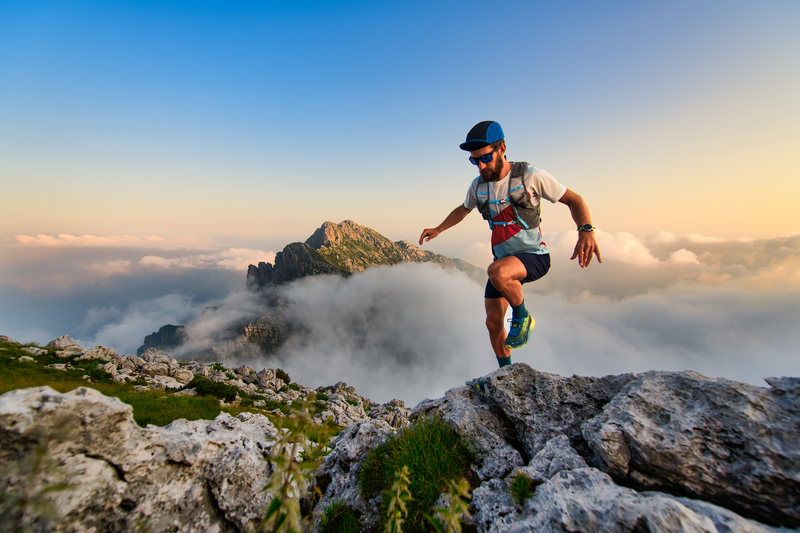
94% of researchers rate our articles as excellent or good
Learn more about the work of our research integrity team to safeguard the quality of each article we publish.
Find out more
ORIGINAL RESEARCH article
Front. Aging Neurosci. , 12 February 2025
Sec. Alzheimer's Disease and Related Dementias
Volume 17 - 2025 | https://doi.org/10.3389/fnagi.2025.1552348
Objective: The 2024 Alzheimer’s Association (AA) research diagnostic criteria for Alzheimer’s Disease (AD) considers fluid biomarkers, including promising blood-based biomarkers for detecting AD. This study aims to identify dementia subtypes and their cognitive and neuroimaging profiles in older adults with dementia in the Democratic Republic of Congo (DRC) using biomarkers and clinical data.
Methods: Forty-five individuals with dementia over 65 years old were evaluated using the Community Screening Instrument for Dementia and the informant-based Alzheimer’s Questionnaire. Core AD biomarkers (Aβ42/40 and p-tau181) and non-specific neurodegeneration biomarkers (NfL, GFAP) were measured in blood plasma. Neuroimaging structures were assessed using magnetic resonance imaging (MRI). Dementia subtypes were determined based on plasma biomarker pathology and vascular markers. Biomarker cutoff scores were identified to optimize sensitivity and specificity. Individuals were stratified into one of four dementia subtypes—AD only, non-AD vascular, non-AD other, or mixed – based on combinations of abnormalities in these markers.
Results: Among the 45 individuals with dementia, mixed dementia had the highest prevalence (42.4%), followed by AD-only (24.4%), non-AD other dementia (22.2%), and non-AD vascular dementia subtypes (11.1%). Both cognitive and neuroimaging profiles aligned poorly with biomarker classifications in the full sample. Cognitive tests varied across dementia subtypes. The cognitive profile of the AD-only and mixed groups suggested relatively low cognitive performance, while the non-AD and other groups had the best scores on average.
Conclusion: Consistent with studies in other settings, our preliminary findings suggest that neurodegenerative plasma biomarkers may help to identify dementia subtypes and provide insight into cognitive and neuroimaging profiles among older adults in the DRC.
Alzheimer’s disease (AD) is the most common neurodegenerative disease, with pathology characterized by the accumulation of amyloid-beta (Aβ) plaques and neurofibrillary tangles composed of hyperphosphorylated tau protein (Balasaheb Chavan et al., 2023). With the advancements in assay technology, plasma biomarkers have increasingly been shown to have potential for the detection and monitorization of AD, increasing accessibility beyond catchment areas of major medical centers (Dimtsu Assfaw et al., 2024; Palmqvist et al., 2024). Current revised 2024 Alzheimer’s Association (AA) criteria distinguish three broad categories of AD fluid biomarkers related to AD pathogenesis: (1) core AD fluid biomarkers [the CSF ratio of amyloid-β (Aβ42/40), phosphorylated and secreted AD tau (p-tau 217, p-tau-181, and p-tau 231)], (2) non-specific biomarkers involved in other neurodegenerative pathology, including neurofilament light (NfL) and glial fibrillary acidic protein (GFAP), and (3) biomarkers of non-AD pathology (vascular brain injury, alpha-synuclein [αSyn]) (Jack et al., 2024). Identifying plasma biomarkers for underlying pathologies of dementia can especially benefit prodromal or pre-clinical stages, for which current and emerging disease-modifying therapies are more likely to be effective (Ashton et al., 2020).
Using blood biomarkers known to provide early indication of a disease may facilitate more timely diagnosis for patients exhibiting early symptoms, particularly in early-onset and atypical presentations. Blood-based biomarkers in AD are associated with both early indicators of cognitive decline and longitudinal cognitive outcomes (Dimtsu Assfaw et al., 2024). For example, lower plasma Aβ42/Aβ40 ratios correlate with higher amyloid plaque burden and cognitive impairment and can be detected in preclinical disease stages (Nakamura et al., 2018), making it useful for early diagnosis and tracking disease progression (Palmqvist et al., 2019). Elevated levels of p-tau181 and p-tau217 are observed in AD, serving as indicators of both early and late stages of AD (Janelidze et al., 2020; Karikari et al., 2020). Plasma p-tau increases in early symptomatic stages, aligning with clinical transition from mild cognitive impairment (MCI) to AD dementia (Thijssen et al., 2020). NfL is a marker of axonal damage; while less specific, elevated levels of NfL reflect more widespread neuronal damage (Mattsson et al., 2017). GFAP reflects astrocytic activation and neuroinflammation, with increased levels observed in AD. GFAP may be used to complement other biomarkers to enhance diagnostic accuracy, particularly in advanced stages (Oeckl et al., 2022). However, additional data are still needed to demonstrate the utility and validity of blood biomarkers in diverse clinical cohorts and to accurately detect disease profiles, particularly given overlapping symptom profiles across different pathologies. For example, vascular damage and protein alterations are present in most forms of dementia, which adds a layer of uncertainty to diagnosis given the potential for mixed dementia pathology (Dodge et al., 2017). In vascular disease, NfL may also be elevated as axonal injury can be seen in cerebrovascular disease. NfL concentrations reflect acute and chronic cerebrovascular injury, which is useful for both early detection and monitoring progression (Mattsson et al., 2019). In addition to plasma biomarkers, structural neuroimaging may also provide important additional diagnostic data. Specifically, the entorhinal cortex and hippocampal regions are particularly affected in the early stages of AD (Balasaheb Chavan et al., 2023; Igarashi, 2023). Hippocampal volume loss is a feature differentiating AD dementia from other dementias, such frontotemporal dementia (FTD) and vascular dementia (VaD), and is closely linked to the course of AD (Killiany et al., 2002; MacLin et al., 2019).
A significant caveat is that most research involving neurodegenerative biomarkers in AD primarily have been conducted using Western cohorts. Studies have shown that CSF biomarkers, such as reduced levels of Aβ42 and p-tau, correlate with AD pathology and can aid in distinguishing AD from other forms of dementia (Van Harten et al., 2021), but less is known about the biomarker and neuroimaging parameters and profiles in diverse populations, particularly in Sub-Saharan African (SSA) populations. This study expands on previous biomarker research by focusing exclusively on SSA populations, where the prevalence and profiles of dementia subtypes remain underexplored. Unlike previous studies, the current study focuses on the variability in biomarker expression and neuroimaging findings in SSA, contributing to the small literature on this topic in this underrepresented region.
The current study aims to explore dementia subtypes based on blood-based biomarkers and vascular factors, and their neuroimaging and cognitive profiles in adult individuals with clinical dementia in Kinshasa, Democratic Republic of Congo (DRC), in SSA. We expected that there is higher prevalence of participants with non-AD pathologies compared to those with AD dementia subtype. Based on previous studies that have linked amyloid-β deposition, tau protein, and neurodegeneration (NfL) accumulations with impairments in language, learning and memory, and executive function, we hypothesized that the cognitive patterns aligning with neurodegenerative biomarkers are characterized by deficits in these cognitive domains (Mattsson et al., 2017; Nakamura et al., 2018; Palmqvist et al., 2019; Slot et al., 2019; Janelidze et al., 2020; Karikari et al., 2020; Thijssen et al., 2020; Oeckl et al., 2022). The current study aims to focus on well-defined dementia cases, ensuring robust differentiation between probable AD dementia and healthy controls (HC); thus, cases with MCI were not included. Focusing on probable AD dementia provides a clearer understanding of advanced disease stages and reduces diagnostic ambiguities associated with MCI, where conversion to dementia is not guaranteed. Similarly, since amyloid-β deposition and tau protein accumulation in the brain are associated with atrophy in the hippocampus, temporal lobe, medial temporal, and entorhinal cortex, we expected that the neuroimaging patterns that align with neurodegenerative biomarkers are characterized by atrophy in these structures (Killiany et al., 2002; MacLin et al., 2019; Balasaheb Chavan et al., 2023; Igarashi, 2023). A general comparison of cognitive deficits and brain atrophy reveals more severe and distinct patterns of deficits and atrophy in Alzheimer’s disease (AD) participants, followed by those with mixed dementia, and vascular dementia.
Participants of this study are community-dwellers from Kinshasa/DRC diagnosed with dementia and selected from a prevalence study of dementia (Ikanga et al., 2023b). Study design details have been published previously (Ikanga et al., 2023b). Briefly, participants were included if they were at least 65 years or older, had a family member or close friend to serve as an informant, and fluent in French or Lingala. We excluded individuals who had history of schizophrenia, neurological, or other medical conditions potentially affecting the central nervous system (CNS), yielding a sample of 1,432 eligible participants. To establish neurological status in the absence of established diagnostic criteria for AD in Sub-Saharan Africa (SSA), we screened eligible participants using the Alzheimer’s Questionnaire (AQ) (Malek-Ahmadi and Sabbagh, 2015) and the Community Screening Instrument for Dementia (CSID) (Hall et al., 2000; Imarhiagbe et al., 2005). The AQ assesses activities of daily living and symptoms of AD in participants (Hall et al., 2000). The CSID Questionnaire, used in several SSA dementia studies (Akinyemi et al., 2014; Farombi et al., 2016; Ogbimi et al., 2023), was used to screen cognitive abilities.
Based on cognitive and functional deficits per the Diagnostic and Statistical Manual of Mental Disorders, Fifth Edition, Text Revision (DSM-5-TR) diagnostic criteria (American Psychiatric Association, 2013), we classified eligible participants using CSID cut-offs from a previous study conducted in Congo-Brazzaville, the closest city from Kinshasa (Guerchet et al., 2010). Similar to our prior study (Ikanga et al., 2023b), eligible participants were classified using CSID and AQ scores (see Figure 1) which resulted in 1,161 individuals being excluded based on their having only mild neurocognitive disorder (MND) or subjective cognitive impairment.
Figure 1. Flow chart of recruitment status from those assessed for eligibility at enrollment (n = 1,432) to the individuals that were allocated to the dementia and donated blood for biomarkers (n = 45).
A panel consisting of a neurologist (EE), psychiatrist (GG), and neuropsychologist (JI) reviewed screening tests, clinical interview, and neurological examination of 271 subjects, of whom 59 from 88 were confirmed with a diagnosis of dementia and 58 from 183 were considered HC. Of these 117 participants, 29 refused to provide blood samples, leaving 85 participants (75%) in whom plasma biomarkers were obtained (45 dementia and 40 HC) who were matched on age, education, and sex. For the present analysis, only participants with dementia were included (see Figure 1). Written informed consent was obtained prior to participants’ undergoing any study procedures. Participants were financially compensated for their time. The procedures were approved by the Ethics Committee/Institutional Review Boards of the University of Kinshasa and Emory University.
Participants underwent a comprehensive clinical evaluation, including cognitive testing, self-report questionnaires, and standard psychiatric and neurological evaluations. Subjects were interviewed to obtain demographic, socioeconomic, and medical history and were subsequently administered cognitive testing with African Neuropsychological Battery (ANB) subtests.
Blood samples were drawn at the Medical Center of Kinshasa (CMK) blood laboratory by antecubital venipuncture into dipotassium ethylene diamine tetra acetic acid (K2 EDTA) tubes. Samples were centrifuged within 15 min at 1,800 g house temperature, and 5 mL of plasma was aliquoted into 0.5 mL polypropylene tubes and stored initially at −20°C for less than a week and stored in a −80°C freezer for longer term storage at a CMK laboratory. These aliquots were shipped frozen on dry ice to Emory University for storage and then to University of California San Francisco (UCSF) for measurements.
Plasma biomarker concentrations were measured using commercially available Neurology 4-PLEX E (Aβ40, Aβ42, NfL, and GFAP; lot #503819), P-Tau181 (P-Tau181 v2; lot #503732), IL-1b (lot #503806) and IL-10 (IL-10 2.0, lot #503533) Quanterix kits on the Simoa HD-X platform (Billerica, MA) at UCSF. P-tau217 was measured using the proprietary ALZpath pTau-217 CARe Advantage kit (lot #MAB231122, ALZpath, Inc.) on the Simoa HD-X platform. The instrument operator was blinded to clinical variables. All analytes were measured in duplicate, except for IL-1b, which was measured as a singlicate due to low sample availability (Kirmess et al., 2021; West et al., 2021; Hu et al., 2022). For Aβ40, Aβ42, NfL, and GFAP, all samples were measured above the lower limit of quantification (LLOQ) of 1.02 pg/mL, 0.378 pg/mL, 0.4 pg/mL and 2.89 pg/mL, respectively. The average coefficient of variation (CV) for Aβ40, Aβ42, NfL, and GFAP were 6.0, 6.5, 5 and 4.6%, respectively. For IL-1b and IL-10, the LLOQ were 0.083 and 0.021 pg/mL, respectively. The average CV for IL-10 was 6.1%. For P-tau217 the LLOQ was 0.024 pg/mL and the average CV was 19.8%.
All subjects were imaged on a 1.5 Tesla MRI unit (Siemens, Magneton Sonata) scanner at HJ Hospitals in Kinshasa using the same standardized imaging acquisition protocol based on the Alzheimer’s Disease Research Center (ADRC) protocol of Emory University (Ikanga et al., 2023a). This consisted of sagittal volumetric T1-weighted (MPRAGE), coronal T2-weighted, and axial diffusion-weighted, T2-weighted, and T2-FLAIR sequences. Typical acquisition parameters for the MPRAGE sequence were TR = 2,200 ms, minimum full TE, TI = 1,000 ms, flip angle = 8°, FOV = 25 cm, with a 192 × 184 acquisition matrix, yielding a voxel size of approximately 1.25 × 1.25 × 1.2 mm. The standard MPRAGE, with a shorter TE of 4 ms and a TI of 1,000 ms, was used to achieve better contrast and image quality.
Images were reviewed by a subspecialty certified neuroradiologist (AMS) with 14 years of experience. White matter hyperintensities were graded according to the Age-Related White Matter Changes (ARWMC) scale (Wahlund et al., 2001), The WMH were graded on the T2 FLAIR images, as they are most readily visible and graded on that sequence. The number of chronic brain parenchymal microhemorrhages were recorded. MPRAGE images were reoriented into the oblique coronal plane orthogonal to the principal axis of the hippocampal formation, and medial temporal lobe atrophy (MTLA) (Claus et al., 2017) and entorhinal cortex atrophy (EriCa) (Enkirch et al., 2018) scores were assessed. Finally, the presence or absence of any additional abnormalities was noted, and patients were excluded if neuroimaging evidence indicated an etiology other than probable AD (e.g., presence of a brain tumor).
The 3D T1 images were segmented using Freesurfer (v.6, MGH, MA), which includes a full processing stream for MR imaging data that involves skull-stripping, bias field correction, registration, and anatomical segmentation as well as cortical surface reconstruction, registration, and parcelation. Regional brain volume for both cortical and subcortical brain regions were calculated. The left and right hippocampal volume were averaged. Interindividual variation in head size were accounted for in further statistical analysis by controlling for the effects of the total intracranial volume.
Dementia subtypes were determined based on the plasma biomarkers (Aβ42/40, p-tau181, NfL, GFAP), alongside vascular markers, hemoglobin A1c (HbA1c), blood pressure, and total cholesterol. Given lack of established AD biomarker thresholds in the DRC/SSA, determination of biomarker thresholds was informed by prior analysis conducted by Ikanga et al. (2024). These analyses utilized logistic regression models to assess the relationship between individual biomarkers and neurological status (healthy or suspected AD) (Ikanga et al., 2023a). Receiver operating characteristic (ROC) curve analyses were conducted to evaluate the diagnostic accuracy of the biomarkers, calculating the area under the curve (AUC) for each. Biomarker cutoff scores were defined by maximizing sensitivity and specificity, determined by the highest Youden’s Index, to optimize the classification of neurological status in this population. Thresholds for vascular markers, HbA1c, hypertension, and hypercholesterolemia, were sourced from existing literature (Kowall and Rathmann, 2013; Whelton et al., 2017; Nantsupawat et al., 2019; Li et al., 2021). Individuals with elevated HbA1c (≥ 6.5%), blood pressure (systolic ≥ 130 mmHg or diastolic ≥ 80 mmHg), or total cholesterol (≥ 200 mg/dL) were deemed to have dementia of potential vascular etiology. Subsequently, individuals were classified into one of four dementia subtypes—AD only, non-AD vascular, non-AD other, or mixed—based on their presence or absence of these biomarkers (Table 1).
Table 1. Organization of biomarkers into pathological subtypes of dementia utilizing (Ikanga et al., 2024 ; Hu et al., 2022) threshold for core AD biomarkers.
Descriptive statistics were employed to summarize the data, with continuous variables reported as means and standard deviations, and categorical variables reported as frequencies and row percentages. We used linear regression models to compare differences in demographics, biomarkers, vascular markers, neuroimaging measures, and cognitive tests by dementia subtype. Models were adjusted for age, gender, years of education, total intracranial volume (for neuroimaging variables), and Geriatric Depression Scale (GDS) score. Subsequently, Dunn’s post-hoc test for pairwise comparisons was conducted to explore differences in neuroimaging and cognitive assessment measure between biomarker-defined dementia subtypes. Results were evaluated with a significance set at p < 0.05. Statistical analysis was conducted using R version 4 statistical software.
Demographic data, neurodegenerative plasma biomarkers, vascular markers, neuroimaging, and cognitive characteristics are presented in Table 2. The sample comprised 45 clinically adjudicated dementia participants, of whom 20 (44%) were males, with an average age of 73.8 years (SD = 8 years) and an average of 7.4 years of education (SD = 5 years). Clinically, the sample exhibited high symptoms of depression (GDS = 7.5), and 58% of the participants had clinical hypertension (see Table 2).
Table 3 presents the dementia subtype defined by neurodegenerative plasma biomarkers using Ikanga and colleagues’ threshold (Ikanga et al., 2024). As anticipated, there is a higher prevalence of mixed dementia, followed by AD-only, non-AD other dementia, and non-AD vascular dementia patterns.
Table 4 presents the cognitive profiles for each dementia subtype based on the cutoff criteria established by Ikanga et al. (2024). We excluded the vascular dementia subtype from these analyses due to the small sample size (only five participants). Cognitively, there were no clinically or statistically significant differences between the dementia subtypes. The cognitive profiles do not align well with biomarker-based dementia subtypes.
Table 5 presents the neuroimaging profile for each dementia subtype based on Ikanga and colleagues’ threshold (Ikanga et al., 2024). As in the previous analyses, we did not include the vascular dementia subtype because there are only five participants in this subtype. The neuroimaging profiles do not align well with biomarker-based dementia subtypes. The AD group showed reduced scores in many neuroanatomical structures compared to other dementia subtypes. There was a statistical difference in left hippocampal volume between various dementia subtypes, mostly between AD-only and non-AD other subtypes, and between AD-only and mixed subtypes. There was a trend in terms of microhemorrhage between dementia subtypes (see Table 5).
This study primarily aimed to evaluate the feasibility of using neurodegenerative plasma biomarkers to characterize dementia subtypes and describe their cognitive and neuroimaging profiles in a novel sample of older adults with clinical dementia in SSA. Experts have reported gaps in neuropsychological testing instruments, diagnostic procedures, fluid biomarkers, and neuropathological correlative studies. This exploratory study aimed to address the gap in plasma biomarkers in the DRC/SSA.
Despite the absence of a gold standard threshold for neurodegenerative fluid biomarkers in the DRC/SSA, we investigated the cognitive and neuroimaging profiles of adults with dementia in the DRC/SSA, using plasma neurodegenerative biomarkers. We found a high prevalence of mixed, followed by AD only, non-AD other dementia, and non-AD vascular dementia patterns, despite cultural, racial, and geographic differences. These results contrast with Western findings, which indicate that the most prevalent dementias among older adults (65 years and over) are Alzheimer’s Disease (60–80% of cases), vascular dementia (10–20% of cases), mixed dementia (5–15% of cases), and other dementias, such as dementia with Lewy bodies (2–5% of cases), dementia associated with Parkinson’s disease (3.6% of cases), and frontotemporal dementia (2–5% of cases) (Jack et al., 2019; Nichols et al., 2022; No author list, 2023).
These differences in classifying dementia based on clinical and biological markers can be explained by the heterogeneous and continuous nature of Alzheimer’s disease, which is complex to characterize (Young et al., 2018). Clinical adjudication relies on medical history, neuropsychological assessments, cognitive symptoms, and behavioral changes, which can be subjective and prone to variability among clinicians (Liss et al., 2021). Fluid biomarkers can assess specific proteins or molecules, detect biological changes, and provide objective, quantitative measures to refine clinical diagnosis. Therefore, fluid biomarkers can identify pre-symptomatic or prodromal stages of dementia (Giampietri et al., 2022). Thus, there could be changes in the classification of AD prevalence.
Contrary to our second hypothesis, which predicted that the neuroimaging profile would align better with biomarker-based dementia subtypes than with the cognitive profile, we found that neither cognitive nor neuroimaging profile tracked well with plasma biomarkers among clinical dementia participants. The cognitive profile in the AD-only and Mixed groups suggests relatively low cognitive performance, while the Non-AD Other group demonstrated some of the highest average scores. Biological underpinnings may explain some further variance in cognitive profiles, particularly in the AD only group.
Similarly, the neuroimaging profile appeared to track poorly with biomarker classifications among those with clinical dementia. While the Mixed group showed a relatively preserved neuroimaging profile, including the hippocampus, entorhinal cortex, WMH, microhemorrhage, mesial temporal atrophy score, and entorhinal cortex atrophy score, the AD-only biomarker group has significantly lower neurological volumes. Several factors may explain the lack of alignment between neuroimaging, cognitive profiles, and biomarkers. The small sample size may have reduced the ability to detect meaningful differences between subtypes. Additionally, variability in disease stage among participants could obscure relationships, as neuroimaging measures may reflect different phases of pathology. The resolution of neuroimaging techniques may also be insufficient to capture subtype-specific brain changes. Moreover, lifestyle factors that were not controlled for in analysis, such as levels of physical activity or the presence of comorbidities, likely contributed to variability in cognitive and neuroimaging profiles within subtypes. These factors collectively underscore the complexity of linking biomarkers with dementia subtypes.
As noted, this is the first study to explore biomarker-based dementia subtypes and to examine cognitive and neuroimaging profiles in the DRC/SSA using culturally appropriate neuropsychological tests, neuroimaging tools, and fluid biomarkers. The exploratory findings of this study provide evidence of the usefulness of ANB tests and their importance in the algorithm for clinical adjudication of different subtypes of dementia. Our analyses also showed the importance of MRI and plasma biomarkers as diagnostic tools for dementia in SSA/DRC. Overall, the strengths of the current study include the use of culturally validated neuropsychological tests, the ability to collect neuroimaging data in participants who are not familiar with MRI, and plasma biomarkers in a population where there is resistance to donating blood for research due to fear of witchcraft. Lastly, this study used a case-control design to obtain cross-sectional results.
Some limitations of this exploratory study include the modest sample size in this first DRC effort (which may limit the generalizability of the findings and statistical power), given the cost of collection, shipping, and the analyses of plasma biomarkers, MRI scans, and the novel nature of their introduction in the DRC, which created some hesitancy for many potential participants to enroll in the study. Future studies should recruit larger, more diverse cohorts for robust stratified analyses and consider longitudinal designs to explore the temporal relationship between cognitive decline and biomarker changes. These approaches will enhance diagnostic precision and deepen understanding of dementia subtypes in underrepresented populations. Furthermore, limited alignment between cognitive, neuroimaging, and biomarker-based classifications suggests a need for further exploration of methodology. Future studies should also prioritize the recruitment of larger and more diverse cohorts to enable nuanced analyses of sex-based factors in AD and other dementia subtypes within Congolese populations. Growing evidence highlights the differential impact of sex on the risk, progression, and presentation of AD, driven by a combination of biological, hormonal, and sociocultural factors. In the context of the DRC, where sociocultural roles and access to healthcare often vary by gender, such analyses are particularly critical. Larger sample sizes would allow for statistically robust investigations into how sex interacts with biomarkers, cognitive outcomes, and neuroimaging profiles in this population. Additionally, incorporating diverse cohorts reflecting a range of socioeconomic backgrounds, education levels, and urban versus rural living conditions will be essential for identifying unique risk and protective factors. These efforts are vital for developing tailored diagnostic and therapeutic strategies that address the complex interplay between sex and dementia in underrepresented and underserved populations. The discrepancy between cognitive and biomarker-based classifications may reflect limitations in cognitive tests, biomarkers, or both. Cognitive assessments can be influenced by cultural, educational, and linguistic factors, while biomarker-based classifications, especially emerging blood biomarkers, may show variability across ethnic groups and lack validation in non-Western populations. These findings underscore the need for culturally adapted cognitive measures and biomarker validation in diverse settings.
Overall, we were pleasantly surprised by the success of our project, and we hope to recruit even larger samples in the future, and to analyze other neurodegenerative fluid biomarkers and the staging of various dementia subtypes. We are very hopeful that our work will contribute to improving clinical and biological adjudication of the accuracy of the diagnosis of AD and other neurodegenerative dementias in SSA, which will, in turn, decrease the potential diagnostic heterogeneity that might currently exist. Additionally, we only focused on participants with dementia without including other intermediary cognitive decline (e.g., MCI cohort), which could be seen as a limitation as well. The decision to exclude MCI was based on both funding limitations and the opportunity to investigate patterns of dementia that are well characterized in the Western world, as an opportunity to establish the validity of these techniques in SSA. With this success, we ultimately want to build a cohort of more diverse Congolese older adults to investigate many other fluid biomarker hypotheses tested in the West.
In conclusion, despite some limitations, the current study provides the first and preliminary patterns of dementia based on the biological definition of dementia and their cognitive and neuroimaging profiles in elderly adults with clinical dementia in Kinshasa/DRC. Future research should build on the methods and findings provided by our exploratory study to establish gold standard thresholds for different fluid biomarkers, the classification of various dementia subtypes based on these biomarkers, and the harmonization with clinical classification in probable AD and related dementia patients in SSA and DRC. Future research should also include cohorts of patients with intermediary status of cognitive decline, amnestic and non-amnestic dementia.
The raw data supporting the conclusions of this article will be made available by the authors, without undue reservation.
The studies involving humans were approved by Emory University and University of Kinshasa. The studies were conducted in accordance with the local legislation and institutional requirements. The participants provided their written informed consent to participate in this study.
JI: Conceptualization, Data curation, Formal analysis, Funding acquisition, Investigation, Methodology, Project administration, Resources, Software, Supervision, Validation, Visualization, Writing – original draft, Writing – review & editing. SP: Writing – original draft, Writing – review & editing. MS: Writing – original draft, Writing – review & editing. CO: Writing – review & editing. EE: Writing – review & editing. GG: Writing – review & editing. NT: Writing – review & editing. IK: Writing – review & editing. SM: Writing – review & editing. LM: Writing – review & editing. CT: Writing – review & editing. JR: Writing – review & editing. BC: Writing – review & editing. AL: Writing – review & editing. JK: Writing – review & editing. AB: Writing – review & editing. AJ: Writing – review & editing. EO: Writing – review & editing. AA: Writing – review & editing. AG: Writing – review & editing.
The author(s) declare that financial support was received for the research, authorship, and/or publication of this article. The Emory Goizueta Alzheimer’s disease Research Center (ADRC) was supported by NIH/NIA grant P30AG066511. Supported by the National Center for Advancing Translational Sciences of the National Institutes of Health under Award Number UL1TR002378. The content was solely the responsibility of the authors and does not necessarily represent the official views of the National Institutes of Health.
BC and AJ were employed by ALZpath, Inc.
The remaining authors declare that the research was conducted in the absence of any commercial or financial relationships that could be construed as a potential conflict of interest.
The authors declare that no Generative AI was used in the creation of this manuscript.
All claims expressed in this article are solely those of the authors and do not necessarily represent those of their affiliated organizations, or those of the publisher, the editors and the reviewers. Any product that may be evaluated in this article, or claim that may be made by its manufacturer, is not guaranteed or endorsed by the publisher.
Akinyemi, R. O., Allan, L., Owolabi, M. O., Akinyemi, J. O., Ogbole, G., Ajani, A., et al. (2014). Profile and determinants of vascular cognitive impairment in African stroke survivors: the CogFAST Nigeria Study. J. Neurol. Sci. 346, 241–249. doi: 10.1016/J.JNS.2014.08.042
American Psychiatric Association (2013). Diagnostic and Statistical Manual of Mental Disorders, 5th Edn. Washington, DC: American Psychiatric Association.
Ashton, N. J., Hye, A., Rajkumar, A. P., Leuzy, A., Snowden, S., Suárez-Calvet, M., et al. (2020). An update on blood-based biomarkers for non-Alzheimer neurodegenerative disorders. Nat. Rev. Neurol. 16, 265–284. doi: 10.1038/S41582-020-0348-0
Balasaheb Chavan, A., Rangrao Patil, S., Mansur Patel, A., Vijay Chaugule, S., Krishnat Gharal, S., and Author, C. (2023). A comprehensive review on Alzheimer’s disease its pathogenesis, epidermiology, diagnostics and treatment. J. Res. Appl. Sci. Biotechnol. 2, 66–72. doi: 10.55544/jrasb.2.4.8
Claus, J. J., Staekenborg, S. S., Holl, D. C., Roorda, J. J., Schuur, J., Koster, P., et al. (2017). Practical use of visual medial temporal lobe atrophy cut-off scores in Alzheimer’s disease: validation in a large memory clinic population. Eur. Radiol. 27, 3147–3155. doi: 10.1007/s00330-016-4726-3
Dimtsu Assfaw, A., Schindler, S. E., Morris, J. C., and John Morris, C. C. (2024). Advances in blood biomarkers for Alzheimer disease (AD): a review. Kaohsiung J. Med. Sci. 40, 692–698. doi: 10.1002/kjm2.12870
Dodge, H. H., Zhu, J., Woltjer, R., Nelson, P. T., Bennett, D. A., Cairns, N. J., et al. (2017). Risk of incident clinical diagnosis of Alzheimer’s diseaseâ type dementia attributable to pathologyâ confirmed vascular disease. Alzheimers Dement. 13, 613–623. doi: 10.1016/j.jalz.2016.11.003
Enkirch, S. J., Traschütz, A., Müller, A., Widmann, C. N., Gielen, G. H., Heneka, M. T., et al. (2018). The ERICA score: an MR imaging–based visual scoring system for the assessment of entorhinal cortex atrophy in Alzheimer disease. Radiology 288, 226–333. doi: 10.1148/RADIOL.2018171888
Farombi, T. H., Owolabi, M. O., and Ogunniyi, A. (2016). Falls and their associated risks in Parkinson’s disease patients in nigeria. J. Mov. Disord. 9, 160–165. doi: 10.14802/JMD.16011
Giampietri, L., Belli, E., Beatino, M. F., Giannoni, S., Palermo, G., Campese, N., et al. (2022). Fluid biomarkers in Alzheimer’s disease and other neurodegenerative disorders: toward integrative diagnostic frameworks and tailored treatments. Diagnostics 12:796. doi: 10.3390/diagnostics12040796
Guerchet, M., M’Belesso, P., Mouanga, A. M., Bandzouzi, B., Tabo, A., Houinato, D. S., et al. (2010). Prevalence of dementia in elderly living in two cities of Central Africa: the EDAC Survey. Dement. Geriatr. Cogn. Disord. 30, 261–268. doi: 10.1159/000320247
Hall, K. S., Gao, S., Emsley, C. L., Ogunniyi, A. O., Morgan, O., and Hendrie, H. C. (2000). Community screening interview for dementia (CSI ‘D’); performance in five disparate study sites. Int. J. Geriatr. Psychiatry 15, 521–531. doi: 10.1002/1099-1166(200006)15:6<521::aid-gps182<3.0.co;2-f
Hu, Y., Kirmess, K. M., Meyer, M. R., Rabinovici, G. D., Gatsonis, C., Siegel, B. A., et al. (2022). Assessment of a plasma amyloid probability score to estimate amyloid positron emission tomography findings among adults with cognitive impairment. JAMA Netw. Open 5:e228392. doi: 10.1001/JAMANETWORKOPEN.2022.8392
Igarashi, K. M. (2023). Entorhinal cortex dysfunction in Alzheimer’s disease. Trends Neurosci. 46, 124–136. doi: 10.1016/J.TINS.2022.11.006
Ikanga, J., Hickle, S., Schwinne, M., Epenge, E., Gikelekele, G., Kavugho, I., et al. (2023a). Association between hippocampal volume and African neuropsychology memory tests in adult individuals with probable Alzheimer’s disease in democratic republic of Congo. J. Alzheimers Dis. 96, 395–408. doi: 10.3233/JAD-230206
Ikanga, J., Jean, K., Medina, P., Patel, S. S., Schwinne, M., Epenge, E., et al. (2024). Preliminary reference values for Alzheimer’s disease plasma biomarkers in Congolese individuals with and without Alzheimer’s disease. medRxiv [Preprint] doi: 10.1101/2024.08.06.24311577
Ikanga, J., Reyes, A., Kaba, D., Akilimali, P., Mampunza, S., Epenge, E., et al. (2023b). Prevalence of suspected dementia in a sample of adults living in Kinshasa-Democratic Republic of the Congo. Alzheimers Dement. 19, 3783–3793. doi: 10.1002/ALZ.13003
Imarhiagbe, F., Ogunrin, O., and Ogunniyi, A. (2005). Cognitive performance of hypertensive elderly Nigerians: a case control study. Afr. J. Med. Med. Sci. 34, 269–273.
Jack, C. R. Jr., Scott Andrews, J., Beach, T. G., Buracchio, T., Dunn, B., Graf, A., et al. (2024). Revised criteria for diagnosis and staging of Alzheimer’s disease: Alzheimer’s Association Workgroup. Alzheimers Dement. 20, 5143–5169. doi: 10.1002/ALZ.13859
Jack, C. R., Therneau, T. M., Weigand, S. D., Wiste, H. J., Knopman, D. S., Vemuri, P., et al. (2019). Prevalence of biologically vs clinically defined Alzheimer spectrum entities using the National Institute on Aging–Alzheimer’s Association research framework. JAMA Neurol. 76, 1174–1183. doi: 10.1001/jamaneurol.2019.1971
Janelidze, S., Stomrud, E., Smith, R., Palmqvist, S., Mattsson, N., Airey, D. C., et al. (2020). Cerebrospinal fluid p-tau217 performs better than p-tau181 as a biomarker of Alzheimer’s disease. Nat. Commun. 11:1683. doi: 10.1038/s41467-020-15436-0
Karikari, T. K., Pascoal, T. A., Ashton, N. J., Janelidze, S., Benedet, A. L., Rodriguez, J. L., et al. (2020). Blood phosphorylated tau 181 as a biomarker for Alzheimer’s disease: a diagnostic performance and prediction modelling study using data from four prospective cohorts. Lancet Neurol. 19, 422–433. doi: 10.1016/S1474-4422(20)30071-5
Killiany, R. J., Hyman, B. T., Gomez-Isla, T., Moss, M. B., Kikinis, R., Jolesz, F., et al. (2002). MRI measures of entorhinal cortex vs hippocampus in preclinical AD. Neurology 58, 1188–1196. doi: 10.1212/WNL.58.8.1188
Kirmess, K., Meyer, M., Holubasch, M., Knapik, S. S., Hu, Y., and Jackson, E. N. (2021). The PrecivityADTM test: accurate and reliable LC-MS/MS assays for quantifying plasma amyloid beta 40 and 42 and apolipoprotein E proteotype for the assessment of brain amyloidosis. Clin. Chim. Acta 519, 267–275. doi: 10.1016/j.cca.2021.05.011
Kowall, B., and Rathmann, W. (2013). HbA1c for diagnosis of type 2 diabetes. Is there an optimal cut point to assess high risk of diabetes complications, and how well does the 6.5% cutoff perform? Diabetes Metab. Syndr. Obes. 6, 6–477. doi: 10.2147/DMSO.S39093
Li, J., Somers, V. K., Gao, X., Chen, Z., Ju, J., Lin, Q., et al. (2021). Evaluation of optimal diastolic blood pressure range among adults with treated systolic blood pressure less than 130 mm Hg. JAMA Netw. Open 4:2037554. doi: 10.1001/jamanetworkopen.2020.37554
Liss, J. L., Seleri Assunc, S., Cummings, J., Atri, A., Geldmacher, D. S., Candela, S. F., et al. (2021). Practical recommendations for timely, accurate diagnosis of symptomatic Alzheimer’s disease (MCI and dementia) in primary care: a review and synthesis. J. Intern. Med. 290, 310–334. doi: 10.1111/joim.13244
MacLin, J. M. A., Wang, T., and Xiao, S. (2019). Biomarkers for the diagnosis of Alzheimer’s disease, dementia Lewy body, frontotemporal dementia and vascular dementia. Gen. Psychiatr. 32:e100054. doi: 10.1136/GPSYCH-2019-100054
Malek-Ahmadi, M., and Sabbagh, M. N. (2015). Development and validation of the Alzheimer’s Questionnaire (AQ). J. Nat. Sci. 1:e104.
Mattsson, N., Andreasson, U., Zetterberg, H., Blennow, K., and Alzheimer’s Disease Neuroimaging Initiative. (2017). Association of plasma neurofilament light with neurodegeneration in patients with Alzheimer disease. JAMA Neurol. 74, 557–566. doi: 10.1001/JAMANEUROL.2016.6117
Mattsson, N., Cullen, N. C., Andreasson, U., Zetterberg, H., and Blennow, K. (2019). Association between longitudinal plasma neurofilament light and neurodegeneration in patients with Alzheimer disease. JAMA Neurol. 76, 791–799. doi: 10.1001/JAMANEUROL.2019.0765
Nakamura, A., Kaneko, N., Villemagne, V. L., Kato, T., Doecke, J., Doré, V., et al. (2018). High performance plasma amyloid-β biomarkers for Alzheimer’s disease. Nature 554, 249–254. doi: 10.1038/nature25456
Nantsupawat, N., Booncharoen, A., Wisetborisut, A., Jiraporncharoen, W., Pinyopornpanish, K., Chutarattanakul, L., et al. (2019). Appropriate total cholesterol cut-offs for detection of abnormal LDL cholesterol and non-HDL cholesterol among low cardiovascular risk population. Lipids Health Dis. 18:28. doi: 10.1186/s12944-019-0975-x
Nichols, E., Steinmetz, J., Vollset, S. E., Fukutaki, K., Chalek, J., and Abd-Allah, F. (2022). Estimation of the global prevalence of dementia in 2019 and forecasted prevalence in 2050: an analysis for the Global Burden of Disease Study 2019. Lancet Public Health 7, e105–e125.
No author list (2023). Alzheimer’s disease facts and figures. Alzheimers Dement. 19, 1598–1695. doi: 10.1002/ALZ.13016
Oeckl, P., Anderl-Straub, S., Von Arnim, C. A. F., Baldeiras, I., Diehl-Schmid, J., Grimmer, T., et al. (2022). Serum GFAP differentiates Alzheimer’s disease from frontotemporal dementia and predicts MCI-to-dementia conversion. J. Neurol. Neurosurg. Psychiatry 93, 659–667. doi: 10.1136/JNNP-2021-328547
Ogbimi, E. M., Akemokwe, F. M., and Ogunrin, O. (2023). Frequency, pattern and predictors of cognitive impairments in patients with Parkinson’s disease using the community screening instrument for dementia. Front. Hum. Neurosci. 17:1126526. doi: 10.3389/FNHUM.2023.1126526/FULL
Palmqvist, S., Janelidze, S., Stomrud, E., Zetterberg, H., Karl, J., Zink, K., et al. (2019). Performance of fully automated plasma assays as screening tests for Alzheimer disease–related β-amyloid status. JAMA Neurol. 76, 1060–1069. doi: 10.1001/jamaneurol.2019.1632
Palmqvist, S., Tideman, P., Mattsson-Carlgren, N., Schindler, S. E., Smith, R., Ossenkoppele, R., et al. (2024). Blood biomarkers to detect Alzheimer disease in primary care and secondary care. JAMA 332, 1245–1257. doi: 10.1001/JAMA.2024.13855
Slot, R. E. R., Sikkes, S. A. M., Berkhof, J., Brodaty, H., Buckley, R., Cavedo, E., et al. (2019). Subjective cognitive decline and rates of incident Alzheimer’s disease and non–Alzheimer’s disease dementia. Alzheimers Dement. 15, 465–476. doi: 10.1016/J.JALZ.2018.10.003
Thijssen, E. H., La Joie, R., Wolf, A., Strom, A., Wang, P., Iaccarino, L., et al. (2020). Diagnostic value of plasma phosphorylated tau181 in Alzheimer’s disease and frontotemporal lobar degeneration. Nat. Med. 26:387. doi: 10.1038/S41591-020-0762-2
Van Harten, A. C., Wiste, H. J., Weigand, S. D., Mielke, M. M., Kremers, W. K., Eichenlaub, U., et al. (2021). Detection of Alzheimer’s disease amyloid beta 1-42, p-tau, and t-tau assays. Alzheimers Dement. 18, 635–644. doi: 10.1002/alz.12406
Wahlund, L. O., Barkhof, F., Fazekas, F., Bronge, L., Augustin, M., Sjögren, M., et al. (2001). A new rating scale for age-related white matter changes applicable to MRI and CT. Stroke 32, 1318–1322. doi: 10.1161/01.STR.32.6.1318
West, T., Kirmess, K. M., Meyer, M. R., Holubasch, M. S., Knapik, S. S., Hu, Y., et al. (2021). A blood-based diagnostic test incorporating plasma Aβ42/40 ratio, ApoE proteotype, and age accurately identifies brain amyloid status: findings from a multi cohort validity analysis. Mol. Neurodegener. 16:30. doi: 10.1186/S13024-021-00451-6
Whelton, P. K., Carey, R. M., Aronow, W. S., Casey, D. E., Collins, K. J., Dennison Himmelfarb, C., et al. (2017). ACC/AHA/AAPA/ABC/ACPM/AGS/APHA/ASH/ASPC/NMA/PCNA guideline for the prevention, Detection, evaluation, and management of high blood pressure in adults: a Report of the American College of Cardiology/American heart Association. Task force on clinical practice guidelines. J. Am. Coll. Cardiol. 7, 68–74.
Keywords: dementia, Democratic Republic of the Congo, cognition, neuroimaging, biomarkers
Citation: Ikanga J, Patel SS, Schwinne M, Obenauf C, Epenge E, Gikelekele G, Tshengele N, Kavugho I, Mampunza S, Mananga L, Teunissen CE, Rojas JC, Chan B, Lario Lago A, Kramer JH, Boxer AL, Jeromin A, Omba E, Alonso A and Gross AL (2025) Exploring cognitive and neuroimaging profiles of dementia subtypes of individuals with dementia in the Democratic Republic of Congo. Front. Aging Neurosci. 17:1552348. doi: 10.3389/fnagi.2025.1552348
Received: 27 December 2024; Accepted: 20 January 2025;
Published: 12 February 2025.
Edited by:
Enzo Emanuele, 2E Science, ItalyReviewed by:
Udunna Anazodo, McGill University Health Centre, CanadaCopyright © 2025 Ikanga, Patel, Schwinne, Obenauf, Epenge, Gikelekele, Tshengele, Kavugho, Mampunza, Mananga, Teunissen, Rojas, Chan, Lario Lago, Kramer, Boxer, Jeromin, Omba, Alonso and Gross. This is an open-access article distributed under the terms of the Creative Commons Attribution License (CC BY). The use, distribution or reproduction in other forums is permitted, provided the original author(s) and the copyright owner(s) are credited and that the original publication in this journal is cited, in accordance with accepted academic practice. No use, distribution or reproduction is permitted which does not comply with these terms.
*Correspondence: Jean Ikanga, amlrYW5nYUBlbW9yeS5lZHU=
Disclaimer: All claims expressed in this article are solely those of the authors and do not necessarily represent those of their affiliated organizations, or those of the publisher, the editors and the reviewers. Any product that may be evaluated in this article or claim that may be made by its manufacturer is not guaranteed or endorsed by the publisher.
Research integrity at Frontiers
Learn more about the work of our research integrity team to safeguard the quality of each article we publish.