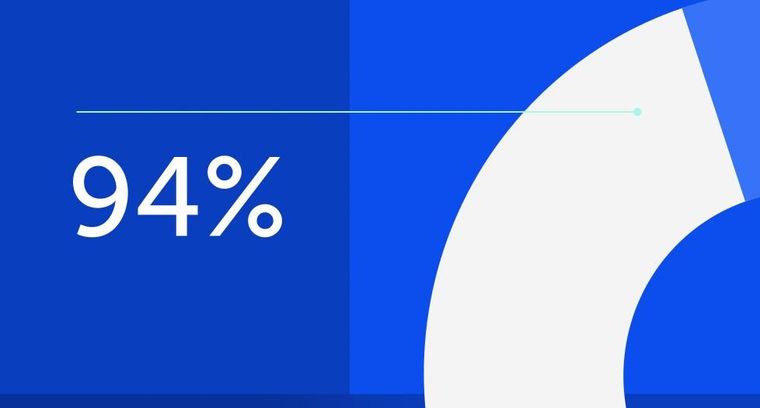
94% of researchers rate our articles as excellent or good
Learn more about the work of our research integrity team to safeguard the quality of each article we publish.
Find out more
ORIGINAL RESEARCH article
Front. Aging
Sec. Musculoskeletal Aging
Volume 6 - 2025 | doi: 10.3389/fragi.2025.1501168
The final, formatted version of the article will be published soon.
Select one of your emails
You have multiple emails registered with Frontiers:
Notify me on publication
Please enter your email address:
If you already have an account, please login
You don't have a Frontiers account ? You can register here
Background: Physical frailty is a pressing public health issue that significantly increases the risk of disability, hospitalization, and mortality. Early and accurate detection of frailty is essential for timely intervention, reducing its widespread impact on healthcare systems, social support networks, and economic stability.Objective: This study aimed to classify frailty status into binary (frail vs. non-frail) and multi-class (frail vs. pre-frail vs. non-frail) categories. The goal was to detect and classify frailty status at a specific point in time. Model development and internal validation were conducted using data from wave 8 of the English Longitudinal Study of Ageing (ELSA), with external validation using wave 6 data to assess model generalizability.Methods: Nine classification algorithms, including Logistic Regression, Random Forest, K-nearest Neighbor, Gradient Boosting, AdaBoost, XGBoost, LightGBM, CatBoost, and Multi-Layer Perceptron, were evaluated and their performance compared.Results: CatBoost demonstrated the best overall performance in binary classification, achieving high recall (0.951), balanced accuracy (0.928), and the lowest Brier score (0.049) on the internal validation set, and maintaining strong performance externally with a recall of 0.950, balanced accuracy of 0.913, and F1-score of 0.951. Multi-class classification was more challenging, with Gradient Boosting emerging as the top model, achieving the highest recall (0.666) and precision (0.663) on the external validation set, with strong F1-score (0.664) and reasonable calibration (Brier Score = 0.223).Conclusions: Machine learning algorithms show promise for the detection of current frailty status, particularly in binary classification. However, distinguishing between frailty subcategories remains challenging, highlighting the need for improved models and feature selection strategies to enhance multi-class classification accuracy.
Keywords: machine learning, Frailty, healthcare, Elderly, Aging, ELSA
Received: 24 Sep 2024; Accepted: 09 Apr 2025.
Copyright: © 2025 Hughes, Zhang, Pourhossein and Jurášová. This is an open-access article distributed under the terms of the Creative Commons Attribution License (CC BY). The use, distribution or reproduction in other forums is permitted, provided the original author(s) or licensor are credited and that the original publication in this journal is cited, in accordance with accepted academic practice. No use, distribution or reproduction is permitted which does not comply with these terms.
* Correspondence: Charmayne Mary Lee Hughes, Technical University of Berlin, Berlin, Germany
Disclaimer: All claims expressed in this article are solely those of the authors and do not necessarily represent those of their affiliated organizations, or those of the publisher, the editors and the reviewers. Any product that may be evaluated in this article or claim that may be made by its manufacturer is not guaranteed or endorsed by the publisher.
Supplementary Material
Research integrity at Frontiers
Learn more about the work of our research integrity team to safeguard the quality of each article we publish.