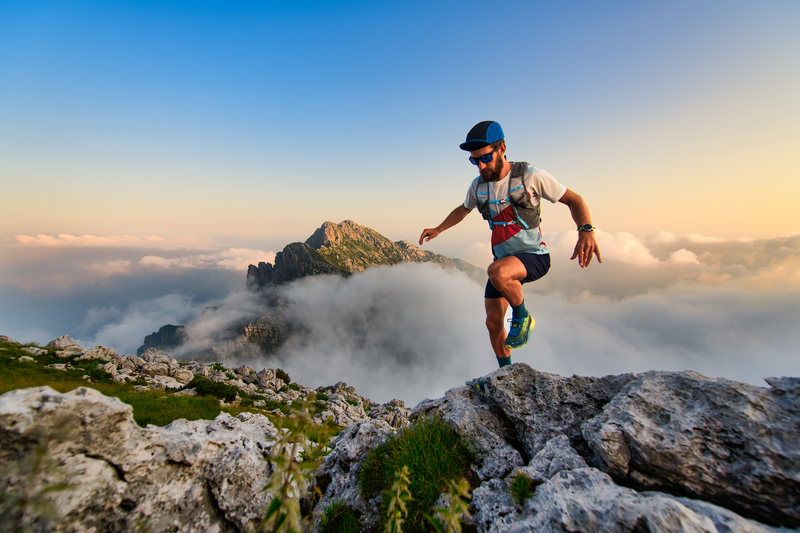
95% of researchers rate our articles as excellent or good
Learn more about the work of our research integrity team to safeguard the quality of each article we publish.
Find out more
ORIGINAL RESEARCH article
Front. Aging Neurosci.
Sec. Neurocognitive Aging and Behavior
Volume 17 - 2025 | doi: 10.3389/fnagi.2025.1582687
This article is part of the Research Topic Decoding Neuroplasticity: Innovations in fMRI Methodologies and Disease Insights View all articles
The final, formatted version of the article will be published soon.
You have multiple emails registered with Frontiers:
Please enter your email address:
If you already have an account, please login
You don't have a Frontiers account ? You can register here
The timely and accurate identification of elderly stroke patients at risk of early neurological deterioration (END) is crucial for guiding clinical management.The present study aimed to create a comprehensive map of lesion location in elderly stroke, and build a machine learning model integrating location features and radiomics to predict END in elderly stroke patients.Methods: A cohort of 709 elderly stroke patients from two centers patients were enrolled. Three machine learning models (logistic regression (LR), random forest (RF), and support vector machine (SVM)) based on location features, radiomics, and Loc-Rad were constructed to predict END in elderly stroke patients, respectively. The performance of models was evaluated using the receiver operating characteristic curves 删除了: L 删除了: models (ROC) and decision curve analysis (DCA). The SHapley Additive exPlanations (SHAP) was used to interpret and visualize the impact of the model predictors on the risk of END. Results: The location maps for elderly stroke patients showed the bilateral cerebellum, left basal ganglia, left corona radiata, and right occipital lobe were significantly associated with END (P < 0.05). For three ML algorithms, the Loc-Rad models based on location features and radiomics demonstrated better performance than the separate location and radiomics -based models in the training cohort (P < 0.05), and the Loc-Rad model constructed with the RF algorithm performed best, with an AUC of 0.883 and accuracy of 0.888, and showed strong prediction performance in the external validation set (AUC of 0.818; accuracy of 0.811). The SHAP plots demonstrated that the most significant contributors to model performance were related to postcentral gyrus left, superior frontal gyrus right, w-HLH_glcm_Correlation, large vessel occlusion and lateral ventricle_body left. Conclusion: We constructed comprehensive maps of strategic lesion network localizations for predicting END in elderly stroke patients and developed a predictive ML model that incorporates both location and radiomics features. This model could facilitate the rapid and robust prediction of the risk of END, enabling timely interventions and personalized treatment strategies to improve patient outcomes.
Keywords: Stroke, Early neurological deterioration, Magnetic Resonance Imaging, machine learning, Predict
Received: 24 Feb 2025; Accepted: 02 Apr 2025.
Copyright: © 2025 Fu, Zhang, Huang, Li, Li, Li, Gao, Peng, Xu and Zhu. This is an open-access article distributed under the terms of the Creative Commons Attribution License (CC BY). The use, distribution or reproduction in other forums is permitted, provided the original author(s) or licensor are credited and that the original publication in this journal is cited, in accordance with accepted academic practice. No use, distribution or reproduction is permitted which does not comply with these terms.
* Correspondence:
Hui Xu, Department of Radiology, Nanjing First Hospital, Nanjing Medical University, Nanjing, China
Disclaimer: All claims expressed in this article are solely those of the authors and do not necessarily represent those of their affiliated organizations, or those of the publisher, the editors and the reviewers. Any product that may be evaluated in this article or claim that may be made by its manufacturer is not guaranteed or endorsed by the publisher.
Research integrity at Frontiers
Learn more about the work of our research integrity team to safeguard the quality of each article we publish.