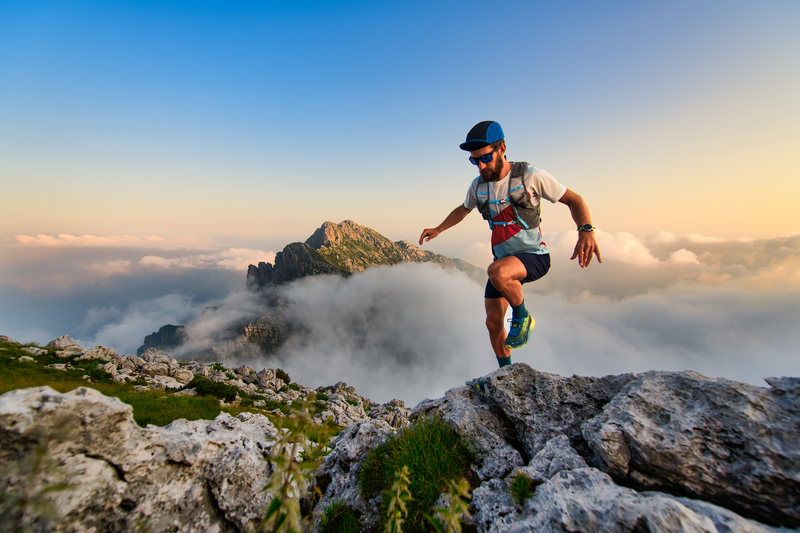
95% of researchers rate our articles as excellent or good
Learn more about the work of our research integrity team to safeguard the quality of each article we publish.
Find out more
REVIEW article
Front. Aging , 24 April 2023
Sec. Genetics, Genomics and Epigenomics of Aging
Volume 4 - 2023 | https://doi.org/10.3389/fragi.2023.1191993
This article is part of the Research Topic Multi-Omics Studies on Aging and Age-related Diseases View all 5 articles
Genomic diversity plays critical roles in risk of disease pathogenesis and diagnosis. While genomic variants—including single nucleotide variants, frameshift variants, and mis-splicing isoforms—are commonly detected at the DNA or RNA level, their translated variant protein or polypeptide products are ultimately the functional units of the associated disease. These products are often released in biofluids and could be leveraged for clinical diagnosis and patient stratification. Recent emergence of integrated analysis of genomics with mass spectrometry-based proteomics for biomarker discovery, also known as proteogenomics, have significantly advanced the understanding disease risk variants, precise medicine, and biomarker discovery. In this review, we discuss variant proteins in the context of cancers and neurodegenerative diseases, outline current and emerging proteogenomic approaches for biomarker discovery, and provide a comprehensive proteogenomic strategy for detection of putative biomarker candidates in human biospecimens. This strategy can be implemented for proteogenomic studies in any field of enquiry. Our review timely addresses the need of biomarkers for aging related diseases.
A biomarker is defined as a biological characteristic that indicates clinically relevant endpoints and outcomes for disease diagnosis, stratification, and/or prognosis (Aronson and Ferner, 2017). To date, biomarkers have been primarily used for early-stage diagnosis, when therapeutic interventions are most effective. Beyond diagnostic applications, biomarkers can also serve as drug targets and proxies of response to treatment. The use of genetic loci as predictive biomarkers has seen a significant advance in recent years, in part due to their high reproducibly and cost-effectiveness which has come with next-generation sequencing (NGS) technology (Schwarze et al., 2018). Disease-based genetics often identifies risk variants associated with diseases, but alone does not provide information on expression at the transcript or protein level. Transcriptome variant markers–such as point mutations, fusion products, and splicing–provide relatively high specificity and sensitivity (Fehse et al., 2000; Janik et al., 2021; Monti et al., 2022). Moreover, while transcriptomics has been widely applied to tissue samples, its application to biofluids is more challenging due to the low quality, quantity, and specificity of RNAs that are recovered from biofluids. The detection of de novo protein biomarkers via antibody and mass spectrometry (MS)-based strategies represents a promising solution (Borrebaeck and Wingren, 2009; Zhou et al., 2017). Although immunoassay-based approaches can analyze several proteins at once, they are limited by the availability of suitable antibodies, while MS is generally “hypothesis-free” and high throughput.
Historically, the fields of genomics and proteomics have evolved independently. “Proteogenomics” was first referred to as the application of MS-based proteomics to complement existing genome annotations (Jaffe et al., 2004). The applications have since become much broader, now encompassing post-translational modifications (PTMs) and integrative modeling of multi-omics data with the advent of robust computational tools (Ruggles et al., 2017). Early proteogenomic applications consisted of evaluating parental proteins and their product peptides to identify and validate informatically predicted open reading frames (ORFs), detect de novo variants, and reveal PTMs. Now, bioinformatics pipelines allow researchers to combine both genomic and proteomic data in their analyses, making so-called “integrated proteogenomics analyses,” more approachable (Ang et al., 2019).
In traditional database search strategies for discovery proteomics, experimental protein identification is predicated on the alignment of experimental mass spectra with reference proteome databases, such as the universal Protein Resource (UniProt) and NCBI Reference Sequence Database (Refseq) (Consortium, 2015; O'Leary et al., 2016). With this approach, protein findings are limited to existing sequences within such databases (Jimmy et al., 1994; Xuemei Han et al., 2008). To identify novel sequences and ORFs, these annotation databases were subsequently expanded with the inclusion of peptide sequences derived from genetically predicted coding regions. However, a number of additional factors, such as translation efficiency and post-transcriptional regulation, complicate the ability to accurately predict biologically relevant peptide products from transcriptional data alone (Schwanhäusser et al., 2011; Vogel and Marcotte, 2012). Additionally, events contributing to the multiplicity of proteoforms, including alternative splicing and PTMs, can be challenging–and at times impossible–to detect at the RNA level (Smith and Kelleher, 2013; Jian et al., 2014). One possible solution is to couple NGS with ultra-high-resolution MS to identify de novo peptides that may serve as promising biomarker candidates (Abecasis, 2010; Ning and Nesvizhskii, 2010; Gargis et al., 2012; Wang et al., 2012; Kamalakaran et al., 2013; Sheynkman et al., 2013; Chrystoja and Diamandis, 2014; Zhang et al., 2019). Disease-specific genomic variants can be identified from high-quality sequencing of disease-relevant tissue samples and used to build customized libraries for peptide biomarker identification via discovery proteomics (Figure 1). Recent success in both integrated proteogenomic analyses as well as variant protein detection is driving biomarker discovery and patient stratification in recent years.
FIGURE 1. Application of proteogenomics in biomarker discovery. Whole-exome sequencing (WES), whole genome sequencing (WGS), and total RNA sequencing of control and patient-derived samples are used to identify canonical, or de novo reads matching to single nucleotide variants (SNVs), short insertions/deletions (indels), mis-splicing, or fusion transcripts. The variant coordinates are integrated within the normal protein sequence to build a custom peptide library. In parallel, discovery proteomics of biofluid samples can be used to build a proteomics database, which can be mined for sequences of interest using the custom-built peptide library. The candidate biomarkers are further validated in large-cohort studies.
Strategies combining genomics and proteomics in the identification of cancer protein biomarkers have perhaps best demonstrated the utility of proteogenomics for biomarker discovery. The Cancer Genome Atlas program (TCGA) represents a rich resource for large-scale genomic data. TCGA comprises more than 30 cancer subtypes and provides data from both cancer and control tissue (Cancer, 2006; Tomczak et al., 2015). By integrating proteomics, the Clinical Proteomic Tumor Analysis Consortium (CPTAC) has sought to expand on this dataset, performing proteomic and PTM analysis on TCGA specimens. This effort has produced robust, multidimensional proteomic datasets of cancer tissue subtypes for groups seeking to conduct integrated proteogenomic analyses (Proteomics Cancer, 2007; Ellis et al., 2013a). Several studies have successfully demonstrated the utility of these datasets in uncovering candidate biomarkers (Rodriguez et al., 2021). For example, Chiou and colleagues successfully used these data to identify S100A9 and GRN as combinatorial biomarkers for early identification of hepatocellular carcinoma (HCC) from urine (Chiou and Lee, 2016). Moreoever, Gillete and colleagues leveraged the CPTAC database to perform proteogenomic characterization of lung adenocarcinoma (LUAD) and normal, adjacent tissue (Gillette et al., 2020). This analysis utilized not only proteomic and PTM data, but also whole-exome sequencing (WES), RNA-sequencing (RNAseq), and DNA methylation analysis, to identify mRNA and peptides derived from somatic mutations as biomarker candidates of LUAD driven by ALK-fusion where fusion proteins EML4-ALK with and HMBOX1-ALK were formed at transcriptome level (Table 1).
Tumor-specific somatic mutations are ideal targets for biomarker development. For example, targeted MS-based detection of mutant KRASp.G12V and KRASp.G12D proteins has proven to be a viable biomarker strategy in colorectal and pancreatic cancers (Wang et al., 2011). In addition to oncogenic mutations, tumors have also been found to contain up to 100 “passenger” mutations, many of which are translated into potentially targetable proteins (Reddy et al., 1982; Wood Laura et al., 2007; Stratton et al., 2009; Bignell et al., 2010; Bozic et al., 2010). Although many disease-associated mutations have been identified over the years, including KRAS (Demory Beckler et al., 2013), P53 (Duffy et al., 2018), and EGFR (Awasthi et al., 2018), the vast heterogeneity of mutation sites not only poses a challenge to forming effective therapies, but also makes the possibility of creating antibodies for each mutation impractical (Leonardi et al., 2012). MS-based proteogenomics is often employed to discover mutant and novel peptides that occur downstream of tumor-specific mutations and hold promise as future biomarker candidates.
Similar to cancer, there is an increasing role for biomarkers of disease characterization and patient stratification in the field of neurodegeneration (DeKosky and Marek, 2003). Despite the fact that there has been limited success in identifying true plasma or cerebrospinal fluid (CSF) biomarkers of neurodegenerative disease thus far (Carlyle et al., 2018), there has been recent, promising progress in this field, assisted by proteogenomic strategies.
Using an integrative proteogenomic pipeline, Li and colleagues successfully identified 496 novel peptides in AD postmortem brain tissue. These identified peptides represent translational products of mutations and mis-splicing events that occur in AD and could serve as putative protein biomarkers (Li et al., 2016a). Applying a proteogenomic approach that was specifically designed to dissect alternative splicing events, Johnson et al. identified modules associated with AD cognitive decline using co-expression network analyses of postmortem brain samples. From these modules, the investigators then identified a number of differentially expressed, novel alternative splice variant proteins (Johnson et al., 2018).
Validation of biomarker candidates through large-scale studies of human samples is an essential component of developing clinical-grade biomarkers. To that end, high-throughput targeted MS-based approaches are often employed to validate findings discovered through companion shotgun proteomics approaches. For example, a targeted proteomics assay was recently used to identify APOE4-specific peptides in the plasma of AD patients (Simon et al., 2012). Expanding on the conventional identification of tau protein for clinical diagnosis of AD, multiple phospho-tau proteins were quantified using targeted proteomics of postmortem brain and CSF from AD patients (Barthelemy et al., 2019). Similarly, exon-specific 4R tau isoform-derived tryptic peptides were successfully quantified by targeted MS in the CSF of patients with Lewy body dementia (Barthelemy et al., 2016).
During the past two decades, several pathological mechanisms of FTD and ALS involving TDP-43, Tau, and SOD1 have been extensively described (Hedl et al., 2019). Mutations in C9orf72, TDP-43, FUS, and VCP have been found to be closely associated with FTD/ALS and represent promising biomarker candidates; however, there is still an absence of protein biomarkers for early disease detection. (Abramzon et al., 2020). Recently, an ultra-sensitive MS assay was used to successfully quantify C9ORF72 isoform levels in human brain tissue, demonstrating a significant decrease of the C9ORF72 long isoform in the brains of C9ORF72 mutation carriers (Viode et al., 2018). Additionally, TDP-43 pathology-related cryptic exon RNAs translated protein product have been observed in induced pluripotent stem cells derived neurons with TDP-43 deficiency as well as in CSF from FTD-ALS patients; this may represent a viable target for peptide-based biomarker development (Ling et al., 2015; Seddighi, 2023).
Huntington’s Disease (HD) is caused by a CAG repeat expansion, leading to accumulation and impaired clearance of mutant huntingtin protein. HD is currently diagnosed on the basis of a direct genetic test for CAG repeats, and performance on cognitive tests is the primary metric for disease progression (Yamamoto et al., 2000; Killoran et al., 2022). The need for an objective and sensitive biomarker for HD prognosis led to the identification of mutant huntingtin protein in CSF via an immunoprecipitation and flow-cytometry based assay (Southwell et al., 2015). A biomarker panel combining mutant and native proteins could aid in earlier diagnosis of the disease. Recent investigations have not only identified mutant huntingtin proteins in the mouse cortex using targeted MS approaches (Sap et al., 2021), but also demonstrated that combining mutant huntingtin protein and native markers (e.g., neurofilament light) can enable earlier HD detection and effective monitoring of disease progression and response to treatment (Rodrigues et al., 2020).
To date, the most common application of biomarkers has been in the context of disease diagnosis. Monitoring the levels of native proteins has paved the way for accurate detection of breast cancer (Gam, 2012), colon cancer (Kuppusamy et al., 2017), pancreatic cancer (Duffy et al., 2010), and neurodegenerative diseases (Heywood et al., 2015). However, there is an emerging role for the implementation of mutant protein biomarkers in disease detection. Following the established role of BRAF mutations in cutaneous melanoma, which often results in the substitution of glutamic acid for valine at position 600 (BRAFV600E), this genetic signature and its protein products have garnered much attention as both a diagnostic and prognostic biomarker for melanoma (Capper et al., 2011; Ghossein et al., 2013; Long et al., 2013).
Biomarker panels have demonstrated utility in detecting disease with both specificity and sensitivity. In 2017, Cohen and colleagues presented a proteogenomic screening test for the detection of pancreatic ductal adenocarcinoma using a joint panel of four conventional protein biomarkers for cancer, combined with the presence of mutant KRAS circulating tumor DNA (ctDNA) from a blood draw. With 64% specificity, 99.5% sensitivity, and a demonstrated prognostic value for overall survival, this combinatorial strategy has considerable promise for earlier detection of pancreatic cancer (Cohen et al., 2017). A year later, this strategy was expanded further by CancerSEEK, implementing a panel of ctDNA, consisting of 61 amplicons spread across 16 genes, combined with 8 protein biomarkers. CancerSEEK allows for detection of breast, colorectal, esophageal, liver, lung, ovarian, pancreatic, and stomach cancers from a single blood sample with a specificity of 99% and a sensitivity between from 69%–98%, depending on the type of cancer (Cohen et al., 2018). The efforts from Cohen et al. highlight the potential of proteogenomic panels for a variety of diseases.
In addition to diagnostic and prognostic applications, biomarkers enable patient stratification, allowing for informed and individualized treatment courses. The use of large-scale data to identify “treatable traits” in patients has been a topic of intense focus (König et al., 2017), as conventional classifications based on generalized markers have led to misclassification and ineffective treatment of clinically and pathologically heterogeneous disorders (Nevo et al., 2016). In an attempt to expand upon the five currently implemented breast-cancer subtypes derived from a set of 50 transcriptional signatures (i.e., PAM50 markers) (Parker et al., 2009), Johansson et al. utilized an integrated proteomics analysis on tumor tissue from patients representing each of the five PAM50 subtypes. (Johansson et al., 2019). In addition to identifying proteins derived from non-coding regions as candidate immunotherapeutic targets, network analyses succeeded in stratifying known patient classifications further, proposing previously unrecognized biomarkers and subclasses to guide therapeutic development.
Two studies in lung adenocarcinoma have also highlighted the potential of applying proteogenomics in patient stratification. Chen et al. revealed 5 mutational profiles previously unidentified in LUAD in an East Asian cohort (Chen et al., 2020). The group identified protein and genetic signatures in these subtypes strongly tied to age, gender, and EGFR-mutation status, contributing important considerations for the development of disease-modifying therapies. Furthermore, integrated analyses of multi-omics data from glioblastoma (GBM) samples unveiled new immune-based subtypes, expanding on previous classifications based only on transcriptomic and genomic data (Wang et al., 2017; Wang et al., 2021). Notably, the study subdivided glioblastoma into two distinct groups, allowing for future, more in-depth mechanistic studies to reveal therapeutic vulnerabilities in these newly discovered subclasses for precision medicine (Oh et al., 2020). Leveraging genomic, transcriptomic, and proteomic data together has provided rich resources for better patient stratification, as well as the identification of potential biomarker and therapeutic targets.
Here, we propose a general MS-based proteogenomic workflow for the identification of variant protein markers in human biospecimens (Figure 2). The first step in creating customized databases capable of detecting variants in MS-based approaches is to identify disease-relevant genomic variants. Informatic tools for variant calling are widely available. The most common variants are SNV variants—commonly identified through tools such as Platypus (Rimmer et al., 2014) and Samtools (Li, 2011)—and splicing variants—which can be identified using MAJIQ (Vaquero-Garcia et al., 2016) and MapSplice (Wang et al., 2010), among other tools. Novel peptide products can be predicted from RNA-sequencing results via ECgene (Lee et al., 2006), FastDB (De La Grange et al., 2005), FANTOM3 (Carninci et al., 2005), or the ASTD (Koscielny et al., 2009). Novel protein sequences generated from in silico translation of the reference genome and/or transcriptome—e.g., via tools such as AGUSTUS (Stanke et al., 2006), GENEID (Parra et al., 2000) or EuGENE (Foissac et al., 2003)—allow for customized databases with the power to identify and validate proteins and peptides translated from antisense strands, non-coding genes, intergenic regions, and untranslated regions (UTRs) (Nesvizhskii, 2014). Once the RNA sequences of interest are identified, in silico translation tools, such as Transeq (CITE), Quilts (Ruggles et al., 2016), and GalaxyP (Sheynkman et al., 2014), can be used to predict the resulting amino acid sequence and build a custom peptide database. With this customized FASTA database, it is possible to perform searches of proteomics raw files for sequences of interest using MS search engines, such as PEAKS (Tran et al., 2019), Proteome Discoverer, and MaxQuant (Cox and Mann, 2008).
FIGURE 2. A comprehensive proteogenomic strategy in biomarker discovery. Genomic sequencing reads are aligned to the reference transcriptome to generate BAM files. Variants are called from aligned reads (i.e., Variant Call Format, VCFs). The VCF files are 6-frame (for DNA) or 3-frame (for RNA) translated to produce customized protein sequence (FASTA) files. Mass spectrometry (MS)-based protein sequencing using data-dependent acquisition (DDA) or data-independent acquisition (DIA) is performed. The MS raw files are searched against the custom library generated from genomic data. Identified variant biomarker candidates are validated using targeted proteomics or antibody-based immunoassays in large cohort studies.
Integrated proteogenomic algorithms are also available for “one-stop” analyses, starting from variant calling to MS-spectra annotation (Table 2); however, some tools are not as popular as database search engines and have not been thoroughly validated. Beyond generating patient-specific databases, common mutations from existing databases (Table 3) can be introduced to native proteome databases. For example, Catalogue of Somatic Mutations in Cancer (COSMIC), containing somatic mutations from variety of cancer types, has been widely used for generating customized reference and identifying cancer-specific mutations (Zhu et al., 2018). Qi and colleagues utilized LNCipedia to predict lncRNAs regions and discovered lncRNA-coded neoantigens in lung adenocarcinoma (Qi et al., 2021). A key consideration in developing a proteogenomic database search strategy is the determination of an appropriate false-discovery rate (FDR). By increasing the database size through the integration of native plus variants proteome, the identified variant peptides are prone to high false positive rates from multiple comparisons. Therefore, additional targeted methods are required for validation.
Similar to NGS approaches, MS-based proteomics has rapidly advanced throughout the past two decades. Performing total RNA-seq in biofluids has proven to be technically challenging (Everaert et al., 2019). Given the low quantity and quality of RNA in biofluids, most biomarker studies focus on circulating DNA and small RNAs (Buschmann et al., 2016; Vo et al., 2019). Therefore, protein biomarkers have become the most common clinical markers in body fluids. To increase proteome coverage, various approaches have been adopted. These include a) offline fractionation to reduce sample complexity; b) high-abundant protein depletion to remove housekeeping proteins in biofluids; c) enrichment for tissue-derived extracellular vehicles (EVs) (Fiandaca et al., 2015; Mustapic et al., 2017; Heath et al., 2018); d) nanoparticle-based enrichment of low-abundant proteins and co-depletion of high-abundant proteins (Kim et al., 2018; Tiambeng et al., 2020); and e) use of multiple proteases to detect peptides not typically generated by standard trypsin cleavage (Giansanti et al., 2016).
For data acquisition in discovery proteomics, data-dependent acquisition (DDA) and data-independent acquisition (DIA) are commonly used in MS. Previous studies demonstrated that DDA and DIA acquire different groups of peptides; this could extend the pool of total peptide identification and protein coverage (Reilly et al., 2021). DDA typically generates less complex, but more specific, MS2 spectra of selected peptides; however, only the most abundant peptide precursors are selected. On the other hand, DIA is a more inclusive approach to fragment all peptide precursors, including low-abundant ones. Although DDA has been more widely applied in biomarker studies, DIA has gained traction more recently for its applications in identifying low-abundant peptides (Guo et al., 2015; Latonen et al., 2018). The increased scan speed of high-resolution MS allows DIA to use narrower isolation windows and cover a broader m/z range (e.g., 400–1,000). DIA generally provides higher confident peptides due to the longer MS2 injection time, which allows for high-resolution MS2 spectra. Database search of DIA data typically requires a spectral library generated from the respective DDA MS run; notably, recent studies demonstrate the direct application of DIA data using a protein sequence library where “pseudo-spectra” and predicted retention times of each precursor ion is generated by search engines, such as DIA-Umpire (Tsou et al., 2015), Spectronaut, and DIA-NN (Demichev et al., 2020). Emerging evidence shows DIA is the next-generation data acquisition approach for label-free proteomics.
Targeted proteomic analyses are commonly employed to validate mutant peptides discovered through DIA/DDA shotgun proteomics and to generate high-throughput MS-based assays for clinical use. Targeted approaches, including multiple reaction monitoring (MRM) and parallel reaction monitoring (PRM), align select or all MS2 transitions and retention times of in vivo peptides and their “heavy isotope” synthetic counterparts that serve as internal standards. Typically, a list of m/z ratio of the precursor ions and their daughter ions is built into the MS instrumentation method to selectively monitor targets. Furthermore, DIA is a “semi-targeted” approach, as the MS2 transitions that are used for qualification can also be visualized as PRM-like spectra in Skyline (MacLean et al., 2010) and SpectroDive. Many proof-of-concept studies have utilized targeted methods to validate variant peptides, as the “gold standard,” ultra-sensitive approach. The biomarker specificity of validated peptides should also be demonstrated in large-scale cohorts containing disease and healthy control samples. If the variant peptides are validated as specific biomarkers, scalable MS-based MRM assays can be developed to rapidly detect such biomarkers in patient samples for point-of-care diagnosis and disease subtype stratification.
Combining NGS and MS-based proteomics represents a powerful strategy for both biomarker discovery and investigation of fundamental biology. However, obtaining sufficient high-quality RNA-seq reads can be challenged by the integrity and quantity of available biospecimens. Furthermore, short-read RNA-seq could easily miss mutation sites and mis-splicing events; therefore, long-read RNA-seq has emerged as a complementary approach, despite its shallower sequencing depth. Although proteome coverage has significantly improved in recent years, low-abundant proteins may still be difficult to identify with current tools. Many approaches have been applied to increase protein coverage, but they are generally time-consuming and increase intra-sample variation. Clinical assays must be quick, robust, and highly reproducible. Therefore, MS instrumentation and proteomic sample preparation need further improvement to boost sensitivity and specificity. De novo proteins could also be structurally unstable and degraded by proteases and peptidases within the lysosome and endosome, thereby evading detection. Overall, despite these challenges, sequence-centric approaches, combined with state-of-the-art mass spectrometry, contribute to the evolving role of proteogenomics in biomedical research and precision-medicine based initiatives in cancer, neurodegeneration, and beyond.
YQ and MW. Conceptualized the research study. LR and SS conducted the literature search and table generation. LR, SS, AS, MC, and YQ. Drafted the manuscript. YQ, MW, MC, and AS. Supervised the project. All authors have read and agreed to the published version of the manuscript.
This research was supported, in part, by the Intramural Research Program of the NIH National institute on Aging (Project number ZO1 AG000535) at the Center for Alzheimer’s and Related Dementias, NIH grant T32 GM136577 (S.S.), and the NIH Oxford-Cambridge Scholars Program (S.S.).
We thank Drs. Ying Hao and Ziyi Li from National Institute on Aging for providing insights for data acquisition and data analyses of mass spectrometry-based proteomics. We thank Drs. Erika Lara, Daniel Ramos, Marianita Santiana, and Caroline Pantazis from National Institute on Aging for providing insights for variant calling and data analyses in genomics.
The authors declare that the research was conducted in the absence of any commercial or financial relationships that could be construed as a potential conflict of interest.
All claims expressed in this article are solely those of the authors and do not necessarily represent those of their affiliated organizations, or those of the publisher, the editors and the reviewers. Any product that may be evaluated in this article, or claim that may be made by its manufacturer, is not guaranteed or endorsed by the publisher.
Abecasis, G. (2010). A map of human genome variation from population-scale sequencing. Nature 467, 1061–1073. doi:10.1038/nature09534
Abramzon, Y. A., Fratta, P., Traynor, B. J., and Chia, R. (2020). The overlapping genetics of amyotrophic lateral sclerosis and frontotemporal dementia. Front. Neurosci. 14, 42. doi:10.3389/fnins.2020.00042
Ang, M. Y., Low, T. Y., Lee, P. Y., Wan Mohamad Nazarie, W. F., Guryev, V., and Jamal, R. (2019). Proteogenomics: From next-generation sequencing (NGS) and mass spectrometry-based proteomics to precision medicine. Clin. Chim. Acta 498, 38–46. doi:10.1016/j.cca.2019.08.010
Anurag, M., Jaehnig, E. J., Krug, K., Lei, J. T., Bergstrom, E. J., Kim, B. J., et al. (2022). Proteogenomic markers of chemotherapy resistance and response in triple negative breast cancer. Cancer Discov. 12, 2586. doi:10.1158/2159-8290.CD-22-0200
Aronson, J. K., and Ferner, R. E. (2017). Biomarkers-A general review. Curr. Protoc. Pharmacol. 76, 1–9. 23 17. doi:10.1002/cpph.19
Askenazi, M., Ruggles, K. V., and Fenyo, D. (2016). PGx: Putting peptides to BED. J. Proteome Res. 15 (3), 795–799. doi:10.1021/acs.jproteome.5b00870
Awasthi, S., Maity, T., Oyler, B. L., Qi, Y., Zhang, X., Goodlett, D. R., et al. (2018). Quantitative targeted proteomic analysis of potential markers of tyrosine kinase inhibitor (TKI) sensitivity in EGFR mutated lung adenocarcinoma. J. Proteomics 189, 48–59. doi:10.1016/j.jprot.2018.04.005
Barthelemy, N. R., Gabelle, A., Hirtz, C., Fenaille, F., Sergeant, N., Schraen-Maschke, S., et al. (2016). Differential mass spectrometry profiles of tau protein in the cerebrospinal fluid of patients with alzheimer's disease, progressive supranuclear palsy, and dementia with Lewy bodies. J. Alzheimers Dis. 51 (4), 1033–1043. doi:10.3233/JAD-150962
Barthelemy, N. R., Mallipeddi, N., Moiseyev, P., Sato, C., and Bateman, R. J. (2019). Tau phosphorylation rates measured by mass spectrometry differ in the intracellular brain vs. Extracellular cerebrospinal fluid compartments and are differentially affected by alzheimer's disease. Front. Aging Neurosci. 11, 121. doi:10.3389/fnagi.2019.00121
Bignell, G. R., Greenman, C. D., Davies, H., Butler, A. P., Edkins, S., Andrews, J. M., et al. (2010). Signatures of mutation and selection in the cancer genome. Nature 463 (7283), 893–898. doi:10.1038/nature08768
Borrebaeck, C. A., and Wingren, C. (2009). Design of high-density antibody microarrays for disease proteomics: Key technological issues. J. Proteomics 72 (6), 928–935. doi:10.1016/j.jprot.2009.01.027
Bozic, I., Antal, T., Ohtsuki, H., Carter, H., Kim, D., Chen, S., et al. (2010). Accumulation of driver and passenger mutations during tumor progression. Proc. Natl. Acad. Sci. 107 (43), 18545–18550. doi:10.1073/pnas.1010978107
Buschmann, D., Haberberger, A., Kirchner, B., Spornraft, M., Riedmaier, I., Schelling, G., et al. (2016). Toward reliable biomarker signatures in the age of liquid biopsies - how to standardize the small RNA-Seq workflow. Nucleic Acids Res. 44 (13), 5995–6018. doi:10.1093/nar/gkw545
Cancer (2006). The cancer genome Atlas. Available from: https://www.cancer.gov/about-nci/organization/ccg/research/structural-genomics/tcga.
Cao, L., Huang, C., Cui Zhou, D., Hu, Y., Lih, T. M., Savage, S. R., et al. (2021). Proteogenomic characterization of pancreatic ductal adenocarcinoma. Cell 184 (19), 5031–5052 e26. doi:10.1016/j.cell.2021.08.023
Capper, D., Preusser, M., Habel, A., Sahm, F., Ackermann, U., Schindler, G., et al. (2011). Assessment of BRAF V600E mutation status by immunohistochemistry with a mutation-specific monoclonal antibody. Acta Neuropathol. 122 (1), 11–19. doi:10.1007/s00401-011-0841-z
Carlyle, B. C., Trombetta, B. A., and Arnold, S. E. (2018). Proteomic approaches for the discovery of biofluid biomarkers of neurodegenerative dementias. Proteomes 6 (3), 32. doi:10.3390/proteomes6030032
Carninci, P., Kasukawa, T., Katayama, S., Gough, J., Frith, M. C., Maeda, N., et al. (2005). The transcriptional landscape of the mammalian genome. science 309 (5740), 1559–1563. doi:10.1126/science.1112014
Chen, Y. J., Roumeliotis, T. I., Chang, Y. H., Chen, C. T., Han, C. L., Lin, M. H., et al. (2020). Proteogenomics of non-smoking lung cancer in East asia delineates molecular signatures of pathogenesis and progression. Cell 182 (1), 226–244. doi:10.1016/j.cell.2020.06.012
Chiou, S.-H., and Lee, K.-T. (2016). Proteomic analysis and translational perspective of hepatocellular carcinoma: Identification of diagnostic protein biomarkers by an onco-proteogenomics approach. Kaohsiung J. Med. Sci. 32 (11), 535–544. doi:10.1016/j.kjms.2016.09.002
Chrystoja, C. C., and Diamandis, E. P. (2014). Whole genome sequencing as a diagnostic test: Challenges and opportunities. Clin. Chem. 60 (5), 724–733. doi:10.1373/clinchem.2013.209213
Cohen, J. D., Javed, A. A., Thoburn, C., Wong, F., Tie, J., Gibbs, P., et al. (2017). Combined circulating tumor DNA and protein biomarker-based liquid biopsy for the earlier detection of pancreatic cancers. Proc. Natl. Acad. Sci. U. S. A. 114 (38), 10202–10207. doi:10.1073/pnas.1704961114
Cohen, J. D., Li, L., Wang, Y., Thoburn, C., Afsari, B., Danilova, L., et al. (2018). Detection and localization of surgically resectable cancers with a multi-analyte blood test. Science 359 (6378), 926–930. doi:10.1126/science.aar3247
Consortium, U. (2015). UniProt: A hub for protein information. Nucleic Acids Res. 43 (D1), D204–D212. doi:10.1093/nar/gku989
Cox, J., and Mann, M. (2008). MaxQuant enables high peptide identification rates, individualized p.p.b.-range mass accuracies and proteome-wide protein quantification. Nat. Biotechnol. 26 (12), 1367–1372. doi:10.1038/nbt.1511
Crappe, J., Ndah, E., Koch, A., Steyaert, S., Gawron, D., De Keulenaer, S., et al. (2015). Proteoformer: Deep proteome coverage through ribosome profiling and MS integration. Nucleic Acids Res. 43 (5), e29. doi:10.1093/nar/gku1283
De La Grange, P., Dutertre, M., Martin, N., and Auboeuf, D. (2005). Fast db: A website resource for the study of the expression regulation of human gene products. Nucleic acids Res. 33 (13), 4276–4284. doi:10.1093/nar/gki738
DeKosky, S. T., and Marek, K. (2003). Looking backward to move forward: Early detection of neurodegenerative disorders. Science 302 (5646), 830–834. doi:10.1126/science.1090349
Demichev, V., Messner, C. B., Vernardis, S. I., Lilley, K. S., and Ralser, M. (2020). DIA-NN: Neural networks and interference correction enable deep proteome coverage in high throughput. Nat. Methods 17 (1), 41–44. doi:10.1038/s41592-019-0638-x
Demory Beckler, M., Higginbotham, J. N., Franklin, J. L., Ham, A. J., Halvey, P. J., Imasuen, I. E., et al. (2013). Proteomic analysis of exosomes from mutant KRAS colon cancer cells identifies intercellular transfer of mutant KRAS. Mol. Cell Proteomics 12 (2), 343–355. doi:10.1074/mcp.M112.022806
Desiere, F., Deutsch, E. W., King, N. L., Nesvizhskii, A. I., Mallick, P., Eng, J., et al. (2006). The PeptideAtlas project. Nucleic Acids Res. 34, D655–D658. doi:10.1093/nar/gkj040
Dou, Y., Kawaler, E. A., Cui Zhou, D., Gritsenko, M. A., Huang, C., Blumenberg, L., et al. (2020). Proteogenomic characterization of endometrial carcinoma. Cell 180 (4), 729–748 e26. doi:10.1016/j.cell.2020.01.026
Duan, G., Li, X., and Kohn, M. (2015). The human DEPhOsphorylation database DEPOD: A 2015 update. Nucleic Acids Res. 43, D531–D535. doi:10.1093/nar/gku1009
Duffy, M. J., Sturgeon, C., Lamerz, R., Haglund, C., Holubec, V. L., Klapdor, R., et al. (2010). Tumor markers in pancreatic cancer: A European group on tumor markers (EGTM) status report. Ann. Oncol. 21 (3), 441–447. doi:10.1093/annonc/mdp332
Duffy, M. J., Synnott, N. C., and Crown, J. (2018). Mutant p53 in breast cancer: Potential as a therapeutic target and biomarker. Breast Cancer Res. Treat. 170 (2), 213–219. doi:10.1007/s10549-018-4753-7
Ellis, M. J., Gillette, M., Carr, S. A., Paulovich, A. G., Smith, R. D., Rodland, K. K., et al. (2013). Connecting genomic alterations to cancer biology with proteomics: The NCI clinical proteomic tumor analysis Consortium. Cancer Discov. 3 (10), 1108–1112. doi:10.1158/2159-8290.CD-13-0219
Ellis, M. J., Gillette, M., Carr, S. A., Paulovich, A. G., Smith, R. D., Rodland, K. K., et al. (2013). Connecting genomic alterations to cancer biology with proteomics: The NCI clinical proteomic tumor analysis Consortium. Cancer Discov. 3 (10), 1108–1112. doi:10.1158/2159-8290.CD-13-0219
Everaert, C., Helsmoortel, H., Decock, A., Hulstaert, E., Van Paemel, R., Verniers, K., et al. (2019). Performance assessment of total RNA sequencing of human biofluids and extracellular vesicles. Sci. Rep. 9 (1), 17574. doi:10.1038/s41598-019-53892-x
Fehse, B., Richters, A., Putimtseva-Scharf, K., Klump, H., Li, Z., Ostertag, W., et al. (2000). CD34 splice variant: An attractive marker for selection of gene-modified cells. Mol. Ther. 1 (5), 448–456. doi:10.1006/mthe.2000.0068
Fiandaca, M. S., Kapogiannis, D., Mapstone, M., Boxer, A., Eitan, E., Schwartz, J. B., et al. (2015). Identification of preclinical alzheimer's disease by a profile of pathogenic proteins in neurally derived blood exosomes: A case-control study. Alzheimers Dement. 11 (6), 600–607. doi:10.1016/j.jalz.2014.06.008
Foissac, S., Bardou, P., Moisan, A., Cros, M. J., and Schiex, T. (2003). EUGÈNE'HOM: A generic similarity-based gene finder using multiple homologous sequences. Nucleic Acids Res. 31 (13), 3742–3745. doi:10.1093/nar/gkg586
Gam, L. H. (2012). Breast cancer and protein biomarkers. World J. Exp. Med. 2 (5), 86–91. doi:10.5493/wjem.v2.i5.86
Gargis, A. S., Kalman, L., Berry, M. W., Bick, D. P., Dimmock, D. P., Hambuch, T., et al. (2012). Assuring the quality of next-generation sequencing in clinical laboratory practice. Nat. Biotechnol. 30 (11), 1033–1036. doi:10.1038/nbt.2403
Ghossein, R. A., Katabi, N., and Fagin, J. A. (2013). Immunohistochemical detection of mutated BRAF V600E supports the clonal origin of BRAF-induced thyroid cancers along the spectrum of disease progression. J. Clin. Endocrinol. Metabolism 98 (8), E1414–E1421. doi:10.1210/jc.2013-1408
Giansanti, P., Tsiatsiani, L., Low, T. Y., and Heck, A. J. R. (2016). Six alternative proteases for mass spectrometry-based proteomics beyond trypsin. Nat. Protoc. 11 (5), 993–1006. doi:10.1038/nprot.2016.057
Gillette, M. A., Satpathy, S., Cao, S., Dhanasekaran, S. M., Vasaikar, S. V., Krug, K., et al. (2020). Proteogenomic characterization reveals therapeutic vulnerabilities in lung adenocarcinoma. Cell 182 (1), 200–225.e35. doi:10.1016/j.cell.2020.06.013
Guo, T., Kouvonen, P., Koh, C. C., Gillet, L. C., Wolski, W. E., Röst, H. L., et al. (2015). Rapid mass spectrometric conversion of tissue biopsy samples into permanent quantitative digital proteome maps. Nat. Med. 21 (4), 407–413. doi:10.1038/nm.3807
Guruceaga, E., Garin-Muga, A., and Segura, V. (2020). MiTPeptideDB: A proteogenomic resource for the discovery of novel peptides. Bioinformatics 36 (1), 205–211. doi:10.1093/bioinformatics/btz530
Heath, N., Grant, L., De Oliveira, T. M., Rowlinson, R., Osteikoetxea, X., Dekker, N., et al. (2018). Rapid isolation and enrichment of extracellular vesicle preparations using anion exchange chromatography. Sci. Rep. 8 (1), 5730. doi:10.1038/s41598-018-24163-y
Hedl, T. J., San Gil, R., Cheng, F., Rayner, S. L., Davidson, J. M., De Luca, A., et al. (2019). Proteomics approaches for biomarker and drug target discovery in ALS and FTD. Front. Neurosci. 13, 548. doi:10.3389/fnins.2019.00548
Heywood, W. E., Galimberti, D., Bliss, E., Sirka, E., Paterson, R. W., Magdalinou, N. K., et al. (2015). Identification of novel CSF biomarkers for neurodegeneration and their validation by a high-throughput multiplexed targeted proteomic assay. Mol. Neurodegener. 10, 64. doi:10.1186/s13024-015-0059-y
Hoadley, K. A., Yau, C., Hinoue, T., Wolf, D. M., Lazar, A. J., Drill, E., et al. (2018). Cell-of-Origin patterns dominate the molecular classification of 10,000 tumors from 33 types of cancer. Cell 173 (2), 291–304 e6. doi:10.1016/j.cell.2018.03.022
Huang, C. H., Kuo, C. J., Liang, S. S., Chi, S. W., Hsi, E., Chen, C. C., et al. (2015). Onco-proteogenomics identifies urinary S100A9 and GRN as potential combinatorial biomarkers for early diagnosis of hepatocellular carcinoma. BBA Clin. 3, 205–213. doi:10.1016/j.bbacli.2015.02.004
Jaffe, J. D., Berg, H. C., and Church, G. M. (2004). Proteogenomic mapping as a complementary method to perform genome annotation. Proteomics 4 (1), 59–77. doi:10.1002/pmic.200300511
Janik, M. K., Smyk, W., Kruk, B., Szczepankiewicz, B., Górnicka, B., Lebiedzińska-Arciszewska, M., et al. (2021). MARC1 p.A165T variant is associated with decreased markers of liver injury and enhanced antioxidant capacity in autoimmune hepatitis. Sci. Rep. 11 (1), 24407. doi:10.1038/s41598-021-03521-3
Jian, X., Boerwinkle, E., and Liu, X. (2014). In silico prediction of splice-altering single nucleotide variants in the human genome. Nucleic Acids Res. 42(22), 13534–13544. doi:10.1093/nar/gku1206
Jimmy, K., Eng, A. L. M., and Yates, J. R. (1994). An approach to correlate tandem mass spectral data of peptides with amino acid sequences in a protein database. J. Am. Soc. Mass Spectrom. 5 (11), 976–989. doi:10.1016/1044-0305(94)80016-2
Johansson, H. J., Socciarelli, F., Vacanti, N. M., Haugen, M. H., Zhu, Y., Siavelis, I., et al. (2019). Breast cancer quantitative proteome and proteogenomic landscape. Nat. Commun. 10 (1), 1600. doi:10.1038/s41467-019-09018-y
Johnson, E. C. B., Dammer, E. B., Duong, D. M., Yin, L., Thambisetty, M., Troncoso, J. C., et al. (2018). Deep proteomic network analysis of Alzheimer’s disease brain reveals alterations in RNA binding proteins and RNA splicing associated with disease. Mol. Neurodegener. 13 (1), 52. doi:10.1186/s13024-018-0282-4
Kamalakaran, S., Varadan, V., Janevski, A., Banerjee, N., Tuck, D., McCombie, W. R., et al. (2013). Translating next generation sequencing to practice: Opportunities and necessary steps. Mol. Oncol. 7 (4), 743–755. doi:10.1016/j.molonc.2013.04.008
Keerthikumar, S., Gangoda, L., Liem, M., Fonseka, P., Atukorala, I., Ozcitti, C., et al. (2015). Proteogenomic analysis reveals exosomes are more oncogenic than ectosomes. Oncotarget 6 (17), 15375–15396. doi:10.18632/oncotarget.3801
Killoran, A. (2022). “Biomarkers in Huntington’s DiseaseHuntington’s disease (HD),” in Neurodegenerative diseases biomarkers: Towards translating research to clinical practice Editors P. V. Peplow, B. Martinez, and T. A. Gennarelli (New York, NY: Springer US), 235–262.
Kim, B., Araujo, R., Howard, M., Magni, R., Liotta, L. A., and Luchini, A. (2018). Affinity enrichment for mass spectrometry: Improving the yield of low abundance biomarkers. Expert Rev. Proteomics 15 (4), 353–366. doi:10.1080/14789450.2018.1450631
Kim, C. Y., Na, K., Park, S., Jeong, S. K., Cho, J. Y., Shin, H., et al. (2019). FusionPro, a versatile proteogenomic tool for identification of novel fusion transcripts and their potential translation products in cancer cells. Mol. Cell Proteomics 18 (8), 1651–1668. doi:10.1074/mcp.RA119.001456
Komor, M. A., Pham, T. V., Hiemstra, A. C., Piersma, S. R., Bolijn, A. S., Schelfhorst, T., et al. (2017). Identification of differentially expressed splice variants by the proteogenomic pipeline splicify. Mol. Cell Proteomics 16 (10), 1850–1863. doi:10.1074/mcp.TIR117.000056
König, I. R., Fuchs, O., Hansen, G., von Mutius, E., and Kopp, M. V. (2017). What is precision medicine? Eur. Respir. J. 50 (4), 1700391. doi:10.1183/13993003.00391-2017
Koscielny, G., Le Texier, V., Gopalakrishnan, C., Kumanduri, V., Riethoven, J. J., Nardone, F., et al. (2009). Astd: The alternative splicing and transcript diversity database. Genomics 93 (3), 213–220. doi:10.1016/j.ygeno.2008.11.003
Krassowski, M., Paczkowska, M., Cullion, K., Huang, T., Dzneladze, I., Ouellette, B. F. F., et al. (2018). ActiveDriverDB: Human disease mutations and genome variation in post-translational modification sites of proteins. Nucleic Acids Res. 46 (D1), D901–D910. doi:10.1093/nar/gkx973
Kuppusamy, P., Govindan, N., Yusoff, M. M., and Ichwan, S. J. A. (2017). Proteins are potent biomarkers to detect colon cancer progression. Saudi J. Biol. Sci. 24 (6), 1212–1221. doi:10.1016/j.sjbs.2014.09.017
Latonen, L., Afyounian, E., Jylhä, A., Nättinen, J., Aapola, U., Annala, M., et al. (2018). Integrative proteomics in prostate cancer uncovers robustness against genomic and transcriptomic aberrations during disease progression. Nat. Commun. 9 (1), 1176. doi:10.1038/s41467-018-03573-6
Laumont, C. M., Daouda, T., Laverdure, J. P., Bonneil, É., Caron-Lizotte, O., Hardy, M. P., et al. (2016). Global proteogenomic analysis of human MHC class I-associated peptides derived from non-canonical reading frames. Nat. Commun. 7, 10238. doi:10.1038/ncomms10238
Lawrence, R. T., Perez, E. M., Hernández, D., Miller, C. P., Haas, K. M., Irie, H. Y., et al. (2015). The proteomic landscape of triple-negative breast cancer. Cell Rep. 11 (6), 990. doi:10.1016/j.celrep.2015.04.059
Lee, Y., Kim, B., Shin, Y., Nam, S., Kim, P., et al. (2006). ECgene: An alternative splicing database update. Nucleic Acids Res. 35 (1), D99–D103. doi:10.1093/nar/gkl992
Leonardi, G. C., Candido, S., Cervello, M., Nicolosi, D., Raiti, F., Travali, S., et al. (2012). The tumor microenvironment in hepatocellular carcinoma (review). Int. J. Oncol. 40 (6), 1733–1747. doi:10.3892/ijo.2012.1408
Li, H. (2011). A statistical framework for SNP calling, mutation discovery, association mapping and population genetical parameter estimation from sequencing data. Bioinformatics 27 (21), 2987–2993. doi:10.1093/bioinformatics/btr509
Li, Y., Wang, X., Cho, J. H., Shaw, T. I., Wu, Z., Bai, B., et al. (2016). JUMPg: An integrative proteogenomics pipeline identifying unannotated proteins in human brain and cancer cells. J. proteome Res. 15 (7), 2309–2320. doi:10.1021/acs.jproteome.6b00344
Li, Y., Wang, X., Cho, J. H., Shaw, T. I., Wu, Z., Bai, B., et al. (2016). JUMPg: An integrative proteogenomics pipeline identifying unannotated proteins in human brain and cancer cells. J. Proteome Res. 15 (7), 2309–2320. doi:10.1021/acs.jproteome.6b00344
Ling, J. P., Pletnikova, O., Troncoso, J. C., and Wong, P. C. (2015). TDP-43 repression of nonconserved cryptic exons is compromised in ALS-FTD. Science 349 (6248), 650–655. doi:10.1126/science.aab0983
Long, G. V., Wilmott, J. S., Capper, D., Preusser, M., Zhang, Y. E., Thompson, J. F., et al. (2013). Immunohistochemistry is highly sensitive and specific for the detection of V600E BRAF mutation in melanoma. Am. J. Surg. Pathology 37 (1), 61–65. doi:10.1097/PAS.0b013e31826485c0
MacLean, B., Tomazela, D. M., Shulman, N., Chambers, M., Finney, G. L., Frewen, B., et al. (2010). Skyline: An open source document editor for creating and analyzing targeted proteomics experiments. Bioinformatics 26 (7), 966–968. doi:10.1093/bioinformatics/btq054
Mertins, P., Mani, D. R., Ruggles, K. V., Gillette, M. A., Clauser, K. R., Wang, P., et al. (2016). Proteogenomics connects somatic mutations to signalling in breast cancer. Nature 534 (7605), 55–62. doi:10.1038/nature18003
Monti, R., Rautenstrauch, P., Ghanbari, M., James, A. R., Kirchler, M., Ohler, U., et al. (2022). Identifying interpretable gene-biomarker associations with functionally informed kernel-based tests in 190,000 exomes. Nat. Commun. 13 (1), 5332. doi:10.1038/s41467-022-32864-2
Mustapic, M., Eitan, E., Werner, J. K., Berkowitz, S. T., Lazaropoulos, M. P., Tran, J., et al. (2017). Plasma extracellular vesicles enriched for neuronal origin: A potential window into brain pathologic processes. Front. Neurosci. 11, 278. doi:10.3389/fnins.2017.00278
Nesvizhskii, A. I. (2014). Proteogenomics: Concepts, applications and computational strategies. Nat. methods 11 (11), 1114–1125. doi:10.1038/nmeth.3144
Nevo, D., Zucker, D. M., Tamimi, R. M., and Wang, M. (2016). Accounting for measurement error in biomarker data and misclassification of subtypes in the analysis of tumor data. Statistics Med. 35 (30), 5686–5700. doi:10.1002/sim.7083
Ning, K., and Nesvizhskii, A. I. (2010). The utility of mass spectrometry-based proteomic data for validation of novel alternative splice forms reconstructed from RNA-seq data: A preliminary assessment. BMC Bioinforma. 11 (11), S14. doi:10.1186/1471-2105-11-S11-S14
O'Leary, N. A., Wright, M. W., Brister, J. R., Ciufo, S., Haddad, D., McVeigh, R., et al. (2016). Reference sequence (RefSeq) database at NCBI: Current status, taxonomic expansion, and functional annotation. Nucleic Acids Res. 44 (D1), D733–D745. doi:10.1093/nar/gkv1189
Oh, S., Yeom, J., Cho, H. J., Kim, J. H., Yoon, S. J., Kim, H., et al. (2020). Integrated pharmaco-proteogenomics defines two subgroups in isocitrate dehydrogenase wild-type glioblastoma with prognostic and therapeutic opportunities. Nat. Commun. 11 (1), 3288. doi:10.1038/s41467-020-17139-y
Parker, J. S., Mullins, M., Cheang, M. C. U., Leung, S., Voduc, D., Vickery, T., et al. (2009). Supervised risk predictor of breast cancer based on intrinsic subtypes. J. Clin. Oncol. 27 (8), 1160–1167. doi:10.1200/JCO.2008.18.1370
Parra, G., Blanco, E., and Guigó, R. (2000). GeneID in Drosophila. Genome Res. 10 (4), 511–515. doi:10.1101/gr.10.4.511
Petralia, F., Tignor, N., Reva, B., Koptyra, M., Chowdhury, S., Rykunov, D., et al. (2020). Integrated proteogenomic characterization across major histological types of pediatric brain cancer. Cell 183 (7), 1962–1985 e31. doi:10.1016/j.cell.2020.10.044
Proteomics Cancer (2007). Office of cancer clinical proteomics research. Available from: https://proteomics.cancer.gov/programs/cptac.
Qi, Y. A., Maity, T. K., Cultraro, C. M., Misra, V., Zhang, X., Ade, C., et al. (2021). Proteogenomic analysis unveils the HLA class I-presented immunopeptidome in melanoma and EGFR-mutant lung adenocarcinoma. Mol. Cell Proteomics 20, 100136. doi:10.1016/j.mcpro.2021.100136
Reddy, E. P., Reynolds, R. K., Santos, E., and Barbacid, M. (1982). A point mutation is responsible for the acquisition of transforming properties by the T24 human bladder carcinoma oncogene. Nature 300 (5888), 149–152. doi:10.1038/300149a0
Reilly, L., Peng, L., Lara, E., Ramos, D., Iben, J., Cookson, M. R., et al. (2021). A fully automated FAIMS-DIA proteomic pipeline for high-throughput characterization of iPSC-derived neurons. bioRxiv, 2021.
Rimmer, A., Phan, H., Mathieson, I., Iqbal, Z., Twigg, S. R. F., et al. WGS500 Consortium (2014). Integrating mapping-assembly- and haplotype-based approaches for calling variants in clinical sequencing applications. Nat. Genet. 46 (8), 912–918. doi:10.1038/ng.3036
Risk, B. A., Spitzer, W. J., and Giddings, M. C. (2013). Peppy: Proteogenomic search software. J. Proteome Res. 12 (6), 3019–3025. doi:10.1021/pr400208w
Rodrigues, F. B., Byrne, L. M., Tortelli, R., Johnson, E. B., Wijeratne, P. A., Arridge, M., et al. (2020). Mutant huntingtin and neurofilament light have distinct longitudinal dynamics in Huntington's disease. Sci. Transl. Med. 12 (574), eabc2888. doi:10.1126/scitranslmed.abc2888
Rodriguez, H., Zenklusen, J. C., Staudt, L. M., Doroshow, J. H., and Lowy, D. R. (2021). The next horizon in precision oncology: Proteogenomics to inform cancer diagnosis and treatment. Cell 184 (7), 1661–1670. doi:10.1016/j.cell.2021.02.055
Ruggles, K. V., Krug, K., Wang, X., Clauser, K. R., Wang, J., Payne, S. H., et al. (2017). Methods, tools and current perspectives in proteogenomics. Mol. Cell Proteomics 16 (6), 959–981. doi:10.1074/mcp.MR117.000024
Ruggles, K. V., Tang, Z., Wang, X., Grover, H., Askenazi, M., Teubl, J., et al. (2016). An analysis of the sensitivity of proteogenomic mapping of somatic mutations and novel splicing events in cancer. Mol. Cell Proteomics 15 (3), 1060–1071. doi:10.1074/mcp.M115.056226
Sap, K. A., Guler, A. T., Bury, A., Dekkers, D., Demmers, J. A. A., and Reits, E. A. (2021). Identification of full-length wild-type and mutant huntingtin interacting proteins by crosslinking immunoprecipitation in mice brain cortex. J. Huntingt. Dis. 10 (3), 335–347. doi:10.3233/JHD-210476
Satpathy, S., Krug, K., Jean Beltran, P. M., Savage, S. R., Petralia, F., Kumar-Sinha, C., et al. (2021). A proteogenomic portrait of lung squamous cell carcinoma. Cell 184 (16), 4348–4371 e40. doi:10.1016/j.cell.2021.07.016
Schlaffner, C. N., Pirklbauer, G. J., Bender, A., and Choudhary, J. S. (2017). Fast, quantitative and variant enabled mapping of peptides to genomes. Cell Syst. 5 (2), 152–156. doi:10.1016/j.cels.2017.07.007
Schwanhäusser, B., Busse, D., Dittmar, G., Schuchhardt, J., Wolf, J., et al. (2011). Global quantification of mammalian gene expression control. Nature 473 (7347), 337–342. doi:10.1038/nature10098
Schwarze, K., Buchanan, J., Taylor, J. C., and Wordsworth, S. (2018). Are whole-exome and whole-genome sequencing approaches cost-effective? A systematic review of the literature. Genet. Med. 20 (10), 1122–1130. doi:10.1038/gim.2017.247
Seddighi, S. (2023). Mis-spliced transcripts generate <em>de novo</em> proteins in TDP-43-related ALS/FTD. bioRxiv, 2023.
Sheynkman, G. M., Johnson, J. E., Jagtap, P. D., Shortreed, M. R., Onsongo, G., Frey, B. L., et al. (2014). Using Galaxy-P to leverage RNA-Seq for the discovery of novel protein variations. BMC Genomics 15, 703. doi:10.1186/1471-2164-15-703
Sheynkman, G. M., Shortreed, M. R., Frey, B. L., and Smith, L. M. (2013). Discovery and mass spectrometric analysis of novel splice-junction peptides using RNA-seq. Mol. Cell. Proteomics 12 (8), 2341–2353. doi:10.1074/mcp.O113.028142
Simon, R., Girod, M., Fonbonne, C., Salvador, A., Clément, Y., Lantéri, P., et al. (2012). Total ApoE and ApoE4 isoform assays in an alzheimer's disease case-control study by targeted mass spectrometry (n=669): A pilot assay for methionine-containing proteotypic peptides. Mol. Cell Proteomics 11 (11), 1389–1403. doi:10.1074/mcp.M112.018861
Sinha, A., Huang, V., Livingstone, J., Wang, J., Fox, N. S., Kurganovs, N., et al. (2019). The proteogenomic landscape of curable prostate cancer. Cancer Cell 35 (3), 414–427. doi:10.1016/j.ccell.2019.02.005
Smith, L. M., and Kelleher, N. L. (2013). Proteoform: A single term describing protein complexity. Nat. Methods 10 (3), 186–187. doi:10.1038/nmeth.2369
Southwell, A. L., Smith, S. E. P., Davis, T. R., Caron, N. S., Villanueva, E. B., Xie, Y., et al. (2015). Ultrasensitive measurement of huntingtin protein in cerebrospinal fluid demonstrates increase with Huntington disease stage and decrease following brain huntingtin suppression. Sci. Rep. 5 (1), 12166. doi:10.1038/srep12166
Stanke, M. S. O., Morgenstern, B., and Waack, S. (2006). Gene prediction in eukaryotes with a generalized hidden Markov model that uses hints from external sources. BMC Bioinforma. 7 (62), 62. doi:10.1186/1471-2105-7-62
Stratton, M. R., Campbell, P. J., and Futreal, P. A. (2009). The cancer genome. Nature 458 (7239), 719–724. doi:10.1038/nature07943
Tate, J. G., Bamford, S., Jubb, H. C., Sondka, Z., Beare, D. M., Bindal, N., et al. (2019). Cosmic: The Catalogue of somatic mutations in cancer. Nucleic Acids Res. 47 (D1), D941-D947–D947. doi:10.1093/nar/gky1015
Tiambeng, T. N., Roberts, D. S., Brown, K. A., Zhu, Y., Chen, B., Wu, Z., et al. (2020). Nanoproteomics enables proteoform-resolved analysis of low-abundance proteins in human serum. Nat. Commun. 11 (1), 3903. doi:10.1038/s41467-020-17643-1
Tomczak, K., Czerwińska, P., and Wiznerowicz, M. (2015). The cancer genome Atlas (TCGA): An immeasurable source of knowledge. Contemp. Oncol. (Poznan, Pol. 19 (1A), A68–A77. doi:10.5114/wo.2014.47136
Tran, N. H., Qiao, R., Xin, L., Chen, X., Liu, C., Zhang, X., et al. (2019). Deep learning enables de novo peptide sequencing from data-independent-acquisition mass spectrometry. Nat. Methods 16 (1), 63–66. doi:10.1038/s41592-018-0260-3
Tsou, C. C., Avtonomov, D., Larsen, B., Tucholska, M., Choi, H., Gingras, A. C., et al. (2015). DIA-umpire: Comprehensive computational framework for data-independent acquisition proteomics. Nat. Methods 12 (3), 258–264. 7 p following 264. doi:10.1038/nmeth.3255
Uhlen, M., Fagerberg, L., Hallström, B. M., Lindskog, C., Oksvold, P., Mardinoglu, A., et al. (2015). Proteomics. Tissue-based map of the human proteome. Science 347 (6220), 1260419. doi:10.1126/science.1260419
Vaquero-Garcia, J., Barrera, A., Gazzara, M. R., González-Vallinas, J., Lahens, N. F., Hogenesch, J. B., et al. (2016). A new view of transcriptome complexity and regulation through the lens of local splicing variations. Elife 5, e11752. doi:10.7554/eLife.11752
Viode, A., Fournier, C., Camuzat, A., Fenaille, F., Latouche, M., et al. NeuroCEB Brain Bank (2018). New antibody-free mass spectrometry-based quantification reveals that C9ORF72 long protein isoform is reduced in the frontal cortex of hexanucleotide-repeat expansion carriers. Front. Neurosci. 12, 589. doi:10.3389/fnins.2018.00589
Vizcaino, J. A., Deutsch, E. W., Wang, R., Csordas, A., Reisinger, F., Ríos, D., et al. (2014). ProteomeXchange provides globally coordinated proteomics data submission and dissemination. Nat. Biotechnol. 32 (3), 223–226. doi:10.1038/nbt.2839
Vo, J. N., Cieslik, M., Zhang, Y., Shukla, S., Xiao, L., Zhang, Y., et al. (2019). The landscape of circular RNA in cancer. Cell 176 (4), 869–881. doi:10.1016/j.cell.2018.12.021
Vogel, C., and Marcotte, E. M. (2012). Insights into the regulation of protein abundance from proteomic and transcriptomic analyses. Nat. Rev. Genet. 13 (4), 227–232. doi:10.1038/nrg3185
Volders, P. J., Anckaert, J., Verheggen, K., Nuytens, J., Martens, L., Mestdagh, P., et al. (2019). LNCipedia 5: Towards a reference set of human long non-coding RNAs. Nucleic Acids Res. 47 (D1), D135–D139. doi:10.1093/nar/gky1031
Wang, K., Singh, D., Zeng, Z., Coleman, S. J., Huang, Y., Savich, G. L., et al. (2010). MapSplice: Accurate mapping of RNA-seq reads for splice junction discovery. Nucleic Acids Res. 38 (18), e178. doi:10.1093/nar/gkq622
Wang, L. B., Karpova, A., Gritsenko, M. A., Kyle, J. E., Cao, S., Li, Y., et al. (2021). Proteogenomic and metabolomic characterization of human glioblastoma. Cancer Cell 39 (4), 509–528 e20. doi:10.1016/j.ccell.2021.01.006
Wang, Q., Chaerkady, R., Wu, J., Hwang, H. J., Papadopoulos, N., Kopelovich, L., et al. (2011). Mutant proteins as cancer-specific biomarkers. Proc. Natl. Acad. Sci. 108 (6), 2444–2449. doi:10.1073/pnas.1019203108
Wang, Q., Hu, B., Hu, X., Kim, H., Squatrito, M., Scarpace, L., et al. (2017). Tumor evolution of glioma-intrinsic gene expression subtypes associates with immunological changes in the microenvironment. Cancer Cell 32 (1), 42–56. doi:10.1016/j.ccell.2017.06.003
Wang, X., Slebos, R. J. C., Wang, D., Halvey, P. J., Tabb, D. L., Liebler, D. C., et al. (2012). Protein identification using customized protein sequence databases derived from RNA-Seq data. J. proteome Res. 11 (2), 1009–1017. doi:10.1021/pr200766z
Wen, B., Xu, S., Zhou, R., Zhang, B., Wang, X., Liu, X., et al. (2016). Pga: An R/bioconductor package for identification of novel peptides using a customized database derived from RNA-seq. BMC Bioinforma. 17 (1), 244. doi:10.1186/s12859-016-1133-3
Wood Laura, D., Parsons, D. W., Jones, S., Lin, J., Sjöblom, T., Leary, R. J., et al. (2007). The genomic landscapes of human breast and colorectal cancers. Science 318 (5853), 1108–1113. doi:10.1126/science.1145720
Xuemei Han, A. A., Yates, J. R., and Yates, J. R. (2008). Mass spectrometry for proteomics. Curr. Opin. Chem. Biol. 12 (5), 483–490. doi:10.1016/j.cbpa.2008.07.024
Yamamoto, A., Lucas, J. J., and Hen, R. (2000). Reversal of neuropathology and motor dysfunction in a conditional model of huntington's disease. Cell 101 (1), 57–66. doi:10.1016/S0092-8674(00)80623-6
Zhang, X., Qi, Y., Zhang, Q., and Liu, W. (2019). Application of mass spectrometry-based MHC immunopeptidome profiling in neoantigen identification for tumor immunotherapy. Biomed. Pharmacother. 120, 109542. doi:10.1016/j.biopha.2019.109542
Zhou, W., Petricoin, E. F., and Longo, C. (2017). Mass spectrometry-based biomarker discovery. Methods Mol. Biol. 1606, 297–311. doi:10.1007/978-1-4939-6990-6_19
Keywords: biomarker, proteogenomics, aging, neurodegenerative, cancers
Citation: Reilly L, Seddighi S, Singleton AB, Cookson MR, Ward ME and Qi YA (2023) Variant biomarker discovery using mass spectrometry-based proteogenomics. Front. Aging 4:1191993. doi: 10.3389/fragi.2023.1191993
Received: 22 March 2023; Accepted: 13 April 2023;
Published: 24 April 2023.
Edited by:
Victoria Belancio, Tulane University, United StatesReviewed by:
Junfeng Ma, Georgetown University, United StatesCopyright © 2023 Reilly, Seddighi, Singleton, Cookson, Ward and Qi. This is an open-access article distributed under the terms of the Creative Commons Attribution License (CC BY). The use, distribution or reproduction in other forums is permitted, provided the original author(s) and the copyright owner(s) are credited and that the original publication in this journal is cited, in accordance with accepted academic practice. No use, distribution or reproduction is permitted which does not comply with these terms.
*Correspondence: Yue A. Qi, YW5keS5xaUBuaWguZ292
†These authors have contributed equally to this work
Disclaimer: All claims expressed in this article are solely those of the authors and do not necessarily represent those of their affiliated organizations, or those of the publisher, the editors and the reviewers. Any product that may be evaluated in this article or claim that may be made by its manufacturer is not guaranteed or endorsed by the publisher.
Research integrity at Frontiers
Learn more about the work of our research integrity team to safeguard the quality of each article we publish.