling approach might help maintain an accurate evaluation of weed infestation by offering ranks of severity rate for each weed infestation, the use of convertors to describe this qualitative value into quantitative value such as abundance or biomass, might be a different kettle of fish.
The original contributions presented in the study are included in the article/supplementary material, further inquiries can be directed to the corresponding author/s.
J-NA and M-HR designed the study and funded the research. OL, M-HR, and SC reviewed the literature on Cirsium arvense. OL led the workshops with experts and designed the IPSIM-Cirsium model based on principles developed by J-NA, M-HR, and MB. Field data were collected and gathered by SC. OL, J-NA, and DC analyzed the data. All authors were involved in the interpretation of the results and contributed to writing the original version of the manuscript and improving the subsequent ones.
This work was supported by the European Union: ERA-NET-Cofund on Sustainable Crop Production, SusCrop as funder, in the framework of AC/DC-weeds project. MB acknowledges the financial support from the Slovenian Research Agency, research core funding P2-0103.
The authors declare that the research was conducted in the absence of any commercial or financial relationships that could be construed as a potential conflict of interest.
Authors would like to thank Guillaume Adeux, Nicolas Munier-Jolain, Dominique Meunier from UMR Agroécologie (INRAE, Dijon) who collected/synthesize Cirsium data in the U2E of Epoisses (https://doi.org/10.15454/1.5483251081186758E12), Margaux Thirard from the Agricultural Chamber of Eure-et-Loir (France) who collected and synthesized data from Sours, France and Régis Hélias from Arvalis (France), who collected and synthesized data from Salvagnac, France. The authors would like to thank the experts mobilized for the design of the model: Alain Rodriguez (ACTA, France); Claire Cros (Agrotransfert, France); Bruno Chauvel, Muriel Valantin-Morison (INRAE, France); Catherine Vacher, Jean-Luc Verdier, Ludovic Bonin (Arvalis, France); Fanny Vuillemin (Terres Inovia, France); Enguerrand Burel (CREAB, France); Jean Arino (Agriculture Chamber of Gers, France); Bärbel Gerowitt, Marian Malte Weigel, Sabine Andert, Han Zhang (Universität Rostock, Germany); Lars Olav Brandsæter (Norwegian University of Life Sciences, Norway); Kirsten Tørresen, Björn Ringselle (Norwegian Institute of Bioeconomy Research, Norway); Jukka Salonen, Timo Lötjönen (Natural Resources Institute Finland, Finland); and Jesper Rasmussen (University of Copenhagen, Denmark).
Adeux, G., Giuliano, S., Cordeau, S., Savoie, J.-M., and Alletto, L. (2017). Low-input maize-based cropping systems implementing IWM match conventional maize monoculture productivity and weed control. Agriculture 7:74. doi: 10.3390/agriculture7090074
Adeux, G., Munier-Jolain, N., Meunier, D., Farcy, P., Carlesi, S., Barberi, P., et al. (2019). Diversified grain-based cropping systems provide long-term weed control while limiting herbicide use and yield losses. Agron. Sustain. Dev. 39:42. doi: 10.1007/s13593-019-0587-x
Armengot, L., Berner, A., Blanco-Moreno, J. M., Mäder, P., and Sans, F. X. (2015). Long-term feasibility of reduced tillage in organic farming. Agron. Sustain. Dev. 35, 339–346. doi: 10.1007/s13593-014-0249-y
Arvalis (2020). Déterminer la densité de semis du blé tendre 2020/2021 en Centre. Available online at: https://www.arvalis-infos.fr/ble-tendre-a-quelle-densite-semer–@/view-33421-arvarticle.html (accessed December 9, 2020).
Aubertot, J.-N., Barbier, J. M., Carpentier, A., Gril, J.-N., Guichard, L., Lucas, P., et al. (2005). Pesticides, Agriculture and the Environment. Reducing the Use of Pesticides and Limiting Their Environmental Impact. INRA-CEMAGREF. Available online at: https://hal.archives-ouvertes.fr/hal-01173732 (accessed March 2, 2021).
Aubertot, J.-N., and Robin, M.-H. (2013). Injury profile SIMulator, a qualitative aggregative modelling framework to predict crop injury profile as a function of cropping practices, and the abiotic and biotic environment. I. Conceptual Bases. PLoS ONE 8:e73202. doi: 10.1371/journal.pone.0073202
Bohanec, M. (2020). DEXi: Program for Multi-Attribute Decision Making, User's Manual, Version 5.04. IJS Report DP-13100. Available online at: http://kt.ijs.si/MarkoBohanec/pub/DEXiManual504p.pdf (accessed August 19, 2020).
Bostock, S. J. (1978). Seed germination strategies of five perennial weeds. Oecologia 36, 113–126. doi: 10.1007/BF00344576
Brandsæter Bakken, A. K., Mangerud, K., Riley, H., Eltun, R., and Fykse, H. (2011). Effects of tractor weight, wheel placement and depth of ploughing on the infestation of perennial weeds in organically farmed cereals. Eur. J. Agron. 34, 239–246. doi: 10.1016/j.eja.2011.02.001
Brandsæter, L. O., Goul Thomsen, M., Wærnhus, K., and Fykse, H. (2012). Effects of repeated clover undersowing in spring cereals and stubble treatments in autumn on Elymus repens, Sonchus arvensis and Cirsium arvense. Crop Protect. 32, 104–110. doi: 10.1016/j.cropro.2011.09.022
Brandsæter, L. O., Mangerud, K., Helgheim, M., and Berge, T. W. (2017). Control of perennial weeds in spring cereals through stubble cultivation and mouldboard ploughing during autumn or spring. Crop Protect. 98, 16–23. doi: 10.1016/j.cropro.2017.03.006
Campiglia, E., Radicetti, E., and Mancinelli, R. (2012). Weed control strategies and yield response in a pepper crop (Capsicum annuum L.) mulched with hairy vetch (Vicia villosa Roth.) and oat (Avena sativa L.) residues. Crop Protect. 33, 65–73. doi: 10.1016/j.cropro.2011.09.016
Chauvel, B., Guillemin, J.-P., Gasquez, J., and Gauvrit, C. (2012). History of chemical weeding from 1944 to 2011 in France: changes and evolution of herbicide molecules. Crop Protect. 42, 320–326. doi: 10.1016/j.cropro.2012.07.011
Chicouene, D., and Arbiotech, B. (2000). Methods for Assessing the Density of a Weed Peuplement Within a Field: Streamlined Procedures. Phytoma La Defense des Vegetaux (France). Available online at: https://agris.fao.org/agris-search/search.do?recordID=FR2000003727 (accessed January 12, 2021).
Cohen, J. (1960). A coefficient of agreement for nominal scales. Educ. Psychol. Meas. doi: 10.1177/001316446002000104
Colbach, N., Colas, F., Cordeau, S., Maillot, T., Queyrel, W., Villerd, J., et al. (2021). The FLORSYS crop-weed canopy model, a tool to investigate and promote agroecological weed management. Field Crops Res. 261:108006. doi: 10.1016/j.fcr.2020.108006
Davis, S., Mangold, J., Menalled, F., Orloff, N., Miller, Z., and Lehnhoff, E. (2018). A meta-analysis of Canada Thistle (Cirsium arvense) management. Weed Sci. 66, 548–557. doi: 10.1017/wsc.2018.6
Donald, W. W. (1990). Management and control of Canada thistle (Cirsium arvense). Rev. Weed Sci. 5, 193–249.
Donald, W. W. (2000). A degree-day model of Cirsium arvense shoot emergence from adventitious root buds in spring. Wees 48, 333–341. doi: 10.1614/0043-1745(2000)048(0333:ADDMOC)2.0.CO
Donald, W. W., and Khan, M. (1996). Canada thistle (Cirsium arvense) effects on yield components of spring wheat (Triticum aestivum). Weed Sci. 44, 114–121. doi: 10.1017/S0043174500093632
Edwards, G. R., Bourdôt, G. W., and Crawley, M. J. (2000). Influence of herbivory, competition and soil fertility on the abundance of Cirsium arvense in acid grassland. J. Appl. Ecol. 37, 321–334. doi: 10.1046/j.1365-2664.2000.00495.x
Favrelière (2019). Gestion des adventices vivaces en Agriculture Biologique. Agro Transfert. Available online at: http://www.agro-transfert-rt.org/wp-content/uploads/2019/10/Gestion-des-adventices-vivaces-en-AB-oct-19.pdf (accessed July 23, 2020).
Favrelière, E., Ronceux, A., Pernel, J., and Meynard, J.-M. (2020). Nonchemical control of a perennial weed, Cirsium arvense, in arable cropping systems. A review. Agron. Sustain. Dev. 40:31. doi: 10.1007/s13593-020-00635-2
Favrelière, E., Ronceux, A., Pernel, J., and Rodriguez, A. (2016). Développement d'un outil d'aide à la décision pour la gestion du chardon des champs (Cirsium arvense) en systèmes de culture biologiques. Available online at: http://www.agro-transfert-rt.org/wp-content/uploads/2017/01/COLUMA-2016-outil-chardon_VF_FAVRELIERE_web.pdf (accessed December 11, 2020).
Fleiss, J. L., and Cohen, J. (1973). The equivalence of weighted kappa and the intraclass correlation coefficient as measures of reliability. Educ. Psychol. Meas. 33, 613–619. doi: 10.1177/001316447303300309
Gaskin, J. F., Schwarzlander, M., Hinz, H. L., Williams, L., Gerber, E., Rector, B. G., et al. (2013). Genetic identity and diversity of perennial pepperweed (Lepidium latifolium) in its native and invaded ranges. Invasive Plant Sci. Manag. 6, 268–280. doi: 10.1614/IPSM-D-12-00075.1
Gee, C., and Denimal, E. (2020). RGB image-derived indicators for spatial assessment of the impact of broadleaf weeds on wheat biomass. Remote Sens. 12:2982. doi: 10.3390/rs12182982
Graglia, E., Melander, B., and Jensen, R. K. (2006). Mechanical and cultural strategies to control Cirsium arvense in organic arable cropping systems. Weed Res. 46, 304–312. doi: 10.1111/j.1365-3180.2006.00514.x
Gruber, S., and Claupein, W. (2009). Effect of tillage intensity on weed infestation in organic farming. Soil Tillage Res. 105, 104–111. doi: 10.1016/j.still.2009.06.001
Hamdoun, A. M. (1972). Regenerative capacity of root fragments of cirsium arvense (l.) Scop. Weed Res. 12, 128–136. doi: 10.1111/j.1365-3180.1972.tb01196.x
Hansen, A. A. (1918). Canada Thistle and Methods of Eradication. Washington D.C.: U.S. Department of Agriculture.
Hausman, C. E., Jaeger, J. F., and Rocha, O. J. (2010). Impacts of the emerald ash borer (EAB) eradication and tree mortality: potential for a secondary spread of invasive plant species. Biol. Invasions 12, 2013–2023. doi: 10.1007/s10530-009-9604-3
Hochstrasse, M., Meerstetter, A., and Popow, G. (2012). Lutte contre le chardon des champs. Agridea: Nature et agriculture 1733. Available at: https://www.agridea.ch/old/fr/publications/publications/production-vegetale-environnement/faune-et-flore/lutte-contre-le-chardon-des-champs/ (accessed March 12, 2020).
Hodgson, J. M. (1968). The nature, ecology, and control of Canada thistle. Available at: https://core.ac.uk/display/66219670 (accessed August 19, 2020).
Hume, L. (1982). The long-term effects of fertilizer application and three rotations on weed communities in wheat (after 21–22 years at Indian Head, Saskatchewan). Can. J. Plant Sci. 62, 741–750. doi: 10.4141/cjps82-107
Landis, J. R., and Koch, G. G. (1977). An application of hierarchical Kappa-type statistics in the assessment of majority agreement among multiple observers. Biometrics 33, 363–374. doi: 10.2307/2529786
Liebman, M., Gallandt, E. R., and Jackson, L. (1997). “Many little hammers: ecological management of crop-weed interactions,” in Ecology in Agriculture, ed L. E. Jackson (San Diego, CA: Academic Press), 291–343. doi: 10.1016/B978-012378260-1/50010-5
Liew, J., Andersson, L., Boström, U., Forkman, J., Hakman, I., and Magnuski, E. (2012). Influence of temperature and photoperiod on sprouting capacity of Cirsium arvense and Sonchus arvensis root buds. Weed Res. 52, 449–457. doi: 10.1111/j.1365-3180.2012.00936.x
Líška, E., Hunková, E., and Demjanová, E. (2007). Creeping thistle (Cirsium arvense (L.) Scop.) - an important competitor of nutrients consumption in grain maize stands (Zea mays L.). J. Cent. Eur. Agric. 8, 461–468.
Liu, C., Scursoni, J. A., Moreno, R., Zelaya, I. A., Sol Munoz, M., and Kaundun, S. S. (2019). An individual-based model of seed- and rhizome-propagated perennial plant species and sustainable management of Sorghum halepense in soybean production systems in Argentina. Ecol. Evol. 9, 10017–10028. doi: 10.1002/ece3.5578
Lukashyk, P., Berg, M., and Köpke, U. (2008). Strategies to control Canada thistle (Cirsium arvense) under organic farming conditions. Renew. Agric. Food Syst. 23, 13–18. doi: 10.1017/S1742170507002013
MacLaren, C., Labuschagne, J., and Swanepoel, P. A. (2021). Tillage practices affect weeds differently in monoculture vs. crop rotation. Soil Tillage Res. 205:104795. doi: 10.1016/j.still.2020.104795
Malard, J. J., Adamowski, J. F., Diaz, M. R., Nassar, J. B., Anandaraja, N., Tuy, H., et al. (2020). Agroecological food web modelling to evaluate and design organic and conventional agricultural systems. Ecol. Model. 421:108961. doi: 10.1016/j.ecolmodel.2020.108961
McHugh, M. L. (2012). Interrater reliability: the kappa statistic. Biochem. Med. 22, 276–282. doi: 10.11613/BM.2012.031
Melander, B., Holst, N., Rasmussen, I. A., and Hansen, P. K. (2012). Direct control of perennial weeds between crops—implications for organic farming. Crop Protect. 40, 36–42. doi: 10.1016/j.cropro.2012.04.029
Melander, B., Munier-Jolain, N., Charles, R., Wirth, J., Schwarz, J., van der Weide, R., et al. (2013). European perspectives on the adoption of nonchemical weed management in reduced-tillage systems for arable crops. Weed Technol. 27, 231–240. doi: 10.1614/WT-D-12-00066.1
Mézière, D., Petit, S., Granger, S., Biju-Duval, L., and Colbach, N. (2015). Developing a set of simulation-based indicators to assess harmfulness and contribution to biodiversity of weed communities in cropping systems. Ecol. Indicat. 48, 157–170. doi: 10.1016/j.ecolind.2014.07.028
Miller, T. W. (2016). Integrated strategies for management of perennial weeds. Invasive Plant Sci. Manag. 9, 148–159. doi: 10.1614/IPSM-D-15-00037.1
Moulin, V. (2011). Maîtriser le chardon des champs (Cirsium arvense) en agriculture biologique. Centre et Ile-de-France.
Nguwi, Y.-Y., and Cho, S.-Y. (2010). An unsupervised self-organizing learning with support vector ranking for imbalanced datasets. Exp. Syst. Appl. 37, 8303–8312. doi: 10.1016/j.eswa.2010.05.054
Pekrun, C., and Claupein, W. (2004). The effect of stubble tillage and primary tillage on population dynamics of Canada Thistle (Cirsium arvense) in organic farming. J. Plant Dis. Protect. Special Issue XIX, 483–490.
Powles, S. B., and Yu, Q. (2010). “Evolution in action: plants resistant to herbicides,” in Annual Review of Plant Biology, Vol. 61, eds. S. Merchant, W. R. Briggs, and D. Ort (Palo Alto: Annual Reviews), 317–347. doi: 10.1146/annurev-arplant-042809-112119
Rasmussen (2011). “Use of competitive crops to reduce Cirsium arvense. organic is life—knowledge for tomorrow. Volume 1—organic crop production,” Proceedings of the Third Scientific Conference of the International Society of Organic Agriculture Research (ISOFAR), held at the 17th IFOAM Organic World Congress in cooperation with the International Federation of Organic Agriculture Movements (IFOAM) and the Korean Organizing Committee (KOC), 28. September 1. October 2011 in Namyangju, Korea Republic, 692–695.
Rasmussen, J., and Nielsen, J. (2020). A novel approach to estimating the competitive ability of Cirsium arvense in cereals using unmanned aerial vehicle imagery. Weed Res. 60, 150–160. doi: 10.1111/wre.12402
Sande, M. T., van der Peña-Claros, M., Ascarrunz, N., Arets, E. J. M. M., Licona, J. C., Toledo, M., et al. (2017). Abiotic and biotic drivers of biomass change in a Neotropical forest. J. Ecol. 105, 1223–1234. doi: 10.1111/1365-2745.12756
Schreiber, M. M. (1967). Effect of density and control of Canada Thistle on production and utilization of Alfalfa pasture. Weeds 15, 138–142. doi: 10.2307/4041184
Sciegienka, J., Keren, E., and Menalled, F. (2011). Impact of root fragment dimension, weight, burial depth, and water regime on Cirsium arvense emergence and growth. Can. J. Plant Sci. 91, 1027–1036. doi: 10.4141/cjps2011-059
Stoate, C., Báldi, A., Beja, P., Boatman, N. D., Herzon, I., van Doorn, A., et al. (2009). Ecological impacts of early 21st century agricultural change in Europe —a review. J. Environ. Manag. 91, 22–46. doi: 10.1016/j.jenvman.2009.07.005
Taramarcaz, J. (2019). Ferme Pilote de Mapraz : Bilan 2000-2017. Agridea: Nature et Agriculture. Available online at: https://www.agridea.ch/old/fileadmin/user_upload/Mapraz_Bilan_CHARDONS_2000-2017.pdf (accessed March 12, 2020).
Tavaziva, V. J., Lundkvist, A., and Verwijst, T. (2019). Effects of selective cutting and timing of herbicide application on growth and development of Cirsium arvense in spring barley. Weed Res. 59, 349–356. doi: 10.1111/wre.12371
Teixeira, H. M., Bianchi, F. J. J. A., Cardoso, I. M., Tittonell, P., and Peña-Claros, M. (2021). Impact of agroecological management on plant diversity and soil-based ecosystem services in pasture and coffee systems in the Atlantic forest of Brazil. Agric. Ecosyst. Environ. 305:107171. doi: 10.1016/j.agee.2020.107171
Thomsen, M. G., Mangerud, K., Riley, H., and Brandsæter, L. O. (2015). Method, timing and duration of bare fallow for the control of Cirsium arvense and other creeping perennials. Crop Protect. 77, 31–37. doi: 10.1016/j.cropro.2015.05.020
Tiley, G. E. D. (2010). Biological flora of the British Isles: Cirsium arvense (L.) Scop. J. Ecol. 98, 938–983. doi: 10.1111/j.1365-2745.2010.01678.x
Verwijst, T., Tavaziva, V. J., and Lundkvist, A. (2017). Effects of selective cutting and herbicide use in spring barley on seed production of Cirsium arvense. Acta Agric. Scand. Sect. B Soil Plant Sci. 67, 562–570. doi: 10.1080/09064710.2017.1318164
Weill (2015). Effet de la jachère de printemps sur la répression du chardon, du laiteron et du tussilage. Available online at: https://www.cetab.org/system/files/publications/6_mars_gc_10h15_anne_weill_chardon_laiteron.pdf (accessed March 12, 2020).
Weill, A. (2018). Répression du laiteron des champs, du chardon des champs et du tussilage. Agri Réseau.
Keywords: integrated weed management, Canada thistle, qualitative modeling, injury profile simulator, cropping practices, soil, climate
Citation: Lacroix O, Aubertot J-N, Bohanec M, Cordeau S, Corrales DC and Robin M-H (2021) IPSIM-Cirsium, a Qualitative Expert-Based Model to Predict Infestations of Cirsium arvense. Front. Agron. 3:655383. doi: 10.3389/fagro.2021.655383
Received: 18 January 2021; Accepted: 15 March 2021;
Published: 09 April 2021.
Edited by:
Karla Leigh Gage, Southern Illinois University Carbondale, United StatesReviewed by:
Ilias Travlos, Agricultural University of Athens, GreeceCopyright © 2021 Lacroix, Aubertot, Bohanec, Cordeau, Corrales and Robin. This is an open-access article distributed under the terms of the Creative Commons Attribution License (CC BY). The use, distribution or reproduction in other forums is permitted, provided the original author(s) and the copyright owner(s) are credited and that the original publication in this journal is cited, in accordance with accepted academic practice. No use, distribution or reproduction is permitted which does not comply with these terms.
*Correspondence: Marie-Hélène Robin, bWgucm9iaW5AcHVycGFuLmZy
Disclaimer: All claims expressed in this article are solely those of the authors and do not necessarily represent those of their affiliated organizations, or those of the publisher, the editors and the reviewers. Any product that may be evaluated in this article or claim that may be made by its manufacturer is not guaranteed or endorsed by the publisher.
Research integrity at Frontiers
Learn more about the work of our research integrity team to safeguard the quality of each article we publish.