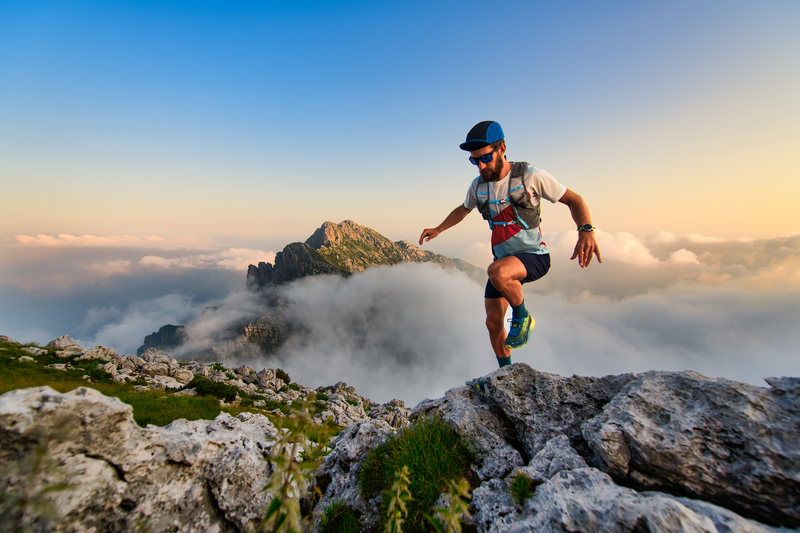
95% of researchers rate our articles as excellent or good
Learn more about the work of our research integrity team to safeguard the quality of each article we publish.
Find out more
ORIGINAL RESEARCH article
Front. Aging Neurosci.
Sec. Neurocognitive Aging and Behavior
Volume 17 - 2025 | doi: 10.3389/fnagi.2025.1580910
This article is part of the Research Topic Artificial Intelligence-based Diagnosis and Neuromodulation in Neurological and Psychiatric Diseases View all 7 articles
The final, formatted version of the article will be published soon.
You have multiple emails registered with Frontiers:
Please enter your email address:
If you already have an account, please login
You don't have a Frontiers account ? You can register here
Background: Accurate differentiation of Parkinsonian syndromes remains challenging due to overlapping clinical manifestations and subtle neuroimaging variations. This study introduces an explainable graph neural network (GNN) framework integrating a Regional Radiomics Similarity Network (R2SN) and Transformer-based attention mechanisms to address this diagnostic dilemma.Methods: Our study prospectively enrolled 1,495 participants, including 220 healthy controls and 1,275 patients diagnosed with idiopathic Parkinson's disease (IPD), multiple system atrophy (MSA), or progressive supranuclear palsy (PSP), all undergoing standardized 18 F-fluorodeoxyglucose positron emission tomography imaging. Metabolic networks were constructed by encoding edge weights derived from radiomic feature similarity matrices, enabling simultaneous quantification of microscopic metabolic heterogeneity and macroscale network reorganization.The proposed framework achieved superior classification performance with F1-scores of 92.5% (MSA), 96.3% (IPD), and 86.7% (PSP), significantly outperforming comparators by 5.5-8.3%. Multiscale interpretability analysis revealed: 1) Regional hypometabolism in pathognomonic nodes (putamen in IPD, midbrain tegmentum in PSP); 2) Disease-specific connectivity disruptions (midbrainprefrontal disconnection in PSP, cerebellar-pontine decoupling in MSA). The substructure attention mechanism reduced computational complexity by 41% while enhancing diagnostic specificity (PSP precision +5.2%).The proposed R2SN-based explainable GNN framework for Parkinsonian syndrome differentiation establishes a new paradigm for precision subtyping of neurodegenerative disorders, with methodological extensibility to other network-driven neurological conditions.
Keywords: parkinsonism, glucose metabolism, Graph neural network, brain imaging, Classification
Received: 21 Feb 2025; Accepted: 31 Mar 2025.
Copyright: © 2025 Ling, Cen, Wu, Wang, Zhang, Jiang, Lu, Liu, Zuo, Jiang, Yang and Yan. This is an open-access article distributed under the terms of the Creative Commons Attribution License (CC BY). The use, distribution or reproduction in other forums is permitted, provided the original author(s) or licensor are credited and that the original publication in this journal is cited, in accordance with accepted academic practice. No use, distribution or reproduction is permitted which does not comply with these terms.
* Correspondence:
Yinghui Yang, School of Health Management, Shanghai Jianqiao University, Shanghai, 201315, Shanghai, China
Zhuangzhi Yan, School of Life Sciences, Shanghai University, Shanghai, 200444, China
Disclaimer: All claims expressed in this article are solely those of the authors and do not necessarily represent those of their affiliated organizations, or those of the publisher, the editors and the reviewers. Any product that may be evaluated in this article or claim that may be made by its manufacturer is not guaranteed or endorsed by the publisher.
Research integrity at Frontiers
Learn more about the work of our research integrity team to safeguard the quality of each article we publish.